Have a language expert improve your writing
Run a free plagiarism check in 10 minutes, generate accurate citations for free.
- Knowledge Base
Methodology
- Random Assignment in Experiments | Introduction & Examples
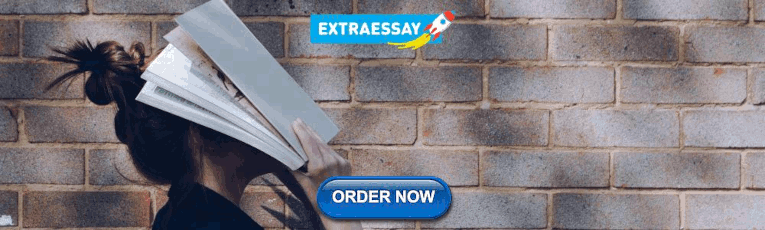
Random Assignment in Experiments | Introduction & Examples
Published on March 8, 2021 by Pritha Bhandari . Revised on June 22, 2023.
In experimental research, random assignment is a way of placing participants from your sample into different treatment groups using randomization.
With simple random assignment, every member of the sample has a known or equal chance of being placed in a control group or an experimental group. Studies that use simple random assignment are also called completely randomized designs .
Random assignment is a key part of experimental design . It helps you ensure that all groups are comparable at the start of a study: any differences between them are due to random factors, not research biases like sampling bias or selection bias .
Table of contents
Why does random assignment matter, random sampling vs random assignment, how do you use random assignment, when is random assignment not used, other interesting articles, frequently asked questions about random assignment.
Random assignment is an important part of control in experimental research, because it helps strengthen the internal validity of an experiment and avoid biases.
In experiments, researchers manipulate an independent variable to assess its effect on a dependent variable, while controlling for other variables. To do so, they often use different levels of an independent variable for different groups of participants.
This is called a between-groups or independent measures design.
You use three groups of participants that are each given a different level of the independent variable:
- a control group that’s given a placebo (no dosage, to control for a placebo effect ),
- an experimental group that’s given a low dosage,
- a second experimental group that’s given a high dosage.
Random assignment to helps you make sure that the treatment groups don’t differ in systematic ways at the start of the experiment, as this can seriously affect (and even invalidate) your work.
If you don’t use random assignment, you may not be able to rule out alternative explanations for your results.
- participants recruited from cafes are placed in the control group ,
- participants recruited from local community centers are placed in the low dosage experimental group,
- participants recruited from gyms are placed in the high dosage group.
With this type of assignment, it’s hard to tell whether the participant characteristics are the same across all groups at the start of the study. Gym-users may tend to engage in more healthy behaviors than people who frequent cafes or community centers, and this would introduce a healthy user bias in your study.
Although random assignment helps even out baseline differences between groups, it doesn’t always make them completely equivalent. There may still be extraneous variables that differ between groups, and there will always be some group differences that arise from chance.
Most of the time, the random variation between groups is low, and, therefore, it’s acceptable for further analysis. This is especially true when you have a large sample. In general, you should always use random assignment in experiments when it is ethically possible and makes sense for your study topic.
Receive feedback on language, structure, and formatting
Professional editors proofread and edit your paper by focusing on:
- Academic style
- Vague sentences
- Style consistency
See an example
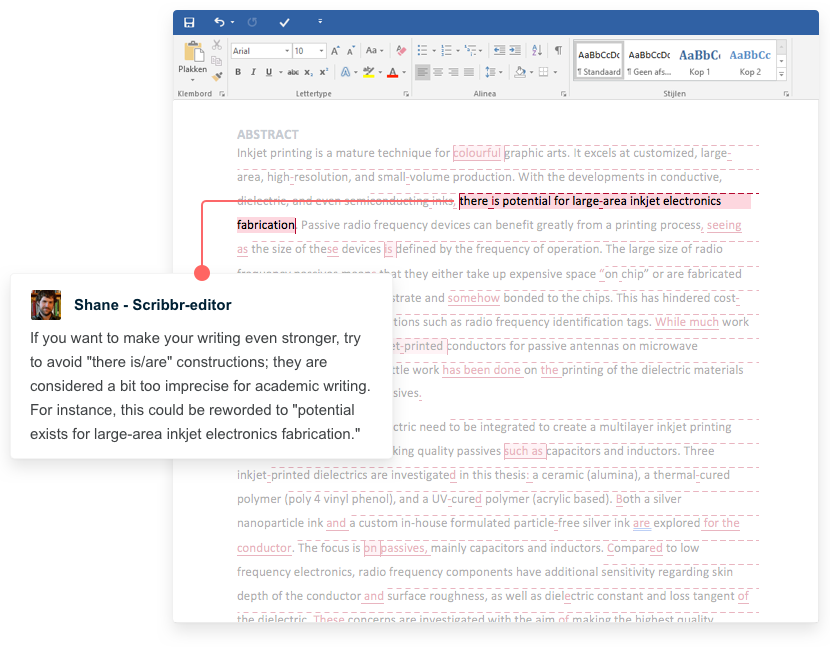
Random sampling and random assignment are both important concepts in research, but it’s important to understand the difference between them.
Random sampling (also called probability sampling or random selection) is a way of selecting members of a population to be included in your study. In contrast, random assignment is a way of sorting the sample participants into control and experimental groups.
While random sampling is used in many types of studies, random assignment is only used in between-subjects experimental designs.
Some studies use both random sampling and random assignment, while others use only one or the other.
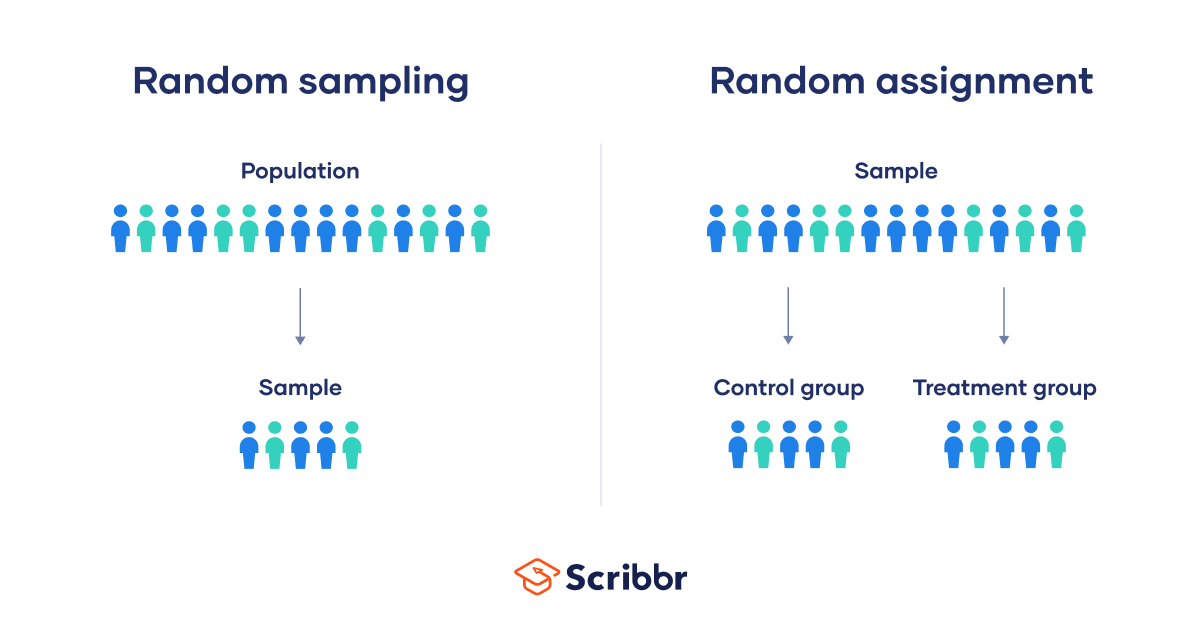
Random sampling enhances the external validity or generalizability of your results, because it helps ensure that your sample is unbiased and representative of the whole population. This allows you to make stronger statistical inferences .
You use a simple random sample to collect data. Because you have access to the whole population (all employees), you can assign all 8000 employees a number and use a random number generator to select 300 employees. These 300 employees are your full sample.
Random assignment enhances the internal validity of the study, because it ensures that there are no systematic differences between the participants in each group. This helps you conclude that the outcomes can be attributed to the independent variable .
- a control group that receives no intervention.
- an experimental group that has a remote team-building intervention every week for a month.
You use random assignment to place participants into the control or experimental group. To do so, you take your list of participants and assign each participant a number. Again, you use a random number generator to place each participant in one of the two groups.
To use simple random assignment, you start by giving every member of the sample a unique number. Then, you can use computer programs or manual methods to randomly assign each participant to a group.
- Random number generator: Use a computer program to generate random numbers from the list for each group.
- Lottery method: Place all numbers individually in a hat or a bucket, and draw numbers at random for each group.
- Flip a coin: When you only have two groups, for each number on the list, flip a coin to decide if they’ll be in the control or the experimental group.
- Use a dice: When you have three groups, for each number on the list, roll a dice to decide which of the groups they will be in. For example, assume that rolling 1 or 2 lands them in a control group; 3 or 4 in an experimental group; and 5 or 6 in a second control or experimental group.
This type of random assignment is the most powerful method of placing participants in conditions, because each individual has an equal chance of being placed in any one of your treatment groups.
Random assignment in block designs
In more complicated experimental designs, random assignment is only used after participants are grouped into blocks based on some characteristic (e.g., test score or demographic variable). These groupings mean that you need a larger sample to achieve high statistical power .
For example, a randomized block design involves placing participants into blocks based on a shared characteristic (e.g., college students versus graduates), and then using random assignment within each block to assign participants to every treatment condition. This helps you assess whether the characteristic affects the outcomes of your treatment.
In an experimental matched design , you use blocking and then match up individual participants from each block based on specific characteristics. Within each matched pair or group, you randomly assign each participant to one of the conditions in the experiment and compare their outcomes.
Sometimes, it’s not relevant or ethical to use simple random assignment, so groups are assigned in a different way.
When comparing different groups
Sometimes, differences between participants are the main focus of a study, for example, when comparing men and women or people with and without health conditions. Participants are not randomly assigned to different groups, but instead assigned based on their characteristics.
In this type of study, the characteristic of interest (e.g., gender) is an independent variable, and the groups differ based on the different levels (e.g., men, women, etc.). All participants are tested the same way, and then their group-level outcomes are compared.
When it’s not ethically permissible
When studying unhealthy or dangerous behaviors, it’s not possible to use random assignment. For example, if you’re studying heavy drinkers and social drinkers, it’s unethical to randomly assign participants to one of the two groups and ask them to drink large amounts of alcohol for your experiment.
When you can’t assign participants to groups, you can also conduct a quasi-experimental study . In a quasi-experiment, you study the outcomes of pre-existing groups who receive treatments that you may not have any control over (e.g., heavy drinkers and social drinkers). These groups aren’t randomly assigned, but may be considered comparable when some other variables (e.g., age or socioeconomic status) are controlled for.
Here's why students love Scribbr's proofreading services
Discover proofreading & editing
If you want to know more about statistics , methodology , or research bias , make sure to check out some of our other articles with explanations and examples.
- Student’s t -distribution
- Normal distribution
- Null and Alternative Hypotheses
- Chi square tests
- Confidence interval
- Quartiles & Quantiles
- Cluster sampling
- Stratified sampling
- Data cleansing
- Reproducibility vs Replicability
- Peer review
- Prospective cohort study
Research bias
- Implicit bias
- Cognitive bias
- Placebo effect
- Hawthorne effect
- Hindsight bias
- Affect heuristic
- Social desirability bias
In experimental research, random assignment is a way of placing participants from your sample into different groups using randomization. With this method, every member of the sample has a known or equal chance of being placed in a control group or an experimental group.
Random selection, or random sampling , is a way of selecting members of a population for your study’s sample.
In contrast, random assignment is a way of sorting the sample into control and experimental groups.
Random sampling enhances the external validity or generalizability of your results, while random assignment improves the internal validity of your study.
Random assignment is used in experiments with a between-groups or independent measures design. In this research design, there’s usually a control group and one or more experimental groups. Random assignment helps ensure that the groups are comparable.
In general, you should always use random assignment in this type of experimental design when it is ethically possible and makes sense for your study topic.
To implement random assignment , assign a unique number to every member of your study’s sample .
Then, you can use a random number generator or a lottery method to randomly assign each number to a control or experimental group. You can also do so manually, by flipping a coin or rolling a dice to randomly assign participants to groups.
Cite this Scribbr article
If you want to cite this source, you can copy and paste the citation or click the “Cite this Scribbr article” button to automatically add the citation to our free Citation Generator.
Bhandari, P. (2023, June 22). Random Assignment in Experiments | Introduction & Examples. Scribbr. Retrieved September 3, 2024, from https://www.scribbr.com/methodology/random-assignment/
Is this article helpful?
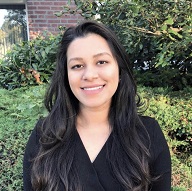
Pritha Bhandari
Other students also liked, guide to experimental design | overview, steps, & examples, confounding variables | definition, examples & controls, control groups and treatment groups | uses & examples, get unlimited documents corrected.
✔ Free APA citation check included ✔ Unlimited document corrections ✔ Specialized in correcting academic texts

An official website of the United States government
The .gov means it’s official. Federal government websites often end in .gov or .mil. Before sharing sensitive information, make sure you’re on a federal government site.
The site is secure. The https:// ensures that you are connecting to the official website and that any information you provide is encrypted and transmitted securely.
- Publications
- Account settings
- My Bibliography
- Collections
- Citation manager
Save citation to file
Email citation, add to collections.
- Create a new collection
- Add to an existing collection
Add to My Bibliography
Your saved search, create a file for external citation management software, your rss feed.
- Search in PubMed
- Search in NLM Catalog
- Add to Search
Randomization and allocation concealment: a practical guide for researchers
Affiliation.
- 1 Northern Clinical School, Royal North Shore Hospital-ICU, University of Sydney, St Leonards, NSW, Australia. [email protected]
- PMID: 16139163
- DOI: 10.1016/j.jcrc.2005.04.005
Although the randomized controlled trial is the most important tool currently available to objectively assess the impact of new treatments, the act of randomization itself is often poorly conducted and incompletely reported. The primary purpose of randomizing patients into treatment arms is to prevent researchers, clinicians, and patients from predicting, and thus influencing, which patients will receive which treatments. This important source of bias can be eliminated by concealing the upcoming allocation sequence from researchers and participants. Although there are many approaches to randomization that are known to effectively conceal the randomization sequence, the use of sequentially numbered, opaque sealed envelopes (SNOSE) is both cheap and effective. The purpose of this tutorial is to describe a step-by-step process for the preparation of SNOSE. We will outline how to prepare SNOSE to preserve allocation concealment in a trial that (a) uses unrestricted (simple) randomization, (b) stratifies randomization on one factor, (c) uses permuted blocks and, and (d) is conducted at more than 1 study site.
PubMed Disclaimer
- Varying the block size does not conceal the allocation. Berger VW. Berger VW. J Crit Care. 2006 Jun;21(2):229; author reply 229-30. doi: 10.1016/j.jcrc.2006.01.002. J Crit Care. 2006. PMID: 16769475 No abstract available.
Similar articles
- Eliminating bias in randomized controlled trials: importance of allocation concealment and masking. Viera AJ, Bangdiwala SI. Viera AJ, et al. Fam Med. 2007 Feb;39(2):132-7. Fam Med. 2007. PMID: 17273956
- Using scratch card technology for random allocation concealment in a clinical trial with a crossover design. Beksinska ME, Joanis C, Smit JA, Pienaar J, Piaggio G. Beksinska ME, et al. Clin Trials. 2013 Feb;10(1):125-30. doi: 10.1177/1740774512465496. Epub 2012 Nov 27. Clin Trials. 2013. PMID: 23188890 Clinical Trial.
- Different methods of allocation to groups in randomized trials are associated with different levels of bias. A meta-epidemiological study. Herbison P, Hay-Smith J, Gillespie WJ. Herbison P, et al. J Clin Epidemiol. 2011 Oct;64(10):1070-5. doi: 10.1016/j.jclinepi.2010.12.018. Epub 2011 Apr 6. J Clin Epidemiol. 2011. PMID: 21474279 Review.
- Quantification methods were developed for selection bias by predictability of allocations with unequal block randomization. Dupin-Spriet T, Fermanian J, Spriet A. Dupin-Spriet T, et al. J Clin Epidemiol. 2005 Dec;58(12):1269-76. doi: 10.1016/j.jclinepi.2005.04.008. J Clin Epidemiol. 2005. PMID: 16291471
- Randomization procedures in orthopaedic trials. Randelli P, Arrigoni P, Lubowitz JH, Cabitza P, Denti M. Randelli P, et al. Arthroscopy. 2008 Jul;24(7):834-8. doi: 10.1016/j.arthro.2008.01.011. Epub 2008 Mar 21. Arthroscopy. 2008. PMID: 18589273 Review.
- The Feasibility of the Diabetes Self-Management Coaching Program in Primary Care: A Mixed-Methods Randomized Controlled Feasibility Trial. Yehualashet FA, Kessler D, Bizuneh SM, Donnelly C. Yehualashet FA, et al. Int J Environ Res Public Health. 2024 Aug 6;21(8):1032. doi: 10.3390/ijerph21081032. Int J Environ Res Public Health. 2024. PMID: 39200642 Free PMC article. Clinical Trial.
- Efficiency of Foot and Ankle Surgeries Completed on the Preoperative Stretcher vs Operating Room Table: A Randomized Controlled Trial. Parker EB, Smith JT, Lausé G, Bluman EM. Parker EB, et al. Foot Ankle Orthop. 2024 Aug 26;9(3):24730114241270272. doi: 10.1177/24730114241270272. eCollection 2024 Jul. Foot Ankle Orthop. 2024. PMID: 39193449 Free PMC article.
- Improving Diabetes Equity and Advancing Care (IDEA) to optimize team-based care at a safety-net health system for Black and Latine patients living with diabetes: study protocol for a sequential, multiple assignment, randomized trial. Jacobs J, Labellarte P, Margellos-Anast H, Garcia L, Qeadan F, Tingey B, Barnick K, Dougherty A, Wagener C. Jacobs J, et al. Trials. 2024 Jul 24;25(1):504. doi: 10.1186/s13063-024-08346-9. Trials. 2024. PMID: 39049044 Free PMC article.
- A 12-month randomized controlled trial to assess the efficacy of revitalization of retreated mature incisors with periapical radiolucency in adolescents. Elheeny AAH, Hussien OS, Abdelmotelb MA, ElMakawi YM, Wahba NKO. Elheeny AAH, et al. Sci Rep. 2024 Jul 16;14(1):16366. doi: 10.1038/s41598-024-66305-5. Sci Rep. 2024. PMID: 39013938 Clinical Trial.
- Effects of pain education on disability, pain, quality of life, and self-efficacy in chronic low back pain: A randomized controlled trial. Sidiq M, Muzaffar T, Janakiraman B, Masoodi S, Vasanthi RK, Ramachandran A, Bansal N, Chahal A, Kashoo FZ, Rizvi MR, Sharma A, Rai RH, Verma R, Sharma M, Alam S, Vajrala KR, Sharma J, Muthukrishnan R. Sidiq M, et al. PLoS One. 2024 May 28;19(5):e0294302. doi: 10.1371/journal.pone.0294302. eCollection 2024. PLoS One. 2024. PMID: 38805446 Free PMC article. Clinical Trial.
Publication types
- Search in MeSH
LinkOut - more resources
Full text sources.
- Elsevier Science
- Ovid Technologies, Inc.
- W.B. Saunders
- Citation Manager
NCBI Literature Resources
MeSH PMC Bookshelf Disclaimer
The PubMed wordmark and PubMed logo are registered trademarks of the U.S. Department of Health and Human Services (HHS). Unauthorized use of these marks is strictly prohibited.
Europe PMC requires Javascript to function effectively.
Either your web browser doesn't support Javascript or it is currently turned off. In the latter case, please turn on Javascript support in your web browser and reload this page.

- Digital Health , Health Sciences Research
Conducting the Study: Randomization and Allocation
Learn More about our Services and how can we help you with your research!
- Introduction
Minimization
Study designs: basics of research.
Conducting a medical study requires precise planning and effective management. One of the crucial aspects experts must consider, revolves around the participants – the main focus of digital health research. Recruitment procedures, sample size, randomization, and allocation are some of the factors that require careful consideration before the actual start of the study.
Since medical professionals cannot test the whole population due to financial, ethical and time limitations, a representative sample is often needed. At the same time, there’s always a chance that the chosen sample won’t represent the population of interest (Peat, 2011). At the end, when only a portion of the population is studied, there’s always a risk that this particular group does not accurately represent the target population.
Randomization and Allocation
To minimize errors and bias, randomization has become a sufficient way to select participants. Random allocation is another paramount method used to assign participants to different research groups (Peat, 2011). In other words, randomization is a practice that’s used to achieve generalizability, while random allocation – is to minimize confounders and eliminate systematic bias. Thus, random selection and random allocation are the most efficient methods, each with its challenges and advantages.
For instance, random allocation can lead to unbalanced and balanced groups (Peat, 2011). When it comes to unbalanced samples, two methods can be employed: simple randomization (via a random number table or a computer-generated sequence) or quasi-randomization (through a random number; e.g., age). When balanced groups are needed, several methods can be employed. Restricted randomization is one of the effective methods, which is achieved by sealed envelopes. Block randomization, on the other hand, is achieved in small blocks. Another technique is replacement allocation which requires experts to reject sequences when they exceed the pre-specified balance. Dynamically balanced randomization is also vital, and it involves forced allocation to unbalanced groups. Bias coin randomization, which implies that probability is changed in unbalanced groups, can be employed as well. Last but not the least, minimization is a popular method that requires allocation by prognostic factors when there are unbalanced groups
Random Selection and Random Allocation in Details
As explained above, random selection is the most beneficial way to ensure representativeness and generalizability. Random selection can be made from an ordered list. This list can include vital indicators (e.g., towns) which have a unique number. Consequently, these unique numbers can be randomly selected from the list. In studies with less than 100 participants, tables prove to be the most effective way to select subjects. When using a table, the pattern of reading it is important: the table can be read by row, by column or by block (Peat, 2011). After experts have decided on the pattern, a random start should be chosen, and a random sequence should be used to select numbers. Note that any number repeated should be discarded. For studies with more than 100 participants, computer software to generate random number sequences is suggested. Again, since duplicates should be excluded, a longer list is recommended to ensure enough numbers.
Random selection is vital, and so is random allocation. Allocation methods, such as the ones described above, are used to assign participants to two or more study groups (e.g., treatment and control groups). Usually, the allocation is used to remove confounders. Note that experts can control for confounders in the analysis (via post-hoc methods and multivariate data); however, it’s better to do that at the design stage of the study (Peat, 2011). The most effective allocation can be achieved via an unpredictable allocation sequence. To avoid bias, staff should be blinded to the results. Allocation concealment, or when staff does not know what the next intervention allocation will be, is also paramount to diminish selection bias. Balanced groups are the most desired outcome of allocation. Nevertheless, the main aspect to consider is to ensure that each subject has the chance to be allocated to any group and to ensure that differences in groups are due to treatment only. A good practice is to keep the allocation code unavailable until subjects are eligible or until they consent.
Simple Randomization
Another important difference between studies is their qualitative or quantitate nature (Moffatt, 2006). While quantitative methods rely on conventional data collection and statistical procedures, qualitative studies involve open questions and other in-depth methods, such as interviews with patients.
As mentioned above, quantitative studies help researchers collect data and transform it into statistics. Such studies are well structured and can include large samples. As a result, they are widely used in research and medical practice.
On the other hand, qualitative studies can help researchers gain insights and generate testable hypotheses. They can be used parallel to quantitative studies to explore patients’ feelings, attitudes towards a new treatment, and personal tactics to cope with a disease.
Simple randomization is one of the most popular methods used to select and assign subjects (Peat, 2011). It’s also called complete unbalanced or unrestricted randomization. In fact, simple randomization is the best method to perform random allocation. It can be achieved by tossing a coin or by selecting random numbers.
Note that when a random number is taken from a table, experts need to decide how to use it. For example, if a team gets the following numbers:
they can use the following combinations:
- 5, 1, 0, 2, 2
On top of that, researchers can use either the first digits of the selected numbers:
or only the last ones:
After the selection of numbers, the subjects are divided into groups.
Simple randomization is a great method that balances prognostic factors. However, it can lead to unbalanced groups. This can become problematic in small studies or across different research centers. Unfortunately, small numbers lack the statistical power to show clinically significant results.
Quasi-randomization
Quasi-randomization is another popular method, which relies on a systematic assignment achieved by essential indicators, such as date of birth or medical number (Kim et al., 2017). Since this method is not exactly random, experts call it quasi-randomization. Note that alternation is another type of quasi-randomization: it’s achieved by following the order via which the subjects were included in the study.
Quasi-randomization may be prone to selection bias and lack of concealment. Another disadvantage of this method is that there’s no balance of confounders or groups.
Block Randomization
Block randomization is a method in which selection and allocation are done by small groups, called blocks (Peat, 2011). This method is beneficial in large studies or multi-centered trials, and also during simple and stratified randomization. Block randomization is used to assure balanced groups. Note that to be effective, blocks must generate all possible combinations.
For example, for a block size of four and two treatment groups (A, B), there are six options:
Blocks should be numbered, after which a number will be selected randomly.
On the other hand, for a group of three for three treatments (A, B, C), the following combinations will be valid:
After forming the blocks, experts need to choose a random order. Let’s look at the following random sequence: 6, 2, 3, 6, 1, etc. In this case, the research team will start with blocks CBA, ACB, and so on and on.
However, the block size can ruin concealment as experts may guess the order at the later stages of research. To minimize bias, the block size can be changed during the study (Peat, 2011).
Another challenge is the need for large blocks. In large blocks, experts may face too many combinations. Note, however, that if there are large blocks but only two treatment groups, random randomization can be used to help experts allocate subjects.
Replacement Randomization
When it comes to selection and allocation, unbalanced groups may distort findings. Thus, replacement randomization is an effective method to guarantee balanced groups. The maximum imbalance should be decided before the study, and new sequences will be continuously generated until the planned criteria are met (Peat, 2011).
However, replacement randomization can become unblinded at the later stages of research. Simply because when the group size increases and the sample decreases, more and more replacements will be needed. For this reason, this method is suitable only in small studies with a few groups, including stratified trials . Yet, replacement randomization guarantees an upper limit of imbalance, and it also provides unpredictable sequences, which is an advantage over other methods, such as block randomization.
Biased Coin Randomization
Biased coin randomization is a popular method which is also known as adaptive randomization. This technique follows the probability of assigning subjects to different treatment groups to the point when the groups become unbalanced. This means that the probability of assigning subjects to small groups increases to maintain balance (Peat, 2011).
In particular, biased coin randomization can be used when the imbalance between groups exceeds a specific number, which is specified before the study.
Minimization is another effective method, which as explained above, is used to ensure a balance of prognostic factors (Peat, 2011). This method is critical for balancing numbers over two and more characteristics. In fact, this is crucial in small studies when differences in confounders can occur just by chance. It’s also vital in large studies – when imbalances may reduce the statistical power of small differences. Note that in minimization, the number of subjects is updated continuously.
It’s interesting to mention that the odds of entry in a group can follow the biased coin method described above; as a result, patients can be allocated to the smaller group. If there’s an equal number, then, simple randomization can be employed.
Dynamic Balanced Randomization
Dynamically balanced randomization is a method that is a variation of replacement randomization (Peat, 2011). In other words, when the pre-specified imbalance has been reached, the next subjects are allocated to the smaller group. This method is beneficial in studies in which randomization by strata is needed. A disadvantage of dynamically balanced randomization, though, is that there’s a risk of ruining concealment. Nevertheless, this research technique is less prone to bias compared to block randomization; also, there’s better protection against imbalance when compared to minimization.
Unequal Randomization
In medical research, equal numbers are the most desired and effective way to achieve meaningful results. Thus, as described above, there are many methods that help experts balance groups during randomization and allocation (Peat, 2011).
However, equal numbers are not always the ultimate solution. For instance, when a new and an old treatment must be compared, more subjects must be randomized in the new treatment group in order to show significant differences (both statistical and clinical differences). In this case, the ratio between new and old treatments can be 2:1 or 3:2. Note that the methods to select and allocate subjects include all the techniques described above (Peat, 2011).
Randomization in Clusters
Last but not the least, epidemiological studies also need some special consideration. When experts need to measure the prevalence of a disease or mortality rates within a community, clusters – not individuals – should be the focus of analysis. For instance, when experts should select children from a particular population, schools must be randomized – not individual students.
However, this method does not account for cultural differences between the chosen communities. There will be a difference between rural and city schools, for example. This can lead to a significant loss of efficiency. However, randomization in clusters can be beneficial in cases when the number of practices is substantial and the number of subjects small. In fact, to reach an adequate sample size, the number of practices and interventions must be considered – not the number of individuals.
Randomization: Conclusion
Randomization and allocation allow experts to exercise control over any clinical trial, eliminate bias, balance groups, and confounders, and give each patient an equal chance of receiving the same treatment (Suresh, 2011). Therefore, randomization is a fundamental part of medical research and sound management practices.
Kim, J., Kim, T., In, J., Lee, D., Lee, S., & Kang, H. (2017). Assessment of risk of bias in quasi-randomized controlled trials and randomized controlled trials reported in the Korean Journal of Anesthesiology between 2010 and 2016. Korean Journal of Anaesthesiology, 70(5), 511-519.
Peat, J. (2011). Conducting the Study. Health Science Research, SAGE Publications, Ltd.
Suresh, K. (2011). An overview of randomization techniques: An unbiased assessment of outcome in clinical research. Journal of Human Reproductive Sciences, 4(1), 8-11.
See more of Our Posts
Digital health category.
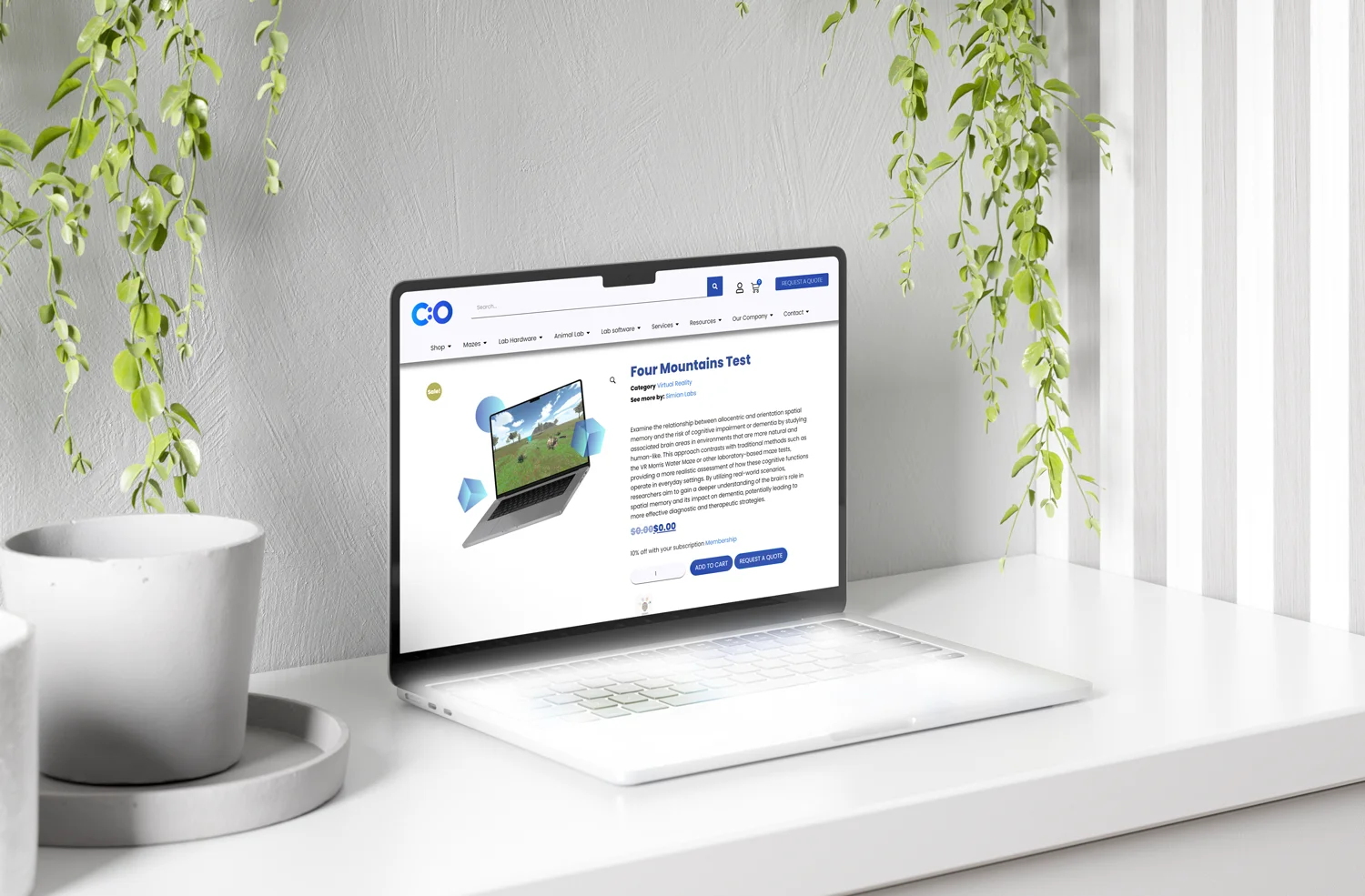
History and Early Tests Leading to the Four Mountains Test
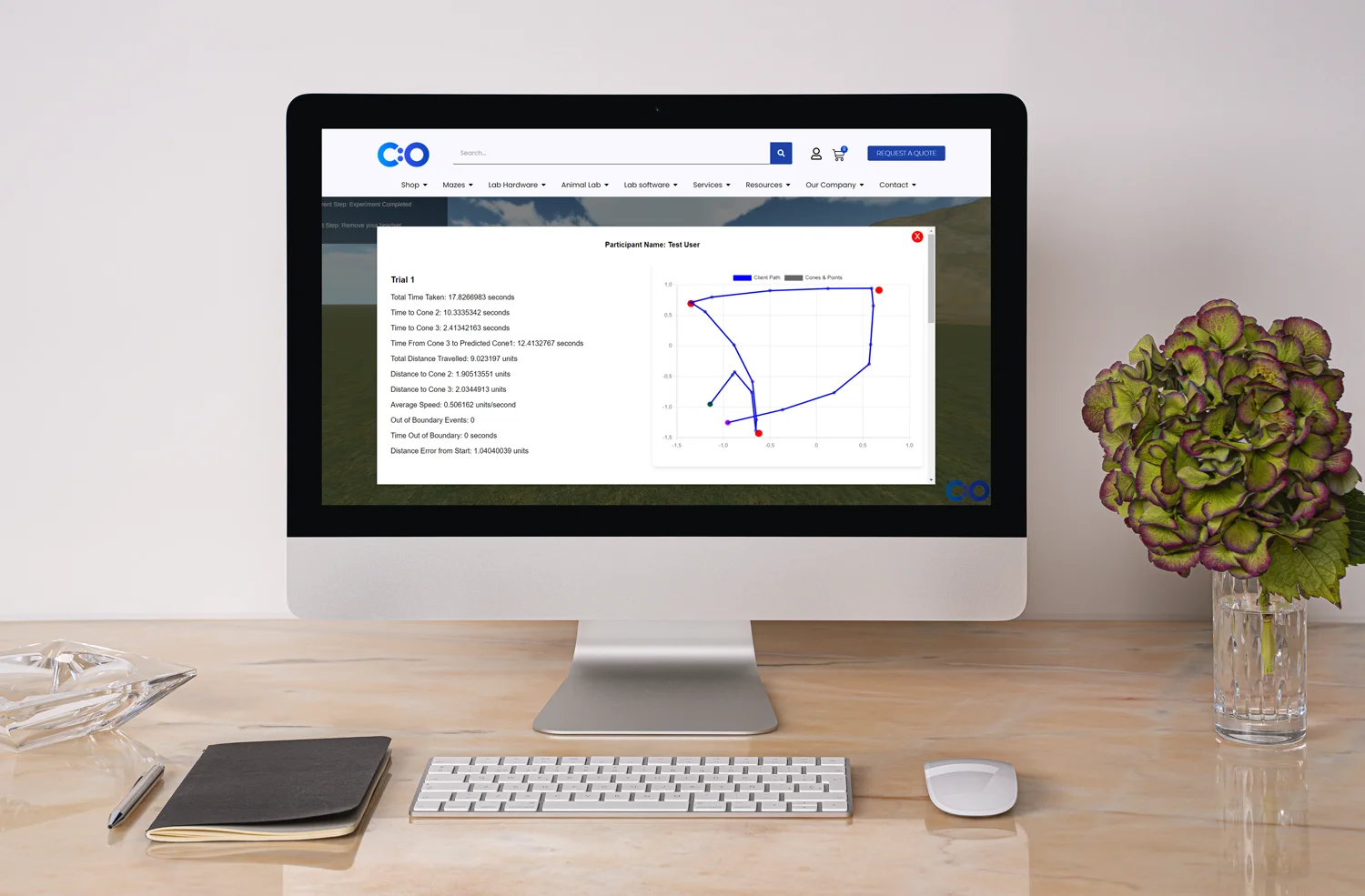
Analyzing Data from the Four Mountains Test
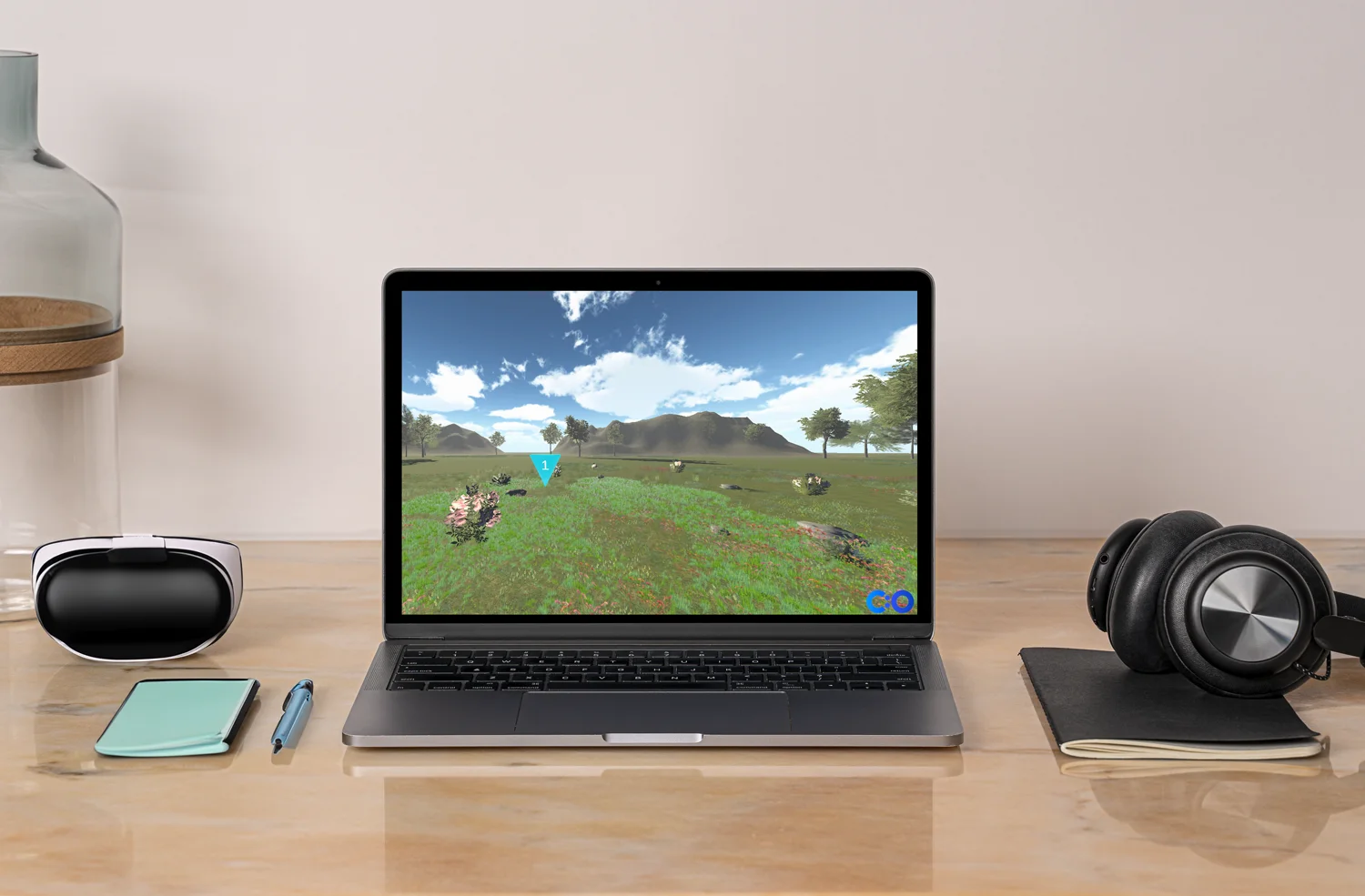
Understanding the Four Mountains Test: A Scientific Exploration
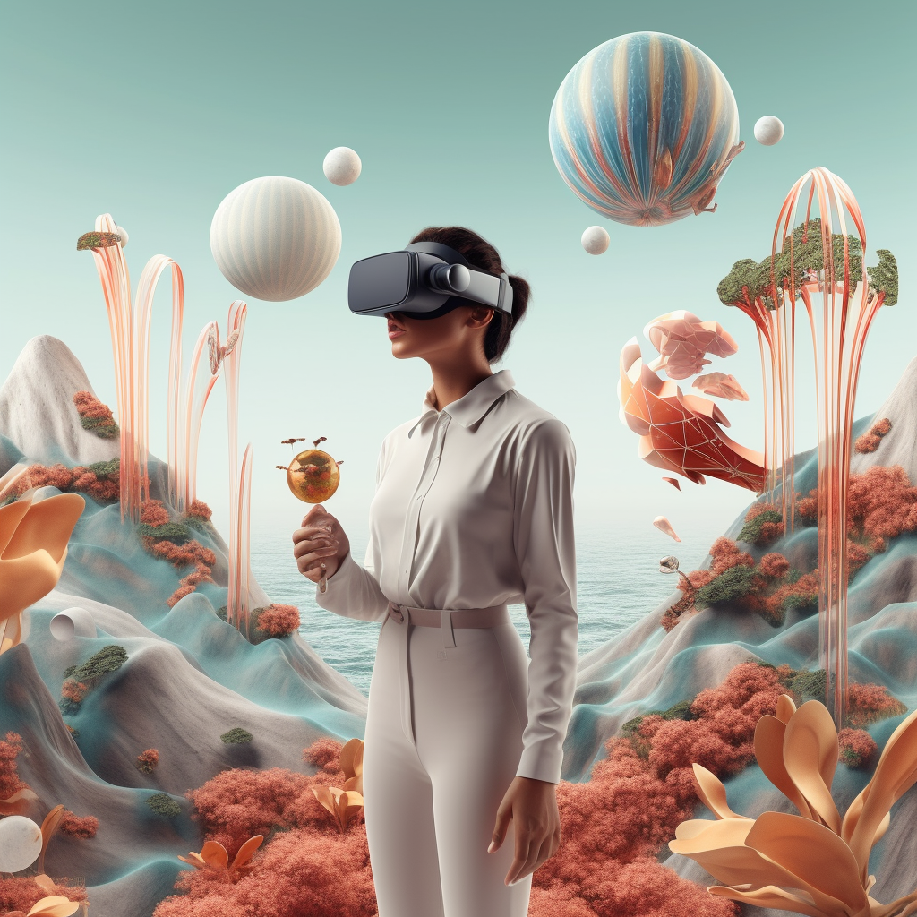
Virtual Reality Science: Exploring the Boundaries of Scientific Discovery
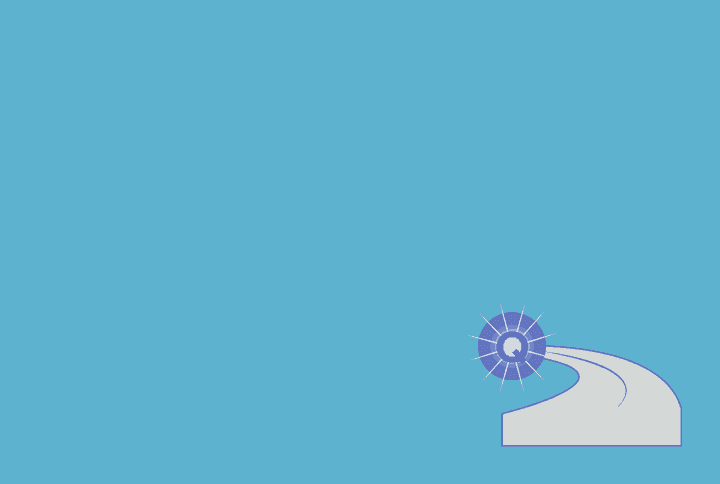
FHIR Claim Response
Corsi block test.
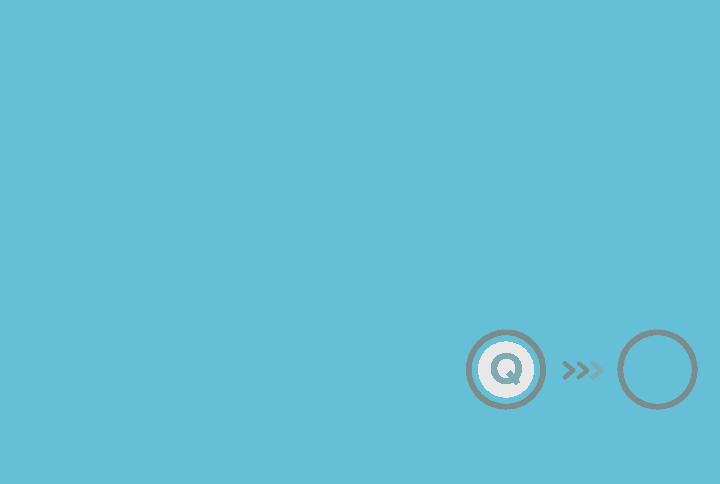
What Adoption of Remote Patient Monitoring will mean for Healthcare Delivery

Digital Health Research: A Guide
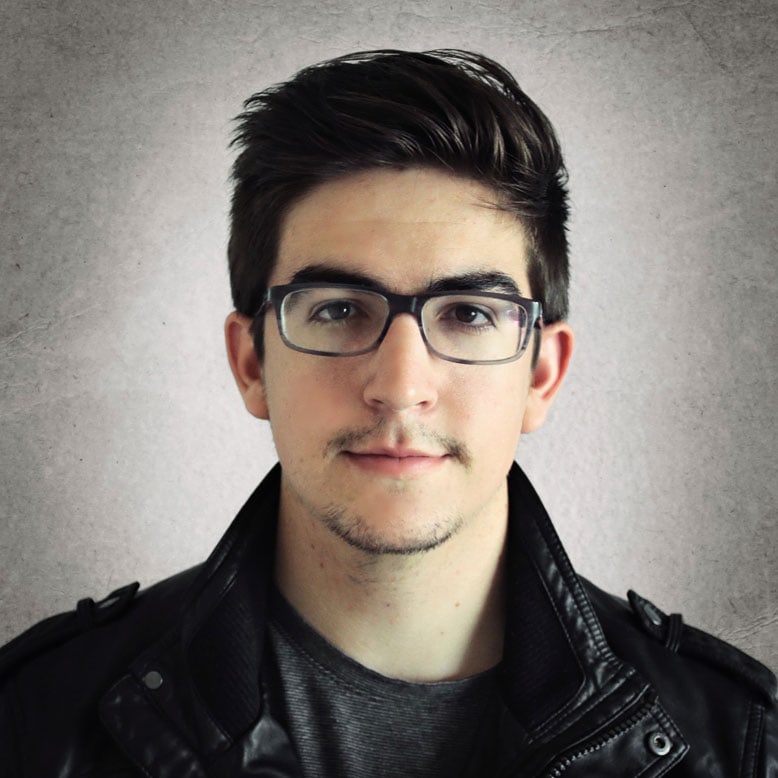
Alex Wormuth: Data Saves Lives
Never miss our posts.
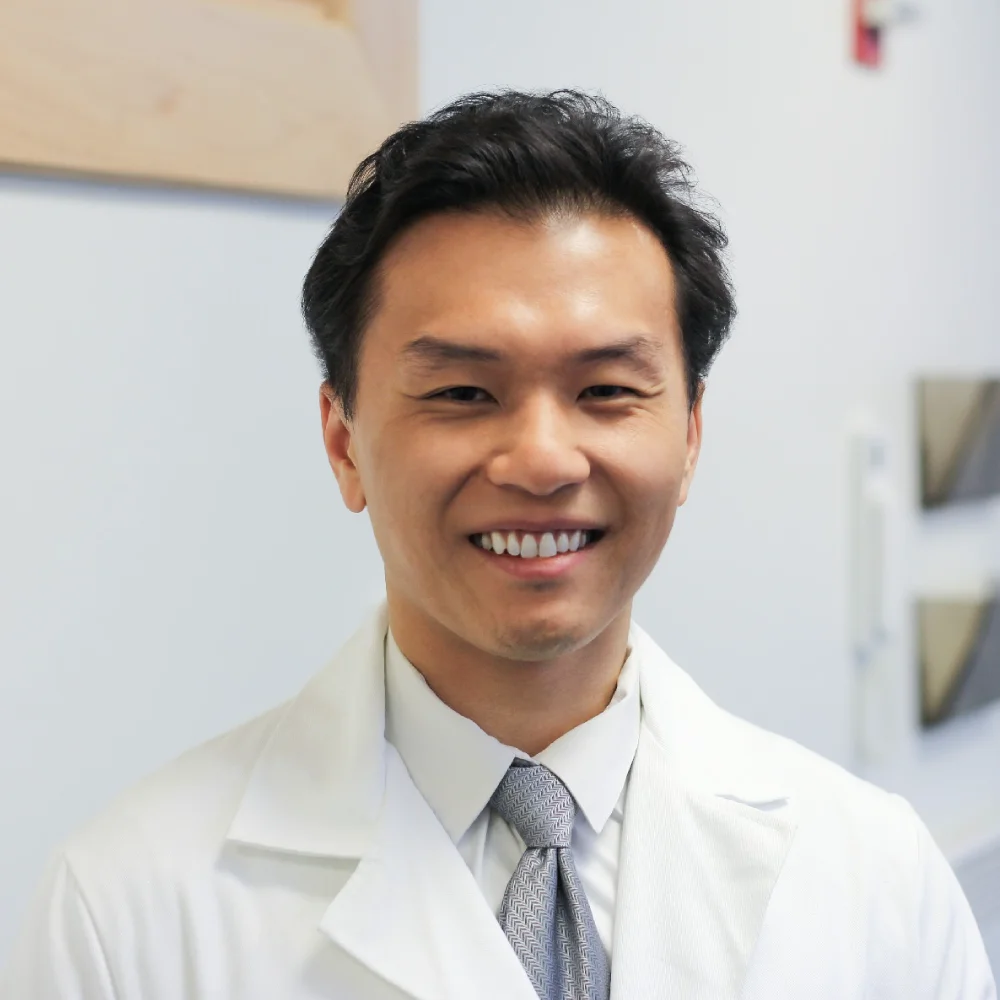
Shuhan He. MD
Shuhan He, MD is a dual-board certified physician with expertise in Emergency Medicine and Clinical Informatics. Dr. He works at the Laboratory of Computer Science, clinically in the Department of Emergency Medicine and Instructor of Medicine at Harvard Medical School. He serves as the Program Director of Healthcare Data Analytics at MGHIHP. Dr. He has interests at the intersection of acute care and computer science, utilizing algorithmic approaches to systems with a focus on large actionable data and Bayesian interpretation. Committed to making a positive impact in the field of healthcare through the use of cutting-edge technology and data analytics.
We’ve collected the items for you to purchase for your convenience.
Get the entire package for up to 50% discount with our Replication program.
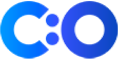
Our Location
- 5250 Old Orchard Rd Suite 300 Skokie, IL 60077
- +1 847 983 3672
Monday – Friday 9 AM – 5 PM EST
Conduct Science
- Become a Partner
- Social Media
- Career/Academia
- Privacy Policy
- Shipping & Returns
- Request a quote
Customer service
- Account Details
- Lost Password
DISCLAIMER: ConductScience and affiliate products are NOT designed for human consumption, testing, or clinical utilization. They are designed for pre-clinical utilization only. Customers purchasing apparatus for the purposes of scientific research or veterinary care affirm adherence to applicable regulatory bodies for the country in which their research or care is conducted.
Log in using your username and password
- Search More Search for this keyword Advanced search
- Latest content
- Current issue
- BMJ Journals
You are here
- Volume 17, Issue 1
- What does randomisation achieve?
- Article Text
- Article info
- Citation Tools
- Rapid Responses
- Article metrics

- Adam La Caze 1 ,
- Benjamin Djulbegovic 2 ,
- Stephen Senn 3
- 1 School of Pharmacy, The University of Queensland, St Lucia, Queensland, Australia
- 2 Center for Evidence-based Medicine & Health Outcome Research, University of South Florida & H Lee Moffitt Cancer Center & Research Institute, Tampa, Florida, USA
- 3 School of Mathematics and Statistics, University of Glasgow, Glasgow, UK
- Correspondence to Adam La Caze School of Pharmacy, The University of Queensland, St Lucia, QLD 4072, Australia; a.lacaze{at}uq.edu.au
https://doi.org/10.1136/ebm.2011.100061
Statistics from Altmetric.com
Request permissions.
If you wish to reuse any or all of this article please use the link below which will take you to the Copyright Clearance Center’s RightsLink service. You will be able to get a quick price and instant permission to reuse the content in many different ways.
What are the benefits of random allocation in clinical studies? John Worrall, a philosopher of science, recently questioned whether evidence-based medicine's advice to base therapeutic decisions on the results of randomised controlled trials (RCTs) could be justified. 1 2 Here we provide a response to Worrall and others who challenge the epistemological value of RCTs. Worrall's primary target is the view that RCTs are the only reliable source of evidence for medicine. But in arguing against this strong view, he posits a similarly strong counterposition. Worrall argues that randomisation offers no advantage over balanced systematic designs in which experimental and control groups are carefully matched according to known confounders. The best we can do (as ever) is test our theories against rivals that seem plausible in the light of background knowledge. Once we have eliminated other explanations that we know are possible (by suitable deliberate, or post hoc , control) we have done as much as we can epistemologically. 2
Focusing on this claim, we first discuss Worrall's arguments and then provide reasons to reject this view. There are good reasons to randomise studies of therapeutic interventions; principally, RCTs have the capacity to avoid a form of selection bias that cannot be avoided in observational studies.
Worrall draws the conclusion that randomised trials provide no epistemological benefit from two key arguments:
He criticises the notion promoted in the literature that random allocation controls for known and unknown confounders at baseline. Since the number of unknown (possible) causes are ‘innumerable’, “it would clearly be a miracle if all of those factors just happened to be balanced in the two groups. . . on a single random division.” 2 Indeed, the probability that any single confounder is unevenly distributed in a given RCT ranges from zero to one. 3 Hence, it is possible that some unknown confounder is distributed unevenly between treatment and control and correlated to the effect of intervention that was tested in the RCT.
Randomisation is not essential for controlling selection bias or concealing allocation. Worrall and others 4 maintain that these sources of bias can be equally well eliminated in studies that are not randomised.
Neither ‘1’ nor ‘2’, however, undermines the epistemological benefits of RCTs over observational studies in testing the efficacy of therapeutic interventions. Worrall's first argument is only relevant if the point he makes is not understood (however, ‘1’ is well understood by trial analysts) and ‘2’ suffers from serious problems.
Worrall's ‘1’, while not always explicitly discussed, is nonetheless well recognised in the statistical and epidemiological literature. The crucial point is that it is not necessary that randomisation control for all known and unknown confounders at baseline to make valid statistical inferences. It is not necessary for the purpose of issuing a probability statement to know the value of unmeasured covariates; it is sufficient to know their distribution in probability, which randomisation is designed to provide. 5 Similar knowledge regarding unmeasured covariates is not possible in observational designs. Analysis of observational studies rely on additional assumptions which cannot be easily verified. In short, Worrall's ‘1’ does not undermine standard frequentist statistical tests.
Another epistemological benefit of RCTs over observational studies is the capacity of RCTs to avoid confounding by indication (choice of treatment bias). Confounding by indication, in which treatment assignment is a function of the risk of future health outcomes (prognosis), is a particular problem for observational studies. Even if we match for a number of known factors, it is difficult in observational designs to rule out or account for all the factors which may influence the physicians' treatment assignment or the patients' reasons to accept or decline the intervention (see, for instance, Collins and MacMahon 6 ). RCTs are prone to other biases but not this one.
Eliminating confounding by indication is a strong argument for interventional studies over observational studies. Worrall's ‘2’ claims that it is possible to use alternatives to randomisation to ensure experimental groups are equally balanced for known confounders and conceal allocation. Worrall does not provide details on these alternatives. Presumably, he is focusing on interventional studies and expressing the idea that it is conceptually possible to devise a method (other than randomisation) that stratifies patients according to relevant prognostic information and allocates them to treatment or control that is independent of the investigator. A number of problems can be raised against this suggestion.
First, alternative methods of allocation are not possible for case-control or cohort studies – in these study designs, the patient decides to (or the patient's circumstances lead them to) take or not take the intervention under investigation. Worrall and Borgerson fail to give sufficient weight to the importance of confounding by indication as a justification for interventional studies (and RCTs in particular) over observational studies.
Second, where randomisation is possible and easily carried out, methods that claim to do better than randomisation should be critically examined. Randomising provides a distinct (and uncontroversial) experimental distribution on which to base statistical inferences; this is the case for frequentist 7 8 and Bayesian 9 10 approaches to statistical inference. Alternative methods will require a more complicated statistical model and additional assumptions of the data. This makes the appropriate choice of statistical model and analysis more cumbersome and typically more difficult to defend. These problems vitiate the proposed advantages of a non-random methods of allocation in interventional studies.
It is important to note that none of the previous discussion invalidates the importance of observational studies. RCTs are neither necessary nor sufficient to conclusively establish therapeutic claims: not necessary because alternative methods may be appropriate when the effect size is large relative to bias or random error that may possibly obscure it, 11 and not sufficient because there is more to assessing a therapeutic claim than success in a randomised trial (eg, assessing if the results from a RCT are generalisable). Furthermore, observational studies may be the best available method for particular questions (eg, assessing rare adverse effects of a medication). The question we are addressing here is: do RCTs offer unique epistemological value above and beyond observational studies?
It should be no surprise that a positive result from a well-conducted RCT falls short of providing conclusive proof of the efficacy of an intervention. A number of assumptions need to hold for a causal conclusion to be valid. Different accounts of causation and different approaches to statistical inference spell out the necessary assumptions in different ways. Nancy Cartwright 12 and Dan Steel 13 discuss the assumptions that need to hold on two probabilistic accounts of causation. Senn, 8 with a focus on clinical trials, discusses assumptions within the frequentist approach. The need for these assumptions does not undermine the importance of RCTs for assessing the efficacy of medical interventions.
RCTs offer unique epistemological advantages that cannot be realised via observational studies. Neither does this mean that the observational studies are unimportant nor does this mean that RCTs are the best method for all questions and in all circumstances (as is reflected in the Oxford Centre for Evidence-based Medicine's Levels of Evidence). 14 Rather, (therapeutic) clinical research should be understood as the means to respond to uncertainties about treatment effects of competing interventions, and the design of clinical study (observational vs RCTs) should be matched to the question/uncertainties at hand. 15
Acknowledgments
This article began as a discussion on the Evidence-Based Health email discussion list initiated by BD. The authors would like to acknowledge the members of the EBM discussion group, whose opinion and free sharing of ideas has informed this work.
- Borgerson K
- Collins R ,
- Glasziou P ,
- Chalmers I ,
- Rawlins M ,
- Cartwright N
- ↵ OCEBM Levels of Evidence Working Group. The Oxford 2011 Levels of Evidence. 2011 . Oxford Centre for Evidence-Based Medicine . http://www.cebm.net/index.aspx?o=5653 (accessed May 2011) .
- Djulbegovic B
Competing interests ALC and BD declare no competing interests. SS notes that he is a consultant to the pharmaceutical industry and an academic whose career is furthered by publishing.
Read the full text or download the PDF:
Scientific Research and Methodology
7.3 random allocation vs random sampling.
Random sampling and random allocation are two different concepts (Fig. 7.4 ), that serve two different purposes, but are often confused:
- Random sampling allows results to be generalised to a larger population, and impacts external validity. It concerns how the sample is found to study.
- Random allocation tries to eliminate confounding issues, by evening-out possible confounders across treatment groups. Random allocation of treatments helps establish cause-and-effect, and impacts internal validity. It concerns how the members of the chosen sample get the treatments .

FIGURE 7.4: Comparing random allocation and random sampling
- Open access
- Published: 09 November 2004
Random allocation software for parallel group randomized trials
- Mahmood Saghaei 1
BMC Medical Research Methodology volume 4 , Article number: 26 ( 2004 ) Cite this article
47k Accesses
487 Citations
Metrics details
Typically, randomization software should allow users to exert control over the different aspects of randomization including block design, provision of unique identifiers and control over the format and type of program output. While some of these characteristics have been addressed by available software, none of them have all of these capabilities integrated into one package. The main objective of the Random Allocation Software project was to enhance the user's control over different aspects of randomization in parallel group trials, including output type and format, structure and ordering of generated unique identifiers and enabling users to specify group names for more than two groups.
The program has different settings for: simple and blocked randomizations; length, format and ordering of generated unique identifiers; type and format of program output; and saving sessions for future use. A formatted random list generated by this program can be used directly (without further formatting) by the coordinator of the research team to prepare and encode different drugs or instruments necessary for the parallel group trial.
Conclusions
Random Allocation Software enables users to control different attributes of the random allocation sequence and produce qualified lists for parallel group trials.
Peer Review reports
An important aspect of any trial that should be clearly stated in the final report is the method used to assign treatments (or other interventions) to participants [ 1 ]. In the final report of the trial, authors should specify the method of sequence generation, i.e. whether they have used mechanical means, a computer generated random list or random number table. After preparing a random sequence, subjects will be allocated to the trial groups using an implementation method such as numbered containers, central telephone line, or allocation by a person who is not involved in the main research and patient care (the encoder). During the process of allocation each subject will be given a unique identification code (Unique Identifier, UI). This UI will be used as a label to uniquely identify the patient's group after completion of the study. During the study period this UI will be given to the main researchers together with the necessary treatment (e.g. drug or placebo). Usually these treatments are prepared by the encoder with the same physical characteristics (shape, color, size, etc), differing only by the UI labels to blind the main researchers about the actual patient group. Following enrollment of all subjects into the study, these UIs are decoded to determine the patient group. Depending on the preference of the researchers or facilities of the research environment, subjects are randomly allocated to intervention groups using either a random list prepared before the study (In Advance method) or a randomized allocation at the moment of intended intervention (Just In Time method; JIT). Both the JIT and In Advance methods produce acceptable allocations, and the actual choice depends on the availability of certain facilities for each method. Usually the randomization components of these two methods are produced by running a randomization software on either an Internet service provider or a local computer. The local encoder will obtain the next allocation (JIT method) or the entire random list (In Advance method) from the service provider or from the software on local computer and prepare the necessary blinded equipments.
Without the use of computer software or Internet services the maintenance of the whole process of randomization and allocation is difficult. In addition, in the case of any necessary restrictions on the process of randomization (i.e. block randomization [ 2 ]) the complexity of the process will be increased even further and be prone to errors. Randomization software may run on a local computer or may be hosted by an Internet server. A complete list of these software and services can be found on Martin Bland's web site [ 3 ]. Most of randomization software are hosted by websites for both JIT and In Advance methods, which require access to the Internet [ 4 – 8 ]. However, most of these Internet services have restricted capabilities with respect to the block design specification, control over the output format and flexibility of UIs. Among these available services, the tool in Randomization.com [ 4 ] seems to be more advanced than the others. It allows users to specify the number of subjects per block, the number of blocks and up to 20 treatment labels. Therefore, this service produces simple and block randomization using fixed and equal block sizes. Unfortunately, this service does not allow further restriction on block design (e.g., multiple block lengths or random variation in block number or size). The generated random list is in the form of UI and group name pairs, formatted in a single column, which in cases of large sample sizes may require further work to fit it in multiple columns for fine printing. Moreover, the block borders are not visible to allow for easy visual inspection of block sizes and equality of cases. Although a minor problem, Randomization.com only produces sequential numeric UIs with variable lengths (e.g. 1, 10, 100). The variability in lengths of UIs may disturb the visual impact of the generated list compared to the fixed length UIs (e.g. 001, 010, 100). Some researchers prefer to use random UIs in mixed alphanumeric format to decrease the likelihood of memorization and to improve the blindness of the study and concealment of allocations. Available randomization software have more restrictions in their capabilities than the Internet randomization services. They have limitations in their output format [ 9 ] and users can not specify the number and naming of the treatment groups [ 10 , 11 ]. In addition, these software are not designed with the capability to produce flexible UIs for participants. Therefore, the main objective of Random Allocation Software was to construct a randomization software for parallel group trials with the following characteristics:
1. Independent running on a local computer without any need to access the Internet
2. Different types of program output: to file (html or text files), window and system clipboard
3. Provisions for different block design
4. Capability to deal with a larger number of groups
5. Specifying a name for each group
6. Control over the format, length and ordering of the generated UIs
7. Control over the format of generated sequence
8. Saving or loading the randomization settings
9. Viewing previously generated randomized sequences
Implementation
Random Allocation Software is a program created in Microsoft Visual Basic 6, and it installs in the same way as ordinary Windows software (i.e. running setup.exe and following on screen instructions). Once installed and run, there are some controls in the main window for specifying the number of groups (2 to 16), sample size and the name of each group. It also contains menu items to determine the program output and randomization settings. The default program output is saved into either html or text files, and it may also have output to a window or to the system clipboard. A variety of randomization options can be set in the options window. The length of generated UIs (named as Code in the program) can be between 3 to 10 characters and there are options for different alphanumeric structures. In addition, these UIs can appear in sequential or random order in the generated random list. The program can generate simple or block randomization in different types, including equal size blocks, multiple block lengths with random variation among the specified block sizes and complete randomized blocks (random number and size of blocks). The generated sequence will appear in a multiple columns format and the number of columns (1 to 10) can be changed in the options window. Output to html file will be formatted in the form of one block per table. Borders of the tables may be shown or hidden. By clicking the 'Generate' button in the main window the random sequence will be generated and opened by the default viewer for the output file (e.g. Internet Explorer for html files). Previously created output files can also be viewed from inside the program. Additional options include saving the current randomization settings, loading a previous setting and enabling the program to save the last setting upon program exit.
During execution, the program produces a random sequence of allocation using the Rnd function that generates a floating point random number. The Rnd function uses the linear-congruent method for pseudo-random number generation as depicted by the following formula:
x1 = (x0 * a + c) MOD (2^24) [12]
x1 = new value
x0 = previous value (an initial value of 327680 is used by Visual Basic unless the Randomize X function is used to specify a different seed as X)
a = 1140671485
c = 12820163
The seed of the Rnd will be the Timer function, which will return the number of seconds elapsed since midnight. Although this version of the program does not produce repeatable lists, it is possible to revise the program in subsequent versions to save the value of the seed to reproduce the same random list. The output consisted of shuffled allocations each of which is a UI, group name pair. The program checks for the uniqueness of the UIs and generates an error message if the specified UI length is insufficient to hold the entire sample size.
Runs test was used to check randomness of the output list with sample sizes from 10 to 190 (10, 30, 50, ..., 190) and from 200 to 3000 (200, 600, 1000, ..., 3000). Each runs test was carried out for the group number of 2, 3, 5 and 6. SPSS 10 software was used to perform the runs test.
The program starts running with the default settings. Users may run the program with the default settings or set the number of groups, the name of each group and the sample size. Clicking the 'Generate' button (figure 1 ) produces the random sequence. Before generating the random sequence, the option window will be displayed and different randomization settings can be entered (figure 2 and 3 ). Consider, for example, that we want to produce a simple randomized list for a sample size of 30 subjects into three groups of Case, Control and Placebo with numeric sequential UIs. After setting different options and clicking the 'Generate' button, the generated list will appear in columns (Table 1 ). Each entry in the list consists of a UI, and a group name pair. Alternatively numeric UIs may appear in random order (Table 2 ). Table 3 shows the output of the program for a block randomization with blocks of equal sizes.
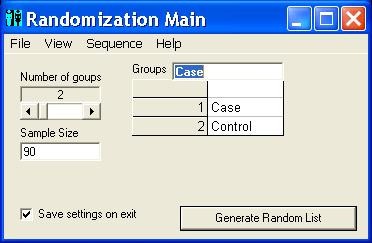
Main window. The main window showing different options for number of groups, sample size, and group names.
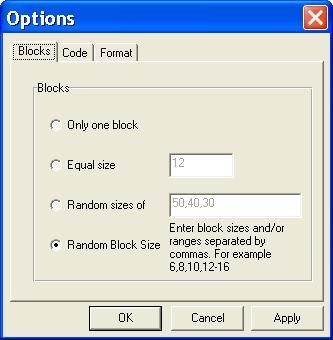
Options window: Blocks. Options window, settings for block design.
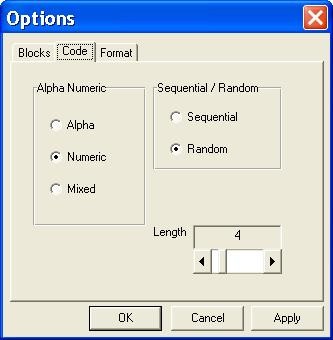
Options window: Code. Options window, setting the format of unique identifier (UI) specified in the program as Code.
Figure 4 is the printed output of the program for a block randomization with random block sizes.
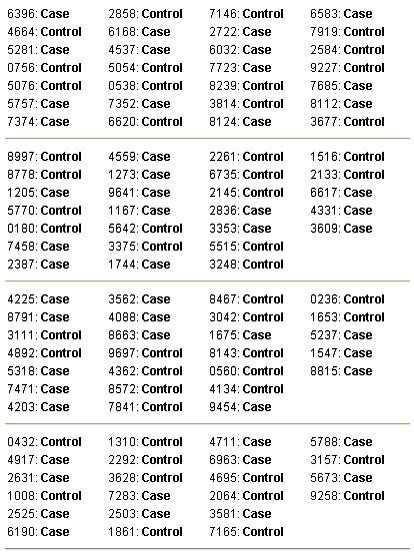
Sample output. Sample output for a block randomization with random block sizes.
In block randomization the final sample size is usually larger than the specified one.
A total of 18 runs test were performed to check the randomness of the program output, which resulted in P values of 0.22 to 0.81.
The main use of Random Allocation Software is to produce simple or block randomized sequences for parallel group trials. Its use is restricted to parallel group randomized trials. Compared with similar software, it enables the user to control the length, order and format of the UIs; and the type and format of the output. It allows specifying up to 16 groups for parallel trials.
Random Allocation Software has been designed to produce random sequences consisting of UI, group name pairs with additional control over the output format and type. Available randomization software generally has limitations in the number of groups, naming each group, generating UIs and control over the output. Many of these problems have been addressed in the present software. As has been stated in previous sections, the main use of this software is for randomization in parallel group trials. The software can be revised to support crossover and other types of randomized trials. The experienced user may test the randomness of the program output by selecting numeric labels for group names and then exporting the generated list into a statistical software such as SPSS to execute a runs test on the exported data.
Availability and requirements
Project name: Random Allocation Software
Public use access:
http://www.msaghaei.com/Softwares/dnld/RA.zip (Latest version)
http://ftp.mui.ac.ir/RA.zip
Operating systems: Windows 98, Me, 2000, XP. It should be noted that on some Windows operating systems (especially Windows 2000) during installation of the program an error message like "Setup Cannot Continue... System Files Are Out of Date" may be displayed. If this happens, click OK and restart the system. Then run the setup.exe again. This is due to a known bug in the installation programs of Microsoft Visual Basic [ 13 ]. This problem has been removed from the newer versions of the program. Users are recommended to download the latest version from the first address.
Programming Language: Visual Basic 6
Other requirements: Internet Browser (Internet Explorer 5 or higher is recommended)
License: Free for academic use.
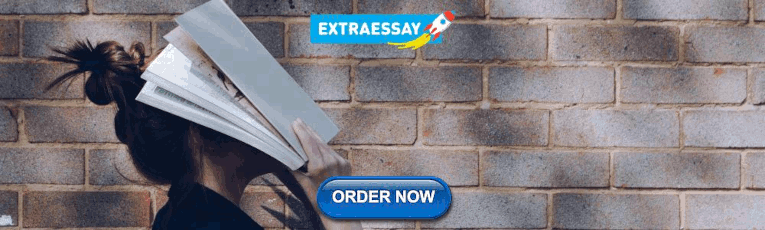
Abbreviations
Just in Time
- Unique Identifier
Schulz KF: Randomized controlled trials. Clin Obstet Gynecol. 1998, 41: 245-56. 10.1097/00003081-199806000-00005.
Article CAS PubMed Google Scholar
Altman DG, Schulz KF, Moher D, Egger M, Davidoff F, Elbourne D, Gøtzsche PC, Lang T, CONSORT GROUP (Consolidated Standards of Reporting Trials): The Revised CONSORT Statement for Reporting Randomized Trials: Explanation and Elaboration. Ann of Intern Med. 2001, 134: 663-694.
Article CAS Google Scholar
Directory of randomization software and services. [ http://www-users.york.ac.uk/~mb55/guide/randsery.htm ]
Randomization.com. [ http://www.randomization.com/ ]
GraphPad QuickCalcs. [ http://www.graphpad.com/quickcalcs/randomize1.cfm ]
EDGAR. [ http://www.jic.bbsrc.ac.uk/services/statistics/edgar.htm ]
PARADIGM Registration-Randomisation Software. [ http://telescan.nki.nl/paradigm.html ]
Randomizer. [ https://medinfo.uni-graz.at/randemo/web/about.php ]
Simple Statistical Software by Martin Bland. [ http://www-users.york.ac.uk/~mb55/soft/soft.htm ]
Randomization Generator download. [ http://www.som.soton.ac.uk/staff/jrg/files/Randomisation%20Generator%20download/ ]
KEYFINDER. [ http://lib.stat.cmu.edu/designs/ ]
Microsoft Knowledge Base Article – 231847. [ http://support.microsoft.com/default.aspx?scid=kb;en-us;231847 ]
Microsoft Knowledge Base Article – 174135. [ http://support.microsoft.com/default.aspx?scid=kb;en-us;174135#appliesto ]
Pre-publication history
The pre-publication history for this paper can be accessed here: http://www.biomedcentral.com/1471-2288/4/26/prepub
Download references
Acknowledgements
The author thanks Azra Kianinejad, Department of Human Development, Kobe University, Kobe, Japan for her kind review of the manuscript.
Author information
Authors and affiliations.
Department of Anaesthesia, Isfahan University of Medical Sciences, Isfahan, Iran
Mahmood Saghaei
You can also search for this author in PubMed Google Scholar
Corresponding author
Correspondence to Mahmood Saghaei .
Additional information
Competing interests.
The author declares that he has no competing interests.
Authors’ original submitted files for images
Below are the links to the authors’ original submitted files for images.
Authors’ original file for figure 1
Authors’ original file for figure 2, authors’ original file for figure 3, authors’ original file for figure 4, rights and permissions.
Reprints and permissions
About this article
Cite this article.
Saghaei, M. Random allocation software for parallel group randomized trials. BMC Med Res Methodol 4 , 26 (2004). https://doi.org/10.1186/1471-2288-4-26
Download citation
Received : 17 August 2004
Accepted : 09 November 2004
Published : 09 November 2004
DOI : https://doi.org/10.1186/1471-2288-4-26
Share this article
Anyone you share the following link with will be able to read this content:
Sorry, a shareable link is not currently available for this article.
Provided by the Springer Nature SharedIt content-sharing initiative
- Block Randomization
- Local Computer
- Option Window
- Randomization Software
BMC Medical Research Methodology
ISSN: 1471-2288
- General enquiries: [email protected]
Experimental Design: Types, Examples & Methods
Saul McLeod, PhD
Editor-in-Chief for Simply Psychology
BSc (Hons) Psychology, MRes, PhD, University of Manchester
Saul McLeod, PhD., is a qualified psychology teacher with over 18 years of experience in further and higher education. He has been published in peer-reviewed journals, including the Journal of Clinical Psychology.
Learn about our Editorial Process
Olivia Guy-Evans, MSc
Associate Editor for Simply Psychology
BSc (Hons) Psychology, MSc Psychology of Education
Olivia Guy-Evans is a writer and associate editor for Simply Psychology. She has previously worked in healthcare and educational sectors.
On This Page:
Experimental design refers to how participants are allocated to different groups in an experiment. Types of design include repeated measures, independent groups, and matched pairs designs.
Probably the most common way to design an experiment in psychology is to divide the participants into two groups, the experimental group and the control group, and then introduce a change to the experimental group, not the control group.
The researcher must decide how he/she will allocate their sample to the different experimental groups. For example, if there are 10 participants, will all 10 participants participate in both groups (e.g., repeated measures), or will the participants be split in half and take part in only one group each?
Three types of experimental designs are commonly used:
1. Independent Measures
Independent measures design, also known as between-groups , is an experimental design where different participants are used in each condition of the independent variable. This means that each condition of the experiment includes a different group of participants.
This should be done by random allocation, ensuring that each participant has an equal chance of being assigned to one group.
Independent measures involve using two separate groups of participants, one in each condition. For example:
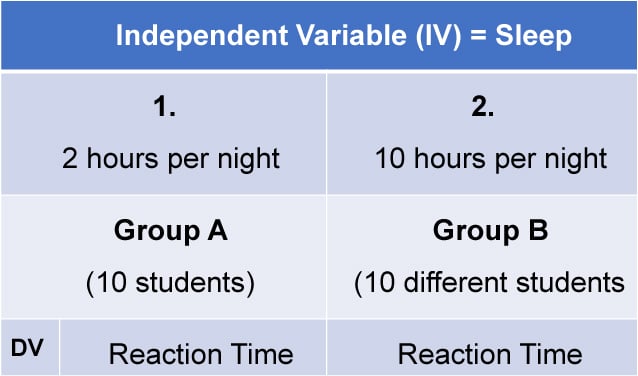
- Con : More people are needed than with the repeated measures design (i.e., more time-consuming).
- Pro : Avoids order effects (such as practice or fatigue) as people participate in one condition only. If a person is involved in several conditions, they may become bored, tired, and fed up by the time they come to the second condition or become wise to the requirements of the experiment!
- Con : Differences between participants in the groups may affect results, for example, variations in age, gender, or social background. These differences are known as participant variables (i.e., a type of extraneous variable ).
- Control : After the participants have been recruited, they should be randomly assigned to their groups. This should ensure the groups are similar, on average (reducing participant variables).
2. Repeated Measures Design
Repeated Measures design is an experimental design where the same participants participate in each independent variable condition. This means that each experiment condition includes the same group of participants.
Repeated Measures design is also known as within-groups or within-subjects design .
- Pro : As the same participants are used in each condition, participant variables (i.e., individual differences) are reduced.
- Con : There may be order effects. Order effects refer to the order of the conditions affecting the participants’ behavior. Performance in the second condition may be better because the participants know what to do (i.e., practice effect). Or their performance might be worse in the second condition because they are tired (i.e., fatigue effect). This limitation can be controlled using counterbalancing.
- Pro : Fewer people are needed as they participate in all conditions (i.e., saves time).
- Control : To combat order effects, the researcher counter-balances the order of the conditions for the participants. Alternating the order in which participants perform in different conditions of an experiment.
Counterbalancing
Suppose we used a repeated measures design in which all of the participants first learned words in “loud noise” and then learned them in “no noise.”
We expect the participants to learn better in “no noise” because of order effects, such as practice. However, a researcher can control for order effects using counterbalancing.
The sample would be split into two groups: experimental (A) and control (B). For example, group 1 does ‘A’ then ‘B,’ and group 2 does ‘B’ then ‘A.’ This is to eliminate order effects.
Although order effects occur for each participant, they balance each other out in the results because they occur equally in both groups.
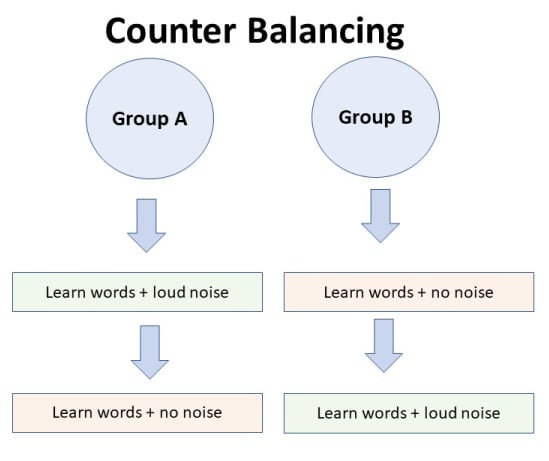
3. Matched Pairs Design
A matched pairs design is an experimental design where pairs of participants are matched in terms of key variables, such as age or socioeconomic status. One member of each pair is then placed into the experimental group and the other member into the control group .
One member of each matched pair must be randomly assigned to the experimental group and the other to the control group.
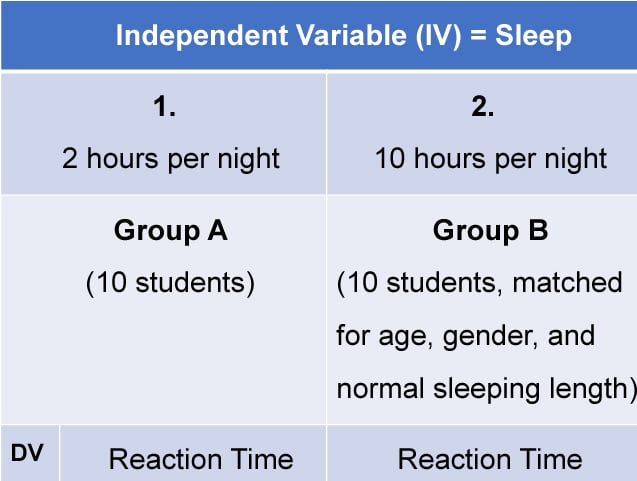
- Con : If one participant drops out, you lose 2 PPs’ data.
- Pro : Reduces participant variables because the researcher has tried to pair up the participants so that each condition has people with similar abilities and characteristics.
- Con : Very time-consuming trying to find closely matched pairs.
- Pro : It avoids order effects, so counterbalancing is not necessary.
- Con : Impossible to match people exactly unless they are identical twins!
- Control : Members of each pair should be randomly assigned to conditions. However, this does not solve all these problems.
Experimental design refers to how participants are allocated to an experiment’s different conditions (or IV levels). There are three types:
1. Independent measures / between-groups : Different participants are used in each condition of the independent variable.
2. Repeated measures /within groups : The same participants take part in each condition of the independent variable.
3. Matched pairs : Each condition uses different participants, but they are matched in terms of important characteristics, e.g., gender, age, intelligence, etc.
Learning Check
Read about each of the experiments below. For each experiment, identify (1) which experimental design was used; and (2) why the researcher might have used that design.
1 . To compare the effectiveness of two different types of therapy for depression, depressed patients were assigned to receive either cognitive therapy or behavior therapy for a 12-week period.
The researchers attempted to ensure that the patients in the two groups had similar severity of depressed symptoms by administering a standardized test of depression to each participant, then pairing them according to the severity of their symptoms.
2 . To assess the difference in reading comprehension between 7 and 9-year-olds, a researcher recruited each group from a local primary school. They were given the same passage of text to read and then asked a series of questions to assess their understanding.
3 . To assess the effectiveness of two different ways of teaching reading, a group of 5-year-olds was recruited from a primary school. Their level of reading ability was assessed, and then they were taught using scheme one for 20 weeks.
At the end of this period, their reading was reassessed, and a reading improvement score was calculated. They were then taught using scheme two for a further 20 weeks, and another reading improvement score for this period was calculated. The reading improvement scores for each child were then compared.
4 . To assess the effect of the organization on recall, a researcher randomly assigned student volunteers to two conditions.
Condition one attempted to recall a list of words that were organized into meaningful categories; condition two attempted to recall the same words, randomly grouped on the page.
Experiment Terminology
Ecological validity.
The degree to which an investigation represents real-life experiences.
Experimenter effects
These are the ways that the experimenter can accidentally influence the participant through their appearance or behavior.
Demand characteristics
The clues in an experiment lead the participants to think they know what the researcher is looking for (e.g., the experimenter’s body language).
Independent variable (IV)
The variable the experimenter manipulates (i.e., changes) is assumed to have a direct effect on the dependent variable.
Dependent variable (DV)
Variable the experimenter measures. This is the outcome (i.e., the result) of a study.
Extraneous variables (EV)
All variables which are not independent variables but could affect the results (DV) of the experiment. Extraneous variables should be controlled where possible.
Confounding variables
Variable(s) that have affected the results (DV), apart from the IV. A confounding variable could be an extraneous variable that has not been controlled.
Random Allocation
Randomly allocating participants to independent variable conditions means that all participants should have an equal chance of taking part in each condition.
The principle of random allocation is to avoid bias in how the experiment is carried out and limit the effects of participant variables.
Order effects
Changes in participants’ performance due to their repeating the same or similar test more than once. Examples of order effects include:
(i) practice effect: an improvement in performance on a task due to repetition, for example, because of familiarity with the task;
(ii) fatigue effect: a decrease in performance of a task due to repetition, for example, because of boredom or tiredness.

We're sorry, but some features of Research Randomizer require JavaScript. If you cannot enable JavaScript, we suggest you use an alternative random number generator such as the one available at Random.org .
RESEARCH RANDOMIZER
Random sampling and random assignment made easy.
Research Randomizer is a free resource for researchers and students in need of a quick way to generate random numbers or assign participants to experimental conditions. This site can be used for a variety of purposes, including psychology experiments, medical trials, and survey research.
GENERATE NUMBERS
In some cases, you may wish to generate more than one set of numbers at a time (e.g., when randomly assigning people to experimental conditions in a "blocked" research design). If you wish to generate multiple sets of random numbers, simply enter the number of sets you want, and Research Randomizer will display all sets in the results.
Specify how many numbers you want Research Randomizer to generate in each set. For example, a request for 5 numbers might yield the following set of random numbers: 2, 17, 23, 42, 50.
Specify the lowest and highest value of the numbers you want to generate. For example, a range of 1 up to 50 would only generate random numbers between 1 and 50 (e.g., 2, 17, 23, 42, 50). Enter the lowest number you want in the "From" field and the highest number you want in the "To" field.
Selecting "Yes" means that any particular number will appear only once in a given set (e.g., 2, 17, 23, 42, 50). Selecting "No" means that numbers may repeat within a given set (e.g., 2, 17, 17, 42, 50). Please note: Numbers will remain unique only within a single set, not across multiple sets. If you request multiple sets, any particular number in Set 1 may still show up again in Set 2.
Sorting your numbers can be helpful if you are performing random sampling, but it is not desirable if you are performing random assignment. To learn more about the difference between random sampling and random assignment, please see the Research Randomizer Quick Tutorial.
Place Markers let you know where in the sequence a particular random number falls (by marking it with a small number immediately to the left). Examples: With Place Markers Off, your results will look something like this: Set #1: 2, 17, 23, 42, 50 Set #2: 5, 3, 42, 18, 20 This is the default layout Research Randomizer uses. With Place Markers Within, your results will look something like this: Set #1: p1=2, p2=17, p3=23, p4=42, p5=50 Set #2: p1=5, p2=3, p3=42, p4=18, p5=20 This layout allows you to know instantly that the number 23 is the third number in Set #1, whereas the number 18 is the fourth number in Set #2. Notice that with this option, the Place Markers begin again at p1 in each set. With Place Markers Across, your results will look something like this: Set #1: p1=2, p2=17, p3=23, p4=42, p5=50 Set #2: p6=5, p7=3, p8=42, p9=18, p10=20 This layout allows you to know that 23 is the third number in the sequence, and 18 is the ninth number over both sets. As discussed in the Quick Tutorial, this option is especially helpful for doing random assignment by blocks.
Please note: By using this service, you agree to abide by the SPN User Policy and to hold Research Randomizer and its staff harmless in the event that you experience a problem with the program or its results. Although every effort has been made to develop a useful means of generating random numbers, Research Randomizer and its staff do not guarantee the quality or randomness of numbers generated. Any use to which these numbers are put remains the sole responsibility of the user who generated them.
Note: By using Research Randomizer, you agree to its Terms of Service .
Information
- Author Services
Initiatives
You are accessing a machine-readable page. In order to be human-readable, please install an RSS reader.
All articles published by MDPI are made immediately available worldwide under an open access license. No special permission is required to reuse all or part of the article published by MDPI, including figures and tables. For articles published under an open access Creative Common CC BY license, any part of the article may be reused without permission provided that the original article is clearly cited. For more information, please refer to https://www.mdpi.com/openaccess .
Feature papers represent the most advanced research with significant potential for high impact in the field. A Feature Paper should be a substantial original Article that involves several techniques or approaches, provides an outlook for future research directions and describes possible research applications.
Feature papers are submitted upon individual invitation or recommendation by the scientific editors and must receive positive feedback from the reviewers.
Editor’s Choice articles are based on recommendations by the scientific editors of MDPI journals from around the world. Editors select a small number of articles recently published in the journal that they believe will be particularly interesting to readers, or important in the respective research area. The aim is to provide a snapshot of some of the most exciting work published in the various research areas of the journal.
Original Submission Date Received: .
- Active Journals
- Find a Journal
- Proceedings Series
- For Authors
- For Reviewers
- For Editors
- For Librarians
- For Publishers
- For Societies
- For Conference Organizers
- Open Access Policy
- Institutional Open Access Program
- Special Issues Guidelines
- Editorial Process
- Research and Publication Ethics
- Article Processing Charges
- Testimonials
- Preprints.org
- SciProfiles
- Encyclopedia
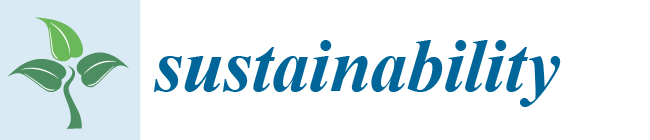
Article Menu
- Subscribe SciFeed
- Recommended Articles
- Google Scholar
- on Google Scholar
- Table of Contents
Find support for a specific problem in the support section of our website.
Please let us know what you think of our products and services.
Visit our dedicated information section to learn more about MDPI.
JSmol Viewer
Advanced predictive modeling for dam occupancy using historical and meteorological data.
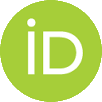
1. Introduction
2. literature review, 3. dams of istanbul, 4. design of the dataset, 5. prediction models, 5.1. long short-term memory, 5.2. orthogonal matching pursuit cv, 5.3. lasso lars cv, 5.4. random forest, 5.5. extra trees, 6. results and discussion, 7. conclusions.
- Ensures water is allocated optimally among various users (agriculture, industry, domestic) based on current water availability.
- Enables proactive management of water resources during periods of drought by accurately predicting available water supplies.
- Helps in managing dam releases to mitigate downstream flooding risks by maintaining appropriate water levels.
- Maintains minimum ecological flows downstream to support aquatic habitats and biodiversity.
- Increases the sustainability of businesses with less hardware requirement and less energy need.
Author Contributions
Data availability statement, conflicts of interest.
- Allen, R.G.; Pereira, L.S.; Raes, D.; Smith, M. Crop Evapotranspiration—Guidelines for Computing Crop Water Requirements—FAO Irrigation and Drainage Paper 56 ; FAO: Rome, Italy, 1998. [ Google Scholar ]
- Foudi, S.; McCartney, M.; Markandya, A.; Pascual, U. The impact of multipurpose dams on the values of nature’s contributions to people under a water-energy-food nexus framing. Ecol. Econ. 2023 , 206 , 107758. [ Google Scholar ] [ CrossRef ]
- Bieber, N.; Ker, J.H.; Wang, X.; Triantafyllidis, C.; van Dam, K.H.; Koppelaar, R.H.; Shah, N. Sustainable planning of the energy-water-food nexus using decision making tools. Energy Policy 2018 , 113 , 584–607. [ Google Scholar ] [ CrossRef ]
- Jalilov, S.M.; Keskinen, M.; Varis, O.; Amer, S.; Ward, F.A. Managing the water-energy-food nexus: Gains and losses from new water development in Amu Darya River Basin. J. Hydrol. 2016 , 539 , 648–661. [ Google Scholar ] [ CrossRef ]
- Lee, E.H. Proactive dam operation based on inflow prediction by modified long short-term memory for improving resilience. Eng. Appl. Artif. Intell. 2024 , 133 , 108525. [ Google Scholar ] [ CrossRef ]
- Li, M.; Ren, Q.; Li, M.; Fang, X.; Xiao, L.; Li, H. A separate modeling approach to noisy displacement prediction of concrete dams via improved deep learning with frequency division. Adv. Eng. Inform. 2024 , 60 , 102367. [ Google Scholar ] [ CrossRef ]
- Zin, M.F.M.; Kamal, F.Z.; Ismail, S.I.; Noh, K.S.S.K.M.; Kassim, A.H. Development of dam controller technology water level and alert system using Arduino UNO. Indones. J. Electr. Eng. Comput. Sci. 2023 , 31 , 1342–1349. [ Google Scholar ] [ CrossRef ]
- Ziggah, Y.Y.; Issaka, Y.; Laari, P.B. Evaluation of different artificial intelligent methods for predicting dam piezometric water level. Model. Earth Syst. Environ. 2022 , 8 , 2715–2731. [ Google Scholar ] [ CrossRef ]
- Tshireletso, T.; Moyo, P.; Kabani, M. Predicting the effects of climate change on water temperatures of roode elsberg dam using nonparametric machine learning models. Infrastructures 2021 , 6 , 14. [ Google Scholar ] [ CrossRef ]
- Vishwakarma, D.K.; Ali, R.; Bhat, S.A.; Elbeltagi, A.; Kushwaha, N.L.; Kumar, R.; Rajput, J.; Heddam, S.; Kuriqi, A. Pre- and post-dam river water temperature alteration prediction using advanced machine learning models. Environ. Sci. Pollut. Res. 2022 , 29 , 83321–83346. [ Google Scholar ] [ CrossRef ] [ PubMed ]
- Ouma, Y.O.; Moalafhi, D.B.; Anderson, G.; Nkwae, B.; Odirile, P.; Parida, B.P.; Qi, J. Dam Water Level Prediction Using Vector AutoRegression, Random Forest Regression and MLP-ANN Models Based on Land-Use and Climate Factors. Sustainability 2022 , 14 , 14934. [ Google Scholar ] [ CrossRef ]
- Ganesh, R.S.; Sasipriya, S.; Gowtham Balaji, M.; Ashok Karthi, G.; Gokul Dharan, S. An IoT-based Dam Water Level Monitoring and Alerting System. In Proceedings of the International Conference on Applied Artificial Intelligence and Computing, ICAAIC 2022, Salem, India, 9–11 May 2022. [ Google Scholar ]
- Kavitha, R.; Jayalakshmi, C.; Senthil Kumar, K. Dam Water Level Monitoring and Alerting System using IOT. Int. J. Electron. Commun. Eng. 2018 , 5 , 19–22. [ Google Scholar ]
- Ngebe, S.; Malunda, K.B.; du Plessis, A. Utility of geospatial techniques in estimating dam water levels: Insights from the Katrivier Dam. Water SA 2022 , 48 , 151–160. [ Google Scholar ]
- Li, W.; Qin, Y.; Sun, Y.; Huang, H.; Ling, F.; Tian, L.; Ding, Y. Estimating the relationship between dam water level and surface water area for the Danjiangkou Reservoir using Landsat remote sensing images. Remote Sens. Lett. 2016 , 7 , 121–130. [ Google Scholar ] [ CrossRef ]
- Ibañez, S.C.; Dajac, C.V.G.; Liponhay, M.P.; Legara, E.F.T.; Esteban, J.M.H.; Monterola, C.P. Forecasting reservoir water levels using deep neural networks: A case study of angat dam in the philippines. Water 2022 , 14 , 34. [ Google Scholar ] [ CrossRef ]
- Ahmed, E.-S.N.; Amr, E.-S. Daily forecasting of dam water levels using machine learning. Int. J. Civ. Eng. Technol. 2019 , 10 , 314–323. [ Google Scholar ]
- Yu, W.; Nakakita, E.; Kim, S.; Yamaguchi, K. Improving the accuracy of flood forecasting with transpositions of ensemble NWP rainfall fields considering orographic effects. J. Hydrol. 2016 , 539 , 345–357. [ Google Scholar ] [ CrossRef ]
- Zhang, R.; Chen, Z.Y.; Xu, L.J.; Ou, C.Q. Meteorological drought forecasting based on a statistical model with machine learning techniques in Shaanxi province, China. Sci. Total Environ. 2019 , 665 , 338–346. [ Google Scholar ] [ CrossRef ]
- Ryu, Y.M.; Lee, E.H. Application of Neural Networks to Predict Daecheong Dam Water Levels. J. Korean Soc. Hazard Mitig. 2022 , 22 , 67–78. [ Google Scholar ] [ CrossRef ]
- Dayal, A.; Bonthu, S.; T, V.N.; Saripalle, P.; Mohan, R. Deep learning for Multi-horizon Water level Forecasting in KRS reservoir, India. Results Eng. 2024 , 21 , 101828. [ Google Scholar ] [ CrossRef ]
- Üneş, F.; Demirci, M.; Kişi, Ö. Prediction of Millers Ferry Dam Reservoir Level in USA Using Artificial Neural Network. Period. Polytech. Civ. Eng. 2015 , 59 , 309–318. [ Google Scholar ] [ CrossRef ]
- Hipni, A.; El-shafie, A.; Najah, A.; Karim, O.A.; Hussain, A.; Mukhlisin, M. Daily Forecasting of Dam Water Levels: Comparing a Support Vector Machine (SVM) Model With Adaptive Neuro Fuzzy Inference System (ANFIS). Water Resour. Manag. 2013 , 27 , 3803–3823. [ Google Scholar ] [ CrossRef ]
- Huang, S.; Xia, J.; Zeng, S.; Wang, Y.; She, D. Effect of Three Gorges Dam on Poyang Lake water level at daily scale based on machine learning. J. Geogr. Sci. 2021 , 31 , 1598–1614. [ Google Scholar ] [ CrossRef ]
- Larrea, P.P.; Ríos, X.Z.; Parra, L.C. Application of neural network models and anfis for water level forecasting of the salve faccha dam in the andean zone in Northern Ecuador. Water 2021 , 13 , 2011. [ Google Scholar ] [ CrossRef ]
- Ayanlade, A.; Radeny, M.; Morton, J.F.; Muchaba, T. Rainfall variability and drought characteristics in two agro-climatic zones: An assessment of climate change challenges in Africa. Sci. Total Environ. 2018 , 630 , 728–737. [ Google Scholar ] [ CrossRef ]
- Fowler, H.J.; Kilsby, C.G. A weather-type approach to analysing water resource drought in the Yorkshire region from 1881 to 1998. J. Hydrol. 2002 , 262 , 177–192. [ Google Scholar ] [ CrossRef ]
Click here to enlarge figure
Method | Horizon | MAPE | Reference |
---|---|---|---|
Random Forest | Daily | 0.121 | [ ] |
Vector autoregressive models | Daily | 0.015 | [ ] |
Linear regression | Daily | 0.059477 | [ ] |
Long Short-Term Memory | Daily | 0.01 | [ ] |
Long Short-Term Memory | Monthly | 0.08 | [ ] |
Support vector machines | Daily | 0.0164 | [ ] |
Weather Data | CD | Dam Occupancy Levels | |||||||||||||||||||
---|---|---|---|---|---|---|---|---|---|---|---|---|---|---|---|---|---|---|---|---|---|
1. Evapotranspiration | 2. Solar Radiation | 3. Sea-Level Pressure | 4. Wind Speed | 5. Temperature | 6. Minimum Felt Temperature | 7. Humidity | 8. Dew Point | 9. Cloud Cover | 10. Rainfall | 11. Snow Depth | 12. Daylight Duration (Hours) | 13. Water Consumption | 14. Ömerli Occupancy | 15. Darlık Occupancy | 16. Elmalı Occupancy | 17. Terkos Occupancy | 18. Alibey Occupancy | 19. B.Çekmece Occupancy | 20. Sazlıdere Occupancy | ||
1 | - | 0.86 | −0.42 | −0.12 | 0.81 | 0.74 | −0.48 | 0.71 | 0.86 | −0.24 | −0.13 | 0.79 | 0.69 | 0.44 | 0.37 | 0.19 | 0.37 | 0.13 | 0.25 | 0.24 | |
Weather Data | 2 | 0.86 | - | −0.21 | −0.11 | 0.6 | 0.52 | −0.52 | 0.47 | 0.99 | −0.34 | −0.11 | 0.7 | 0.54 | 0.48 | 0.43 | 0.27 | 0.38 | 0.2 | 0.31 | 0.27 |
3 | −0.42 | −0.21 | - | 0 | −0.51 | −0.49 | 0.11 | −0.5 | −0.21 | −0.15 | 0.13 | −0.4 | −0.29 | −0.19 | −0.16 | −0.04 | −0.14 | −0.01 | −0.08 | −0.08 | |
4 | −0.12 | −0.11 | 0 | - | 0 | 0.01 | 0.06 | 0.02 | −0.11 | 0.22 | 0.07 | 0.03 | 0.04 | 0.05 | 0.01 | −0.04 | −0.07 | −0.1 | −0.06 | −0.08 | |
5 | 0.81 | 0.6 | −0.51 | 0 | - | 0.98 | −0.37 | 0.95 | 0.6 | −0.17 | −0.25 | 0.65 | 0.79 | 0.19 | 0.11 | −0.06 | 0.17 | −0.1 | 0.04 | 0.07 | |
6 | 0.74 | 0.52 | −0.49 | 0.01 | 0.98 | - | −0.29 | 0.96 | 0.52 | −0.16 | −0.27 | 0.61 | 0.75 | 0.14 | 0.07 | −0.09 | 0.14 | −0.13 | 0.02 | 0.05 | |
7 | −0.48 | −0.52 | 0.11 | 0.06 | −0.37 | −0.29 | - | −0.08 | −0.52 | 0.27 | 0.12 | −0.29 | −0.33 | −0.19 | −0.21 | −0.12 | −0.18 | −0.12 | −0.12 | −0.12 | |
8 | 0.71 | 0.47 | −0.5 | 0.02 | 0.95 | 0.96 | −0.08 | - | 0.47 | −0.11 | −0.24 | 0.6 | 0.74 | 0.13 | 0.05 | −0.1 | 0.12 | −0.15 | 0.01 | 0.04 | |
9 | 0.86 | 0.99 | −0.21 | −0.11 | 0.6 | 0.52 | −0.52 | 0.47 | - | −0.34 | −0.11 | 0.7 | 0.54 | 0.48 | 0.43 | 0.27 | 0.38 | 0.2 | 0.31 | 0.27 | |
10 | −0.24 | −0.34 | −0.15 | 0.22 | −0.17 | −0.16 | 0.27 | −0.11 | −0.34 | - | 0.08 | −0.17 | −0.24 | −0.11 | −0.09 | −0.05 | −0.12 | −0.03 | −0.08 | −0.11 | |
11 | −0.13 | −0.11 | 0.13 | 0.07 | −0.25 | −0.27 | 0.12 | −0.24 | −0.11 | 0.08 | - | −0.11 | −0.13 | −0.04 | −0.04 | 0 | −0.03 | 0.04 | 0 | −0.06 | |
12 | 0.79 | 0.7 | −0.4 | 0.03 | 0.65 | 0.61 | −0.29 | 0.6 | 0.7 | −0.17 | −0.11 | - | 0.49 | 0.67 | 0.63 | 0.38 | 0.56 | 0.31 | 0.45 | 0.45 | |
CD | 13 | 0.69 | 0.54 | −0.29 | 0.04 | 0.79 | 0.75 | −0.33 | 0.74 | 0.54 | −0.24 | −0.13 | 0.49 | - | 0.12 | −0.05 | −0.17 | −0.01 | −0.21 | −0.03 | 0.02 |
Occupancy | 14 | 0.44 | 0.48 | −0.19 | 0.05 | 0.19 | 0.14 | −0.19 | 0.13 | 0.48 | −0.11 | −0.04 | 0.67 | 0.12 | - | 0.79 | 0.71 | 0.67 | 0.44 | 0.51 | 0.41 |
15 | 0.37 | 0.43 | −0.16 | 0.01 | 0.11 | 0.07 | −0.21 | 0.05 | 0.43 | −0.09 | −0.04 | 0.63 | −0.05 | 0.79 | - | 0.74 | 0.75 | 0.64 | 0.58 | 0.51 | |
16 | 0.19 | 0.27 | −0.04 | −0.04 | −0.06 | −0.09 | −0.12 | −0.1 | 0.27 | −0.05 | 0 | 0.38 | −0.17 | 0.71 | 0.74 | - | 0.91 | 0.8 | 0.83 | 0.75 | |
17 | 0.37 | 0.38 | −0.14 | −0.07 | 0.17 | 0.14 | −0.18 | 0.12 | 0.38 | −0.12 | −0.03 | 0.56 | −0.01 | 0.67 | 0.75 | 0.91 | - | 0.76 | 0.88 | 0.8 | |
18 | 0.13 | 0.2 | −0.01 | −0.1 | −0.1 | −0.13 | −0.12 | −0.15 | 0.2 | −0.03 | 0.04 | 0.31 | −0.21 | 0.44 | 0.64 | 0.8 | 0.76 | - | 0.84 | 0.76 | |
19 | 0.25 | 0.31 | −0.08 | −0.06 | 0.04 | 0.02 | −0.12 | 0.01 | 0.31 | −0.08 | 0 | 0.45 | −0.03 | 0.51 | 0.58 | 0.83 | 0.88 | 0.84 | - | 0.89 | |
20 | 0.24 | 0.27 | −0.08 | −0.08 | 0.07 | 0.05 | −0.12 | 0.04 | 0.27 | −0.11 | −0.06 | 0.45 | 0.02 | 0.41 | 0.51 | 0.75 | 0.8 | 0.76 | 0.89 | - |
Historical Data (A Week Before) | Historical Data (One Day Before) | Current Day | ||||||||||||||||||||
---|---|---|---|---|---|---|---|---|---|---|---|---|---|---|---|---|---|---|---|---|---|---|
1. Ömerli Previous Week | 2. Darlık Previous Week | 3. Elmalı Previous Week | 4. Terkos Previous Week | 5. Alibey Previous Week | 6. B.Çekmece Previous Week | 7. Sazlıdere Previous Week | 8. Ömerli Previous Day | 9. Darlık Previous Day | 10. Elmalı Previous Day | 11. Terkos Previous Day | 12. Alibey Previous Day | 13. B.Çekmece Previous Day | 14. Sazlıdere Previous Day | 15. Ömerli Occupancy | 16. Darlık Occupancy | 17. Elmalı Occupancy | 18. Terkos Occupancy | 19. Alibey Occupancy | 20. B.Çekmece Occupancy | 21. Sazlıdere Occupancy | ||
A Week Before | 1 | - | 0.796 | 0.702 | 0.663 | 0.449 | 0.517 | 0.419 | 0.990 | 0.792 | 0.673 | 0.674 | 0.420 | 0.516 | 0.418 | 0.987 | 0.791 | 0.668 | 0.676 | 0.415 | 0.515 | 0.417 |
2 | 0.796 | - | 0.728 | 0.748 | 0.635 | 0.590 | 0.520 | 0.783 | 0.991 | 0.695 | 0.759 | 0.605 | 0.591 | 0.520 | 0.780 | 0.988 | 0.690 | 0.760 | 0.599 | 0.590 | 0.519 | |
3 | 0.702 | 0.728 | - | 0.854 | 0.811 | 0.802 | 0.731 | 0.713 | 0.744 | 0.986 | 0.881 | 0.796 | 0.815 | 0.751 | 0.714 | 0.746 | 0.983 | 0.885 | 0.793 | 0.816 | 0.754 | |
4 | 0.663 | 0.748 | 0.854 | - | 0.711 | 0.878 | 0.803 | 0.642 | 0.727 | 0.817 | 0.994 | 0.670 | 0.862 | 0.795 | 0.637 | 0.722 | 0.811 | 0.993 | 0.663 | 0.859 | 0.792 | |
5 | 0.449 | 0.635 | 0.811 | 0.711 | - | 0.811 | 0.741 | 0.463 | 0.651 | 0.812 | 0.738 | 0.991 | 0.830 | 0.769 | 0.465 | 0.653 | 0.811 | 0.742 | 0.988 | 0.833 | 0.772 | |
6 | 0.517 | 0.590 | 0.802 | 0.878 | 0.811 | - | 0.893 | 0.509 | 0.577 | 0.779 | 0.882 | 0.778 | 0.995 | 0.899 | 0.506 | 0.575 | 0.775 | 0.882 | 0.772 | 0.993 | 0.899 | |
7 | 0.419 | 0.520 | 0.731 | 0.803 | 0.741 | 0.893 | - | 0.409 | 0.506 | 0.699 | 0.801 | 0.698 | 0.876 | 0.995 | 0.406 | 0.503 | 0.693 | 0.800 | 0.690 | 0.872 | 0.993 | |
One Day Before | 8 | 0.990 | 0.783 | 0.713 | 0.642 | 0.463 | 0.509 | 0.409 | - | 0.796 | 0.702 | 0.662 | 0.448 | 0.516 | 0.418 | 0.999 | 0.796 | 0.698 | 0.665 | 0.444 | 0.517 | 0.418 |
9 | 0.792 | 0.991 | 0.744 | 0.727 | 0.651 | 0.577 | 0.506 | 0.796 | - | 0.727 | 0.746 | 0.634 | 0.588 | 0.517 | 0.795 | 0.999 | 0.723 | 0.749 | 0.629 | 0.588 | 0.517 | |
10 | 0.673 | 0.695 | 0.986 | 0.817 | 0.812 | 0.779 | 0.699 | 0.702 | 0.727 | - | 0.853 | 0.812 | 0.802 | 0.730 | 0.705 | 0.731 | 0.999 | 0.858 | 0.810 | 0.804 | 0.734 | |
11 | 0.674 | 0.759 | 0.881 | 0.994 | 0.738 | 0.882 | 0.801 | 0.662 | 0.746 | 0.853 | - | 0.706 | 0.876 | 0.801 | 0.659 | 0.743 | 0.847 | 0.999 | 0.700 | 0.874 | 0.800 | |
12 | 0.420 | 0.605 | 0.796 | 0.670 | 0.991 | 0.778 | 0.698 | 0.448 | 0.634 | 0.812 | 0.706 | - | 0.808 | 0.737 | 0.451 | 0.637 | 0.813 | 0.711 | 0.999 | 0.812 | 0.742 | |
13 | 0.516 | 0.591 | 0.815 | 0.862 | 0.830 | 0.995 | 0.876 | 0.516 | 0.588 | 0.802 | 0.876 | 0.808 | - | 0.892 | 0.515 | 0.586 | 0.798 | 0.878 | 0.803 | 0.999 | 0.893 | |
14 | 0.418 | 0.520 | 0.751 | 0.795 | 0.769 | 0.899 | 0.995 | 0.418 | 0.517 | 0.730 | 0.801 | 0.737 | 0.892 | - | 0.416 | 0.515 | 0.725 | 0.801 | 0.731 | 0.889 | 0.999 | |
Current Day | 15 | 0.987 | 0.780 | 0.714 | 0.637 | 0.465 | 0.506 | 0.406 | 0.999 | 0.795 | 0.705 | 0.659 | 0.451 | 0.515 | 0.416 | - | 0.796 | 0.702 | 0.662 | 0.448 | 0.516 | 0.417 |
16 | 0.791 | 0.988 | 0.746 | 0.722 | 0.653 | 0.575 | 0.503 | 0.796 | 0.999 | 0.731 | 0.743 | 0.637 | 0.586 | 0.515 | 0.796 | - | 0.727 | 0.746 | 0.634 | 0.587 | 0.516 | |
17 | 0.668 | 0.690 | 0.983 | 0.811 | 0.811 | 0.775 | 0.693 | 0.698 | 0.723 | 0.999 | 0.847 | 0.813 | 0.798 | 0.725 | 0.702 | 0.727 | - | 0.853 | 0.812 | 0.802 | 0.730 | |
18 | 0.676 | 0.760 | 0.885 | 0.993 | 0.742 | 0.882 | 0.800 | 0.665 | 0.749 | 0.858 | 0.999 | 0.711 | 0.878 | 0.801 | 0.662 | 0.746 | 0.853 | - | 0.705 | 0.876 | 0.800 | |
19 | 0.415 | 0.599 | 0.793 | 0.663 | 0.988 | 0.772 | 0.690 | 0.444 | 0.629 | 0.810 | 0.700 | 0.999 | 0.803 | 0.731 | 0.448 | 0.634 | 0.812 | 0.705 | - | 0.808 | 0.737 | |
20 | 0.515 | 0.590 | 0.816 | 0.859 | 0.833 | 0.993 | 0.872 | 0.517 | 0.588 | 0.804 | 0.874 | 0.812 | 0.999 | 0.889 | 0.516 | 0.587 | 0.802 | 0.876 | 0.808 | - | 0.891 | |
21 | 0.417 | 0.519 | 0.754 | 0.792 | 0.772 | 0.899 | 0.993 | 0.418 | 0.517 | 0.734 | 0.800 | 0.742 | 0.893 | 0.999 | 0.417 | 0.516 | 0.730 | 0.800 | 0.737 | 0.891 | - |
DAM | + WD + CD + HRD | HRD | |||||||
---|---|---|---|---|---|---|---|---|---|
MAPE | R | MSE | RMSE | MAPE | R | MSE | RMSE | ||
Daily Prediction | Ömerli | 0.00496996 | 0.999754 | 1.80904 × 10 | 0.00425328 | 0.00628446 | 0.999513 | 3.41411 × 10 | 0.00584303 |
Darlık | 0.00337642 | 0.999518 | 2.45226 × 10 | 0.00495203 | 0.00587575 | 0.999081 | 5.18837 × 10 | 0.00720303 | |
Elmalı | 0.0124002 | 0.997419 | 0.000192689 | 0.0138812 | 0.00957619 | 0.993972 | 0.000402123 | 0.020053 | |
Terkos | 0.00601339 | 0.999565 | 2.17048 × 10 | 0.00465884 | 0.00655399 | 0.999733 | 1.46284 × 10 | 0.00382471 | |
Alibey | 0.010467 | 0.997787 | 9.50388 × 10 | 0.00974879 | 0.0098421 | 0.998924 | 4.93963 × 10 | 0.00702825 | |
B.Çekmece | 0.0099909 | 0.999712 | 3.09754 × 10 | 0.00556555 | 0.00967341 | 0.99953 | 3.67732 × 10 | 0.00606409 | |
Sazlıdere | 0.00846746 | 0.999419 | 1.97302 × 10 | 0.00444186 | 0.00656699 | 0.999691 | 9.44456 × 10 | 0.0030732 | |
Weekly Prediction | Ömerli | 0.014751 | 0.996468 | 0.000172075 | 0.0131177 | 0.0155757 | 0.997482 | 0.000155052 | 0.012452 |
Darlık | 0.0152882 | 0.995803 | 0.000207155 | 0.0143929 | 0.0101446 | 0.998517 | 8.08303 × 10 | 0.00899057 | |
Elmalı | 0.0280571 | 0.990919 | 0.000700975 | 0.0264759 | 0.0245444 | 0.990563 | 0.000644626 | 0.0253895 | |
Terkos | 0.0190461 | 0.996293 | 0.000197625 | 0.0140579 | 0.0183852 | 0.996289 | 0.000189051 | 0.0137496 | |
Alibey | 0.0301914 | 0.995427 | 0.000202811 | 0.0142412 | 0.0290158 | 0.996204 | 0.00017287 | 0.013148 | |
B.Çekmece | 0.0235451 | 0.997413 | 0.000150072 | 0.0122504 | 0.028585 | 0.997076 | 0.000203382 | 0.0142612 | |
Sazlıdere | 0.0216837 | 0.996221 | 0.000112867 | 0.0106239 | 0.0374822 | 0.996799 | 0.000116965 | 0.010815 | |
15-Day Prediction | Ömerli | 0.0171061 | 0.993161 | 0.000322059 | 0.017946 | 0.0256526 | 0.993155 | 0.000484254 | 0.0220058 |
Darlık | 0.0188453 | 0.991981 | 0.000443239 | 0.0210532 | 0.0130614 | 0.998414 | 9.16712 × 10 | 0.00957451 | |
Elmalı | 0.0296606 | 0.983374 | 0.00105234 | 0.0324398 | 0.0302839 | 0.991372 | 0.000620677 | 0.0249134 | |
Terkos | 0.019051 | 0.996649 | 0.000168989 | 0.0129996 | 0.0249967 | 0.997302 | 0.000177895 | 0.0133377 | |
Alibey | 0.0348007 | 0.937953 | 0.00277279 | 0.0526573 | 0.0269181 | 0.993971 | 0.00025642 | 0.0160131 | |
B.Çekmece | 0.0397847 | 0.99153 | 0.000542264 | 0.0232866 | 0.0333638 | 0.994774 | 0.000308434 | 0.0175623 | |
Sazlıdere | 0.0261945 | 0.995932 | 0.000122505 | 0.0110682 | 0.0337267 | 0.993445 | 0.000195627 | 0.0139867 | |
Monthly Prediction | Ömerli | 0.012808 | 0.990179 | 0.000453095 | 0.021286 | 0.0224381 | 0.989099 | 0.000504953 | 0.0224712 |
Darlık | 0.0180395 | 0.99514 | 0.000242745 | 0.0155803 | 0.0118325 | 0.998626 | 0.000110456 | 0.0105098 | |
Elmalı | 0.0361916 | 0.984145 | 0.00101564 | 0.0318691 | 0.0196329 | 0.992456 | 0.000483806 | 0.0219956 | |
Terkos | 0.0188625 | 0.997037 | 0.000145501 | 0.0120624 | 0.0142386 | 0.998762 | 7.7495 × 10 | 0.00880313 | |
Alibey | 0.0207037 | 0.995571 | 0.000185797 | 0.0136307 | 0.017139 | 0.997741 | 9.65338 × 10 | 0.00982516 | |
B.Çekmece | 0.0267443 | 0.997734 | 0.000190004 | 0.0137842 | 0.0302374 | 0.998214 | 0.000113374 | 0.0106477 | |
Sazlıdere | 0.0265639 | 0.994443 | 0.000177235 | 0.013313 | 0.0245632 | 0.991577 | 0.000254904 | 0.0159657 |
DAM | + WD + CD + HRD | HRD | |||||||
---|---|---|---|---|---|---|---|---|---|
MAPE | R2 | MSE | RMSE | MAPE | R2 | MSE | RMSE | ||
Daily Prediction | Ömerli | 0.00312237 | 0.999746 | 1.16941 × 10 | 0.00341967 | 0.00305702 | 0.999428 | 2.62668 × 10 | 0.00512511 |
Darlık | 0.00290634 | 0.999763 | 1.15753 × 10 | 0.00340225 | 0.00292748 | 0.999498 | 2.45049 × 10 | 0.00495024 | |
Elmalı | 0.00783492 | 0.997509 | 0.000157006 | 0.0125302 | 0.00773108 | 0.995666 | 0.000273552 | 0.0165394 | |
Terkos | 0.00585859 | 0.999649 | 1.71972 × 10 | 0.00414695 | 0.0049944 | 0.999741 | 1.27535 × 10 | 0.00357121 | |
Alibey | 0.00896704 | 0.999456 | 2.29451 × 10 | 0.00479011 | 0.00705791 | 0.999152 | 3.57359 × 10 | 0.00597795 | |
B.Çekmece | 0.00708775 | 0.999662 | 1.90968 × 10 | 0.00436999 | 0.00692981 | 0.999618 | 2.15249 × 10 | 0.00463949 | |
Sazlıdere | 0.00663554 | 0.999785 | 6.35257 × 10 | 0.00252043 | 0.00611186 | 0.999673 | 9.82439 × 10 | 0.00313439 | |
Weekly Prediction | Ömerli | 0.0264899 | 0.987316 | 0.000589272 | 0.0242749 | 0.0249001 | 0.989311 | 0.000499273 | 0.0223444 |
Darlık | 0.0234549 | 0.990203 | 0.000478221 | 0.0218683 | 0.0233937 | 0.99007 | 0.00048584 | 0.0220418 | |
Elmalı | 0.0361511 | 0.979043 | 0.00132487 | 0.0363988 | 0.0438259 | 0.978049 | 0.00140182 | 0.0374409 | |
Terkos | 0.0284242 | 0.991094 | 0.000436925 | 0.0209027 | 0.0304353 | 0.989684 | 0.000505364 | 0.0224803 | |
Alibey | 0.0454819 | 0.987379 | 0.000530643 | 0.0230357 | 0.0468775 | 0.985498 | 0.000608809 | 0.0246741 | |
B.Çekmece | 0.029464 | 0.993387 | 0.00037363 | 0.0193295 | 0.0357698 | 0.992313 | 0.00043693 | 0.0209029 | |
Sazlıdere | 0.0351539 | 0.993555 | 0.000191085 | 0.0138233 | 0.0353401 | 0.993112 | 0.000204982 | 0.0143172 | |
15-Day Prediction | Ömerli | 0.0597648 | 0.956384 | 0.00203142 | 0.0450713 | 0.0562393 | 0.959656 | 0.00188994 | 0.0434734 |
Darlık | 0.0475681 | 0.970973 | 0.00143549 | 0.0378879 | 0.0488463 | 0.967032 | 0.00161382 | 0.0401724 | |
Elmalı | 0.0749328 | 0.930958 | 0.00436855 | 0.066095 | 0.0816497 | 0.920174 | 0.00506405 | 0.0711621 | |
Terkos | 0.0565462 | 0.976715 | 0.0011414 | 0.0337846 | 0.0627889 | 0.974832 | 0.00123485 | 0.0351404 | |
Alibey | 0.0958814 | 0.953379 | 0.00196719 | 0.044353 | 0.101744 | 0.951644 | 0.00206112 | 0.0453995 | |
B.Çekmece | 0.0649254 | 0.984549 | 0.000877351 | 0.0296201 | 0.0746038 | 0.982518 | 0.00100417 | 0.0316886 | |
Sazlıdere | 0.0863225 | 0.977034 | 0.000680156 | 0.0260798 | 0.0823396 | 0.978331 | 0.000645584 | 0.0254084 | |
Monthly Prediction | Ömerli | 0.115295 | 0.840763 | 0.00730451 | 0.0854664 | 0.117424 | 0.832343 | 0.00769196 | 0.0877038 |
Darlık | 0.096027 | 0.882832 | 0.00572044 | 0.0756336 | 0.101429 | 0.868235 | 0.00644469 | 0.0802788 | |
Elmalı | 0.139233 | 0.78605 | 0.0135715 | 0.116497 | 0.152306 | 0.762178 | 0.0151442 | 0.123062 | |
Terkos | 0.100622 | 0.927497 | 0.00355449 | 0.0596195 | 0.108371 | 0.921444 | 0.00386874 | 0.0621992 | |
Alibey | 0.170993 | 0.834706 | 0.00696293 | 0.0834442 | 0.170672 | 0.81118 | 0.00804831 | 0.0897124 | |
B.Çekmece | 0.152902 | 0.923799 | 0.00433834 | 0.0658661 | 0.140405 | 0.925902 | 0.0041832 | 0.0646777 | |
Sazlıdere | 0.15938 | 0.903594 | 0.00285824 | 0.0534625 | 0.162844 | 0.893396 | 0.00317065 | 0.0563085 |
DAM | + WD + CD + HRD | HRD | |||||||
---|---|---|---|---|---|---|---|---|---|
MAPE | R2 | MSE | RMSE | MAPE | R2 | MSE | RMSE | ||
Daily Prediction | Ömerli | 0.00290696 | 0.999728 | 1.25172 × 10 | 0.00353797 | 0.00305458 | 0.999455 | 2.50039 × 10 | 0.00500039 |
Darlık | 0.00293826 | 0.999738 | 1.27733 × 10 | 0.00357398 | 0.00293714 | 0.999546 | 2.22023 × 10 | 0.00471193 | |
Elmalı | 0.00770551 | 0.997462 | 0.00015997 | 0.0126479 | 0.00778144 | 0.995675 | 0.000272933 | 0.0165207 | |
Terkos | 0.00398731 | 0.999833 | 8.20228 × 10 | 0.00286396 | 0.00496261 | 0.999749 | 1.23182 × 10 | 0.00350973 | |
Alibey | 0.00682897 | 0.999635 | 1.54796 × 10 | 0.00393441 | 0.00708108 | 0.999156 | 3.55807 × 10 | 0.00596496 | |
B.Çekmece | 0.00596853 | 0.999819 | 1.02405 × 10 | 0.00320008 | 0.00690164 | 0.999618 | 2.15236 × 10 | 0.00463935 | |
Sazlıdere | 0.00519053 | 0.999801 | 5.93328 × 10 | 0.00243583 | 0.00605372 | 0.999724 | 8.29238 × 10 | 0.00287965 | |
Weekly Prediction | Ömerli | 0.0232363 | 0.989629 | 0.000479234 | 0.0218914 | 0.0250712 | 0.989269 | 0.000501665 | 0.0223979 |
Darlık | 0.0216811 | 0.992009 | 0.000389158 | 0.0197271 | 0.0233964 | 0.99009 | 0.000484886 | 0.0220201 | |
Elmalı | 0.0360924 | 0.979585 | 0.00129005 | 0.0359173 | 0.0442911 | 0.977976 | 0.00140899 | 0.0375365 | |
Terkos | 0.0280822 | 0.991154 | 0.00043457 | 0.0208463 | 0.030388 | 0.989652 | 0.000506874 | 0.0225139 | |
Alibey | 0.0421883 | 0.988026 | 0.000504023 | 0.0224505 | 0.0468775 | 0.985499 | 0.000608808 | 0.024674 | |
B.Çekmece | 0.0303865 | 0.993051 | 0.000393111 | 0.019827 | 0.03585 | 0.992356 | 0.000434515 | 0.020845 | |
Sazlıdere | 0.0315555 | 0.993665 | 0.000187303 | 0.0136859 | 0.0353786 | 0.993109 | 0.000205063 | 0.01432 | |
15-Day Prediction | Ömerli | 0.0533375 | 0.964082 | 0.00169918 | 0.0412211 | 0.0562392 | 0.959656 | 0.00188994 | 0.0434734 |
Darlık | 0.0457542 | 0.972184 | 0.00137807 | 0.0371224 | 0.0494345 | 0.966858 | 0.00162141 | 0.0402667 | |
Elmalı | 0.0743163 | 0.933334 | 0.00423471 | 0.0650746 | 0.0815954 | 0.919795 | 0.00508274 | 0.0712933 | |
Terkos | 0.0540278 | 0.978052 | 0.00107749 | 0.0328252 | 0.0630082 | 0.974824 | 0.00123525 | 0.0351461 | |
Alibey | 0.0920288 | 0.959514 | 0.0017189 | 0.0414596 | 0.101746 | 0.951492 | 0.00206217 | 0.0454111 | |
B.Çekmece | 0.0617624 | 0.985375 | 0.000839167 | 0.0289684 | 0.0746552 | 0.982521 | 0.00100362 | 0.0316799 | |
Sazlıdere | 0.0781948 | 0.980241 | 0.000591595 | 0.0243227 | 0.082677 | 0.978315 | 0.000645751 | 0.0254116 | |
Monthly Prediction | Ömerli | 0.104782 | 0.86507 | 0.00619161 | 0.0786868 | 0.117424 | 0.832343 | 0.00769196 | 0.0877038 |
Darlık | 0.0906292 | 0.896114 | 0.00507109 | 0.0712116 | 0.101429 | 0.868235 | 0.00644469 | 0.0802788 | |
Elmalı | 0.132347 | 0.791852 | 0.0132286 | 0.115016 | 0.153111 | 0.761777 | 0.0151531 | 0.123098 | |
Terkos | 0.0940711 | 0.935074 | 0.0031903 | 0.0564828 | 0.108759 | 0.921323 | 0.0038717 | 0.062223 | |
Alibey | 0.15876 | 0.842755 | 0.00664744 | 0.0815318 | 0.170872 | 0.811342 | 0.00803104 | 0.0896161 | |
B.Çekmece | 0.115172 | 0.940945 | 0.00332585 | 0.0576702 | 0.140404 | 0.925902 | 0.0041832 | 0.0646777 | |
Sazlıdere | 0.148663 | 0.911828 | 0.00260789 | 0.0510675 | 0.162844 | 0.893396 | 0.00317065 | 0.0563085 |
DAM | + WD + CD + HRD | HRD | |||||||
---|---|---|---|---|---|---|---|---|---|
MAPE | R2 | MSE | RMSE | MAPE | R2 | MSE | RMSE | ||
Daily Prediction | Ömerli | 0.00478378 | 0.999315 | 3.14498 × 10 | 0.00560801 | 0.00463194 | 0.999336 | 3.05549 × 10 | 0.00552765 |
Darlık | 0.00451081 | 0.999451 | 2.67768 × 10 | 0.00517463 | 0.00483447 | 0.999142 | 4.17943 × 10 | 0.00646485 | |
Elmalı | 0.00784472 | 0.995614 | 0.00027652 | 0.0166289 | 0.00866333 | 0.995561 | 0.000279763 | 0.0167261 | |
Terkos | 0.00702186 | 0.999559 | 2.1677 × 10 | 0.00465586 | 0.00765764 | 0.999522 | 2.3537 × 10 | 0.0048515 | |
Alibey | 0.00972596 | 0.998967 | 4.3398 × 10 | 0.00658772 | 0.0102261 | 0.998795 | 5.05776 × 10 | 0.00711179 | |
B.Çekmece | 0.00893934 | 0.999442 | 3.1638 × 10 | 0.00562477 | 0.00946453 | 0.999326 | 3.81665 × 10 | 0.00617791 | |
Sazlıdere | 0.00773455 | 0.999674 | 9.58492 × 10 | 0.00309595 | 0.00895021 | 0.999584 | 1.22647 × 10 | 0.0035021 | |
Weekly Prediction | Ömerli | 0.0116603 | 0.997192 | 0.000129324 | 0.0113721 | 0.012716 | 0.996169 | 0.000176682 | 0.0132922 |
Darlık | 0.00935386 | 0.99813 | 9.12824 × 10 | 0.00955418 | 0.0107126 | 0.997725 | 0.000112508 | 0.010607 | |
Elmalı | 0.016616 | 0.994286 | 0.000362236 | 0.0190325 | 0.0184659 | 0.993695 | 0.00039896 | 0.019974 | |
Terkos | 0.0136593 | 0.997804 | 0.000107734 | 0.0103795 | 0.0117802 | 0.998326 | 8.20781 × 10 | 0.0090597 | |
Alibey | 0.0209691 | 0.99649 | 0.000147373 | 0.0121397 | 0.0219477 | 0.996266 | 0.000157022 | 0.0125308 | |
B.Çekmece | 0.0180959 | 0.996682 | 0.000187673 | 0.0136994 | 0.0165157 | 0.997882 | 0.000119767 | 0.0109438 | |
Sazlıdere | 0.0140014 | 0.99839 | 4.75184 × 10 | 0.00689336 | 0.0143782 | 0.998286 | 5.06218 × 10 | 0.0071149 | |
15-Day Prediction | Ömerli | 0.0163452 | 0.99262 | 0.000338375 | 0.018395 | 0.0154685 | 0.992315 | 0.000352475 | 0.0187743 |
Darlık | 0.0139163 | 0.995521 | 0.000219014 | 0.0147991 | 0.0153309 | 0.994914 | 0.000248236 | 0.0157555 | |
Elmalı | 0.0141098 | 0.997065 | 0.000186092 | 0.0136416 | 0.0178281 | 0.995037 | 0.000313711 | 0.0177119 | |
Terkos | 0.0128188 | 0.997946 | 0.000100647 | 0.0100323 | 0.011547 | 0.998247 | 8.60822 × 10 | 0.00927805 | |
Alibey | 0.0256763 | 0.992852 | 0.000301602 | 0.0173667 | 0.0276765 | 0.993673 | 0.000265811 | 0.0163037 | |
B.Çekmece | 0.0175794 | 0.995509 | 0.000256572 | 0.0160179 | 0.0183209 | 0.99189 | 0.000465323 | 0.0215714 | |
Sazlıdere | 0.0168793 | 0.99781 | 6.45727 × 10 | 0.00803571 | 0.0183391 | 0.99753 | 7.27287 × 10 | 0.00852811 | |
Monthly Prediction | Ömerli | 0.0170516 | 0.988768 | 0.000523402 | 0.022878 | 0.0144575 | 0.991073 | 0.000413032 | 0.0203232 |
Darlık | 0.0195599 | 0.991975 | 0.000397903 | 0.0199475 | 0.0204259 | 0.987695 | 0.000599909 | 0.024493 | |
Elmalı | 0.0155737 | 0.995631 | 0.000276242 | 0.0166205 | 0.0200283 | 0.989257 | 0.000678663 | 0.0260512 | |
Terkos | 0.0163963 | 0.996502 | 0.000171737 | 0.0131048 | 0.0103605 | 0.99769 | 0.00011409 | 0.0106813 | |
Alibey | 0.020811 | 0.994479 | 0.000233697 | 0.0152871 | 0.0198052 | 0.993651 | 0.000268836 | 0.0163962 | |
B.Çekmece | 0.019981 | 0.997114 | 0.000168074 | 0.0129643 | 0.0139536 | 0.998958 | 6.0931 × 10 | 0.00780583 | |
Sazlıdere | 0.0222951 | 0.990182 | 0.000292531 | 0.0171036 | 0.0202211 | 0.988411 | 0.000347147 | 0.0186319 |
DAM | + WD + CD + HRD | HRD | |||||||
---|---|---|---|---|---|---|---|---|---|
MAPE | R2 | MSE | RMSE | MAPE | R2 | MSE | RMSE | ||
Daily Prediction | Ömerli | 0.00388508 | 0.999396 | 2.77199 × 10 | 0.00526497 | 0.00409498 | 0.999321 | 3.11635 × 10 | 0.00558243 |
Darlık | 0.00316241 | 0.999506 | 2.41255 × 10 | 0.00491177 | 0.00419955 | 0.99908 | 4.47778 × 10 | 0.00669163 | |
Elmalı | 0.00787504 | 0.995719 | 0.00026978 | 0.016425 | 0.00817141 | 0.995634 | 0.000275109 | 0.0165864 | |
Terkos | 0.00539017 | 0.99952 | 2.35185 × 10 | 0.00484958 | 0.00689247 | 0.999458 | 2.66004 × 10 | 0.00515756 | |
Alibey | 0.00898673 | 0.99892 | 4.5326 × 10 | 0.00673246 | 0.00940576 | 0.99884 | 4.86806 × 10 | 0.00697715 | |
B.Çekmece | 0.00792777 | 0.99922 | 4.40525 × 10 | 0.00663721 | 0.00901684 | 0.999183 | 4.62988 × 10 | 0.00680433 | |
Sazlıdere | 0.00585024 | 0.999691 | 9.11219 × 10 | 0.00301864 | 0.00813567 | 0.999521 | 1.41438 × 10 | 0.00376083 | |
Weekly Prediction | Ömerli | 0.00847044 | 0.998648 | 6.28527 × 10 | 0.00792797 | 0.00840095 | 0.998775 | 5.71785 × 10 | 0.00756165 |
Darlık | 0.00837819 | 0.998271 | 8.51257 × 10 | 0.00922636 | 0.0085435 | 0.998717 | 6.50426 × 10 | 0.0080649 | |
Elmalı | 0.011828 | 0.997304 | 0.000170395 | 0.0130535 | 0.0145746 | 0.995954 | 0.000256698 | 0.0160218 | |
Terkos | 0.00810674 | 0.99879 | 5.91995 × 10 | 0.00769412 | 0.00790464 | 0.998976 | 5.0213 × 10 | 0.00708611 | |
Alibey | 0.0137394 | 0.998191 | 7.60133 × 10 | 0.00871856 | 0.0147247 | 0.998268 | 7.28824 × 10 | 0.00853712 | |
B.Çekmece | 0.0114554 | 0.998938 | 6.02968 × 10 | 0.0077651 | 0.0103776 | 0.99894 | 6.06342 × 10 | 0.00778679 | |
Sazlıdere | 0.0100015 | 0.999083 | 2.71342 × 10 | 0.00520905 | 0.00966369 | 0.999046 | 2.81089 × 10 | 0.00530178 | |
15-Day Prediction | Ömerli | 0.009211 | 0.997901 | 9.69083 × 10 | 0.0098442 | 0.00885978 | 0.996761 | 0.000148606 | 0.0121904 |
Darlık | 0.0105688 | 0.997329 | 0.000134036 | 0.0115774 | 0.00965656 | 0.998596 | 7.19347 × 10 | 0.00848143 | |
Elmalı | 0.0118076 | 0.996929 | 0.000195466 | 0.0139809 | 0.0121011 | 0.996713 | 0.000207453 | 0.0144032 | |
Terkos | 0.00792414 | 0.999192 | 3.98185 × 10 | 0.00631019 | 0.00846756 | 0.998901 | 5.38046 × 10 | 0.00733517 | |
Alibey | 0.0167746 | 0.99691 | 0.000129863 | 0.0113957 | 0.0156182 | 0.997685 | 9.71879 × 10 | 0.00985839 | |
B.Çekmece | 0.00992255 | 0.999053 | 5.40958 × 10 | 0.00735499 | 0.0110676 | 0.997837 | 0.00012336 | 0.0111068 | |
Sazlıdere | 0.0105529 | 0.998642 | 4.02893 × 10 | 0.00634738 | 0.0110314 | 0.998534 | 4.32387 × 10 | 0.00657561 | |
Monthly Prediction | Ömerli | 0.0119018 | 0.995007 | 0.000232166 | 0.015237 | 0.0065634 | 0.997882 | 9.80702 × 10 | 0.00990304 |
Darlık | 0.0131245 | 0.995145 | 0.000240917 | 0.0155215 | 0.0112884 | 0.994835 | 0.000251898 | 0.0158713 | |
Elmalı | 0.0115828 | 0.997537 | 0.000156458 | 0.0125083 | 0.0111112 | 0.997498 | 0.000158287 | 0.0125812 | |
Terkos | 0.00872503 | 0.998963 | 5.12378 × 10 | 0.00715806 | 0.00647818 | 0.999267 | 3.62939 × 10 | 0.00602444 | |
Alibey | 0.015773 | 0.997818 | 9.30908 × 10 | 0.00964836 | 0.0133573 | 0.997926 | 8.80544 × 10 | 0.00938373 | |
B.Çekmece | 0.0100021 | 0.999439 | 3.2496 × 10 | 0.00570052 | 0.00723422 | 0.999597 | 2.27595 × 10 | 0.00477069 | |
Sazlıdere | 0.0145424 | 0.996183 | 0.00011351 | 0.0106541 | 0.0137383 | 0.993712 | 0.00018706 | 0.013677 |
Test | Dataset | p-Value | Statistic | Description |
---|---|---|---|---|
Kolmogorov–Smirnov | , WD, CD | 4.74 × 10 | 0.5012 | Evaluation of the fit to the normal distribution |
Kolmogorov–Smirnov | 4.73 × 10 | 0.5012 | ||
Z-Test (two-sided) | MAPE of the , WD, CD HRD model, MAPE of the HRD model | 0.0155 | −2.4197 | Assessment of performance equivalence |
Z-Test (less than) | MAPE of the , WD, CD HRD model, MAPE of the HRD model | 0.0078 | −2.4197 | To validate that the additional data reduced the error rate |
Paired T-Test (less than) | MAPE of the , WD, CD HRD model, MAPE of the HRD model | 2.59 × 10 | −4.742 (DF: 139) | To validate that the additional data reduced the error rate |
Paired T-Test (less than) | MAPE of the LLCV model and MAPE of the OMPCV model | 0.0008 | −3.464 (DF:27) | To compare the performance of LLCV and OMPCV |
Paired T-Test (less than) | MAPE of the LSTM model and MAPE of the LLCV model | 5.535 × 10 | −4.52 (DF:27) | To compare the performance of LSTM and LLCV |
Paired T-Test (less than) | MAPE of the RF model and MAPE of the LSTM model | 6.54 × 10 | −4.457 (DF:27) | To compare the performance of RF and LSTM |
Paired T-Test (less than) | MAPE of the ET model and MAPE of the RF model | 2.085 × 10 | −8.493 (DF:27) | To compare the performance of ET and RF |
The statements, opinions and data contained in all publications are solely those of the individual author(s) and contributor(s) and not of MDPI and/or the editor(s). MDPI and/or the editor(s) disclaim responsibility for any injury to people or property resulting from any ideas, methods, instructions or products referred to in the content. |
Share and Cite
Badem, A.C.; Yılmaz, R.; Cesur, M.R.; Cesur, E. Advanced Predictive Modeling for Dam Occupancy Using Historical and Meteorological Data. Sustainability 2024 , 16 , 7696. https://doi.org/10.3390/su16177696
Badem AC, Yılmaz R, Cesur MR, Cesur E. Advanced Predictive Modeling for Dam Occupancy Using Historical and Meteorological Data. Sustainability . 2024; 16(17):7696. https://doi.org/10.3390/su16177696
Badem, Ahmet Cemkut, Recep Yılmaz, Muhammet Raşit Cesur, and Elif Cesur. 2024. "Advanced Predictive Modeling for Dam Occupancy Using Historical and Meteorological Data" Sustainability 16, no. 17: 7696. https://doi.org/10.3390/su16177696
Article Metrics
Further information, mdpi initiatives, follow mdpi.
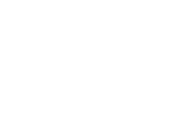
Subscribe to receive issue release notifications and newsletters from MDPI journals

An official website of the United States government
The .gov means it’s official. Federal government websites often end in .gov or .mil. Before sharing sensitive information, make sure you’re on a federal government site.
The site is secure. The https:// ensures that you are connecting to the official website and that any information you provide is encrypted and transmitted securely.
- Publications
- Account settings
Preview improvements coming to the PMC website in October 2024. Learn More or Try it out now .
- Advanced Search
- Journal List
- Indian Dermatol Online J
- v.10(5); Sep-Oct 2019
Selection of Control, Randomization, Blinding, and Allocation Concealment
Department of Pharmacology, Rampurhat Government Medical College, Rampurhat, Birbhum, West Bengal, India
Piyush Kumar
1 Department of Dermatology, Katihar Medical College and Hospital, Bihar, India
Rajesh Kumar
2 Department of Dermatology, Grant Medical College and Bombay Hospital Institute of Medical Sciences, Mumbai, Maharashtra, India
Nilay Kanti Das
3 Department of Dermatology, Bankura Sammilani Medical College, Kenduadihi, Bankura, West Bengal, India
Clinical trials looking at which treatment is better must have certain checks in place. Appropriate “control” selection while comparing the investigating agent to the “control group is essential to rule out selection bias. Randomization is another step to minimize variability or “confounders.” By randomization, research participants have an equal chance of being selected into any treatment group of the study, generating comparable intervention groups, thereby distributing the confounders. A trial can be “open labeled” or “blinded.” By the process of blinding, we make the participant and/or assessing physician unaware of the treatment he/she is going to receive. Thus, the element of bias which can creep in owing to personal preference or subjective component to the assessment of outcome can be eliminated. Concealment of allocation is done as the participant enters the trial. Concealment secures randomization and prevents “selection bias”.
Introduction
Clinical trials or interventional studies are to be designed in such a way that it gives a comprehensive idea about the effectiveness/efficacy or safety of any new agent introduced for the treatment of any clinical condition. To understand the fact that the improvement (or deterioration) is not happening by chance , it is essential that the treatment modality is compared against another modality of treatment (active control) or no treatment (placebo control). Thus, the role of having control is paramount, and it decides the level of evidence of any trial and in-turn decides the grade of recommendation.
Apart from having control, there is another important factor which can affect the interpretation of result in any clinical trial, and this factor is “bias.” The bias can be while selecting the participant and the control (selection bias), owing to the confounding factors (confounding bias) and also while assessing the outcome (assessment bias). Randomization is the method adopted to eliminate the bias of selection and confounder. It has got two steps; generation of random number and concealment of the random number from the dispensing physician (allocation concealment). For eliminating the assessment bias, the method adopted is blinding and it can be done at a different level using the participant of the trial, assessing physician, and even the statistician analyzing the results.
The article will attempt in elaborating on these facets of clinical trial and impart practical clues to implement the same.
A. Selection of control- The “control” is used in clinical trials to nullify the effect of known or unknown factors (other than the factor being tested) on the research outcome and hence, to increase the reliability of the results. For example, if a new topical medication is shown to be effective in psoriasis patient, the inclusion of “control” in the study allows the investigator to conclude that the new medication is truly effective and improvement did not happen by chance.
Controls in case-control studies:
- While choosing control, two principles should be followed:[ 1 ]
- The control or the comparison group should be representative of the source population from which cases are derived
- The controls should be independent of the exposure, i.e., less likely to have the exposure of interest.
For e.g., to test the role of “topical corticosteroids causing the unresponsiveness to standard antifungal therapy” it is essential that case (those who have used steroid) and control (those who have not) be chosen from the same socio-cultural strata to eliminate the confounders such as hot and humid working environment, cleanliness, etc. This prevents “selection bias.” Thus, a control minimizes the effects of variables other than the variable under evaluation.
Controls in clinical trials:
In making a decision about a new treatment, the control arm is usually taken as the “gold standard treatment” (or the “best available treatment”). Comparison between the “test” arm (or “experimental” arm) and the “control” arm in such clinical studies makes a fruitful assessment of the new treatment compared with the previous one and increases the reliability of the study.
- Placebo control: A placebo is an inactive substance that looks like the drug or treatment being tested.[ 2 ] A placebo control may be used where no standard treatment exists or else using a placebo control becomes unethical and substandard care in patients with active disease, where there is an approved treatment. Guidelines state that there should be a condition of “clinical equipoise” before a placebo-controlled trial is started. Clinical equipoise assumes that there exists not one better intervention either for the control or experimental group during the design of the trial, e.g., a trial on systemic sclerosis by using an experimental drug understanding the fact that there is no gold-standard therapy for systemic sclerosis.[ 3 ] The use of “active” control or “historical” control (stated below) can address this issue. Participants who are going to receive placebo are not going to benefit from the trial. This therapeutic misconception should be eliminated during the informed consent process.
- Dose-response control: A new dose for a known drug makes it a “new drug.”[ 4 ] During its clinical trial, the control is usually the previously used dose. For e.g., 10 mg levocetirizine compared to 5 mg levocetirizine
- Active control: Here, the control is an active drug, usually the standard therapy or known effective treatment
- Historical control: Such control uses data from previously conducted studies and administrative databases. The studies that can be chosen for historical control can be a prospective natural history study or a control group from a previous randomized controlled trial.
B. Randomization
Minimizing variability of evaluation is the core of conducting good research or experiment. This variability is also known as “confounders.” Confounders can be known or unknown. Confounders have the possibility of generating erroneous results because of the unknown effects of unmeasured variables. The process by which confounders can be reduced is known as “randomization.” By randomization, research participants have an equal chance of being selected into any treatment group of the study, generating comparable intervention groups, thereby distributing the confounders. The confounders can, therefore, be ignored. Thus, the difference in outcome and results can be explained by treatment alone. However, if one wants to gain greater experience using a new treatment or drug, one may opt for “Unequal randomization”- randomization in 2:1 ratio (2/3 of patients on new treatment). The power of the study does get reduced [power decreases from 0.95 (for 1:1) to 0.925 (for 2:1)], but this technique is statistically feasible and is especially suited to phase II randomized trials.
Benefits of randomization:
- Balances the treatment groups with respect to baseline variability, known, and unknown confounding factors, thus eliminates “confounding bias”
- Eliminates “selection bias.” Selection bias occurs when the researcher voluntarily or involuntarily steers the less sick patients to the treatment he feels is better and vice versa
- Forms the basis for statistical tests.
How to randomize?
- Computer generated random number table. The statistical software has a provision of choosing equal or unequal randomized groups, choosing stratification, etc
- Random number table from statistical textbooks
- For smaller experiments: tossing coins (heads-control and tails-treatment), roll of dice (≤3-treatment and >3-control), and shuffled deck of cards (even-Group A and odd-Group B). However, these methods are replaced by the aforementioned methods.
What is not randomization?
- Alternate assignment: Study participants alternatively assigned to treatment, e.g., Odd numbers go to Treatment A and even numbers go to Treatment B
- Assignment according to the date of entry to study, e.g., 2 weeks of the month to Group A, next 2 weeks to Group B
- Assignment according to the days of the week, e.g., Monday OPD patients to Group A, Wednesday OPD patients to Group B. This gives rise to Berksonian bias.
Techniques for randomization
- Simple randomization: Randomization according to a single sequence of random assignments is known as simple randomization.[ 5 ] Assignment to the treatment groups is random and not concerned with other variables. For e.g. toss of coin, roll of dice, etc. This is the most simple and easy approach of randomization. In clinical studies with large sample size (at least 1,000 participants), simple randomization usually balances the number of subjects in each group. However, simple randomization could be problematic in smaller samples resulting in an unequal number of participants in treatment arms
e.g., Block randomization of two treatment groups A and B, number of blocks = 5, size of blocks = 10, and fixed size blocks.
1: A 2: B 3: B 4: A 5: A 6: B 7: B 8: A 9: B 10: A
1: A 2: B 3: B 4: B 5: A 6: B 7: A 8: A 9: B 10: A
1: A 2: B 3: B 4: A 5: B 6: A 7: B 8: A 9: A 10: B
1: A 2: B 3: B 4: B 5: B 6: A 7: A 8: A 9: A 10: B
1: B 2: A 3: B 4: A 5: B 6: A 7: B 8: B 9: A 10: A
- Stratified randomization: When specific variables are known to influence the outcome, stratification of the sample is required to keep the variables (e.g., age, gender, weight, prognostic status, etc) as similar as possible in between the treatment groups. This method achieves a balance between baseline characteristics. At first, variable is identified, strata is created. Participants are assigned to strata. Simple randomization is then applied to each stratum to assign subjects to either group, e.g. in case of assessing results of immunotherapy for viral warts, stratification can be done with respect to the types of warts viz. verruca vulgaris, verruca plana, plantar wart, condyloma acuminata.
- Cluster randomization: This method randomizes groups of people instead of individuals. This method is also known as “group randomization.” Cluster randomization is particularly favored to avoid complaint among the group of people living in close vicinity, e.g., vaccine trials where all participants of the same locality receive the same vaccine, lifestyle modification studies, and studies involving nutritional interventions. Here, sampling units are groups and not individuals.
C. Blinding
A trial can be “open labeled” or “blinded”. By the process of blinding, we make the participant and/or assessing physician unaware of the treatment he/she is going to receive. Thus, the element of bias which can creep in owing to personal preference or subjective component to the assessment of outcome (e.g., a tool like physician global score is used to assess the outcome) can be eliminated. The process has now been further extended to include the statistician analyzing the result to make it fool proof. Thus, blinding is helpful in eliminating intentional or unintentional bias, increasing the objectivity of results, and ensuring the credibility of study conclusions.
Types of blinding:
- Open-labeled or unblinded: All parties involved in a study are aware of the treatment the participants are receiving. Although blinding is desirable, sometimes it may not be possible or feasible. This type of study design suffers from low credibility but may be acceptable if endpoints are indisputably objective (e.g., survival or death)
- Single-blind: The participants in a study might drop out from study or might give false assessment if they come to know that they are receiving “no treatment.” In addition, they might develop a placebo effect, if they know they are receiving “new treatment.” All these biases can be eliminated by single-blinding. In this, a group of individuals (usually the participants) do not know the intervention he or she is going to receive. Conventionally, it refers to participant-blinded but logically the group of individuals blinded can also be the outcome assessor. Thus, a single-blind trial can be either participant-blind or assessor-blind, and it is better to specify who is blinded, instead of saying single-blind
- Double-blind: Like participants, the investigator/observer may influence the results of the study, if they are aware if a group of individuals are receiving a particular treatment. For example, if the endpoint is subjective (e.g. physician global scale), they might record a more favorable response for treatment of their preference. In addition, they might influence participants’ assessment of a particular treatment during follow-up meetings. In double-blinding, neither the participant nor the investigator/observer/outcome assessor is aware of the treatment allotted. The investigator is the person carrying out the research. The observer or the outcome assessor is the person who assesses the parameters of the study
- Triple-blind: Triple-blinding is done to eliminate the bias of data analysts. In triple-blinding, the participant, investigator, and the data analyst are unaware of the treatment given.
However, instead of expressing whether the trial is single, double, or triple blinded, it is more pertinent to specify who exactly is going to be blinded.
Masking: It is a term used interchangeably with “blinding” and is usually used by ophthalmologists.
Advantages of blinding:
- Avoids observation bias. For e.g., during the evaluation of a subjective score like urticaria severity score, blinding prevents favoring of the test drug by the investigator
- Can also reduce the opportunity for bias to enter into the evaluation of the trial results owing to the knowledge of the treatment.
Procedures of blinding a trial:
- By using identical looking dosage forms, for e.g. in a placebo-controlled trial, the placebo used should be similar looking in shape, size, color, and odor as that of the active drug
Investigational group = Active drug + Placebo
Control group = Placebo + Active control
- Both the active drugs can be taken out from their packaging and repacked in similar looking opaque containers. The containers can be labeled according to randomization
- The observer can be blinded by separating the room where the person is dispensing the drug and the person observing the effects of the drug.
Assessment of the efficacy of blinding:
There might be untoward effects in which the trial can be unblinded. The curiosity of participants or staff, differences in taste and smell of the drug, and placebo or a cross-over study are such instances. Ideal placebos are not always easy to procure or manufacture. Thus, the assessment of blinding should be done prior to the decoding of randomization. The participants, investigators, and staff are asked to guess what the participants had received. If the guess is 50% in each group, blinding has been maintained. If the guess is >50%, there had been a breach in blinding. If guess is <50% a suspicion about non-admittance of breach of blinding should be made.
Instances when blinding may be broken:
- The study is completed and data analyzed
- For individual patient during an emergency, e.g., road traffic accident due to an antihistamine trial in urticaria, participant in psoriasis trial progressing to erythroderma.
Instances when blinding is not possible or difficult to achieve:
- A surgical discipline is tested against a medical therapy, e.g., electrosurgery in pyogenic granuloma is tested against topical timolol
- “Sham procedure” is the improvisation, while working with surgical therapy that can be utilized to make it blinded, e.g., creating a dermal pocket without introducing any warty tissue against a regular auto-inoculation of wart can be done to make the placebo-arm blinded.[ 8 ] Ethical issues limits it uses of sham procedure, but in this instance, the authors have argued to have used the procedure to rule out the role of psychological effect, which has proven its role in wart therapy.
What to do if blinding is not possible or ethical:
- Researchers should ensure that the outcomes being measured are as objective as possible
- In addition, a duplicate assessment of outcome may be considered and researchers should report the level of agreement achieved
- Expertise-based trial design- It can be done for surgical procedures, where patients are randomly assigned to different surgeons
- Partial blinding- Sometimes, independent blinded evaluators may be sufficient to reduce bias
- Limitations and potential biases due to lack of blinding need to be acknowledged and discussed.
D. Allocation concealment
Concealment of allocation is done as the participant enters the trial. Concealment secures randomization and prevents “selection bias.”
Every researcher tries to prove his hypothesis as correct. This can lead to conscious or unconscious steering of certain “good” patients to the desired group and others to the alternate group. If the investigator knows the randomization, such bias can lead to an imbalance in the study and wrong conclusions can be drawn. Such bias can be avoided by the following allocation of concealment techniques:
- Third party randomization by phone or pharmacy. In large multi-centric trials, interactive-voice-response-service is used to ensure the allocation concealment in different centers
- Sequentially numbered, opaque, sealed envelope (SNOSE) technique: the randomization group is written on a paper and is kept in an opaque sealed envelope. The envelope is labeled with a serial number. The investigator opens the sealed envelope once the patient has consented to participate and then assigns the treatment group accordingly
- Sequentially numbered opaque containers: Similar to SNOSE, but here, instead of a piece of paper, the medicines are stored in opaque containers according to randomization, and there is no possibility of the dispenser to know which medicine is kept in which container.[ 9 ] Thus, the allocation is concealed.
E. Differences between allocation concealment and blinding
Allocation concealment | Blinding | |
---|---|---|
Purpose | Conceals randomization sequence | Makes participant or investigator or both unaware of the treatment received |
Bias prevented | Selection bias | Observation bias |
Time in the trial | Done when the patient enters the trial (during recruitment) | Occurs after the patient has entered the trial (after recruitment) |
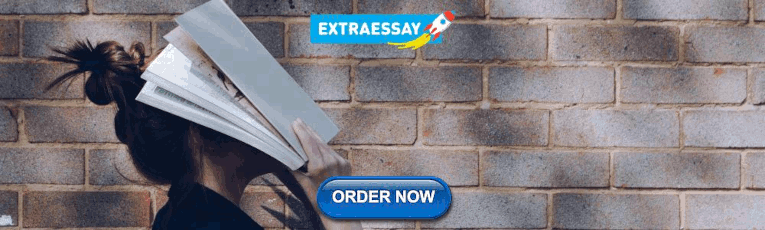
IMAGES
VIDEO
COMMENTS
Random allocation is a technique that chooses individuals for treatment groups and control groups entirely by chance with no regard to the will of researchers or patients' condition and preference. This allows researchers to control all known and unknown factors that may affect results in treatment groups and control groups.
The key phrase in an RCT is "random allocation" and it must be done properly, using two steps: generating the random sequence, implementing the sequence in a way that it is concealed. One should consider using a random numbers table or computer program to generate the random allocation sequence.
Randomization is the foundation of any RCT involving treatment comparison. Randomization is not a single technique, but a very broad class of statistical methodologies for design and analysis of clinical trials . In this paper, we focused on the randomized controlled two-arm trial designed with equal allocation, which is the gold standard ...
Random Assignment in Experiments | Introduction & Examples. Published on March 8, 2021 by Pritha Bhandari.Revised on June 22, 2023. In experimental research, random assignment is a way of placing participants from your sample into different treatment groups using randomization. With simple random assignment, every member of the sample has a known or equal chance of being placed in a control ...
Abstract. A randomized controlled trial is a prospective, comparative, quantitative study/experiment performed under controlled conditions with random allocation of interventions to comparison groups. The randomized controlled trial is the most rigorous and robust research method of determining whether a cause-effect relation exists between an ...
An example of randomization when the block size is four. Fig. 3. Block randomization when the block size is two and four. Total eight blocks in the red-dotted line are assigned at random. The left column is for allocation and the right column is for the total sample size. Fig. 4. www.randomization.com can do block randomization more easily.
This review provides an update on various random allocation techniques so as to correct existing misconceptions on this all important procedure. Methods: This is a review of literatures published in the Pubmed database on concepts of common allocation techniques used in controlled clinical trials. Results: Allocation methods that use; case ...
In addition, block randomization is done by randomly choosing a treatment allocation order for a certain block of participants, that is, if the size of each block is four and there are two treatment groups A and B, there are multiple allocation orders possible (AABB, BBAA, ABAB, BABA, ABBA, and BAAB). 3 The randomization step randomizes the ...
Randomization is widely applied in various fields, especially in scientific research, statistical analysis, and resource allocation, to ensure fairness and validity in the outcomes. [8] [9] [10] In various contexts, randomization may involve Generating Random Permutations: This is essential in various situations, such as shuffling cards. By ...
Randomized Controlled Trials (RCTs) are considered the "gold standard" in medical and health research due to their rigorous design. Random allocation in a Randomized Control Trial (RCT) is the process of assigning participants to either the experimental group or the control group purely by chance.
Good methods of generating a random allocation sequence include using a random-numbers table or a computer software program that generates the random sequence. There are manual methods of achieving random allocation such as tossing a coin, drawing lots or throwing dice. However, these manual methods in practice often be-come nonrandom, are ...
The purpose of this tutorial is to describe a step-by-step process for the preparation of SNOSE. We will outline how to prepare SNOSE to preserve allocation concealment in a trial that (a) uses unrestricted (simple) randomization, (b) stratifies randomization on one factor, (c) uses permuted blocks and, and (d) is conducted at more than 1 study ...
As for simple randomization, the probability is first determined according to the ratio between the groups, and then the subjects are allocated. If the ratio between group A and group B is 2 : 1, the probability of group A is 2/3 and that of group B is 1/3. Block randomization often uses a jar model with a random allocation rule.
Olivia Guy-Evans, MSc. In psychology, random assignment refers to the practice of allocating participants to different experimental groups in a study in a completely unbiased way, ensuring each participant has an equal chance of being assigned to any group. In experimental research, random assignment, or random placement, organizes participants ...
Random allocation is a technique that chooses individuals for treatment groups and control groups entirely by chance with no regard to the will of researchers or patients' condition and preference. This allows researchers to control all known and unknown factors that may affect results in treatment groups and control groups.
Random allocation is another paramount method used to assign participants to different research groups (Peat, 2011). In other words, randomization is a practice that's used to achieve generalizability, while random allocation - is to minimize confounders and eliminate systematic bias.
What are the benefits of random allocation in clinical studies? John Worrall, a philosopher of science, recently questioned whether evidence-based medicine's advice to base therapeutic decisions on the results of randomised controlled trials (RCTs) could be justified.1 2 Here we provide a response to Worrall and others who challenge the epistemological value of RCTs. Worrall's primary target ...
7.3 Random allocation vs random sampling. Random sampling and random allocation are two different concepts (Fig. 7.4), that serve two different purposes, but are often confused:. Random sampling allows results to be generalised to a larger population, and impacts external validity. It concerns how the sample is found to study.; Random allocation tries to eliminate confounding issues, by ...
Random Allocation Software enables users to control different attributes of the random allocation sequence and produce qualified lists for parallel group trials. ... by the coordinator of the research team to prepare and encode different drugs or instruments necessary for the parallel group trial. Random Allocation Software enables users to ...
This should be done by random allocation, ensuring that each participant has an equal chance of being assigned to one group. Independent measures involve using two separate groups of participants, one in each condition. For example: Con: More people are needed than with the repeated measures design (i.e., more time-consuming).
Research Randomizer. Research Randomizer is also a closed-source, free, randomization web service. It can be used for random sampling, random assignment to treatment groups, and block randomization. ... The Random Allocation Software is also available as a free open source web service.[10,11] Both have different settings for simple and blocked ...
RANDOM SAMPLING AND. RANDOM ASSIGNMENT MADE EASY! Research Randomizer is a free resource for researchers and students in need of a quick way to generate random numbers or assign participants to experimental conditions. This site can be used for a variety of purposes, including psychology experiments, medical trials, and survey research.
Dams significantly impact the environment, industries, residential areas, and agriculture. Efficient dam management can mitigate negative impacts and enhance benefits such as flood and drought reduction, energy efficiency, water access, and improved irrigation. This study tackles the critical issue of predicting dam occupancy levels precisely to contribute to sustainable water management by ...
Randomization is another step to minimize variability or "confounders.". By randomization, research participants have an equal chance of being selected into any treatment group of the study, generating comparable intervention groups, thereby distributing the confounders. A trial can be "open labeled" or "blinded.".