Have a language expert improve your writing
Run a free plagiarism check in 10 minutes, generate accurate citations for free.
- Knowledge Base
Methodology
- Systematic Review | Definition, Example, & Guide
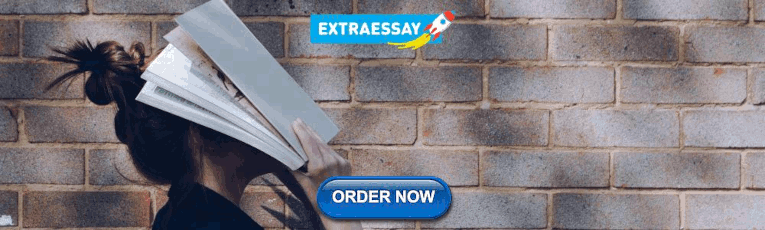
Systematic Review | Definition, Example & Guide
Published on June 15, 2022 by Shaun Turney . Revised on November 20, 2023.
A systematic review is a type of review that uses repeatable methods to find, select, and synthesize all available evidence. It answers a clearly formulated research question and explicitly states the methods used to arrive at the answer.
They answered the question “What is the effectiveness of probiotics in reducing eczema symptoms and improving quality of life in patients with eczema?”
In this context, a probiotic is a health product that contains live microorganisms and is taken by mouth. Eczema is a common skin condition that causes red, itchy skin.
Table of contents
What is a systematic review, systematic review vs. meta-analysis, systematic review vs. literature review, systematic review vs. scoping review, when to conduct a systematic review, pros and cons of systematic reviews, step-by-step example of a systematic review, other interesting articles, frequently asked questions about systematic reviews.
A review is an overview of the research that’s already been completed on a topic.
What makes a systematic review different from other types of reviews is that the research methods are designed to reduce bias . The methods are repeatable, and the approach is formal and systematic:
- Formulate a research question
- Develop a protocol
- Search for all relevant studies
- Apply the selection criteria
- Extract the data
- Synthesize the data
- Write and publish a report
Although multiple sets of guidelines exist, the Cochrane Handbook for Systematic Reviews is among the most widely used. It provides detailed guidelines on how to complete each step of the systematic review process.
Systematic reviews are most commonly used in medical and public health research, but they can also be found in other disciplines.
Systematic reviews typically answer their research question by synthesizing all available evidence and evaluating the quality of the evidence. Synthesizing means bringing together different information to tell a single, cohesive story. The synthesis can be narrative ( qualitative ), quantitative , or both.
Receive feedback on language, structure, and formatting
Professional editors proofread and edit your paper by focusing on:
- Academic style
- Vague sentences
- Style consistency
See an example
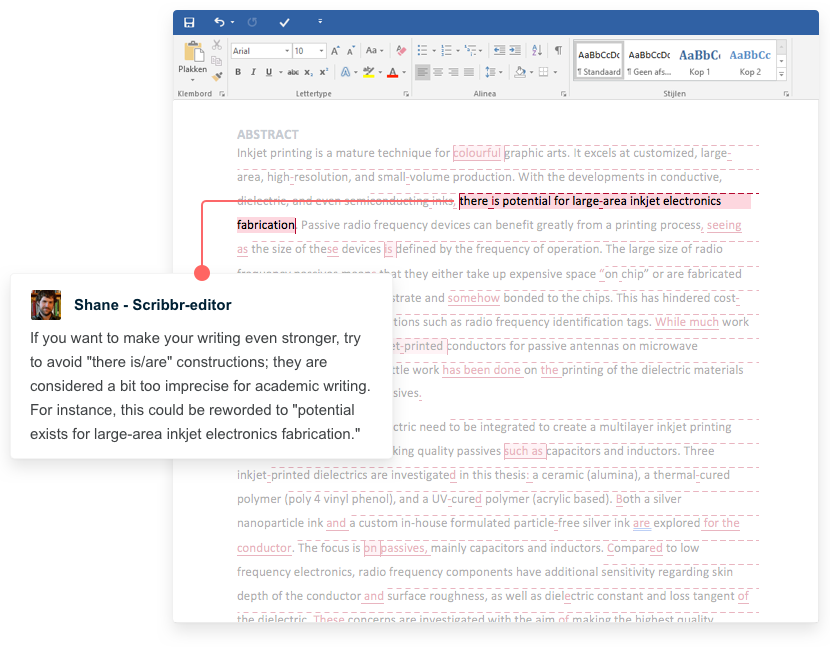
Systematic reviews often quantitatively synthesize the evidence using a meta-analysis . A meta-analysis is a statistical analysis, not a type of review.
A meta-analysis is a technique to synthesize results from multiple studies. It’s a statistical analysis that combines the results of two or more studies, usually to estimate an effect size .
A literature review is a type of review that uses a less systematic and formal approach than a systematic review. Typically, an expert in a topic will qualitatively summarize and evaluate previous work, without using a formal, explicit method.
Although literature reviews are often less time-consuming and can be insightful or helpful, they have a higher risk of bias and are less transparent than systematic reviews.
Similar to a systematic review, a scoping review is a type of review that tries to minimize bias by using transparent and repeatable methods.
However, a scoping review isn’t a type of systematic review. The most important difference is the goal: rather than answering a specific question, a scoping review explores a topic. The researcher tries to identify the main concepts, theories, and evidence, as well as gaps in the current research.
Sometimes scoping reviews are an exploratory preparation step for a systematic review, and sometimes they are a standalone project.
Prevent plagiarism. Run a free check.
A systematic review is a good choice of review if you want to answer a question about the effectiveness of an intervention , such as a medical treatment.
To conduct a systematic review, you’ll need the following:
- A precise question , usually about the effectiveness of an intervention. The question needs to be about a topic that’s previously been studied by multiple researchers. If there’s no previous research, there’s nothing to review.
- If you’re doing a systematic review on your own (e.g., for a research paper or thesis ), you should take appropriate measures to ensure the validity and reliability of your research.
- Access to databases and journal archives. Often, your educational institution provides you with access.
- Time. A professional systematic review is a time-consuming process: it will take the lead author about six months of full-time work. If you’re a student, you should narrow the scope of your systematic review and stick to a tight schedule.
- Bibliographic, word-processing, spreadsheet, and statistical software . For example, you could use EndNote, Microsoft Word, Excel, and SPSS.
A systematic review has many pros .
- They minimize research bias by considering all available evidence and evaluating each study for bias.
- Their methods are transparent , so they can be scrutinized by others.
- They’re thorough : they summarize all available evidence.
- They can be replicated and updated by others.
Systematic reviews also have a few cons .
- They’re time-consuming .
- They’re narrow in scope : they only answer the precise research question.
The 7 steps for conducting a systematic review are explained with an example.
Step 1: Formulate a research question
Formulating the research question is probably the most important step of a systematic review. A clear research question will:
- Allow you to more effectively communicate your research to other researchers and practitioners
- Guide your decisions as you plan and conduct your systematic review
A good research question for a systematic review has four components, which you can remember with the acronym PICO :
- Population(s) or problem(s)
- Intervention(s)
- Comparison(s)
You can rearrange these four components to write your research question:
- What is the effectiveness of I versus C for O in P ?
Sometimes, you may want to include a fifth component, the type of study design . In this case, the acronym is PICOT .
- Type of study design(s)
- The population of patients with eczema
- The intervention of probiotics
- In comparison to no treatment, placebo , or non-probiotic treatment
- The outcome of changes in participant-, parent-, and doctor-rated symptoms of eczema and quality of life
- Randomized control trials, a type of study design
Their research question was:
- What is the effectiveness of probiotics versus no treatment, a placebo, or a non-probiotic treatment for reducing eczema symptoms and improving quality of life in patients with eczema?
Step 2: Develop a protocol
A protocol is a document that contains your research plan for the systematic review. This is an important step because having a plan allows you to work more efficiently and reduces bias.
Your protocol should include the following components:
- Background information : Provide the context of the research question, including why it’s important.
- Research objective (s) : Rephrase your research question as an objective.
- Selection criteria: State how you’ll decide which studies to include or exclude from your review.
- Search strategy: Discuss your plan for finding studies.
- Analysis: Explain what information you’ll collect from the studies and how you’ll synthesize the data.
If you’re a professional seeking to publish your review, it’s a good idea to bring together an advisory committee . This is a group of about six people who have experience in the topic you’re researching. They can help you make decisions about your protocol.
It’s highly recommended to register your protocol. Registering your protocol means submitting it to a database such as PROSPERO or ClinicalTrials.gov .
Step 3: Search for all relevant studies
Searching for relevant studies is the most time-consuming step of a systematic review.
To reduce bias, it’s important to search for relevant studies very thoroughly. Your strategy will depend on your field and your research question, but sources generally fall into these four categories:
- Databases: Search multiple databases of peer-reviewed literature, such as PubMed or Scopus . Think carefully about how to phrase your search terms and include multiple synonyms of each word. Use Boolean operators if relevant.
- Handsearching: In addition to searching the primary sources using databases, you’ll also need to search manually. One strategy is to scan relevant journals or conference proceedings. Another strategy is to scan the reference lists of relevant studies.
- Gray literature: Gray literature includes documents produced by governments, universities, and other institutions that aren’t published by traditional publishers. Graduate student theses are an important type of gray literature, which you can search using the Networked Digital Library of Theses and Dissertations (NDLTD) . In medicine, clinical trial registries are another important type of gray literature.
- Experts: Contact experts in the field to ask if they have unpublished studies that should be included in your review.
At this stage of your review, you won’t read the articles yet. Simply save any potentially relevant citations using bibliographic software, such as Scribbr’s APA or MLA Generator .
- Databases: EMBASE, PsycINFO, AMED, LILACS, and ISI Web of Science
- Handsearch: Conference proceedings and reference lists of articles
- Gray literature: The Cochrane Library, the metaRegister of Controlled Trials, and the Ongoing Skin Trials Register
- Experts: Authors of unpublished registered trials, pharmaceutical companies, and manufacturers of probiotics
Step 4: Apply the selection criteria
Applying the selection criteria is a three-person job. Two of you will independently read the studies and decide which to include in your review based on the selection criteria you established in your protocol . The third person’s job is to break any ties.
To increase inter-rater reliability , ensure that everyone thoroughly understands the selection criteria before you begin.
If you’re writing a systematic review as a student for an assignment, you might not have a team. In this case, you’ll have to apply the selection criteria on your own; you can mention this as a limitation in your paper’s discussion.
You should apply the selection criteria in two phases:
- Based on the titles and abstracts : Decide whether each article potentially meets the selection criteria based on the information provided in the abstracts.
- Based on the full texts: Download the articles that weren’t excluded during the first phase. If an article isn’t available online or through your library, you may need to contact the authors to ask for a copy. Read the articles and decide which articles meet the selection criteria.
It’s very important to keep a meticulous record of why you included or excluded each article. When the selection process is complete, you can summarize what you did using a PRISMA flow diagram .
Next, Boyle and colleagues found the full texts for each of the remaining studies. Boyle and Tang read through the articles to decide if any more studies needed to be excluded based on the selection criteria.
When Boyle and Tang disagreed about whether a study should be excluded, they discussed it with Varigos until the three researchers came to an agreement.
Step 5: Extract the data
Extracting the data means collecting information from the selected studies in a systematic way. There are two types of information you need to collect from each study:
- Information about the study’s methods and results . The exact information will depend on your research question, but it might include the year, study design , sample size, context, research findings , and conclusions. If any data are missing, you’ll need to contact the study’s authors.
- Your judgment of the quality of the evidence, including risk of bias .
You should collect this information using forms. You can find sample forms in The Registry of Methods and Tools for Evidence-Informed Decision Making and the Grading of Recommendations, Assessment, Development and Evaluations Working Group .
Extracting the data is also a three-person job. Two people should do this step independently, and the third person will resolve any disagreements.
They also collected data about possible sources of bias, such as how the study participants were randomized into the control and treatment groups.
Step 6: Synthesize the data
Synthesizing the data means bringing together the information you collected into a single, cohesive story. There are two main approaches to synthesizing the data:
- Narrative ( qualitative ): Summarize the information in words. You’ll need to discuss the studies and assess their overall quality.
- Quantitative : Use statistical methods to summarize and compare data from different studies. The most common quantitative approach is a meta-analysis , which allows you to combine results from multiple studies into a summary result.
Generally, you should use both approaches together whenever possible. If you don’t have enough data, or the data from different studies aren’t comparable, then you can take just a narrative approach. However, you should justify why a quantitative approach wasn’t possible.
Boyle and colleagues also divided the studies into subgroups, such as studies about babies, children, and adults, and analyzed the effect sizes within each group.
Step 7: Write and publish a report
The purpose of writing a systematic review article is to share the answer to your research question and explain how you arrived at this answer.
Your article should include the following sections:
- Abstract : A summary of the review
- Introduction : Including the rationale and objectives
- Methods : Including the selection criteria, search method, data extraction method, and synthesis method
- Results : Including results of the search and selection process, study characteristics, risk of bias in the studies, and synthesis results
- Discussion : Including interpretation of the results and limitations of the review
- Conclusion : The answer to your research question and implications for practice, policy, or research
To verify that your report includes everything it needs, you can use the PRISMA checklist .
Once your report is written, you can publish it in a systematic review database, such as the Cochrane Database of Systematic Reviews , and/or in a peer-reviewed journal.
In their report, Boyle and colleagues concluded that probiotics cannot be recommended for reducing eczema symptoms or improving quality of life in patients with eczema. Note Generative AI tools like ChatGPT can be useful at various stages of the writing and research process and can help you to write your systematic review. However, we strongly advise against trying to pass AI-generated text off as your own work.
If you want to know more about statistics , methodology , or research bias , make sure to check out some of our other articles with explanations and examples.
- Student’s t -distribution
- Normal distribution
- Null and Alternative Hypotheses
- Chi square tests
- Confidence interval
- Quartiles & Quantiles
- Cluster sampling
- Stratified sampling
- Data cleansing
- Reproducibility vs Replicability
- Peer review
- Prospective cohort study
Research bias
- Implicit bias
- Cognitive bias
- Placebo effect
- Hawthorne effect
- Hindsight bias
- Affect heuristic
- Social desirability bias
A literature review is a survey of scholarly sources (such as books, journal articles, and theses) related to a specific topic or research question .
It is often written as part of a thesis, dissertation , or research paper , in order to situate your work in relation to existing knowledge.
A literature review is a survey of credible sources on a topic, often used in dissertations , theses, and research papers . Literature reviews give an overview of knowledge on a subject, helping you identify relevant theories and methods, as well as gaps in existing research. Literature reviews are set up similarly to other academic texts , with an introduction , a main body, and a conclusion .
An annotated bibliography is a list of source references that has a short description (called an annotation ) for each of the sources. It is often assigned as part of the research process for a paper .
A systematic review is secondary research because it uses existing research. You don’t collect new data yourself.
Cite this Scribbr article
If you want to cite this source, you can copy and paste the citation or click the “Cite this Scribbr article” button to automatically add the citation to our free Citation Generator.
Turney, S. (2023, November 20). Systematic Review | Definition, Example & Guide. Scribbr. Retrieved September 3, 2024, from https://www.scribbr.com/methodology/systematic-review/
Is this article helpful?
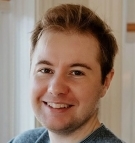
Shaun Turney
Other students also liked, how to write a literature review | guide, examples, & templates, how to write a research proposal | examples & templates, what is critical thinking | definition & examples, "i thought ai proofreading was useless but..".
I've been using Scribbr for years now and I know it's a service that won't disappoint. It does a good job spotting mistakes”
- A-Z Publications
Annual Review of Psychology
Volume 70, 2019, review article, how to do a systematic review: a best practice guide for conducting and reporting narrative reviews, meta-analyses, and meta-syntheses.
- Andy P. Siddaway 1 , Alex M. Wood 2 , and Larry V. Hedges 3
- View Affiliations Hide Affiliations Affiliations: 1 Behavioural Science Centre, Stirling Management School, University of Stirling, Stirling FK9 4LA, United Kingdom; email: [email protected] 2 Department of Psychological and Behavioural Science, London School of Economics and Political Science, London WC2A 2AE, United Kingdom 3 Department of Statistics, Northwestern University, Evanston, Illinois 60208, USA; email: [email protected]
- Vol. 70:747-770 (Volume publication date January 2019) https://doi.org/10.1146/annurev-psych-010418-102803
- First published as a Review in Advance on August 08, 2018
- Copyright © 2019 by Annual Reviews. All rights reserved
Systematic reviews are characterized by a methodical and replicable methodology and presentation. They involve a comprehensive search to locate all relevant published and unpublished work on a subject; a systematic integration of search results; and a critique of the extent, nature, and quality of evidence in relation to a particular research question. The best reviews synthesize studies to draw broad theoretical conclusions about what a literature means, linking theory to evidence and evidence to theory. This guide describes how to plan, conduct, organize, and present a systematic review of quantitative (meta-analysis) or qualitative (narrative review, meta-synthesis) information. We outline core standards and principles and describe commonly encountered problems. Although this guide targets psychological scientists, its high level of abstraction makes it potentially relevant to any subject area or discipline. We argue that systematic reviews are a key methodology for clarifying whether and how research findings replicate and for explaining possible inconsistencies, and we call for researchers to conduct systematic reviews to help elucidate whether there is a replication crisis.
Article metrics loading...
Full text loading...
Literature Cited
- APA Publ. Commun. Board Work. Group J. Artic. Rep. Stand. 2008 . Reporting standards for research in psychology: Why do we need them? What might they be?. Am. Psychol . 63 : 848– 49 [Google Scholar]
- Baumeister RF 2013 . Writing a literature review. The Portable Mentor: Expert Guide to a Successful Career in Psychology MJ Prinstein, MD Patterson 119– 32 New York: Springer, 2nd ed.. [Google Scholar]
- Baumeister RF , Leary MR 1995 . The need to belong: desire for interpersonal attachments as a fundamental human motivation. Psychol. Bull. 117 : 497– 529 [Google Scholar]
- Baumeister RF , Leary MR 1997 . Writing narrative literature reviews. Rev. Gen. Psychol. 3 : 311– 20 Presents a thorough and thoughtful guide to conducting narrative reviews. [Google Scholar]
- Bem DJ 1995 . Writing a review article for Psychological Bulletin. Psychol . Bull 118 : 172– 77 [Google Scholar]
- Borenstein M , Hedges LV , Higgins JPT , Rothstein HR 2009 . Introduction to Meta-Analysis New York: Wiley Presents a comprehensive introduction to meta-analysis. [Google Scholar]
- Borenstein M , Higgins JPT , Hedges LV , Rothstein HR 2017 . Basics of meta-analysis: I 2 is not an absolute measure of heterogeneity. Res. Synth. Methods 8 : 5– 18 [Google Scholar]
- Braver SL , Thoemmes FJ , Rosenthal R 2014 . Continuously cumulating meta-analysis and replicability. Perspect. Psychol. Sci. 9 : 333– 42 [Google Scholar]
- Bushman BJ 1994 . Vote-counting procedures. The Handbook of Research Synthesis H Cooper, LV Hedges 193– 214 New York: Russell Sage Found. [Google Scholar]
- Cesario J 2014 . Priming, replication, and the hardest science. Perspect. Psychol. Sci. 9 : 40– 48 [Google Scholar]
- Chalmers I 2007 . The lethal consequences of failing to make use of all relevant evidence about the effects of medical treatments: the importance of systematic reviews. Treating Individuals: From Randomised Trials to Personalised Medicine PM Rothwell 37– 58 London: Lancet [Google Scholar]
- Cochrane Collab. 2003 . Glossary Rep., Cochrane Collab. London: http://community.cochrane.org/glossary Presents a comprehensive glossary of terms relevant to systematic reviews. [Google Scholar]
- Cohn LD , Becker BJ 2003 . How meta-analysis increases statistical power. Psychol. Methods 8 : 243– 53 [Google Scholar]
- Cooper HM 2003 . Editorial. Psychol. Bull. 129 : 3– 9 [Google Scholar]
- Cooper HM 2016 . Research Synthesis and Meta-Analysis: A Step-by-Step Approach Thousand Oaks, CA: Sage, 5th ed.. Presents a comprehensive introduction to research synthesis and meta-analysis. [Google Scholar]
- Cooper HM , Hedges LV , Valentine JC 2009 . The Handbook of Research Synthesis and Meta-Analysis New York: Russell Sage Found, 2nd ed.. [Google Scholar]
- Cumming G 2014 . The new statistics: why and how. Psychol. Sci. 25 : 7– 29 Discusses the limitations of null hypothesis significance testing and viable alternative approaches. [Google Scholar]
- Earp BD , Trafimow D 2015 . Replication, falsification, and the crisis of confidence in social psychology. Front. Psychol. 6 : 621 [Google Scholar]
- Etz A , Vandekerckhove J 2016 . A Bayesian perspective on the reproducibility project: psychology. PLOS ONE 11 : e0149794 [Google Scholar]
- Ferguson CJ , Brannick MT 2012 . Publication bias in psychological science: prevalence, methods for identifying and controlling, and implications for the use of meta-analyses. Psychol. Methods 17 : 120– 28 [Google Scholar]
- Fleiss JL , Berlin JA 2009 . Effect sizes for dichotomous data. The Handbook of Research Synthesis and Meta-Analysis H Cooper, LV Hedges, JC Valentine 237– 53 New York: Russell Sage Found, 2nd ed.. [Google Scholar]
- Garside R 2014 . Should we appraise the quality of qualitative research reports for systematic reviews, and if so, how. Innovation 27 : 67– 79 [Google Scholar]
- Hedges LV , Olkin I 1980 . Vote count methods in research synthesis. Psychol. Bull. 88 : 359– 69 [Google Scholar]
- Hedges LV , Pigott TD 2001 . The power of statistical tests in meta-analysis. Psychol. Methods 6 : 203– 17 [Google Scholar]
- Higgins JPT , Green S 2011 . Cochrane Handbook for Systematic Reviews of Interventions, Version 5.1.0 London: Cochrane Collab. Presents comprehensive and regularly updated guidelines on systematic reviews. [Google Scholar]
- John LK , Loewenstein G , Prelec D 2012 . Measuring the prevalence of questionable research practices with incentives for truth telling. Psychol. Sci. 23 : 524– 32 [Google Scholar]
- Juni P , Witschi A , Bloch R , Egger M 1999 . The hazards of scoring the quality of clinical trials for meta-analysis. JAMA 282 : 1054– 60 [Google Scholar]
- Klein O , Doyen S , Leys C , Magalhães de Saldanha da Gama PA , Miller S et al. 2012 . Low hopes, high expectations: expectancy effects and the replicability of behavioral experiments. Perspect. Psychol. Sci. 7 : 6 572– 84 [Google Scholar]
- Lau J , Antman EM , Jimenez-Silva J , Kupelnick B , Mosteller F , Chalmers TC 1992 . Cumulative meta-analysis of therapeutic trials for myocardial infarction. N. Engl. J. Med. 327 : 248– 54 [Google Scholar]
- Light RJ , Smith PV 1971 . Accumulating evidence: procedures for resolving contradictions among different research studies. Harvard Educ. Rev. 41 : 429– 71 [Google Scholar]
- Lipsey MW , Wilson D 2001 . Practical Meta-Analysis London: Sage Comprehensive and clear explanation of meta-analysis. [Google Scholar]
- Matt GE , Cook TD 1994 . Threats to the validity of research synthesis. The Handbook of Research Synthesis H Cooper, LV Hedges 503– 20 New York: Russell Sage Found. [Google Scholar]
- Maxwell SE , Lau MY , Howard GS 2015 . Is psychology suffering from a replication crisis? What does “failure to replicate” really mean?. Am. Psychol. 70 : 487– 98 [Google Scholar]
- Moher D , Hopewell S , Schulz KF , Montori V , Gøtzsche PC et al. 2010 . CONSORT explanation and elaboration: updated guidelines for reporting parallel group randomised trials. BMJ 340 : c869 [Google Scholar]
- Moher D , Liberati A , Tetzlaff J , Altman DG PRISMA Group. 2009 . Preferred reporting items for systematic reviews and meta-analyses: the PRISMA statement. BMJ 339 : 332– 36 Comprehensive reporting guidelines for systematic reviews. [Google Scholar]
- Morrison A , Polisena J , Husereau D , Moulton K , Clark M et al. 2012 . The effect of English-language restriction on systematic review-based meta-analyses: a systematic review of empirical studies. Int. J. Technol. Assess. Health Care 28 : 138– 44 [Google Scholar]
- Nelson LD , Simmons J , Simonsohn U 2018 . Psychology's renaissance. Annu. Rev. Psychol. 69 : 511– 34 [Google Scholar]
- Noblit GW , Hare RD 1988 . Meta-Ethnography: Synthesizing Qualitative Studies Newbury Park, CA: Sage [Google Scholar]
- Olivo SA , Macedo LG , Gadotti IC , Fuentes J , Stanton T , Magee DJ 2008 . Scales to assess the quality of randomized controlled trials: a systematic review. Phys. Ther. 88 : 156– 75 [Google Scholar]
- Open Sci. Collab. 2015 . Estimating the reproducibility of psychological science. Science 349 : 943 [Google Scholar]
- Paterson BL , Thorne SE , Canam C , Jillings C 2001 . Meta-Study of Qualitative Health Research: A Practical Guide to Meta-Analysis and Meta-Synthesis Thousand Oaks, CA: Sage [Google Scholar]
- Patil P , Peng RD , Leek JT 2016 . What should researchers expect when they replicate studies? A statistical view of replicability in psychological science. Perspect. Psychol. Sci. 11 : 539– 44 [Google Scholar]
- Rosenthal R 1979 . The “file drawer problem” and tolerance for null results. Psychol. Bull. 86 : 638– 41 [Google Scholar]
- Rosnow RL , Rosenthal R 1989 . Statistical procedures and the justification of knowledge in psychological science. Am. Psychol. 44 : 1276– 84 [Google Scholar]
- Sanderson S , Tatt ID , Higgins JP 2007 . Tools for assessing quality and susceptibility to bias in observational studies in epidemiology: a systematic review and annotated bibliography. Int. J. Epidemiol. 36 : 666– 76 [Google Scholar]
- Schreiber R , Crooks D , Stern PN 1997 . Qualitative meta-analysis. Completing a Qualitative Project: Details and Dialogue JM Morse 311– 26 Thousand Oaks, CA: Sage [Google Scholar]
- Shrout PE , Rodgers JL 2018 . Psychology, science, and knowledge construction: broadening perspectives from the replication crisis. Annu. Rev. Psychol. 69 : 487– 510 [Google Scholar]
- Stroebe W , Strack F 2014 . The alleged crisis and the illusion of exact replication. Perspect. Psychol. Sci. 9 : 59– 71 [Google Scholar]
- Stroup DF , Berlin JA , Morton SC , Olkin I , Williamson GD et al. 2000 . Meta-analysis of observational studies in epidemiology (MOOSE): a proposal for reporting. JAMA 283 : 2008– 12 [Google Scholar]
- Thorne S , Jensen L , Kearney MH , Noblit G , Sandelowski M 2004 . Qualitative meta-synthesis: reflections on methodological orientation and ideological agenda. Qual. Health Res. 14 : 1342– 65 [Google Scholar]
- Tong A , Flemming K , McInnes E , Oliver S , Craig J 2012 . Enhancing transparency in reporting the synthesis of qualitative research: ENTREQ. BMC Med. Res. Methodol. 12 : 181– 88 [Google Scholar]
- Trickey D , Siddaway AP , Meiser-Stedman R , Serpell L , Field AP 2012 . A meta-analysis of risk factors for post-traumatic stress disorder in children and adolescents. Clin. Psychol. Rev. 32 : 122– 38 [Google Scholar]
- Valentine JC , Biglan A , Boruch RF , Castro FG , Collins LM et al. 2011 . Replication in prevention science. Prev. Sci. 12 : 103– 17 [Google Scholar]
- Article Type: Review Article
Most Read This Month
Most cited most cited rss feed, job burnout, executive functions, social cognitive theory: an agentic perspective, on happiness and human potentials: a review of research on hedonic and eudaimonic well-being, sources of method bias in social science research and recommendations on how to control it, mediation analysis, missing data analysis: making it work in the real world, grounded cognition, personality structure: emergence of the five-factor model, motivational beliefs, values, and goals.
Have a language expert improve your writing
Run a free plagiarism check in 10 minutes, automatically generate references for free.
- Knowledge Base
- Methodology
- Systematic Review | Definition, Examples & Guide
Systematic Review | Definition, Examples & Guide
Published on 15 June 2022 by Shaun Turney . Revised on 18 July 2024.
A systematic review is a type of review that uses repeatable methods to find, select, and synthesise all available evidence. It answers a clearly formulated research question and explicitly states the methods used to arrive at the answer.
They answered the question ‘What is the effectiveness of probiotics in reducing eczema symptoms and improving quality of life in patients with eczema?’
In this context, a probiotic is a health product that contains live microorganisms and is taken by mouth. Eczema is a common skin condition that causes red, itchy skin.
Table of contents
What is a systematic review, systematic review vs meta-analysis, systematic review vs literature review, systematic review vs scoping review, when to conduct a systematic review, pros and cons of systematic reviews, step-by-step example of a systematic review, frequently asked questions about systematic reviews.
A review is an overview of the research that’s already been completed on a topic.
What makes a systematic review different from other types of reviews is that the research methods are designed to reduce research bias . The methods are repeatable , and the approach is formal and systematic:
- Formulate a research question
- Develop a protocol
- Search for all relevant studies
- Apply the selection criteria
- Extract the data
- Synthesise the data
- Write and publish a report
Although multiple sets of guidelines exist, the Cochrane Handbook for Systematic Reviews is among the most widely used. It provides detailed guidelines on how to complete each step of the systematic review process.
Systematic reviews are most commonly used in medical and public health research, but they can also be found in other disciplines.
Systematic reviews typically answer their research question by synthesising all available evidence and evaluating the quality of the evidence. Synthesising means bringing together different information to tell a single, cohesive story. The synthesis can be narrative ( qualitative ), quantitative , or both.
Prevent plagiarism, run a free check.
Systematic reviews often quantitatively synthesise the evidence using a meta-analysis . A meta-analysis is a statistical analysis, not a type of review.
A meta-analysis is a technique to synthesise results from multiple studies. It’s a statistical analysis that combines the results of two or more studies, usually to estimate an effect size .
A literature review is a type of review that uses a less systematic and formal approach than a systematic review. Typically, an expert in a topic will qualitatively summarise and evaluate previous work, without using a formal, explicit method.
Although literature reviews are often less time-consuming and can be insightful or helpful, they have a higher risk of bias and are less transparent than systematic reviews.
Similar to a systematic review, a scoping review is a type of review that tries to minimise bias by using transparent and repeatable methods.
However, a scoping review isn’t a type of systematic review. The most important difference is the goal: rather than answering a specific question, a scoping review explores a topic. The researcher tries to identify the main concepts, theories, and evidence, as well as gaps in the current research.
Sometimes scoping reviews are an exploratory preparation step for a systematic review, and sometimes they are a standalone project.
A systematic review is a good choice of review if you want to answer a question about the effectiveness of an intervention , such as a medical treatment.
To conduct a systematic review, you’ll need the following:
- A precise question , usually about the effectiveness of an intervention. The question needs to be about a topic that’s previously been studied by multiple researchers. If there’s no previous research, there’s nothing to review.
- If you’re doing a systematic review on your own (e.g., for a research paper or thesis), you should take appropriate measures to ensure the validity and reliability of your research.
- Access to databases and journal archives. Often, your educational institution provides you with access.
- Time. A professional systematic review is a time-consuming process: it will take the lead author about six months of full-time work. If you’re a student, you should narrow the scope of your systematic review and stick to a tight schedule.
- Bibliographic, word-processing, spreadsheet, and statistical software . For example, you could use EndNote, Microsoft Word, Excel, and SPSS.
A systematic review has many pros .
- They minimise research b ias by considering all available evidence and evaluating each study for bias.
- Their methods are transparent , so they can be scrutinised by others.
- They’re thorough : they summarise all available evidence.
- They can be replicated and updated by others.
Systematic reviews also have a few cons .
- They’re time-consuming .
- They’re narrow in scope : they only answer the precise research question.
The 7 steps for conducting a systematic review are explained with an example.
Step 1: Formulate a research question
Formulating the research question is probably the most important step of a systematic review. A clear research question will:
- Allow you to more effectively communicate your research to other researchers and practitioners
- Guide your decisions as you plan and conduct your systematic review
A good research question for a systematic review has four components, which you can remember with the acronym PICO :
- Population(s) or problem(s)
- Intervention(s)
- Comparison(s)
You can rearrange these four components to write your research question:
- What is the effectiveness of I versus C for O in P ?
Sometimes, you may want to include a fourth component, the type of study design . In this case, the acronym is PICOT .
- Type of study design(s)
- The population of patients with eczema
- The intervention of probiotics
- In comparison to no treatment, placebo , or non-probiotic treatment
- The outcome of changes in participant-, parent-, and doctor-rated symptoms of eczema and quality of life
- Randomised control trials, a type of study design
Their research question was:
- What is the effectiveness of probiotics versus no treatment, a placebo, or a non-probiotic treatment for reducing eczema symptoms and improving quality of life in patients with eczema?
Step 2: Develop a protocol
A protocol is a document that contains your research plan for the systematic review. This is an important step because having a plan allows you to work more efficiently and reduces bias.
Your protocol should include the following components:
- Background information : Provide the context of the research question, including why it’s important.
- Research objective(s) : Rephrase your research question as an objective.
- Selection criteria: State how you’ll decide which studies to include or exclude from your review.
- Search strategy: Discuss your plan for finding studies.
- Analysis: Explain what information you’ll collect from the studies and how you’ll synthesise the data.
If you’re a professional seeking to publish your review, it’s a good idea to bring together an advisory committee . This is a group of about six people who have experience in the topic you’re researching. They can help you make decisions about your protocol.
It’s highly recommended to register your protocol. Registering your protocol means submitting it to a database such as PROSPERO or ClinicalTrials.gov .
Step 3: Search for all relevant studies
Searching for relevant studies is the most time-consuming step of a systematic review.
To reduce bias, it’s important to search for relevant studies very thoroughly. Your strategy will depend on your field and your research question, but sources generally fall into these four categories:
- Databases: Search multiple databases of peer-reviewed literature, such as PubMed or Scopus . Think carefully about how to phrase your search terms and include multiple synonyms of each word. Use Boolean operators if relevant.
- Handsearching: In addition to searching the primary sources using databases, you’ll also need to search manually. One strategy is to scan relevant journals or conference proceedings. Another strategy is to scan the reference lists of relevant studies.
- Grey literature: Grey literature includes documents produced by governments, universities, and other institutions that aren’t published by traditional publishers. Graduate student theses are an important type of grey literature, which you can search using the Networked Digital Library of Theses and Dissertations (NDLTD) . In medicine, clinical trial registries are another important type of grey literature.
- Experts: Contact experts in the field to ask if they have unpublished studies that should be included in your review.
At this stage of your review, you won’t read the articles yet. Simply save any potentially relevant citations using bibliographic software, such as Scribbr’s APA or MLA Generator .
- Databases: EMBASE, PsycINFO, AMED, LILACS, and ISI Web of Science
- Handsearch: Conference proceedings and reference lists of articles
- Grey literature: The Cochrane Library, the metaRegister of Controlled Trials, and the Ongoing Skin Trials Register
- Experts: Authors of unpublished registered trials, pharmaceutical companies, and manufacturers of probiotics
Step 4: Apply the selection criteria
Applying the selection criteria is a three-person job. Two of you will independently read the studies and decide which to include in your review based on the selection criteria you established in your protocol . The third person’s job is to break any ties.
To increase inter-rater reliability , ensure that everyone thoroughly understands the selection criteria before you begin.
If you’re writing a systematic review as a student for an assignment, you might not have a team. In this case, you’ll have to apply the selection criteria on your own; you can mention this as a limitation in your paper’s discussion.
You should apply the selection criteria in two phases:
- Based on the titles and abstracts : Decide whether each article potentially meets the selection criteria based on the information provided in the abstracts.
- Based on the full texts: Download the articles that weren’t excluded during the first phase. If an article isn’t available online or through your library, you may need to contact the authors to ask for a copy. Read the articles and decide which articles meet the selection criteria.
It’s very important to keep a meticulous record of why you included or excluded each article. When the selection process is complete, you can summarise what you did using a PRISMA flow diagram .
Next, Boyle and colleagues found the full texts for each of the remaining studies. Boyle and Tang read through the articles to decide if any more studies needed to be excluded based on the selection criteria.
When Boyle and Tang disagreed about whether a study should be excluded, they discussed it with Varigos until the three researchers came to an agreement.
Step 5: Extract the data
Extracting the data means collecting information from the selected studies in a systematic way. There are two types of information you need to collect from each study:
- Information about the study’s methods and results . The exact information will depend on your research question, but it might include the year, study design , sample size, context, research findings , and conclusions. If any data are missing, you’ll need to contact the study’s authors.
- Your judgement of the quality of the evidence, including risk of bias .
You should collect this information using forms. You can find sample forms in The Registry of Methods and Tools for Evidence-Informed Decision Making and the Grading of Recommendations, Assessment, Development and Evaluations Working Group .
Extracting the data is also a three-person job. Two people should do this step independently, and the third person will resolve any disagreements.
They also collected data about possible sources of bias, such as how the study participants were randomised into the control and treatment groups.
Step 6: Synthesise the data
Synthesising the data means bringing together the information you collected into a single, cohesive story. There are two main approaches to synthesising the data:
- Narrative ( qualitative ): Summarise the information in words. You’ll need to discuss the studies and assess their overall quality.
- Quantitative : Use statistical methods to summarise and compare data from different studies. The most common quantitative approach is a meta-analysis , which allows you to combine results from multiple studies into a summary result.
Generally, you should use both approaches together whenever possible. If you don’t have enough data, or the data from different studies aren’t comparable, then you can take just a narrative approach. However, you should justify why a quantitative approach wasn’t possible.
Boyle and colleagues also divided the studies into subgroups, such as studies about babies, children, and adults, and analysed the effect sizes within each group.
Step 7: Write and publish a report
The purpose of writing a systematic review article is to share the answer to your research question and explain how you arrived at this answer.
Your article should include the following sections:
- Abstract : A summary of the review
- Introduction : Including the rationale and objectives
- Methods : Including the selection criteria, search method, data extraction method, and synthesis method
- Results : Including results of the search and selection process, study characteristics, risk of bias in the studies, and synthesis results
- Discussion : Including interpretation of the results and limitations of the review
- Conclusion : The answer to your research question and implications for practice, policy, or research
To verify that your report includes everything it needs, you can use the PRISMA checklist .
Once your report is written, you can publish it in a systematic review database, such as the Cochrane Database of Systematic Reviews , and/or in a peer-reviewed journal.
A systematic review is secondary research because it uses existing research. You don’t collect new data yourself.
A literature review is a survey of scholarly sources (such as books, journal articles, and theses) related to a specific topic or research question .
It is often written as part of a dissertation , thesis, research paper , or proposal .
There are several reasons to conduct a literature review at the beginning of a research project:
- To familiarise yourself with the current state of knowledge on your topic
- To ensure that you’re not just repeating what others have already done
- To identify gaps in knowledge and unresolved problems that your research can address
- To develop your theoretical framework and methodology
- To provide an overview of the key findings and debates on the topic
Writing the literature review shows your reader how your work relates to existing research and what new insights it will contribute.
Cite this Scribbr article
If you want to cite this source, you can copy and paste the citation or click the ‘Cite this Scribbr article’ button to automatically add the citation to our free Reference Generator.
Turney, S. (2024, July 17). Systematic Review | Definition, Examples & Guide. Scribbr. Retrieved 3 September 2024, from https://www.scribbr.co.uk/research-methods/systematic-reviews/
Is this article helpful?
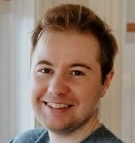
Shaun Turney
Other students also liked, what is a literature review | guide, template, & examples, exploratory research | definition, guide, & examples, what is peer review | types & examples.

An official website of the United States government
The .gov means it’s official. Federal government websites often end in .gov or .mil. Before sharing sensitive information, make sure you’re on a federal government site.
The site is secure. The https:// ensures that you are connecting to the official website and that any information you provide is encrypted and transmitted securely.
- Publications
- Account settings
- My Bibliography
- Collections
- Citation manager
Save citation to file
Email citation, add to collections.
- Create a new collection
- Add to an existing collection
Add to My Bibliography
Your saved search, create a file for external citation management software, your rss feed.
- Search in PubMed
- Search in NLM Catalog
- Add to Search
Easy guide to conducting a systematic review
Affiliations.
- 1 Discipline of Child and Adolescent Health, University of Sydney, Sydney, New South Wales, Australia.
- 2 Department of Nephrology, The Children's Hospital at Westmead, Sydney, New South Wales, Australia.
- 3 Education Department, The Children's Hospital at Westmead, Sydney, New South Wales, Australia.
- PMID: 32364273
- DOI: 10.1111/jpc.14853
A systematic review is a type of study that synthesises research that has been conducted on a particular topic. Systematic reviews are considered to provide the highest level of evidence on the hierarchy of evidence pyramid. Systematic reviews are conducted following rigorous research methodology. To minimise bias, systematic reviews utilise a predefined search strategy to identify and appraise all available published literature on a specific topic. The meticulous nature of the systematic review research methodology differentiates a systematic review from a narrative review (literature review or authoritative review). This paper provides a brief step by step summary of how to conduct a systematic review, which may be of interest for clinicians and researchers.
Keywords: research; research design; systematic review.
© 2020 Paediatrics and Child Health Division (The Royal Australasian College of Physicians).
PubMed Disclaimer
Similar articles
- Summarizing systematic reviews: methodological development, conduct and reporting of an umbrella review approach. Aromataris E, Fernandez R, Godfrey CM, Holly C, Khalil H, Tungpunkom P. Aromataris E, et al. Int J Evid Based Healthc. 2015 Sep;13(3):132-40. doi: 10.1097/XEB.0000000000000055. Int J Evid Based Healthc. 2015. PMID: 26360830
- Conducting systematic reviews of association (etiology): The Joanna Briggs Institute's approach. Moola S, Munn Z, Sears K, Sfetcu R, Currie M, Lisy K, Tufanaru C, Qureshi R, Mattis P, Mu P. Moola S, et al. Int J Evid Based Healthc. 2015 Sep;13(3):163-9. doi: 10.1097/XEB.0000000000000064. Int J Evid Based Healthc. 2015. PMID: 26262566
- Cervical spondylotic myelopathy: methodological approaches to evaluate the literature and establish best evidence. Skelly AC, Hashimoto RE, Norvell DC, Dettori JR, Fischer DJ, Wilson JR, Tetreault LA, Fehlings MG. Skelly AC, et al. Spine (Phila Pa 1976). 2013 Oct 15;38(22 Suppl 1):S9-18. doi: 10.1097/BRS.0b013e3182a7ebbf. Spine (Phila Pa 1976). 2013. PMID: 24026148
- What are the best methodologies for rapid reviews of the research evidence for evidence-informed decision making in health policy and practice: a rapid review. Haby MM, Chapman E, Clark R, Barreto J, Reveiz L, Lavis JN. Haby MM, et al. Health Res Policy Syst. 2016 Nov 25;14(1):83. doi: 10.1186/s12961-016-0155-7. Health Res Policy Syst. 2016. PMID: 27884208 Free PMC article. Review.
- What guidance is available for researchers conducting overviews of reviews of healthcare interventions? A scoping review and qualitative metasummary. Pollock M, Fernandes RM, Becker LA, Featherstone R, Hartling L. Pollock M, et al. Syst Rev. 2016 Nov 14;5(1):190. doi: 10.1186/s13643-016-0367-5. Syst Rev. 2016. PMID: 27842604 Free PMC article. Review.
- A Systematic Literature Review of Substance-Use Prevention Programs Amongst Refugee Youth. Aleer E, Alam K, Rashid A. Aleer E, et al. Community Ment Health J. 2024 Aug;60(6):1151-1170. doi: 10.1007/s10597-024-01267-6. Epub 2024 Apr 9. Community Ment Health J. 2024. PMID: 38592351 Free PMC article.
- The validity of electronic health data for measuring smoking status: a systematic review and meta-analysis. Haque MA, Gedara MLB, Nickel N, Turgeon M, Lix LM. Haque MA, et al. BMC Med Inform Decis Mak. 2024 Feb 2;24(1):33. doi: 10.1186/s12911-024-02416-3. BMC Med Inform Decis Mak. 2024. PMID: 38308231 Free PMC article.
- Methodological quality and reporting quality of COVID-19 living systematic review: a cross-sectional study. Luo J, Chen Z, Liu D, Li H, He S, Zeng L, Yang M, Liu Z, Xiao X, Zhang L. Luo J, et al. BMC Med Res Methodol. 2023 Jul 31;23(1):175. doi: 10.1186/s12874-023-01980-y. BMC Med Res Methodol. 2023. PMID: 37525117 Free PMC article.
- State of the Art of the Molecular Biology of the Interaction between Cocoa and Witches' Broom Disease: A Systematic Review. Santos AS, Mora-Ocampo IY, de Novais DPS, Aguiar ERGR, Pirovani CP. Santos AS, et al. Int J Mol Sci. 2023 Mar 16;24(6):5684. doi: 10.3390/ijms24065684. Int J Mol Sci. 2023. PMID: 36982760 Free PMC article. Review.
- Clarke M, Chalmers I. Reflections on the history of systematic reviews. BMJ Evid. Based Med. 2018; 23: 121-2.
- Oxman AD, Guyatt GH. The science of reviewing research. Ann. N. Y. Acad. Sci. 1993; 703: 125-33.
- Chalmers I, Hedges LV, Cooper H. A brief history of research synthesis. Eval. Health Prof. 2002; 25: 12-37.
- Lind J. A treatise of the scurvy. Three Parts Containing an Inquiry into the Nature, Causes and Cure, of that Disease Together with a Critical and Chronological View of what has been Published on the Subject. Edinburgh: Sands, Murray & Cochran; 1753. Available from: https://www.jameslindlibrary.org/lind-j-1753/ [accessed 16 February 2020].
- Oxman AD, Guyatt GH. Guidelines for reading literature reviews. CMAJ 1988; 138: 697-703.
Publication types
- Search in MeSH
Related information
Linkout - more resources, full text sources.
- Ovid Technologies, Inc.
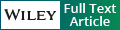
- Citation Manager
NCBI Literature Resources
MeSH PMC Bookshelf Disclaimer
The PubMed wordmark and PubMed logo are registered trademarks of the U.S. Department of Health and Human Services (HHS). Unauthorized use of these marks is strictly prohibited.
1.2.2 What is a systematic review?
A systematic review attempts to collate all empirical evidence that fits pre-specified eligibility criteria in order to answer a specific research question. It uses explicit, systematic methods that are selected with a view to minimizing bias, thus providing more reliable findings from which conclusions can be drawn and decisions made (Antman 1992, Oxman 1993) . The key characteristics of a systematic review are:
a clearly stated set of objectives with pre-defined eligibility criteria for studies;
an explicit, reproducible methodology;
a systematic search that attempts to identify all studies that would meet the eligibility criteria;
an assessment of the validity of the findings of the included studies, for example through the assessment of risk of bias; and
a systematic presentation, and synthesis, of the characteristics and findings of the included studies.
Many systematic reviews contain meta-analyses. Meta-analysis is the use of statistical methods to summarize the results of independent studies (Glass 1976). By combining information from all relevant studies, meta-analyses can provide more precise estimates of the effects of health care than those derived from the individual studies included within a review (see Chapter 9, Section 9.1.3 ). They also facilitate investigations of the consistency of evidence across studies, and the exploration of differences across studies.

Official websites use .gov
A .gov website belongs to an official government organization in the United States.
Secure .gov websites use HTTPS
A lock ( ) or https:// means you've safely connected to the .gov website. Share sensitive information only on official, secure websites.

Systematic Reviews
Describes what is involved with conducting a systematic review of the literature for evidence-based public health and how the librarian is a partner in the process.
Several CDC librarians have special training in conducting literature searches for systematic reviews. Literature searches for systematic reviews can take a few weeks to several months from planning to delivery.
Fill out a search request form here or contact the Stephen B. Thacker CDC Library by email [email protected] or telephone 404-639-1717.
Campbell Collaboration
Cochrane Collaboration
Eppi Centre
Joanna Briggs Institute
McMaster University
PRISMA Statement
Systematic Reviews – CRD’s Guide
Systematic Reviews of Health Promotion and Public Health Interventions
The Guide to Community Preventive Services
Look for systematic reviews that have already been published.
- To ensure that the work has not already been done.
- To provides examples of search strategies for your topic
Look in PROSPERO for registered systematic reviews.
Search Cochrane and CRD-York for systematic reviews.
Search filter for finding systematic reviews in PubMed
Other search filters to locate systematic reviews
A systematic review attempts to collect and analyze all evidence that answers a specific question. The question must be clearly defined and have inclusion and exclusion criteria. A broad and thorough search of the literature is performed and a critical analysis of the search results is reported and ultimately provides a current evidence-based answer to the specific question.
Time: According to Cochrane , it takes 18 months on average to complete a Systematic Review.
The average systematic review from beginning to end requires 18 months of work. “…to find out about a healthcare intervention it is worth searching research literature thoroughly to see if the answer is already known. This may require considerable work over many months…” ( Cochrane Collaboration )
Review Team: Team Members at minimum…
- Content expert
- 2 reviewers
- 1 tie breaker
- 1 statistician (meta-analysis)
- 1 economist if conducting an economic analysis
- *1 librarian (expert searcher) trained in systematic reviews
“Expert searchers are an important part of the systematic review team, crucial throughout the review process-from the development of the proposal and research question to publication.” ( McGowan & Sampson, 2005 )
*Ask your librarian to write a methods section regarding the search methods and to give them co-authorship. You may also want to consider providing a copy of one or all of the search strategies used in an appendix.
The Question to Be Answered: A clearly defined and specific question or questions with inclusion and exclusion criteria.
Written Protocol: Outline the study method, rationale, key questions, inclusion and exclusion criteria, literature searches, data abstraction and data management, analysis of quality of the individual studies, synthesis of data, and grading of the evidience for each key question.
Literature Searches: Search for any systematic reviews that may already answer the key question(s). Next, choose appropriate databases and conduct very broad, comprehensive searches. Search strategies must be documented so that they can be duplicated. The librarian is integral to this step of the process. Before your librarian creates a search strategy and starts searching in earnest you should write a detailed PICO question , determine the inclusion and exclusion criteria for your study, run a preliminary search, and have 2-4 articles that already fit the criteria for your review.
What is searched depends on the topic of the review but should include…
- At least 3 standard medical databases like PubMed/Medline, CINAHL, Embase, etc..
- At least 2 grey literature resources like Clinicaltrials.gov, COS Conference Papers Index, Grey Literature Report, etc…
Citation Management: EndNote is a bibliographic management tools that assist researchers in managing citations. The Stephen B. Thacker CDC Library oversees the site license for EndNote.
To request installation: The library provides EndNote to CDC staff under a site-wide license. Please use the ITSO Software Request Tool (SRT) and submit a request for the latest version (or upgraded version) of EndNote. Please be sure to include the computer name for the workstation where you would like to have the software installed.
EndNote Training: CDC Library offers training on EndNote on a regular basis – both a basic and advanced course. To view the course descriptions and upcoming training dates, please visit the CDC Library training page .
For assistance with EndNote software, please contact [email protected]
Vendor Support and Services: EndNote – Support and Services (Thomson Reuters) EndNote – Tutorials and Live Online Classes (Thomson Reuters)
Getting Articles:
Articles can be obtained using DocExpress or by searching the electronic journals at the Stephen B. Thacker CDC Library.
IOM Standards for Systematic Reviews: Standard 3.1: Conduct a comprehensive systematic search for evidence
The goal of a systematic review search is to maximize recall and precision while keeping results manageable. Recall (sensitivity) is defined as the number of relevant reports identified divided by the total number of relevant reports in existence. Precision (specificity) is defined as the number of relevant reports identified divided by the total number of reports identified.
Issues to consider when creating a systematic review search:
- All concepts are included in the strategy
- All appropriate subject headings are used
- Appropriate use of explosion
- Appropriate use of subheadings and floating subheadings
- Use of natural language (text words) in addition to controlled vocabulary terms
- Use of appropriate synonyms, acronyms, etc.
- Truncation and spelling variation as appropriate
- Appropriate use of limits such as language, years, etc.
- Field searching, publication type, author, etc.
- Boolean operators used appropriately
- Line errors: when searches are combined using line numbers, be sure the numbers refer to the searches intended
- Check indexing of relevant articles
- Search strategy adapted as needed for multiple databases
- Cochrane Handbook: Searching for Studies See Part 2, Chapter 6
A step-by-step guide to systematically identify all relevant animal studies
Materials listed in these guides are selected to provide awareness of quality public health literature and resources. A material’s inclusion does not necessarily represent the views of the U.S. Department of Health and Human Services (HHS), the Public Health Service (PHS), or the Centers for Disease Control and Prevention (CDC), nor does it imply endorsement of the material’s methods or findings. HHS, PHS, and CDC assume no responsibility for the factual accuracy of the items presented. The selection, omission, or content of items does not imply any endorsement or other position taken by HHS, PHS, and CDC. Opinion, findings, and conclusions expressed by the original authors of items included in these materials, or persons quoted therein, are strictly their own and are in no way meant to represent the opinion or views of HHS, PHS, or CDC. References to publications, news sources, and non-CDC Websites are provided solely for informational purposes and do not imply endorsement by HHS, PHS, or CDC.
Systematic Reviews
- What is a Systematic Review?
A systematic review is an evidence synthesis that uses explicit, reproducible methods to perform a comprehensive literature search and critical appraisal of individual studies and that uses appropriate statistical techniques to combine these valid studies.
Key Characteristics of a Systematic Review:
Generally, systematic reviews must have:
- a clearly stated set of objectives with pre-defined eligibility criteria for studies
- an explicit, reproducible methodology
- a systematic search that attempts to identify all studies that would meet the eligibility criteria
- an assessment of the validity of the findings of the included studies, for example through the assessment of the risk of bias
- a systematic presentation, and synthesis, of the characteristics and findings of the included studies.
A meta-analysis is a systematic review that uses quantitative methods to synthesize and summarize the pooled data from included studies.
Additional Information
- How-to Books
- Beyond Health Sciences
- Cochrane Handbook For Systematic Reviews of Interventions Provides guidance to authors for the preparation of Cochrane Intervention reviews. Chapter 6 covers searching for reviews.
- Systematic Reviews: CRD’s Guidance for Undertaking Reviews in Health Care From The University of York Centre for Reviews and Dissemination: Provides practical guidance for undertaking evidence synthesis based on a thorough understanding of systematic review methodology. It presents the core principles of systematic reviewing, and in complementary chapters, highlights issues that are specific to reviews of clinical tests, public health interventions, adverse effects, and economic evaluations.
- Cornell, Sytematic Reviews and Evidence Synthesis Beyond the Health Sciences Video series geared for librarians but very informative about searching outside medicine.
- << Previous: Getting Started
- Next: Levels of Evidence >>
- Getting Started
- Levels of Evidence
- Locating Systematic Reviews
- Searching Systematically
- Developing Answerable Questions
- Identifying Synonyms & Related Terms
- Using Truncation and Wildcards
- Identifying Search Limits/Exclusion Criteria
- Keyword vs. Subject Searching
- Where to Search
- Search Filters
- Sensitivity vs. Precision
- Core Databases
- Other Databases
- Clinical Trial Registries
- Conference Presentations
- Databases Indexing Grey Literature
- Web Searching
- Handsearching
- Citation Indexes
- Documenting the Search Process
- Managing your Review
Research Support
- Last Updated: Aug 14, 2024 11:07 AM
- URL: https://guides.library.ucdavis.edu/systematic-reviews
Study Design 101: Systematic Review
- Case Report
- Case Control Study
- Cohort Study
- Randomized Controlled Trial
- Practice Guideline
- Systematic Review
- Meta-Analysis
- Helpful Formulas
- Finding Specific Study Types
A document often written by a panel that provides a comprehensive review of all relevant studies on a particular clinical or health-related topic/question. The systematic review is created after reviewing and combining all the information from both published and unpublished studies (focusing on clinical trials of similar treatments) and then summarizing the findings.
- Exhaustive review of the current literature and other sources (unpublished studies, ongoing research)
- Less costly to review prior studies than to create a new study
- Less time required than conducting a new study
- Results can be generalized and extrapolated into the general population more broadly than individual studies
- More reliable and accurate than individual studies
- Considered an evidence-based resource
Disadvantages
- Very time-consuming
- May not be easy to combine studies
Design pitfalls to look out for
Studies included in systematic reviews may be of varying study designs, but should collectively be studying the same outcome.
Is each study included in the review studying the same variables?
Some reviews may group and analyze studies by variables such as age and gender; factors that were not allocated to participants.
Do the analyses in the systematic review fit the variables being studied in the original studies?
Fictitious Example
Does the regular wearing of ultraviolet-blocking sunscreen prevent melanoma? An exhaustive literature search was conducted, resulting in 54 studies on sunscreen and melanoma. Each study was then evaluated to determine whether the study focused specifically on ultraviolet-blocking sunscreen and melanoma prevention; 30 of the 54 studies were retained. The thirty studies were reviewed and showed a strong positive relationship between daily wearing of sunscreen and a reduced diagnosis of melanoma.
Real-life Examples
Yang, J., Chen, J., Yang, M., Yu, S., Ying, L., Liu, G., ... Liang, F. (2018). Acupuncture for hypertension. The Cochrane Database of Systematic Reviews, 11 (11), CD008821. https://doi.org/10.1002/14651858.CD008821.pub2
This systematic review analyzed twenty-two randomized controlled trials to determine whether acupuncture is a safe and effective way to lower blood pressure in adults with primary hypertension. Due to the low quality of evidence in these studies and lack of blinding, it is not possible to link any short-term decrease in blood pressure to the use of acupuncture. Additional research is needed to determine if there is an effect due to acupuncture that lasts at least seven days.
Parker, H.W. and Vadiveloo, M.K. (2019). Diet quality of vegetarian diets compared with nonvegetarian diets: a systematic review. Nutrition Reviews , https://doi.org/10.1093/nutrit/nuy067
This systematic review was interested in comparing the diet quality of vegetarian and non-vegetarian diets. Twelve studies were included. Vegetarians more closely met recommendations for total fruit, whole grains, seafood and plant protein, and sodium intake. In nine of the twelve studies, vegetarians had higher overall diet quality compared to non-vegetarians. These findings may explain better health outcomes in vegetarians, but additional research is needed to remove any possible confounding variables.
Related Terms
Cochrane Database of Systematic Reviews
A highly-regarded database of systematic reviews prepared by The Cochrane Collaboration , an international group of individuals and institutions who review and analyze the published literature.
Exclusion Criteria
The set of conditions that characterize some individuals which result in being excluded in the study (i.e. other health conditions, taking specific medications, etc.). Since systematic reviews seek to include all relevant studies, exclusion criteria are not generally utilized in this situation.
Inclusion Criteria
The set of conditions that studies must meet to be included in the review (or for individual studies - the set of conditions that participants must meet to be included in the study; often comprises age, gender, disease type and status, etc.).
Now test yourself!
1. Systematic Reviews are similar to Meta-Analyses, except they do not include a statistical analysis quantitatively combining all the studies.
a) True b) False
2. The panels writing Systematic Reviews may include which of the following publication types in their review?
a) Published studies b) Unpublished studies c) Cohort studies d) Randomized Controlled Trials e) All of the above
Evidence Pyramid - Navigation
- Meta- Analysis
- Case Reports
- << Previous: Practice Guideline
- Next: Meta-Analysis >>

- Last Updated: Sep 25, 2023 10:59 AM
- URL: https://guides.himmelfarb.gwu.edu/studydesign101

- Himmelfarb Intranet
- Privacy Notice
- Terms of Use
- GW is committed to digital accessibility. If you experience a barrier that affects your ability to access content on this page, let us know via the Accessibility Feedback Form .
- Himmelfarb Health Sciences Library
- 2300 Eye St., NW, Washington, DC 20037
- Phone: (202) 994-2962
- [email protected]
- https://himmelfarb.gwu.edu
Thank you for visiting nature.com. You are using a browser version with limited support for CSS. To obtain the best experience, we recommend you use a more up to date browser (or turn off compatibility mode in Internet Explorer). In the meantime, to ensure continued support, we are displaying the site without styles and JavaScript.
- View all journals
- Explore content
- About the journal
- Publish with us
- Sign up for alerts
- Open access
- Published: 06 September 2024
Systematic re-review of WASH trials to assess women’s engagement in intervention delivery and research activities
- Bethany A. Caruso ORCID: orcid.org/0000-0001-9738-9857 1 ,
- April M. Ballard ORCID: orcid.org/0000-0003-3839-5637 2 ,
- Julia Sobolik ORCID: orcid.org/0000-0002-4759-8690 2 ,
- Madeleine Patrick ORCID: orcid.org/0000-0002-5599-8459 1 ,
- Janice Dsouza ORCID: orcid.org/0009-0001-0779-2544 1 ,
- Sheela S. Sinharoy ORCID: orcid.org/0000-0003-3077-3824 1 ,
- Oliver Cumming ORCID: orcid.org/0000-0002-5074-8709 3 ,
- Jennyfer Wolf ORCID: orcid.org/0000-0002-4242-4149 4 &
- Isha Ray ORCID: orcid.org/0000-0002-9966-8822 5
Nature Water ( 2024 ) Cite this article
Metrics details
- Development studies
- Environmental social sciences
- Infectious diseases
Water, sanitation and hygiene (WASH) interventions significantly reduce health risks in low- and middle-income countries. Many rely on women, but the extent of women’s engagement remains undocumented. Here we conducted a re-review of papers from two systematic reviews that assessed the effectiveness of water, sanitation and/or handwashing with soap interventions on diarrhoeal disease and acute respiratory infections to assess women’s roles in WASH research and intervention activities. A total of 133 studies were included. Among studies that specified gender, women were the most sought-after group for engagement in research ( n = 91/132; 68.9%) and intervention ( n = 49/120; 40.8%) activities. Reporting time burden for research ( n = 1; 1%) and intervention activities ( n = 3; 2.5%) was rare. All interventions were classified as gender unequal (36.7%) or gender unaware (63.3%) according to the World Health Organization Gender Responsiveness Assessment Scale, indicating exploitative engagement. Women play a critical but instrumentalized role in WASH, and both research and interventions need to change to enable, and not hinder, gender equality.
Water, sanitation and hygiene (WASH) services are foundational to human health and well-being. The results from two recent systematic reviews found that WASH services can significantly reduce the risk of diarrhoea and acute respiratory infections (ARIs) in low- and middle-income countries 1 , 2 . Recent disease burden estimates suggest that 1.4 million deaths and 74 million disability-adjusted life years were attributable to unsafe WASH in 2019 3 . However, due to a paucity of evidence, these estimates do not account for multiple other health outcomes related to WASH, including bodily injury, violence and stress 3 , which are often of particular concern for women and girls 4 , 5 , 6 , 7 .
In 2022, an estimated 27% of the global population (2.2 billion) lacked access to safely managed drinking water services, 43% (3.5 billion) to safely managed sanitation services and 25% (2 billion) to basic hygiene services 8 . As women and girls play a central, and sometimes outsized 9 , role in managing household WASH resources, these inadequate conditions place considerable burdens on them 4 . Due to gender norms, women and girls often bear responsibility for household WASH: time-consuming and physically arduous activities such as water fetching, latrine cleaning and keeping children clean 9 , 10 , 11 , 12 , 13 . While these activities may produce improvements in overall health, they demand women’s time and energy, limit opportunities 14 and may result in risks to their own health and safety 4 , 15 . They could, therefore, perpetuate gender inequity 16 .
While these unpaid burdens and norms have been acknowledged, they also have been exploited by research and practice initiatives 15 , where women are often intentionally targeted by those delivering WASH programmes as key instruments for their success. For example, women have been trained to carry out water treatment, safe child faeces management and hand hygiene promotion, largely justified by the expected benefits for child health outcomes 12 , 17 , 18 , 19 . Yet assessment of the extent, and impact, of women’s engagement in WASH programmes for public health on women’s own health and well-being has been limited. A recent review of adoption of point-of-use chlorination for treating household drinking water found that most interventions deliberately targeted women to perform water treatment tasks, leveraging their household water management and caregiving roles. The time burden associated with this work was often reported to be a barrier to use but was seldom quantified 18 . Similarly, women are routinely expected to participate in research activities as part of large - scale WASH evaluations to provide detailed data about family members’ behaviours (for example, defecation practices) 19 and health (for example, diarrhoea) 20 , 21 , 22 . These examples point to the need for comprehensive assessment of the central part that women play in health-related WASH research and practice.
Despite heavily involving women, rarely have WASH interventions and evaluations been designed and delivered specifically to improve or even understand their impact on women’s lives. WASH interventions should, however, be evaluated to understand whether and how potential burdens and benefits from these interventions have been distributed, and whether and how participants’ engagement reinforces existing gender roles. While some WASH interventions, such as household water treatment, place demands on women, others could relieve them. For example, piped water systems or passive chlorination devices may not only reduce child illness, they also could eliminate the time and labour required to fetch and treat water and the time, financial and psycho-social costs of caring for sick household members. Yet, these co-benefits are rarely assessed 23 .
The aim of this re-review is to assess how women are engaged in health-related WASH research and intervention activities. To do so, we conducted a re-review of papers from two recent systematic reviews published in The Lancet that assessed effectiveness of water, sanitation and/or handwashing with soap interventions on diarrhoeal disease 1 and ARIs 2 . Specifically, in eligible studies we (1) identify the gender of the individual(s) engaged in research and intervention activities; (2) determine whether time required for engagement was reported and, if so, compensated; (3) discern whether additional intervention impacts specific to women were assessed; and (4) characterize intervention engagement overall and by intervention type using the World Health Organization (WHO) Gender Responsiveness Assessment Scale (GRAS) 24 .
Assessing gender responsiveness in interventions
The WHO GRAS 24 for assessing gender responsiveness in health interventions, policies and programmes, and its elaboration by Pederson et al. 25 is well suited for assessing intervention engagement. The WHO GRAS presents a spectrum of five gender approaches, from those that should be avoided (gender unequal and gender blind) to those that are more desirable (gender sensitive, gender specific and gender transformative). The modification by Pederson et al. 25 includes all five approaches and also shows how health programmes, policies and interventions along these various levels can exploit, accommodate or transform gender inequities, depending on how they are designed and delivered. A recent iteration of the scale critiques the use of the term ‘gender blind’ and collapses the gender-unequal and gender-blind categories into one (‘gender insensitive’) 26 . We feel that these two categories are distinct and critical to retain for our analysis. Our slightly modified scale changes the term ‘gender blind’ to ‘gender unaware’, includes the definitions of all five categories as presented by the WHO 24 and Pederson et al. 25 , builds upon the definition of gender unequal and provides hypothetical WASH examples along the scale (Fig. 1 ).
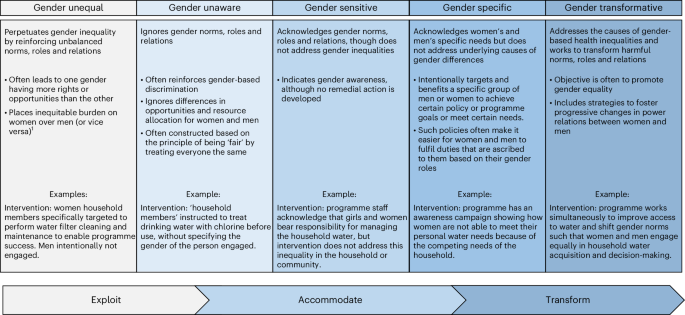
The GRAS was developed by the WHO 24 to assess health programmes, policies and interventions, depicting those approaches that should be avoided (gender unequal and gender blind) to those that are more desirable (gender sensitive, gender specific and gender transformative). In 2014, Pederson et al. modified the GRAS by adding the labels at the bottom to show that approaches aligned with the noted categories can exploit, accommodate or transform gender inequities, depending on how they are designed and delivered. The figure depicted here leverages the WHO GRAS and its modification by Pederson et al. (2014), changes one label from ‘gender blind’ to ‘gender unaware’ based on a recent critique (MacArthur 26 ), builds upon the definition of gender unequal and provides hypothetical WASH examples along the scale. 1 This portion of the ‘gender unequal’ definition was expanded upon by the co-authors.
Reporting sex-disaggregated outcome data was rare
We assessed all 150 studies included in the 2 reviews: 14 were duplicates and 3 were excluded due to language (Chinese, Danish and French), resulting in a final sample of 133 studies (Supplementary Fig. 1 , flow diagram). We identified one intervention that appeared in two studies: one study 27 was included in the diarrhoea systematic review and the other 28 was in the ARI systematic review. As these papers could have engaged individuals differently in the research activities assessing their focal outcomes (diarrhoea and ARI) and because each could have described individual engagement in intervention activities differently, we elected to retain both and treat them independently. Studies with interventions that focused solely on drinking water ( n = 64; 48.1%) or hygiene ( n = 46; 34.6%) were the most common. Most studies took place in rural settings ( n = 80; 60.2%) and in Asia ( n = 51; 38.3%) and Africa ( n = 43; 32.3%). Only eight (6.0%) studies presented sex-disaggregated outcome data (Table 1 ). Supplementary Table 1 presents a list of included studies and key characteristics.
Research activities largely depended on women
Individual-level participation in research activities was almost universal. Nearly all studies ( n = 132; 99.2%) included at least one research activity that necessitated individual-level participation (Table 2 ). Of those, 92 (69.7%) studies engaged multiple groups in research activities and over half ( n = 89; 67.4%) did not clearly specify who was engaged for at least one research activity. Overall, among participants specified, women were the most frequently engaged, followed by children. Women were reported to be engaged in 91 (68.9%) studies for research activities and were the only such group in 14 (10.6%) studies. The most common research activities that women were engaged in were surveys (for example, baseline and endline) ( n = 63; 47.7%), diarrhoea recall (independent of other surveys) (53; 40.2%), behaviour/practice recall (independent of other surveys) (18; 13.6%) and observation (18; 13.6%) (Table 3 ). Children were engaged in 47 (35.6%) studies and were never the only participating group. Their participation was most sought for biological specimens, including stool or rectal swabs (15; 11.4%) and sera samples (9; 6.8%). Only two (1.5%) studies reported engaging men for any research activities (survey and qualitative research).
The majority of studies depended on women to report the primary outcome (for example, child diarrhoea), yet few reported additional outcomes related to women’s own health or co-benefits (if any) such as time savings. In 83 (63.4%) studies, women—specifically mothers—were noted to have reported the study’s focal outcome. Most studies (111; 83.5%) reported additional outcomes other than diarrhoea and/or ARI. These additional outcomes related mainly to children ( n = 60; 54.1%). Almost all ( n = 59; 98.3%) reported on well-being outcomes (for example, growth, parasitic infection or school absence) and 10 (16.7%) reported on programme-related outcomes (for example, hand hygiene or defecation behaviour). Sixteen studies (14.4%) reported outcomes specific to women; three reported outcomes related to women’s well-being (for example, childcare hours saved, satisfaction with sanitation and water fetching time), while the rest focused on programme-related compliance (for example, hand hygiene, water treatment behaviours and so on). Four (3.6%) studies reported outcomes specific to men; two focused on men’s well-being (for example, time fetching water and satisfaction with sanitation) and two on programmatic outcomes (for example, defecation behaviours). Only one study (0.8%) comprehensively reported how much time was required for participants to engage in the research activities 29 and five (3.8%) studies compensated those engaged in research for their time (Table 2 ).
Women most targeted to carry out intervention activities
The majority of interventions required individual-level participation and most depended on women. One hundred twenty studies (90.2%) included at least one intervention activity that necessitated individual-level participation. Of those, 48 (40.0%) studies included intervention activities that engaged multiple groups, but over half of the studies ( n = 76; 63.3%) did not specify who was engaged. Among those studies that specified, women were the most targeted for engagement in intervention activities, followed by children. Forty-nine (40.8%) studies specifically targeted women for participation, including 21 (17.5%) that targeted only women (Table 2 ). Among intervention activities, women were the most targeted group, including for all water- (46; 38.3%), sanitation- (15; 12.5%), hygiene- (35; 29.2%) and health promotion-related (29; 24.2%) activities, which included WASH-related health education, water treatment and child faeces management, among other activities (Table 4 ). Twenty (16.7%) intervention activities targeted children, including 2 (1.7%) that only targeted children. Children were most engaged in activities focused on hygiene practices and education. Only two (1.7%) studies reported targeting men for any intervention activities (hygiene-related health education and health promotion). Table 4 summarizes all intervention activities by populations engaged.
Despite the near ubiquitous need for individual-level participation in the WASH interventions assessed, studies rarely reported time burden or compensation: only 3 (2.5%) studies reported the time burden of engaging in intervention activities and 13 (10.8%) reported providing compensation to individuals for their time (Table 2 ). From the information reported, ten (8.4%) studies explicitly referenced gender norms as an intentional part of their interventions; these referenced shame, honour or upholding traditional values (for example, campaigns that promoted handwashing as practiced by ‘good mothers’).
All interventions were gender unequal or gender unaware
We classified all study interventions as either gender unequal (36.7%) or gender unaware (63.7%) (Table 5 ), categorizations that are termed exploitative by WHO and that are, by extension, not recommended.
Of the 77 studies that included water interventions, 9 (11.7%) did not require any individual participation and among the remaining 68 that did, 24 (35.3%) are gender unequal and 44 (64.7%) are gender unaware (Table 5 ). Supplementary Table 2 presents examples of gender-unequal and gender-unaware water intervention activities. Disaggregating by different levels of drinking water services, 57 (74.0%) involved point-of-use water treatment for sources off premises, a low level of service according to the exposure scenario. Among these, 22 (38.6%) are categorized as gender unequal and 35 (61.4%) as gender unaware. The one intervention that provided improved, on premises, continuous water supply—one of the higher levels of water service represented by the exposure scenario—was not evaluated using the GRAS framework as it did not require individual-level involvement (Fig. 2 ).
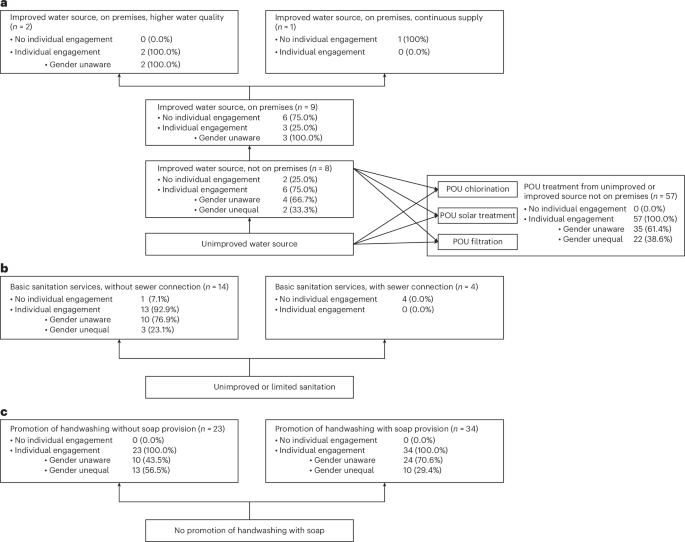
a – c , Depicted exposure scenarios adapted from Wolf et al. 1 who used the exposure scenarios to determine diarrhoea risk at the various WASH service levels. The authors concluded that higher service levels provided increased protection from diarrhoeal pathogens. We used the same exposure scenarios to determine whether and how gender responsiveness varied at the different service levels for water ( a ), sanitation ( b ) and hygiene ( c ). We found high levels of exploitative engagement at lower service levels. POU, point of use.
Of the 18 studies that included sanitation interventions, five (27.8%) did not require any individual involvement and among the remaining 13 that did, all provided basic sanitation services without sewage connection with three (23.1%) categorized as gender unequal and 10 (76.9%) as gender unaware. (Table 5 ). The four interventions that provided basic sanitation with sewer connections were not evaluated using the GRAS framework as they did not require individual-level involvement (Fig. 2 ).
Of the 57 studies that included hygiene interventions, all required individual-level involvement and involved some type of hand hygiene promotion, though only 34 (59.6%) provided soap. Fewer interventions that provided soap were gender unequal ( n = 10; 29.4%) than were gender unaware ( n = 24; 70.6%). Conversely, a greater proportion of the hand hygiene promotion interventions that did not provide soap were gender unequal ( n = 13; 56.5%) rather than gender unaware ( n = 10; 43.5%) (Fig. 2 and Table 5 ).
For the two studies that reported different outcomes related to the same intervention 27 , 28 , the study reporting on diarrhoea as the focal outcome 27 did not specify who was targeted for engagement in intervention activities, leading to a GRAS assessment of ‘gender unaware’. Conversely, the study reporting on ARI as the focal outcome 28 did specify who was targeted for engagement in intervention activities, leading to a GRAS assessment of ‘gender unequal’.
In this re-review of 133 studies from two systematic reviews assessing effectiveness of water, sanitation and/or handwashing with soap interventions on diarrhoeal disease 1 and ARIs 2 , we evaluated the reported engagement of individuals in the evaluation and delivery of WASH interventions. We find that, in many instances, the interventions that were the subject of these studies relied entirely on women as the agents of delivery and as the source of study data. WASH interventions were overwhelmingly gender unequal or gender unaware and therefore classified as gender exploitative under the GRAS framework (Fig. 1 ). Any costs to women’s own time or benefits to their own lives were rarely mentioned. Women appear to play a critical but purely instrumentalized role in advancing WASH. The often-unacknowledged role of women in the evaluation and implementation of health-related WASH studies has several unintended, yet detrimental, consequences that require change for WASH if it is to enable gender equality and not hinder it.
Perhaps the most insidious consequence of taking for free and for granted women’s time and cooperation in WASH is that it cements existing and unequal gender norms. Maintaining family health, which includes WASH tasks, is considered to be women’s work, and women’s labour is ‘understood’ to be of low value. When WASH implementers and researchers not only avoid playing an equalizing role, but actively exploit gender-unequal roles, then existing inequalities are reproduced 30 or even strengthened 16 . These observations—that women perform unpaid WASH labour and that this renders the research itself exploitative—are not new; this bias has been described since the early 1980s 31 , 32 , 33 . Nonetheless, the exploitation of gender stereotypes and acceptance of numerous hours of unpaid labour by women has persisted. While occurring in both research and intervention delivery, the impact of engagement is probably quite different; the demand for women’s unpaid labour in intervention delivery, in particular, could be sustained indefinitely or even scaled up if deemed effective at improving child health. Moving forward, WASH programmes and interventions should be evaluated using the GRAS tool, or similar adaptations 26 , before implementation so those classified as exploitative can be re-designed or abandoned. Exploitative interventions should not be funded for evaluation.
Regardless of the effectiveness of the WASH interventions assessed, the full implementation costs have not been transparently acknowledged in evaluations or reflected in subsequent recommendations. Many WASH approaches, especially household-based approaches, are touted as ‘low cost’ by depending on women’s ‘free’ time and labour. These falsely low costs are routinely highlighted as a benefit for—and even made a stipulation by—policymakers and donors, who often demand evidence of cost-effectiveness, put caps on the total costs allowed for an intervention and restrict the types of allowable expenses (for example, participant compensation). We acknowledge that the extent and nature of compensation must be context specific so as not to place undue burdens on low-income communities and non-governmental organizations, and we recommend that WASH actors (1) budget appropriate compensation for those who shoulder the burdens of making these interventions ‘work’, (2) transparently report who is engaged and (3) rigorously evaluate participant time and opportunity costs. When reporting who is engaged, the Sex and Gender Equity in Research (SAGER) guidelines, which have been adopted by the WHO 34 , are a useful reference. Designed to eliminate gender data gaps, the SAGER guidelines provide recommendations for reporting disaggregated sex and gender data, and also encourage including sex and gender dimensions when designing research, collecting data and undergoing analyses 35 . Further, gender has been acknowledged as just one of many characteristics that intersect to contribute to inequalities related to WASH 36 , 37 , and therefore an intersectional lens is also relevant to identifying additional markers of identity that may compound experiences of inequality beyond gender alone 38 .
The gender-unequal or gender-unaware interventions were largely among interventions that represent lower levels of service, illuminating how these allegedly low-cost interventions not only demand ‘free’ labour, but extract this labour to provide services or promote approaches that are often inferior. Interventions at the lowest service levels often emphasize behaviour change and, as we and others 18 have shown, most target women’s behaviour change. Yet behaviour change approaches are ‘generally the least effective type of intervention’ 39 . Furthermore, ‘the need to urge behavioural change is symptomatic of failure to establish contexts in which healthy choices are default actions’ 39 . As a result, the women conscripted to perform (or enforce) WASH behaviours are probably living in the least enabling environments and therefore may have little chance for impact despite their efforts. Failed behaviour-change interventions tend to be ascribed to poor ‘compliance’, which blames individuals—largely women—for intervention failure as opposed to the possible inappropriateness of the approach itself 40 . Our data show that factors that shape individual ability to adopt interventions (for example, time and finances)—which are useful to assess intervention appropriateness—were rarely documented. In contrast, the most common reported outcomes related to women were about their ‘compliance’ behaviours. Higher WASH service levels are critical for health 3 and for establishing contexts that enable healthy choices including relieving women’s labour, saving energy costs and time and lowering stress.
WASH provision at higher service levels does tend to require less household work, but cannot guarantee that women will not be burdened or that their needs will be met. WASH approaches therefore need to be intentionally gender sensitive, at a minimum. The Joint Monitoring Programme for Water Supply, Sanitation and Hygiene service ladders, which function as the benchmark by which to evaluate the quality of WASH services, are notably gender unaware 41 and therefore insufficient as the only benchmark. As an example, toilets can be categorized to be at the highest service level (safely managed) even if they lack a superstructure or a door because the ladder does not assess privacy. The global WASH community is already calling for a paradigm shift in how WASH services are delivered and evaluated 42 . Consistent with this call, we recommend that potential gender-related needs, burdens and benefits are formally included when assessing the quality of WASH services, as well as in WASH evaluations when assessing their effectiveness in preventing disease.
A shift is also needed in how evaluations of WASH interventions are conceived, conducted and communicated to prevent further gender exploitation. As with intervention delivery, studies are not always explicit about who is engaged in research activities, women are routinely engaged, compensation is rare and few report the time participation required. Women, in effect, act as unpaid research assistants. While there remain debates about research compensation 43 , researchers and donors should be deliberate about time required from research participants and justify compensation decisions transparently.
These conclusions are limited by the information reported in the papers assessed, did not consider studies that may have been published elsewhere, excluded evaluations in languages other than English or Spanish and may have a restricted sample because of the sources from which included studies were identified. Despite any potential sample limitations, our findings demonstrate a clear trend in how women have been engaged in research and intervention delivery, calling into question how women may have been engaged in other WASH intervention studies, whether seeking to improve health outcomes or not. The approach used herein can and should be used to interrogate other WASH research and interventions, regardless of whether they seek to improve health outcomes, and to any other research and intervention activities that rely on individuals to perform activities.
Our re-review nonetheless takes a gender lens to prominent studies used to determine intervention effectiveness on key health outcomes. This lens should be considered when assessing the health impacts of WASH interventions. Specifically, women have been critical to evaluation research and intervention delivery and yet are often invisible and undervalued in the public health literature. Greater awareness and reflexivity are needed within WASH research and practice to elevate and value gender equity alongside health impacts.
We re-reviewed papers from two recent systematic reviews published in The Lancet that assessed effectiveness of water, sanitation and/or handwashing with soap interventions on diarrhoeal disease 1 and ARIs 2 . The search terms, inclusion and exclusion criteria, risk of bias and GRADE (Grading of Recommendations, Assessment, Development and Evaluation) scores can be found in these two reviews and their supplementary appendices. The protocol is registered with PROSPERO (CRD42022346360). We report findings following the Preferred Reporting Items for Systematic Reviews and Meta-Analyses criteria (Supplementary Tables 3 and 4 ).
Inclusion criteria and eligibility
All papers included in the two previously published systematic reviews were eligible for inclusion if published in English or Spanish. Studies were excluded if not in English or Spanish 1 , 2 .
Data extraction
Two reviewers independently extracted data from each article using a common data extraction template in excel (extraction sheet available with the public dataset on Figshare at https://doi.org/10.6084/m9.figshare.25786638 ). To test the data extraction sheet and to ensure consistency in the process, reviewers first extracted data from the same five articles and compared their data. Any disagreements were discussed to ensure common understanding before official extraction was initiated. Once data had been extracted twice from each article, the two completed data extraction sheets were compared to identify any inconsistencies. If there was an inconsistency, one team member returned to the study to re-extract the relevant data.
To identify the gender of individuals engaged in research and intervention activities, reviewers first extracted data on whether intervention and evaluation activities required individual-level participation from the target households/communities. Among those that required individual-level participation, reviewers identified who was engaged (women, men, men and/or women, girls, boys, girls and/or boys, other specified and/or unspecified individuals/populations). The dataset provides more detail on terms used and categorization assumptions (for example, mother, caregiver or categorized as ‘women’). They further extracted data on the time required for engagement and compensation provided (if these were reported); who reported the study’s focal outcome (for example, child diarrhoea); if any additional intervention impacts specific to women, men, girls or boys were assessed; and if the intervention activities included messages that involved shame or honour (for example, establishing norms of ‘good’ parenting).
We used the adapted GRAS figure (Fig. 1 ) as a tool to assess gender responsiveness in those interventions that required individual-level participation. As with the extraction for the other variables, two reviewers independently reviewed intervention descriptions from the included studies and categorized them using the definitions noted in the figure. As categorization is more subjective than extraction for the other variables, any inconsistencies in categorization were reconciled through discussion with a third team member. Interventions with multiple components can have different GRAS categories for each component 24 , thus we categorized each water, sanitation and/or hygiene component in an intervention separately and provided an overall categorization of the intervention. We did not assess any non-WASH (for example, nutrition) intervention components.
We used R Studio v4.0.5 to generate descriptive statistics about which individuals were engaged in the research and interventions assessed; how they were engaged; what additional outcomes, if any, were evaluated; and how the interventions were categorized using the GRAS categories. We further organized the GRAS data by the WASH exposure scenarios presented by Wolf et al. 1 . These exposure scenarios were informed by the definitions and exposure levels of the service ladders created by the WHO/UNICEF Joint Monitoring Programme for Water Supply, Sanitation and Hygiene to assess progress against SDG targets 6.1 and 6.2, and were adapted based on available evidence. Wolf et al. 1 used the exposure scenarios to determine diarrhoea risk at the various WASH service levels and concluded that higher service levels provided increased protection from diarrhoeal pathogens. We used the same exposure scenarios to determine whether and how gender responsiveness varied at the different service levels.
Positioning and role of the funding source
The authors describe how their experiences and perspectives informed the re-review in Supplementary Text 1 .
The funders of the study had no role in study design, data collection, analysis, interpretation, writing of the report or decision to publish.
Data availability
All data are publicly available on Figshare at https://doi.org/10.6084/m9.figshare.25786638 (ref. 44 ).
Wolf, J. et al. Effectiveness of interventions to improve drinking water, sanitation, and handwashing with soap on risk of diarrhoeal disease in children in low-income and middle-income settings: a systematic review and meta-analysis. Lancet 400 , 48–59 (2022).
Article PubMed Google Scholar
Ross, I. et al. Effectiveness of handwashing with soap for preventing acute respiratory infections in low-income and middle-income countries: a systematic review and meta-analysis. Lancet 401 , 1681–1690 (2023).
Wolf, J. et al. Burden of disease attributable to unsafe drinking water, sanitation, and hygiene in domestic settings: a global analysis for selected adverse health outcomes. Lancet 401 , 2060–2071 (2023).
Article PubMed PubMed Central Google Scholar
Caruso, B. A. et al. Water, sanitation, and women’s empowerment: a systematic review and qualitative metasynthesis. PLoS Water 1 , e0000026 (2022).
Article Google Scholar
Sclar, G. D. et al. Exploring the relationship between sanitation and mental and social well-being: a systematic review and qualitative synthesis. Soc. Sci. Med. 217 , 121–134 (2018).
Article CAS PubMed Google Scholar
Crider, Y. S. & Ray I. Water and development: a gender perspective. Oxford Research Encyclopedia of Environmental Science https://doi.org/10.1093/acrefore/9780199389414.013.685 (2022).
Venkataramanan, V. et al. In pursuit of ‘safe’water: the burden of personal injury from water fetching in 21 low-income and middle-income countries. BMJ Glob. Health 5 , e003328 (2020).
Progress on Household Drinking Water, Sanitation and Hygiene 2000–2020: Five Years into the SDGs (World Health Organization/UNICEF, 2021).
Caruso, B. A. Water is life, particularly for women. Nat. Water 1 , 124 (2023).
Sorenson, S. B., Morssink, C. & Campos, P. A. Safe access to safe water in low income countries: water fetching in current times. Soc. Sci. Med. 72 , 1522–1526 (2011).
Graham, J. P., Hirai, M. & Kim, S. S. An analysis of water collection labor among women and children in 24 Sub-Saharan African countries. PLoS ONE 11 , e0155981 (2016).
Cavill, S. & Huggett, C. Good mums: a gender equality perspective on the constructions of the mother in handwashing campaigns. wH2O J. Gend. Water 7 , 26–36 (2020).
Google Scholar
Kwiringira, J., Atekyereza, P., Niwagaba, C. & Günther, I. Gender variations in access, choice to use and cleaning of shared latrines; experiences from Kampala slums, Uganda. BMC Public Health 14 , 1180 (2014).
Cherukumilli, K., Ray, I. & Pickering, A. J. Evaluating the hidden costs of drinking water treatment technologies. Nat. Water 1 , 319–327 (2023).
Caruso, B. A., Chipungu, J., Kulkarni, S. & Ray, I. Women, work, and water. Lancet 401 , 1139–1141 (2023).
Michalak, A. M. et al. The frontiers of water and sanitation. Nat. Water 1 , 10–18 (2023).
Fisher, J., Cavill, S. & Reed, B. Mainstreaming gender in the WASH sector: dilution or distillation? Gend. Dev. 25 , 185–204 (2017).
Crider, Y. S., Tsuchiya, M., Mukundwa, M., Ray, I. & Pickering, A. J. Adoption of point-of-use chlorination for household drinking water treatment: a systematic review. Environ. Health Perspect. 131 , 016001 (2023).
Article CAS PubMed PubMed Central Google Scholar
Caruso, B. A. et al. Effect of a low-cost, behaviour-change intervention on latrine use and safe disposal of child faeces in rural Odisha, India: a cluster-randomised controlled trial. Lancet Planet. Health 6 , e110–e121 (2022).
Arnold, B. F. et al. Cluster-randomised controlled trials of individual and combined water, sanitation, hygiene and nutritional interventions in rural Bangladesh and Kenya: the WASH benefits study design and rationale. BMJ Open 3 , e003476 (2013).
Reese, H. et al. Design and rationale of a matched cohort study to assess the effectiveness of a combined household-level piped water and sanitation intervention in rural Odisha, India. BMJ Open 7 , e012719 (2017).
Humphrey, et al. The Sanitation Hygiene Infant Nutrition Efficacy (SHINE) trial: rationale, design, and methods. Clin. Infect. Dis. 61 , S685–S702 (2015).
Caruso, B. A. & Sinharoy, S. S. Gender data gaps represent missed opportunities in WASH. Lancet Glob. Health 7 , e1617 (2019).
Gender Mainstreaming for Health Managers: A Practical Approach (World Health Organization, 2011).
Pederson, A., Greaves, L. & Poole, N. Gender-transformative health promotion for women: a framework for action. Health Promot. Int. 30 , 140–150 (2014).
MacArthur, J. et al. Gender equality approaches in water, sanitation, and hygiene programs: towards gender-transformative practice. Front. Water 5 , 1090002 (2023).
Luby, S. P. et al. Effect of intensive handwashing promotion on childhood diarrhea in high-risk communities in Pakistan: a randomized controlled trial. JAMA 291 , 2547–2554 (2004).
Luby, S. P. et al. Effect of handwashing on child health: a randomised controlled trial. Lancet 366 , 225–233 (2005).
Harshfield, E., Lantagne, D., Turbes, A. & Null, C. Evaluating the sustained health impact of household chlorination of drinking water in rural Haiti. Am. J. Trop. Med. Hyg. 87 , 786–795 (2012).
Tough, H., Abdallah, A.-K., Zemp, E. & Molesworth, K. Gender dynamics of community-led total sanitation interventions in Mpwapwa District, Tanzania. Glob. Public Health 18 , 2053733 (2022).
Ilahi, N. & Grimard, F. Public infrastructure and private costs: water supply and time allocation of women in rural Pakistan. Econ. Dev. Cult. Change 49 , 45–75 (2000).
Ray, I. Women, water, and development. Annu. Rev. Environ. Resour. 32 , 421–449 (2007).
Beneria, L. & Sen, G. Class and gender inequalities and women’s role in economic development: theoretical and practical implications. Fem. Stud. 8 , 157–176 (1982).
Heidari, S. et al. WHO’s adoption of SAGER guidelines and GATHER: setting standards for better science with sex and gender in mind. Lancet 403 , 226–228 (2024).
Heidari, S., Babor, T. F., De Castro, P., Tort, S. & Curno, M. Sex and gender equity in research: rationale for the SAGER guidelines and recommended use. Res. Integ. Peer Rev. 1 (2016).
Narayanaswamy, L. et al. Why a feminist ethics of care and socio-ecological justice lens matter for global, interdisciplinary research on water security. Front. Hum. Dyn. 5 , 1212188 (2023).
Rhodes-Dicker, L., Brown, N. J. & Currell, M. Unpacking intersecting complexities for WASH in challenging contexts: a review. Water Res. 209 , 117909 (2022).
Macura, B. et al. Systematic mapping of gender equality and social inclusion in WASH interventions: knowledge clusters and gaps. BMJ Globa. Health 8 , e010850 (2023).
Frieden, T. R. A framework for public health action: the health impact pyramid. Am. J. Public Health 100 , 590–595 (2010).
Levy, K. Invited perspective: environmental health interventions are only as good as their adoption. Environ. Health Perspect. 131 , 011303 (2023).
Turning Promises into Action: Gender Equality in the 2030 Agenda for Sustainable Development (UN Women, 2018).
Haque, S. S. & Freeman, M. C. The applications of implementation science in water, sanitation, and hygiene (WASH) research and practice. Environ. Health Perspect. 129 , 065002 (2021).
Pandya, M. & Desai, C. Compensation in clinical research: the debate continues. Perspect. Clin. Res. 4 , 70–74 (2013).
Caruso, B. A. et al. Systematic re-review of WASH trials to assess women’s engagement in intervention delivery and research activities. Figshare https://doi.org/10.6084/m9.figshare.25786638 (2024).
Download references
Acknowledgements
This work was supported, in whole or in part, by the Bill & Melinda Gates Foundation (INV-028835 to B.A.C. and S.S.S.). Under the grant conditions of the Foundation, a Creative Commons Attribution 4.0 Generic License has already been assigned to the author accepted manuscript version that might arise from this submission. We thank R. Das, Emory University, for support.
Author information
Authors and affiliations.
Hubert Department of Global Health, Rollins School of Public Health, Emory University, Atlanta, GA, USA
Bethany A. Caruso, Madeleine Patrick, Janice Dsouza & Sheela S. Sinharoy
Gangarosa Department of Environmental Health, Rollins School of Public Health, Emory University, Atlanta, GA, USA
April M. Ballard & Julia Sobolik
Department of Disease Control, Faculty of Infectious Tropical Disease, London School of Hygiene and Tropical Medicine, London, UK
Oliver Cumming
Department of Environment, Climate Change and Health, World Health Organization, Geneva, Switzerland
Jennyfer Wolf
Energy and Resources Group, University of California, Berkeley, CA, USA
You can also search for this author in PubMed Google Scholar
Contributions
We use the Contributor Roles Taxonomy (CRediT) to indicate contributions of each author. B.A.C.: conceptualization, methodology, writing—original draft, writing—review and editing, visualization, supervision, project administration and funding acquisition. A.M.B.: methodology, investigation, formal analysis, data curation, validation, writing—review and editing. J.S.: investigation and writing—review and editing. M.P.: investigation and writing—review and editing. J.D.: investigation and writing—review and editing. S.S.S.: writing—review and editing. O.C.: methodology and writing—review and editing. J.W.: methodology, resources and writing—review and editing. I.R.: writing—original draft and writing—review and editing.
Corresponding author
Correspondence to Bethany A. Caruso .
Ethics declarations
Competing interests.
B.A.C. and S.S.S. report having received funding from the Bill & Melinda Gates Foundation, UNICEF, the U.S. Agency for International Development (USAID) and World Vision for research related to gender and WASH. O.C. has received funding from the Bill & Melinda Gates Foundation related to gender and sanitation. The remaining authors declare no competing interests.
Peer review
Peer review information.
Nature Water thanks Jess MacArthur and Biljana Macura for their contribution to the peer review of this work.
Additional information
Publisher’s note Springer Nature remains neutral with regard to jurisdictional claims in published maps and institutional affiliations.
Supplementary information
Supplementary information..
Supplementary Fig. 1, Tables 1–4, Text 1 and References.
Rights and permissions
Open Access This article is licensed under a Creative Commons Attribution 4.0 International License, which permits use, sharing, adaptation, distribution and reproduction in any medium or format, as long as you give appropriate credit to the original author(s) and the source, provide a link to the Creative Commons licence, and indicate if changes were made. The images or other third party material in this article are included in the article’s Creative Commons licence, unless indicated otherwise in a credit line to the material. If material is not included in the article’s Creative Commons licence and your intended use is not permitted by statutory regulation or exceeds the permitted use, you will need to obtain permission directly from the copyright holder. To view a copy of this licence, visit http://creativecommons.org/licenses/by/4.0/ .
Reprints and permissions
About this article
Cite this article.
Caruso, B.A., Ballard, A.M., Sobolik, J. et al. Systematic re-review of WASH trials to assess women’s engagement in intervention delivery and research activities. Nat Water (2024). https://doi.org/10.1038/s44221-024-00299-2
Download citation
Received : 10 November 2023
Accepted : 05 August 2024
Published : 06 September 2024
DOI : https://doi.org/10.1038/s44221-024-00299-2
Share this article
Anyone you share the following link with will be able to read this content:
Sorry, a shareable link is not currently available for this article.
Provided by the Springer Nature SharedIt content-sharing initiative
Quick links
- Explore articles by subject
- Guide to authors
- Editorial policies
Sign up for the Nature Briefing: Anthropocene newsletter — what matters in anthropocene research, free to your inbox weekly.

Information
- Author Services
Initiatives
You are accessing a machine-readable page. In order to be human-readable, please install an RSS reader.
All articles published by MDPI are made immediately available worldwide under an open access license. No special permission is required to reuse all or part of the article published by MDPI, including figures and tables. For articles published under an open access Creative Common CC BY license, any part of the article may be reused without permission provided that the original article is clearly cited. For more information, please refer to https://www.mdpi.com/openaccess .
Feature papers represent the most advanced research with significant potential for high impact in the field. A Feature Paper should be a substantial original Article that involves several techniques or approaches, provides an outlook for future research directions and describes possible research applications.
Feature papers are submitted upon individual invitation or recommendation by the scientific editors and must receive positive feedback from the reviewers.
Editor’s Choice articles are based on recommendations by the scientific editors of MDPI journals from around the world. Editors select a small number of articles recently published in the journal that they believe will be particularly interesting to readers, or important in the respective research area. The aim is to provide a snapshot of some of the most exciting work published in the various research areas of the journal.
Original Submission Date Received: .
- Active Journals
- Find a Journal
- Proceedings Series
- For Authors
- For Reviewers
- For Editors
- For Librarians
- For Publishers
- For Societies
- For Conference Organizers
- Open Access Policy
- Institutional Open Access Program
- Special Issues Guidelines
- Editorial Process
- Research and Publication Ethics
- Article Processing Charges
- Testimonials
- Preprints.org
- SciProfiles
- Encyclopedia
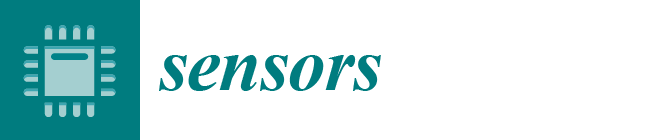
Article Menu

- Subscribe SciFeed
- Google Scholar
- on Google Scholar
- Table of Contents
Find support for a specific problem in the support section of our website.
Please let us know what you think of our products and services.
Visit our dedicated information section to learn more about MDPI.
JSmol Viewer
Using wearable sensors to study musical experience: a systematic review.
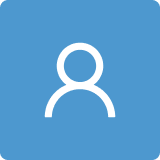
1. Introduction
2. prisma review, 3.1. performance-oriented systems, 3.2. measuring physiological parameters, 3.3. gesture recognition/classification, 3.3.1. performance, 3.3.2. education, 3.4. sensory translation/mapping, 4. discussion, 5. limitations of the reviewed studies, 6. future directions, 7. conclusions, author contributions, institutional review board statement, informed consent statement, data availability statement, conflicts of interest.
- Sudip, S. Electronic Musical Instruments Market Outlook (2022–2032). 2022. Available online: https://www.futuremarketinsights.com/reports/electronic-musical-instruments-market#:~:text=Electronic%20Musical%20Instruments%20Market%20Outlook,period%20(2022%20to%202032) (accessed on 18 November 2023).
- Llamas, R. Enterprise Wearable Market: Information By Technology (IoT, Bluetooth, BLE), Product (Wristwear, Footwear, Eyewear), Application (Infotainment, IT), and Regional Outlook–Forecast Till 2026 ; Technical Report SR1195; Straits Research: Pune, India, 2019. [ Google Scholar ]
- Wexler, D.; Yip, J.; Lee, K.P.; Li, X.; Wong, Y.H. A Touch on Musical Innovation: Exploring Wearables and Their Impact on New Interfaces for Musical Expression. Sensors 2024 , 24 , 250. [ Google Scholar ] [ CrossRef ] [ PubMed ]
- Ometov, A.; Shubina, V.; Klus, L.; Skibińska, J.; Saafi, S.; Pascacio, P.; Flueratoru, L.; Gaibor, D.Q.; Chukhno, N.; Chukhno, O.; et al. A Survey on Wearable Technology: History, State-of-the-Art and Current Challenges. Comput. Netw. 2021 , 193 , 108074. [ Google Scholar ] [ CrossRef ]
- Cavdir, D.; Wang, G. Designing felt experiences with movement-based, wearable musical instruments: From inclusive practices toward participatory design. Wearable Technol. 2022 , 3 , e19. [ Google Scholar ] [ CrossRef ] [ PubMed ]
- Freire, S.; Santos, G.; Armondes, A.; Meneses, E.A.; Wanderley, M.M. Evaluation of inertial sensor data by a comparison with optical motion capture data of guitar strumming gestures. Sensors 2020 , 20 , 5722. [ Google Scholar ] [ CrossRef ] [ PubMed ]
- Collimore, A.N.; Roto Cataldo, A.V.; Aiello, A.J.; Sloutsky, R.; Hutchinson, K.J.; Harris, B.; Ellis, T.; Awad, L.N. Autonomous control of music to retrain walking after stroke. Neurorehabilit. Neural Repair 2023 , 37 , 255–265. [ Google Scholar ] [ CrossRef ]
- Pitale, J.T.; Bolte, J.H. A heel-strike real-time auditory feedback device to promote motor learning in children who have cerebral palsy: A pilot study to test device accuracy and feasibility to use a music and dance-based learning paradigm. Pilot Feasibility Stud. 2018 , 4 , 42. [ Google Scholar ] [ CrossRef ]
- Akinloye, F.O.; Obe, O.; Boyinbode, O. Development of an affective-based e-healthcare system for autistic children. Sci. Afr. 2020 , 9 , e00514. [ Google Scholar ] [ CrossRef ]
- Wang, H. Research on the application of wireless wearable sensing devices in interactive music. J. Sens. 2021 , 2021 , 7608867. [ Google Scholar ] [ CrossRef ]
- Li, L.; Wang, G. Design and application of interactive music equipment based on wireless wearable sensors. Sci. Program. 2022 , 2022 , 4719884. [ Google Scholar ] [ CrossRef ]
- Murray-Browne, T.; Mainstone, D.; Bryan-Kinns, N.; Plumbley, M.D. The Serendiptichord: Reflections on the collaborative design process between artist and researcher. Leonardo 2013 , 46 , 86–87. [ Google Scholar ] [ CrossRef ]
- Pras, A.; Rodrigues, M.G.; Grupp, V.; Wanderley, M.M. Connecting Free Improvisation Performance and Drumming Gestures Through Digital Wearables. Front. Psychol. 2021 , 12 , 576810. [ Google Scholar ] [ CrossRef ] [ PubMed ]
- van Fenema, E.M.; Gal, P.; van de Griend, M.V.; Jacobs, G.E.; Cohen, A.F. A pilot study evaluating the physiological parameters of performance-induced stress in undergraduate music students. Digit. Biomarkers 2018 , 1 , 118–125. [ Google Scholar ] [ CrossRef ] [ PubMed ]
- Sebastiani, L.; Mastorci, F.; Magrini, M.; Paradisi, P.; Pingitore, A. Synchronization between music dynamics and heart rhythm is modulated by the musician’s emotional involvement: A single case study. Front. Psychol. 2022 , 13 , 908488. [ Google Scholar ] [ CrossRef ]
- Kim, H.G.; Lee, G.Y.; Kim, M.S. Dual-function integrated emotion-based music classification system using features from physiological signals. IEEE Trans. Consum. Electron. 2021 , 67 , 341–349. [ Google Scholar ] [ CrossRef ]
- Maragliulo, S.; Lopes, P.F.A.; Osorio, L.B.; De Almeida, A.T.; Tavakoli, M. Foot gesture recognition through dual channel wearable EMG system. IEEE Sens. J. 2019 , 19 , 10187–10197. [ Google Scholar ] [ CrossRef ]
- Rhodes, C.; Allmendinger, R.; Climent, R. New interfaces and approaches to machine learning when classifying gestures within music. Entropy 2020 , 22 , 1384. [ Google Scholar ] [ CrossRef ]
- Moher, D.; Liberati, A.; Tetzlaff, J.; Altman, D.G.; The PRISM Group. Linee guida per il reporting di revisioni sistematiche e meta-analisi: Il PRISMA Statement. PLoS Med. 2009 , 6 , e1000097. [ Google Scholar ] [ CrossRef ]
- Van Der Linden, J.; Schoonderwaldt, E.; Bird, J.; Johnson, R. Musicjacket—Combining motion capture and vibrotactile feedback to teach violin bowing. IEEE Trans. Instrum. Meas. 2010 , 60 , 104–113. [ Google Scholar ] [ CrossRef ]
- Kusserow, M.; Candia, V.; Amft, O.; Hildebrandt, H.; Folkers, G.; Tröster, G. Monitoring stage fright outside the laboratory: An example in a professional musician using wearable sensors. Med. Probl. Perform. Artist. 2012 , 27 , 21–30. [ Google Scholar ] [ CrossRef ]
- Nakra, T.M.; BuSha, B.F. Synchronous sympathy at the symphony: Conductor and audience accord. Music. Perception Interdiscip. J. 2014 , 32 , 109–124. [ Google Scholar ] [ CrossRef ]
- Turchet, L.; Barthet, M. Co-design of Musical Haptic Wearables for electronic music performer’s communication. IEEE Trans. Hum.-Mach. Syst. 2018 , 49 , 183–193. [ Google Scholar ] [ CrossRef ]
- Dalmazzo, D.; Ramírez, R. Bowing gestures classification in violin performance: A machine learning approach. Front. Psychol. 2019 , 10 , 344. [ Google Scholar ] [ CrossRef ] [ PubMed ]
- Provenzale, C.; Di Stefano, N.; Noccaro, A.; Taffoni, F. Assessing the bowing technique in violin beginners using MIMU and optical proximity sensors: A feasibility study. Sensors 2021 , 21 , 5817. [ Google Scholar ] [ CrossRef ]
- Haynes, A.; Lawry, J.; Kent, C.; Rossiter, J. FeelMusic: Enriching our emotive experience of music through audio-tactile mappings. Multimodal Technol. Interact. 2021 , 5 , 29. [ Google Scholar ] [ CrossRef ]
- Turchet, L.; West, T.; Wanderley, M.M. Touching the audience: Musical haptic wearables for augmented and participatory live music performances. Pers. Ubiquitous Comput. 2021 , 25 , 749–769. [ Google Scholar ] [ CrossRef ]
- Muhammed, Z.; Karunakaran, N.; Bhat, P.P.; Arya, A. Ensemble of Multimodal Deep Learning Models for Violin Bowing Techniques Classification. J. Adv. Inf. Technol. 2024 , 15 , 40–48. [ Google Scholar ] [ CrossRef ]
- Li, X.; Shi, Y.; Pan, D. Wearing sensor data integration for promoting the performance skills of music in IoT. Internet Technol. Lett. 2024 , 7 , e517. [ Google Scholar ] [ CrossRef ]
- Provenzale, C.; Di Tommaso, F.; Di Stefano, N.; Formica, D.; Taffoni, F. Real-Time Visual Feedback Based on MIMUs Technology Reduces Bowing Errors in Beginner Violin Students. Sensors 2024 , 24 , 3961. [ Google Scholar ] [ CrossRef ]
- Spence, C.; Di Stefano, N. Sensory translation between audition and vision. Psychon. Bull. Rev. 2024 , 31 , 599–626. [ Google Scholar ] [ CrossRef ]
- Nijs, L.; Leman, M. Interactive technologies in the instrumental music classroom: A longitudinal study with the Music Paint Machine. Comput. Educ. 2014 , 73 , 40–59. [ Google Scholar ] [ CrossRef ]
- Nown, T.H.; Upadhyay, P.; Kerr, A.; Andonovic, I.; Tachtatzis, C.; Grealy, M.A. A mapping review of real-time movement sonification systems for movement rehabilitation. IEEE Rev. Biomed. Eng. 2022 , 16 , 672–686. [ Google Scholar ] [ CrossRef ] [ PubMed ]
- Guerra, J.; Smith, L.; Vicinanza, D.; Stubbs, B.; Veronese, N.; Williams, G. The use of sonification for physiotherapy in human movement tasks: A scoping review. Sci. Sport. 2020 , 35 , 119–129. [ Google Scholar ] [ CrossRef ]
- Schaffert, N.; Janzen, T.B.; Mattes, K.; Thaut, M.H. A review on the relationship between sound and movement in sports and rehabilitation. Front. Psychol. 2019 , 10 , 244. [ Google Scholar ] [ CrossRef ] [ PubMed ]
- Choi, Y.; Jeon, J.; Lee, C.; Noh, Y.G.; Hong, J.H. A Way for Deaf and Hard of Hearing People to Enjoy Music by Exploring and Customizing Cross-modal Music Concepts. In Proceedings of the CHI Conference on Human Factors in Computing Systems, Honolulu, HI, USA, 11–16 May 2024; pp. 1–17. [ Google Scholar ]
- Petry, B.; Illandara, T.; Elvitigala, D.S.; Nanayakkara, S. Supporting rhythm activities of deaf children using music-sensory-substitution systems. In Proceedings of the 2018 CHI Conference on Human Factors in Computing Systems, Montreal, QC, Canada, 21–26 April 2018; pp. 1–10. [ Google Scholar ]
- Jadán, J.; Zapata, M.; Remache, P. Sensory Substitution in Music: Enhancing Deaf Perception Through Vibrotactile Feedback. In Proceedings of the International Congress on Information and Communication Technology, London, UK, 19–22 February 2024; pp. 19–29. [ Google Scholar ]
Click here to enlarge figure
N. | Reference | Category | |
---|---|---|---|
1 | Van Der Linden, J.; Schoonderwaldt, E.; Bird, J.; Johnson, R. Musicjacket—combining motion capture and vibrotactile feedback to teach violin bowing. IEEE Trans. Instrum. Meas. , 60, 104–113. | [ ] | A |
2 | Kusserow, M.; Candia, V.; Amft, O.; Hildebrandt, H.; Folkers, G.; Tröster, G. Monitoring stage fright outside the laboratory: An example in a professional musician using wearable sensors. Med. Probl. Perform. Artist. , 27, 21–30. | [ ] | B |
3 | Murray-Browne, T.; Mainstone, D.; Bryan-Kinns, N.; Plumbley, M.D. The Serendiptichord: Reflections on the collaborative design process between artist and researcher. Leonardo , 46, 86–87. | [ ] | C |
4 | Nakra, T.M.; BuSha, B.F. Synchronous sympathy at the symphony: Conductor and audience accord. Music. Perception Interdiscip. J. , 32, 109–124. | [ ] | B |
5 | van Fenema, E.M.; Gal, P.; van de Griend, M.V.; Jacobs, G.E.; Cohen, A.F. A pilot study evaluating the physiological parameters of performance-induced stress in undergraduate music students. Digit. Biomarkers , 1, 118–125. | [ ] | B |
6 | Turchet, L.; Barthet, M. Co-design of Musical Haptic Wearables for electronic music performer’s communication. IEEE Trans. Hum.-Mach. Syst. , 49, 183–193. | [ ] | D |
7 | Maragliulo, S.; Lopes, P.F.A.; Osorio, L.B.; De Almeida, A.T.; Tavakoli, M. Foot gesture recognition through dual channel wearable EMG system. IEEE Sensors J. , 19, 10187–10197. | [ ] | A |
8 | Dalmazzo, D.; Ramírez, R. Bowing gestures classification in violin performance: a machine learning approach. Front. Psychol. , 10, 344. | [ ] | A |
9 | Freire, S.; Santos, G.; Armondes, A.; Meneses, E.A.; Wanderley, M.M. Evaluation of inertial sensor data by a comparison with optical motion capture data of guitar strumming gestures. Sensors , 20, 5722. | [ ] | A |
10 | Rhodes, C.; Allmendinger, R.; Climent, R. New interfaces and approaches to machine learning when classifying gestures within music. Entropy , 22, 1384. | [ ] | A |
11 | Wang, H. Research on the application of wireless wearable sensing devices in interactive music. J. Sensors , 2021, 7608867. | [ ] | C |
12 | Provenzale, C.; Di Stefano, N.; Noccaro, A.; Taffoni, F. Assessing the bowing technique in violin beginners using MIMU and optical proximity sensors: A feasibility study. Sensors , 21, 5817. | [ ] | A |
13 | Haynes, A.; Lawry, J.; Kent, C.; Rossiter, J. FeelMusic: Enriching our emotive experience of music through audio-tactile mappings. Multimodal Technol. Interact. , 5, 29. | [ ] | D |
14 | Pras, A.; Rodrigues, M.G.; Grupp, V.; Wanderley, M.M. Connecting Free Improvisation Performance and Drumming Gestures Through Digital Wearables. Front. Psychol. , 12, 576810. | [ ] | C |
15 | Turchet, L.; West, T.; Wanderley, M.M. Touching the audience: musical haptic wearables for augmented and participatory live music performances. Pers. Ubiquitous Comput. , 25, 749–769. | [ ] | D |
16 | Kim, H.G.; Lee, G.Y.; Kim, M.S. Dual-function integrated emotion-based music classification system using features from physiological signals. IEEE Trans. Consum. Electron. , 67, 341–349. | [ ] | B |
17 | Sebastiani, L.; Mastorci, F.; Magrini, M.; Paradisi, P.; Pingitore, A. Synchronization between music dynamics and heart rhythm is modulated by the musician’s emotional involvement: A single case study. Front. Psychol. , 13, 908488. | [ ] | B |
18 | Li, L.; Wang, G. Design and application of interactive music equipment based on wireless wearable sensors. Sci. Program. , 2022, 4719884. | [ ] | C |
19 | Cavdir, D.; Wang, G. Designing felt experiences with movement-based, wearable musical instruments: From inclusive practices toward participatory design. Wearable Technol. , 3, e19. | [ ] | C |
20 | Wexler, D.; Yip, J.; Lee, K.P.; Li, X.; Wong, Y.H. A Touch on Musical Innovation: Exploring Wearables and Their Impact on New Interfaces for Musical Expression. Sensors , 24, 250. | [ ] | C |
21 | Muhammed, Z.; Karunakaran, N.; Bhat, P.P.; Arya, A. Ensemble of Multimodal Deep Learning Models for Violin Bowing Techniques Classification. J. Adv. Inf. Technol. , 15, 40–48. | [ ] | A |
22 | Li, X.; Shi, Y.; Pan, D. Wearing sensor data integration for promoting the performance skills of music in IoT. Internet Technol. Lett. , 7, e517. | [ ] | A |
23 | Provenzale, C.; Di Tommaso, F.; Di Stefano, N.; Formica, D.; Taffoni, F. Real-Time Visual Feedback Based on MIMUs Technology Reduces Bowing Errors in Beginner Violin Students. Sensors , 24, 3961. | [ ] | A |
Category | Similarities and Commonalities | Examples |
---|---|---|
Real-time Monitoring and Feedback | Many wearable technologies aim to provide real-time feedback on musicians’ movements and performance. This helps musicians improve technical skills and manage performance-related challenges. | Studies by [ , ] focus on multi-sensor systems providing movement feedback, while physiological monitoring is highlighted by [ , ]. |
Inclusivity and Accessibility | Several technologies are designed to make music-making more accessible, particularly for individuals with sensory or motor impairments, using gesture recognition and adaptive instruments. | Cavdir and Wang [ ], Freire et al. [ ] focus on inclusive instruments for those with disabilities, utilizing gesture recognition technologies from [ , ]. |
Integration of Movement and Music | Wearable devices that enable musicians to use their body as an instrument, expanding the creative possibilities by translating physical movements into musical expression. | “Serendiptichord” [ , ] demonstrate how wearable devices can transform musicians’ physical movements into interactive instruments. |
Cross-disciplinary Innovation | Insights from biomechanics, computer science, and music education are integrated into the development of wearable technologies, fostering interdisciplinary research and new solutions. | Combining physiological monitoring with gesture recognition and real-time feedback is a cross-disciplinary innovation discussed in several studies, e.g., refs. [ , , , ]. |
Economic and Practical Accessibility | Advances in wearable technologies promote cost-reduction strategies and reduce the need for technical expertise, making devices more practical and accessible in educational settings. | Increased adoption could lower costs through economies of scale, simplifying calibration and technical setup. These points are echoed in several research studies, e.g., refs. [ , , ]. |
Comprehensive Music Education | The integration of real-time feedback, physiological monitoring, and inclusive technologies provides a more holistic and personalized approach to music education. | Wearable technologies offer tailored feedback that can address both technical and emotional aspects of learning, enhancing educational outcomes for a wider range of students, e.g., refs. [ , , ]. |
Technology | Usability | Cost | Reliability | Calibration Needed | Technical Staff Needed |
---|---|---|---|---|---|
Myo armband (e.g., ref. [ ]) | Easy to use, suitable for gesture recognition in music | Moderate | High | Yes | No |
EMG system (e.g., ref. [ ]) | Moderate complexity, used for foot gesture recognition | High | High | Yes | Yes |
Wearable sensors (e.g., [ ]) | Usable in performance contexts, data integration capabilities | Moderate | High | Yes | Yes |
New interfaces for performance (e.g., refs. [ , ]) | Moderate complexity, interactive dance-driven music, and music improvisation | Low to Moderate | Moderate | No/Yes | No |
Wireless sensing (e.g., ref. [ ]) | Moderate to high complexity, suitable for interactive music applications | Moderate to High | High | Yes | Yes |
Accessible instruments (e.g., ref. [ ]) | Designed for accessibility, easy to use for DHH community | Low | Moderate | No | No |
Physiological monitoring (e.g., refs. [ , , , ]) | Easy to moderate complexity for stress monitoring | Moderate | Moderate to High | Yes | Yes |
Multi-sensor platforms (e.g., ref. [ ]) | Moderate complexity, used in dance and music performances | Moderate | High | Yes | Yes |
The statements, opinions and data contained in all publications are solely those of the individual author(s) and contributor(s) and not of MDPI and/or the editor(s). MDPI and/or the editor(s) disclaim responsibility for any injury to people or property resulting from any ideas, methods, instructions or products referred to in the content. |
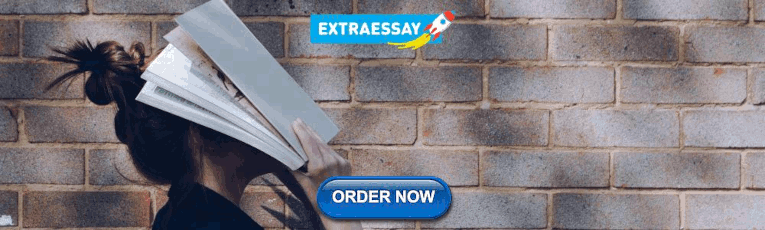
Share and Cite
Volta, E.; Di Stefano, N. Using Wearable Sensors to Study Musical Experience: A Systematic Review. Sensors 2024 , 24 , 5783. https://doi.org/10.3390/s24175783
Volta E, Di Stefano N. Using Wearable Sensors to Study Musical Experience: A Systematic Review. Sensors . 2024; 24(17):5783. https://doi.org/10.3390/s24175783
Volta, Erica, and Nicola Di Stefano. 2024. "Using Wearable Sensors to Study Musical Experience: A Systematic Review" Sensors 24, no. 17: 5783. https://doi.org/10.3390/s24175783
Article Metrics
Article access statistics, further information, mdpi initiatives, follow mdpi.
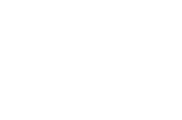
Subscribe to receive issue release notifications and newsletters from MDPI journals

Literature Reviews
What is a Literature Review?
- Steps for Creating a Literature Review
- Providing Evidence / Critical Analysis
- Challenges when writing a Literature Review
- Systematic Literature Reviews
A literature review is an academic text that surveys, synthesizes, and critically evaluates the existing literature on a specific topic. It is typically required for theses, dissertations, or long reports and serves several key purposes:
- Surveying the Literature : It involves a comprehensive search and examination of relevant academic books, journal articles, and other sources related to the chosen topic.
- Synthesizing Information : The literature review summarizes and organizes the information found in the literature, often identifying patterns, themes, and gaps in the current knowledge.
- Critical Analysis : It critically analyzes the collected information, highlighting limitations, gaps, and areas of controversy, and suggests directions for future research.
- Establishing Context : It places the current research within the broader context of the field, demonstrating how the new research builds on or diverges from previous studies.
Types of Literature Reviews
Literature reviews can take various forms, including:
- Narrative Reviews : These provide a qualitative summary of the literature and are often used to give a broad overview of a topic. They may be less structured and more subjective, focusing on synthesizing the literature to support a particular viewpoint.
- Systematic Reviews : These are more rigorous and structured, following a specific methodology to identify, evaluate, and synthesize all relevant studies on a particular question. They aim to minimize bias and provide a comprehensive summary of the existing evidence.
- Integrative Reviews : Similar to systematic reviews, but they aim to generate new knowledge by integrating findings from different studies to develop new theories or frameworks.
Importance of Literature Reviews
- Foundation for Research : They provide a solid background for new research projects, helping to justify the research question and methodology.
Identifying Gaps : Literature reviews highlight areas where knowledge is lacking, guiding future research efforts.
- Building Credibility : Demonstrating familiarity with existing research enhances the credibility of the researcher and their work.
In summary, a literature review is a critical component of academic research that helps to frame the current state of knowledge, identify gaps, and provide a basis for new research.
The research, the body of current literature, and the particular objectives should all influence the structure of a literature review. It is also critical to remember that creating a literature review is an ongoing process - as one reads and analyzes the literature, one's understanding may change, which could require rearranging the literature review.
Paré, G. and Kitsiou, S. (2017) 'Methods for Literature Reviews' , in: Lau, F. and Kuziemsky, C. (eds.) Handbook of eHealth evaluation: an evidence-based approach . Victoria (BC): University of Victoria.
Perplexity AI (2024) Perplexity AI response to Kathy Neville, 31 July.
Royal Literary Fund (2024) The structure of a literature review. Available at: https://www.rlf.org.uk/resources/the-structure-of-a-literature-review/ (Accessed: 23 July 2024).
Library Services for Undergraduate Research (2024) Literature review: a definition . Available at: https://libguides.wustl.edu/our?p=302677 (Accessed: 31 July 2024).
Further Reading:
Methods for Literature Reviews
Literature Review (The University of Edinburgh)
Literature Reviews (University of Sheffield)
- How to Write a Literature Review Paper? Wee, Bert Van ; Banister, David ISBN: 0144-1647
- Next: Steps for Creating a Literature Review >>
- Last Updated: Sep 4, 2024 11:43 AM
- URL: https://library.lsbu.ac.uk/literaturereviews
Artificial intelligence for the study of human ageing: a systematic literature review
- Open access
- Published: 06 September 2024
Cite this article
You have full access to this open access article
- Mary Carlota Bernal ORCID: orcid.org/0000-0001-7565-2909 1 , 2 ,
- Edgar Batista ORCID: orcid.org/0000-0002-8046-6016 1 ,
- Antoni Martínez-Ballesté ORCID: orcid.org/0000-0002-1787-7410 1 &
- Agusti Solanas ORCID: orcid.org/0000-0002-4881-6215 1
As society experiences accelerated ageing, understanding the complex biological processes of human ageing, which are affected by a large number of variables and factors, becomes increasingly crucial. Artificial intelligence (AI) presents a promising avenue for ageing research, offering the ability to detect patterns, make accurate predictions, and extract valuable insights from large volumes of complex, heterogeneous data. As ageing research increasingly leverages AI techniques, we present a timely systematic literature review to explore the current state-of-the-art in this field following a rigorous and transparent review methodology. As a result, a total of 77 articles have been identified, summarised, and categorised based on their characteristics. AI techniques, such as machine learning and deep learning, have been extensively used to analyse diverse datasets, comprising imaging, genetic, behavioural, and contextual data. Findings showcase the potential of AI in predicting age-related outcomes, developing ageing biomarkers, and determining factors associated with healthy ageing. However, challenges related to data quality, interpretability of AI models, and privacy and ethical considerations have also been identified. Despite the advancements, novel approaches suggest that there is still room for improvement to provide personalised AI-driven healthcare services and promote active ageing initiatives with the ultimate goal of enhancing the quality of life and well-being of older adults.
Graphical abstract
Overview of the literature review.
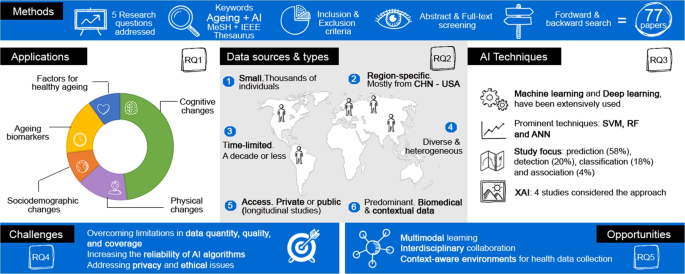
Explore related subjects
- Artificial Intelligence
Avoid common mistakes on your manuscript.
1 Introduction
Recent health and socio-economic developments are leading to an ageing society, characterised by the growth of the world’s population and the increase in average life expectancy. Consequently, the number of persons aged 65 or over is estimated to double by 2050, reaching 1.6 billion globally [ 1 ]. This demographic shift is unsurprisingly forcing global institutions to prioritise initiatives promoting active ageing, such as the Sustainable Development Goals [ 2 ] and the Decade of Healthy Ageing (2021-2030) [ 3 ]. These initiatives aim to establish frameworks towards healthy lifestyles and overall well-being among the elderly cohort.
Understanding ageing is far from straightforward due to its multidisciplinary nature, encompassing changes in various aspects of human life, including physical, metabolic, psychological, social, emotional, and functional. Recently, the World Health Organisation proposed an innovative model aimed at reshaping clinical practices in elderly healthcare, shifting the focus from a disease-centred approach to one centred around function [ 4 ]. Within this framework, the concept of functional ability [ 3 ] emerges to refer to people’s abilities (physical, cognitive, social...) to perform their daily living activities according to their basic needs. The functional ability of an individual is determined by three main factors: (i) their intrinsic capacity (i.e., physical and mental capacities, namely locomotor, sensory, vitality, cognition, and psychological), (ii) the environment in which they live (i.e., context), and (iii) how they interact with that environment. This approach offers a new perspective in ageing focusing not on disease but on a holistic understanding that embraces the positive aspects of ageing.
As the ageing of society is an unavoidable reality, healthcare providers must prepare to meet the increasing demand for clinical and public health services, especially in primary care and long-term care [ 5 ]. Current healthcare paradigms, such as smart health [ 6 ], leveraging technological advances to deliver patient-centred care services tailored to the needs of older adults [ 7 , 8 , 9 , 10 ]. Notably, the potential of these paradigms is expected to grow exponentially with the development of sensors at nanoscopic scales and the deployment of ultra-fast data networks with extremely low latency [ 11 , 12 ]. This advancement enables the collection, transmission, and analysis of vast amounts of heterogeneous data, paving the way for exciting health opportunities, including gaining a comprehensive understanding of the ageing processes, ranging from personalised insights at an intra-body level to broader, cross-national perspectives.
The increasing demand for data-driven solutions in healthcare has propelled the widespread adoption of artificial intelligence (AI) [ 13 ], which encompasses a broad range of capabilities from sensory perception to autonomous decision-making. AI systems outperform humans in identifying relevant patterns within large sets of complex, non-linear data through machine learning (ML) and deep learning (DL) [ 14 , 15 ]. ML, a subset of AI, is the most common method for implementing AI, automating data analysis using algorithms that iteratively recognise patterns and learn from them. In turn, DL is a subset of ML, employing deep neural networks that operate on an unsupervised ML approach, which does not require labelled data. Studies on human ageing, which are evidence-based, require extensive datasets, preferably longitudinal studies that are more likely to capture declines in intrinsic capacity domains [ 16 ]. Hence, contemporary research on ageing leverages advanced AI techniques to develop sophisticated models capable of understanding and predicting ageing processes, assessing factors contributing to age-related diseases, developing biomarkers, and discovering potential anti-ageing treatments, among others. Notwithstanding, AI is not a silver bullet solution. AI-generated models may exhibit biases if trained on inaccurate, incomplete, or discriminatory datasets. Since data on elderly population is paramount in research on ageing, these issues could be exacerbated by digital ageism, and potentially resulting in ineffective AI models [ 17 ]. All in all, there is no doubt that future developments of AI will unleash a new wave of technological research and innovation in the field of ageing research.
As the fields of AI and human ageing are getting increasingly intertwined, stakeholders and key players interested in cutting-edge applications in this arena require a timely systematic literature review of the existing research. In contrast to existing reviews, which do not follow a systematic methodology or are domain-specific, our study provides a comprehensive understanding of the role of AI in ageing research by offering an overview of the current landscape of AI-enabled applications, as well as the most prominent datasets and algorithms used. Through a content analysis approach, we highlight the growing interest from the academic community and identify three key research streams: (i) classification and summary of AI-based applications, techniques, and datasets across ageing research, (ii) evaluation of the suitability of AI technology to create value in this field while considering its inherent limitations, and (iii) guidance for researchers and stakeholders by outlining promising research directions, challenges, and opportunities that require further research.
The remainder of the article is organised as follows. Section 2 outlines the methodology followed to conduct the systematic review. The descriptive analysis of the retrieved literature is presented in Section 3 . Then, Section 4 provides an in-depth discussion on the advancement of AI in ageing research, focusing on key challenges and sketching research opportunities that are expected to be relevant in the years ahead. Finally, Section 5 closes the article with our conclusions.
To provide a rigorous and transparent review, we have adopted the well-known methodology for systematic reviews suggested by Brocke et al. [ 18 ] along with adhering to the guidelines of the PRISMA statement [ 19 ]. Specifically, our review methodology encompasses the following phases: (i) the definition of the review scope, (ii) the contextualisation of the topic, (iii) the literature search, (iv) the literature analysis and synthesis, and (v) the definition of a research agenda.
2.1 Definition of the review scope
The scope of this review is to provide a timely analysis of the current state-of-the-art about the role of AI in the study of human ageing. To this end, we examine and classify the main goals, techniques, and applications proposed by the scientific community. For the sake of completeness, to align the systematic review to specific objectives, five research questions (RQs) have been defined (see Table 1 ).
The target audience of this review is multi-disciplinary because it is intended for researchers, practitioners, and stakeholders in the fields of AI, human ageing, longevity, and biotechnology. To smoothly guide the reader through the topic, the review is organised conceptually by grouping similar ideas from different authors, and the articles from the literature are examined from a neutral but critical viewpoint.
2.2 Conceptualisation of the topic
This review focuses on two main topics: AI and ageing. Both terms are conceptually broad, so it is likely that they are often referenced using a variety of synonyms and related words. For the sake of completeness, additional relevant terms have been included in the search string.
Related terms associated with “artificial intelligence” were identified using the IEEE Thesaurus [ 20 ], which categorises concepts in engineering and computer science. This tool offers related terminology and vocabulary from high-quality scientific sources, including journal articles and standards. Thus, it is considered a suitable tool for obtaining key terms relevant to this subject of study. Numerous terms were retrieved and organised based on their semantic relationships:
As broader terms, the following results were obtained: Computational and artificial intelligence.
As related terms, the following results were obtained: Autonomous automobiles; Autonomous vehicles; Computational Intelligence; Data Mining; Feedforward Neural Networks; Generative Adversarial Networks; Independent Component Analysis; Machine Ethics; Minimax techniques; Natural languages; Neural Networks; Neurocontrollers; Pervasive computing; Posthuman; Prediction theory; Radial Basis Function networks; Robot learning; Semantic Web; Software agents; Support Vector Machines; Synapses.
As narrower terms, the following results were obtained: Artificial intelligence accelerators; Affective computing; Autonomous robots; Bio-inspired computing; Cognitive systems; Context awareness; Cooperative systems; Decision support systems; Intelligent systems; Knowledge based systems; Knowledge engineering; Learning (artificial intelligence); Learning systems; Machine learning; Prediction methods; Virtual artifact.
Related terms associated with “ageing” were identified using the MeSH Thesaurus [ 21 ], a comprehensive and controlled vocabulary related to the life sciences curated by the United States National Library of Medicine. This resources indexes and catalogues biomedical and health-related information. As a result, the following terms were obtained: Aging; Senescence; Biological aging; Aging, biological.
2.3 Literature search
To guarantee the coverage of high-quality scientific literature in this review, we selected the Web of Science database for retrieving publications. The search string was built by combining the two aforementioned terms sets: one containing the list of words related to “artificial intelligence”, and the other one containing the list of words related to “ageing”. Moreover, to obtain the broadest possible coverage on the topic, no timeframe restriction was applied, and the search string was configured to seek across all the article (i.e., title, abstract, keywords, full-text, and metadata). Hence, the resulting search string used to retrieve the literature was as follows:
ALL ((“Artificial Intelligence” OR “Computational and artificial intelligence” OR “Autonomous automobiles” OR “Autonomous vehicles” OR “Computational intelligence” OR “Data Mining” OR “Feedforward neural networks” OR “Generative Adversarial Networks” OR “Independent Component Analysis” OR “Machine Ethics” OR “Minimax techniques” OR “Natural languages” OR “Neural Networks” OR “Neurocontrollers” OR “Pervasive computing” OR “Posthuman” OR “Prediction theory” OR “Radial Basis Function networks” OR “Robot learning” OR “Semantic Web” OR “Software agents” OR “Support Vector Machines” OR “Synapses” OR “Artificial intelligence accelerators” OR “Affective computing” OR “Autonomous robots” OR “Bio-inspired computing” OR “Cognitive systems” OR “Context awareness” OR “Cooperative systems” OR “Decision support systems” OR “Intelligent systems” OR “Knowledge based systems” OR “Knowledge engineering” OR “Learning and Artificial Intelligence” OR “Learning systems” OR “Machine learning” OR “Prediction methods” OR “Virtual artifact”) AND (“ageing” OR “aging” OR “senescence” OR “biological aging” OR “ageing, biological”))
The eligibility of the retrieved literature was evaluated independently by the authors based on a set of predefined inclusion and exclusion criteria, described as follows:
The publication was a peer-reviewed research article.
The publication was written in English.
The full-text of the publication was available.
The publication was published in a Q1 ISI-JCR journal, or the number of citation of the publication was above the 75 th percentile regarding the citations of other retrieved publications of the same year.
The publication provided explicit evidence of the use of AI in the study of human ageing. Thus, publications using AI to study age-related diseases were excluded if they did not specifically address research on human ageing.
Thus publication explicitly specify the AI technique used, i.e., stating that it uses AI was not sufficient.
The publication was focused on older adults and the elderly to provide a more comprehensive understanding of the ageing experience within these cohorts.
The selection process consisted of two screening phases: abstract screening and full-text screening. Each publication was independently evaluated by two reviewers according to the inclusion criteria. During each phase, each reviewer categorised the publications as either accepted or rejected. Any discrepancies were discussed among the reviewers until a consensus was reached, thus minimising potential reviewer bias. After this initial search, the first selection of publications was accepted for inclusion in this review.
From the initial set of selected publications, a reference-checking phase was conducted to discover additional studies. Concretely, two new searches were conducted: (i) a backward search (i.e., reviewing older literature cited in the selected publications) and (ii) a forward search (i.e., reviewing literature that have cited the selected publications). Each search yielded a new set of publications, which were assessed for eligibility again following the same procedure as in the first selection. This iterative approach ensures a more thorough literature search and adds robustness to this systematic literature review. As a result, a new set of publications was accepted for inclusion in this review as well.
2.4 Literature analysis and synthesis
After selecting the publications to be included in this review, accepted publications were rigorously analysed to extract, characterise, and classify the most relevant information. Then, a conceptual synthesis was adopted to identify common topics and characteristics in the publications. For this reason, the results are discussed from multiple angles, such as the ageing-related application, the kind of data and ageing studies needed, and the AI techniques that have been employed.
2.5 Definition of a research agenda
Beyond providing an exhaustive review of the current state-of-the-art in the field, this review helps set the ground for further research on this topic. Hence, Section 4.3 summarises potential research directions based on our main findings.
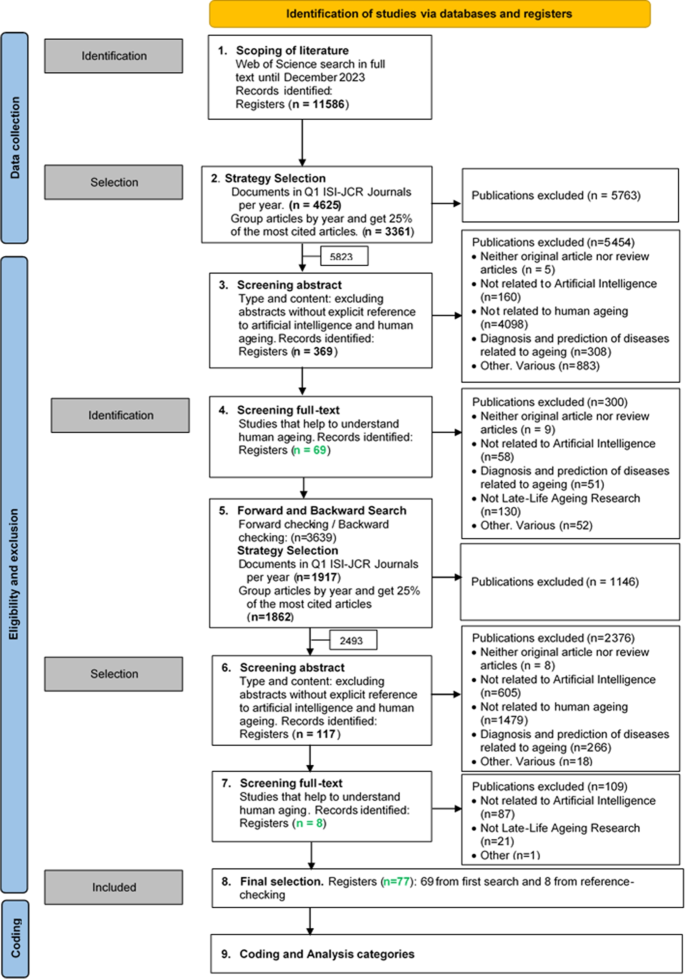
PRISMA flowchart of the systematic review
A total of 11,586 publications were retrieved from the first search, queried on 30 th December 2023. Several publications were excluded at first as they did not fulfil some inclusion criteria. Then, 5,823 publications were assessed for eligibility in the abstract screening phase. After that, 5,763 were excluded, mainly because they were not related to human ageing. Hence, the full-text of 369 publications were screened and assessed for eligibility. After full-text screening, 300 were rejected, and 69 publications were accepted to be included in this review. From those 69 accepted publications in the first search, the reference-checking phase, conducted on 29 th January 2024, yielded 3,639 articles. After removing duplicated records, already-screened records, and applying objective inclusion criteria, 2,493 publications remained. After abstract screening, 2,376 publications were excluded, and 117 were assessed for eligibility. From those, 8 publications met all the inclusion criteria and were accepted to be included in the review. Therefore, a total of 77 publications are considered in this review. Details of the review methodology are depicted in Fig. 1 .
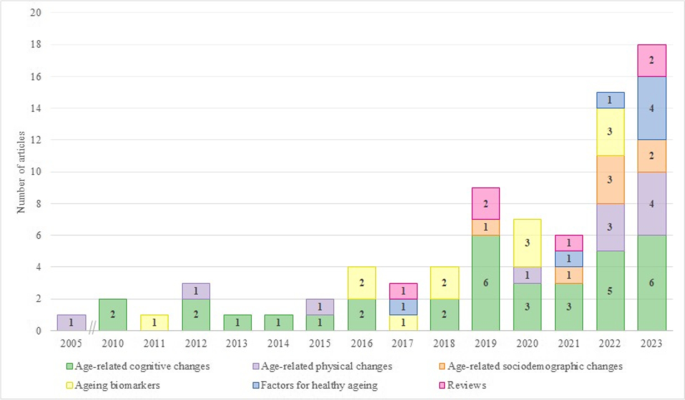
Evolution of the number of articles per application domain
A descriptive analysis of the 77 articles reveals a growing interest of the research community in incorporating AI into ageing studies. While first studies date back to the mid-2000s, when AI was gaining attention, over half of the selected articles (55/77) have been published within the past five years. This trend demonstrates the need for a timely systematic review in this field. Moreover, the majority of these articles have been published in journals within the health and life sciences domains, where Frontiers in Aging Neuroscience, NeuroImage, Human Brain Mapping, and GeroScience stand out.
All 77 articles were thoroughly analysed to extract their main characteristics in terms of scope, used data, and implemented AI techniques (refer to Table 2 for details). The gathered information was used to conceptualise and establish robust classification taxonomies that align with the RQs of this review.
3.1 Taxonomy of AI-based ageing applications
Most authors present AI opportunities within specific application domains, such as frailty [ 57 ] and biomarkers [ 98 ]. In our study, we provide a generic application-oriented classification similar to others proposed in similar research [ 36 , 97 ]. Our approach, however, differs in its utilisation of a rigorous review methodology, thus providing a more suitable framework to illustrate current AI developments and future trends in ageing research. Consequently, considering the actual and forthcoming diversity of AI-driven solutions, we present a more comprehensive and in-depth classification of AI-enabled applications for ageing (RQ1).
As outlined in Table 2 , articles have been classified into six main application domains: (i) age-related cognitive changes (34 articles), (ii) age-related physical changes (11 articles), (iii) age-related sociodemographic changes (7 articles), (iv) ageing biomarkers (12 articles), (v) factors for healthy ageing (7 articles), and (vi) review articles (6 articles).
Figure 2 illustrates the evolution of the number of articles per application domain. We observe that earlier studies leveraged AI to understand ageing processes according to the individuals’ physical and cognitive changes. Since the mid-2010s, the exploitation of biomarkers has been used to predict the biological age of individuals. This growing interest may be due to the incursion of DL techniques to develop age predictors that offer new possibilities for formerly incompatible data types. Likewise, it is worth noting that, in the last three years, there has been a growing interest in identifying sociodemographic changes and factors for healthy ageing.
3.1.1 Age-related cognitive changes
AI has been extensively employed to explore and analyse the anatomical and functional properties of the brain, with the aim of comprehending the mechanisms underlying age-related cognitive changes. Early studies (2010–2014) laid the foundation for understanding how alterations in brain anatomy affect cognitive function. Typically, anatomical neuroimaging techniques have been applied to identify structural brain regions affected by ageing. During the latter half of the 2010s, research focused on comprehensively analysing the functional connectivity between the brain and age-related changes, as well as detecting and predicting cognitive impairments such as dementia, Alzheimer’s disease, and Parkinson’s disease. As research progressed towards predicting cognitive decline, this structural knowledge was used to identify risk factors influencing deterioration. The ability of AI methods to detect subtle and non-linear patterns in cognitive data facilitates the modelling of complex relationships between such cognitive variables and potentially influencing factors, such as genetics, habits, and environment. Recently, the integration of advanced structural data with predictive modelling techniques using multi-modal and multi-dimensional analyses has led to a more holistic and precise understanding of how brain changes predetermine the risk of cognitive decline.
As ageing affects brain regions differently, understanding structural changes provides valuable insights for the development of diagnostic tools. Initial studies identified group-wise differences in the whole-brain network of macroscopic neural tracts across populations with different age distributions [ 75 ]. Also, by means of electroencephalography (EEG), Vysata et al. [ 87 ] conducted a retrospective analysis of healthy professional drivers and found a decrease in EEG coherence with age, particularly in the theta and alpha bands associated with decreased cortical connectivity. These studies allowed detailed exploration of the functional connectivity. In [ 45 ], authors investigated the effects of common cardiovascular risk factors on grey matter networks within the neocortex. Negative associations of smoking, higher blood pressure, elevated glucose levels, and visceral obesity with structural covariance networks were discovered, suggesting that reducing these factors could potentially delay late-life trajectories of grey matter ageing. The study in Salami et al. [ 77 ] revealed a functional connectivity decline within the anterior medial temporal lobe after age 60, and an elevated functional connectivity in the posterior medial temporal lobe that correlates with episodic-memory decline. Likewise, Bidelman et al. [ 26 ] focused on speech comprehension in older adults with and without hearing loss, demonstrating altered brain communication patterns in hearing-impaired individuals and highlighting the impact of even mild hearing loss on neural signalling and information processing pathways in the left hemisphere. The work in Schilling et al. [ 79 ], microstructural and macrostructural geometrical features of the brain’s connections were quantified by examining brain white matter. Their large-scale study indicated that macrostructural features were more sensitive to heterogeneous white matter decline, potentially making them more useful for studying ageing and enabling comparisons among various diseases and abnormal conditions. Using a different approach, Yamada et al. [ 94 ] evaluated changes in brain and cerebrospinal fluid volume with healthy ageing. Results showed that intracranial cerebrospinal fluid volume increased linearly because of the decreased brain volume with ageing, which could help elucidate the pathogenesis of chronic hydrocephalus in adults. Further research on brain ageing patterns, aiming to model the evolution of structural and functional brain changes, has also been conducted by Di et al. [ 34 ] and Sone et al. [ 81 ].
Regarding brain functioning, several studies shed light on the relationship between brain ageing, cognitive functions, and motor skills. For instance, brain-predicted age difference scores (i.e., the difference between an individual’s brain-predicted age using neuroimaging data and an individual’s chronological age) are used to detect accelerated ageing, often associated with increased mortality risk and poorer physical function. In [ 27 ], authors assessed the correlation between these scores and specific cognitive functions, revealing negative correlations between these scores and performance across various cognitive domains, including visual attention, processing speed, and semantic verbal fluency. Thus, the authors demonstrated correlations between increased brain-predicted age differences and reduced cognitive function in domains that are implicated in cognitive ageing. Indeed, substantial differences in linking brain-predicted age differences to cognitive measures may appear depending on the ML algorithm chosen [ 56 ]. In this line, Xiong et al. [ 92 ] evaluated the performance of different ML algorithms and image modalities for brain age prediction. Authors highlighted that image modality selection is more important than ML algorithm selection in improving the accuracy of brain age prediction. This finding has significant implications for future neuroimaging research endeavours. By means of structural covariance networks, the study in Koini et al. [ 48 ] examined the integrity of grey matter networks with age, cognitive performance, and fine motor skills, revealing positive associations between network disintegration and higher-order cognitive functions.
To understand the functional basis of cognitive changes in ageing, researchers have explored default mode (sub-)networks and recognised changes in resting state networks. Lindbergh et al. [ 59 ] observed that older adults with high intelligence maintained a more segregated default mode network profile, while Huang et al. [ 44 ] suggested that age-related network changes are pivotal in understanding cognitive ageing trajectories and, hence, offer valuable diagnostic tools for neurodegenerative diseases. Similarly, the work in Moezzi et al. [ 63 ] aimed to distinguish functional brain networks in young and older adults, revealing reduced connectivity in certain frequency bands associated with attention and consciousness during the resting state in older individuals. The use of resting state data is not new: previous studies already employed it to compare age-related differences in major functional brain networks [ 86 ], examine age-related perturbations in various resting state networks [ 68 ], and reorganise functional brain networks as predictors of age and cognitive performance [ 53 , 61 ]. Additionally, the distinctiveness of different neural representations to explain individual differences in cognitive performance among older adults was already evaluated by Park et al. [ 70 ], which suggested that neural specificity declines are correlated with cognitive decline.
Beyond ageing, accelerated cognitive deterioration has proven correlated to a number of factors according to recent studies. For instance, Ganguli et al. [ 40 ] investigated the role of obesity, diabetes, and other metabolic indices on cognitive decline among older adults. Their findings suggested that obesity and even modest degrees of hyperglycemia could independently predispose individuals to faster cognitive decline. Pereira et al. [ 71 ] found associations between cognitive decline and spatial patterns of tau decomposition. In [ 29 ], authors highlighted the predominant influence of modifiable factors, such as education and cardiovascular health, over genetic risks, in predicting Alzheimer’s disease. Sun et al. [ 82 ] observed correlations between lower pulse pressure, significant stenosis in the posterior circulatory artery, and the risk of cognitive decline. Similarly, the studies in Kimura et al. [ 47 ], Noh et al. [ 66 ] and Wang et al. [ 88 ] identified physical activity, adequate sleep duration, and social engagement as crucial factors for guaranteeing proper cognitive function in older adults. Likewise, Sone et al. [ 80 ] has shown that higher life satisfaction is associated with “younger” brains, while diabetes and alcohol consumption negatively impact life satisfaction. However, the effects of life satisfaction on cognitive function may vary considerably among individuals [ 49 ], necessitating further research to uncover the mechanisms underlying the heterogeneous effects of life satisfaction on cognitive function.
More recently, innovative technological tools have been developed to early detect cognitive decline in large population cohorts. Yamada et al. [ 93 ] provided empirical evidence of the capability of automated cognitive assessment through drawing processes by measuring and analysing several drawing-related features such as speed, speed variability, pressure variability, and pen inclination. Moreover, with the advent of context-aware environments, plenty of opportunities arise to leverage the vast amount of data collected from sensors and other IoT devices. For instance, Khodabandehloo et al. [ 46 ] proposed continuous remote monitoring of elderly people to support early detection of cognitive decline by using data collected within smart homes. The data-driven nature of this explainable AI solution enables clinicians to make more accurate diagnoses. Furthermore, the works in Na [ 65 ] and Tan et al. [ 83 ] demonstrated the ability of current AI techniques to predict cognitive impairment using easy-to-collect and non-invasive data available at community healthcare centres and nursing homes.
3.1.2 Age-related physical changes
Age-related physical changes refer to the transformations that occur within an individual’s body as they age. These transformations, generally manifesting as age-related syndromes or conditions, may be accelerated according to the individual’s quality of life and the degree of active ageing. The use of AI can help understand these transformations and pinpoint the most relevant factors to delay them.
Initial studies used ML techniques to evaluate gait decline, one of the most popular physical impairments among older adults. The work in Begg and Kamruzzaman [ 25 ] identified different gait patterns between younger individuals and older adults through the analysis of basic temporal/spatial, kinetic, and kinematic gait data. Walking differences in terms of gait velocity were later examined in Yuan et al. [ 96 ] using resting state functional MRI data. Indeed, the authors in Hicks et al. [ 43 ] observed that one-off measurements of absolute strength and muscle power can help predict mobility and gait decline. Similarly, the relationship between lower extremity strength and mobility appears to be influenced by accelerated brain ageing [ 85 ]. Physical changes have also been explored through indirect calorimetry methodologies, as evidenced by Paraschiakos et al. [ 69 ], who quantified the physical activity energy expenditure of older people using accelerometer data and physiological indicators.
Frailty, a syndrome characterised by impairments to multiple interrelated physiological systems, has been extensively explored by recent research. In [ 33 ], ML models were used to predict individuals at risk of developing frailty in middle-aged and older adults. Interestingly enough, authors provided insights to overcome the encountered imbalance data problem: far fewer individuals are classified as frail than as non-frail, which affects the performance of ML models once predicting the syndrome. By combining undersampling and oversampling techniques, age, chair-rise test, household wealth, balance problems, and self-rated health were the most important frailty predictors. Similarly, Sajeev et al. [ 76 ] identified higher body mass index and distress levels, lower dexterity, balance problems, incontinence, and poor sleep quality as key predictors of pre-frailty in adults. Beyond prediction, research interest has extended to strategies for frailty reversal. Wei et al. [ 89 ] demonstrated the feasibility of predicting the likelihood of older adults successfully reversing their frailty status after participating in prevention programs.
Sarcopenia, an ageing-associated disorder characterised by a decline in muscle mass, strength, and function, significantly increases the risk of falls, fractures, and physical disability. In this context, Kwon et al. [ 52 ] clustered individuals based on nutritional and health-related factors, aiming to identify the main risk factors for sarcopenia. Results indicated that low total energy intake, inadequate levels of physical activity, a high prevalence of chronic diseases, and bad habits (e.g., smoking and high alcohol consumption) emerged as predominant risk factors across all groups. Regarding physical activity, the work by Bae et al. [ 24 ] developed a DL model for predicting the decline in physical fitness due to sarcopenia in individuals with potential sarcopenic symptoms. Results showed that the waist circumference, body fat percentage, and performance on the timed up-and-go and sit-and-reach tests were crucial factors for predicting possible sarcopenia. Sarcopenia can be diagnosed through medical images, but outlining irregular contours of abdominal body parts is highly laborious and time-consuming. To solve this, Gu et al. [ 41 ] designed a DL-based method to automate the segmentation and quantification of body parts from CT scans, achieving notable accuracy in predicting sarcopenia.
3.1.3 Age-related sociodemographic changes
Populations and societies as a whole play significant roles in shaping ageing processes. Contextual changes, such as social well-being, economic conditions, demographics, and quality of life and care can indirectly affect individuals’ ageing trajectories. The importance of social relations and economic aspects in human longevity was early reported in Ribeiro and Zárate [ 74 ]. This corroborated the idea that the environment is a complex structure and that every part of it influences how fast biological ageing happens, even on the prevalence and development of depressive symptoms in old age [ 91 ]. From a longitudinal study in the western Pacific region, which combined both ageing and low- and middle-income countries, Molassiotis et al. [ 64 ] identified sociodemographic and socioeconomic developments as more important factors to the disease burden among older adults than the health expenditure, whereas non-communicable diseases contributed the most to shorten life expectancy. By clustering similar countries, the authors encouraged regional governments and policymakers to prioritise healthy ageing through coordinated strategic planning. Later, Santamaria-Garcia et al. [ 78 ] identified a number of demographic, social, and economic predictors of cognition and functionality in ageing populations. Also, reducing the level of social deprivation among older adults could positively influence their eating habits and dietary quality, thereby enhancing health outcomes during ageing [ 84 ]. According to the above, considering sociodemographic data into longitudinal studies is paramount to understanding individual ageing trajectories and predicting health status [ 37 ] and quality of life [ 55 ].
3.1.4 Ageing biomarkers
Ageing biomarkers are objective measures used to assess individuals’ ageing process and acquire information about their health and functional status. They help understand the biological processes of ageing and provide insights into the underlying causes of age-related diseases. By combining different types of data, such as gene data, biochemical measurements, and images, complex AI models can help practitioners make better predictions about the evolution of individuals’ ageing. Biomarkers are hence useful to develop novel therapies and prevention strategies to delay ageing effects. According to the type of data used, biomarkers are classified into imaging biomarkers (when using images), molecular biomarkers (when using genomic data), and functional biomarkers (when using haematological data or physiological traits, among others).
Regarding imaging biomarkers, research has centred on brain age prediction to understand the relationship between age and brain morphometrics in the human developmental and ageing process. Studies using magnetic resonance imaging (MRI) have shown that the brain undergoes age-related neuroanatomical changes both regionally and at the network level during ageing. Hence, brain age became an early imaging-based biomarker of neural ageing and a potential biomarker for understanding neurodegenerative and age-associated brain diseases [ 30 ]. Later, Cole et al. [ 31 ] revealed that a brain-predicted age indicative of an older-appearing brain was associated with several aspects, such as weaker grip strength, poorer lung function, slower walking speed, lower fluid intelligence, higher allostatic load, and increased mortality risk. Brain age has been predicted with high accuracy from multiple ways, including topological changes of the brain structural connectivity [ 58 ], brain network perspective [ 51 ], the measures of grey matter morphology, white matter microstructure, and resting state functional connectivity [ 54 ], and structural MRI in combination with blood parameters [ 73 ].
Regarding molecular biomarkers, gene expression profiling is widely used to measure or estimate the passage of time in terms of molecular or genetic changes in organisms. These biomarkers are founded on the idea that concrete biological changes at the molecular or genetic level predictably accumulate over time. Specifically, the work by Freitas et al. [ 39 ] proposed a data mining approach to discriminate between ageing-related and non-ageing-related DNA repair genes. More recently, Mamoshina et al. [ 60 ] developed a tissue-specific biomarker using differential gene expression and pathway analysis to compare signatures of young and old muscle tissues. Supported by the highly accurate prediction results obtained, this kind of biomarkers can be used to identify new molecular targets for anti-ageing therapies. Also, the study in Fielding et al. [ 38 ] showed significant associations between cellular senescence biomarkers and physical performance decline in older adults.
System-wide metabolic changes can also be linked to ageing to develop functional biomarkers. In particular, blood tests have shown promise in predicting human chronological age, with markers such as albumin, glucose, alkaline phosphatase, urea, and erythrocytes emerging as particularly relevant indicators [ 72 ]. Upon that concept, Gullett et al. [ 42 ] later suggested microRNA as a reliable blood-based biomarker of cognitive ageing, and Nusinovici et al. [ 67 ] developed a retinal biological age biomarker using retinal photos, demonstrating its efficacy in predicting mortality and morbidity associated with cardiovascular diseases and cancer.
3.1.5 Factors for healthy ageing
Several articles focus on investigating and understanding the factors that contribute the most to active ageing and the prevention of diseases and disabilities. These studies are paramount to develop effective health interventions and policies aimed at promoting not only healthy and active ageing but also improving the quality of life of the elderly.
Sociodemographic, socioeconomic, and psychosocial factors play a crucial role in healthy ageing [ 28 , 62 ]. For instance, Ahmadi et al. [ 22 ] observed that life satisfaction, quality of life, and social relationships were key factors in predicting successful ageing. Similarly, Yu et al. [ 95 ] found that factors such as the place of residency, household type, and neighbourhood infrastructure and facilities influenced domain-specific determinants of active ageing. Moreover, the impact of combined daily activities on memory decline was investigated in Krakovska et al. [ 50 ]. Authors showed that engaging in various activities had a stronger effect on memory decline, suggesting the potential efficacy of health policies promoting conducting diverse and multiple activities.
Leveraging computational biology, Cruces-Salguero et al. [ 32 ] recently conducted a study on centenarian individuals to identify the key characteristics associated with this demographic. Their research emphasised factors such as the number of diseases, medication usage, frequency of medical consultations, and levels of haemoglobin. Notwithstanding, conducting prolonged studies on humans to understand the diverse nature of the ageing processes is impractical. For this reason, Ala-Korpela et al. [ 23 ] investigated whether cross-sectional modelling could differentiate between slow and accelerated ageing in a human population. Results indicated a lack of evidence suggesting that cross-sectional modelling of ageing provides insights into the rate at which an individual’s metabolic profiles change over time.
3.1.6 Reviews
As observed, the application of AI in ageing has gathered the attention of the research community over the years. Thus, previous literature reviews have already been elaborated to organise and summarise existing studies in this field. For instance, the works in Fabris et al. [ 35 ] and Farrell et al. [ 36 ] examined studies that used supervised ML algorithms to gain insights into ageing processes, including the development of data-driven systems-level models. In [ 97 ], authors presented a holistic vision of the use of AI in ageing and longevity research, while the particular benefits of ageing biomarkers based on DL techniques were explored in Zhavoronkov and Mamoshina [ 98 ]. Interestingly enough, the authors in Woodman and Mangoni [ 90 ] presented a thorough overview of the application of AI in geriatric medicine in older populations, where ageing is a key component. Notwithstanding, all these works present methodological limitations. As a systematic study, Leghissa et al. [ 57 ] recently explored the use of ML and DL for detecting and predicting frailty in elderly people. For comparative purposes, Table 3 outlines the main characteristics of these reviews and highlights how our literature review differs from them.
3.2 Data in ageing studies: Types and sources
Research on human ageing, especially when adopting AI-driven strategies, relies on data. A wide range of data types has been used, as indicated in Table 2 and graphically depicted in Fig. 3 . This section aims to identify the most relevant data types and data sources in ageing studies (RQ2).
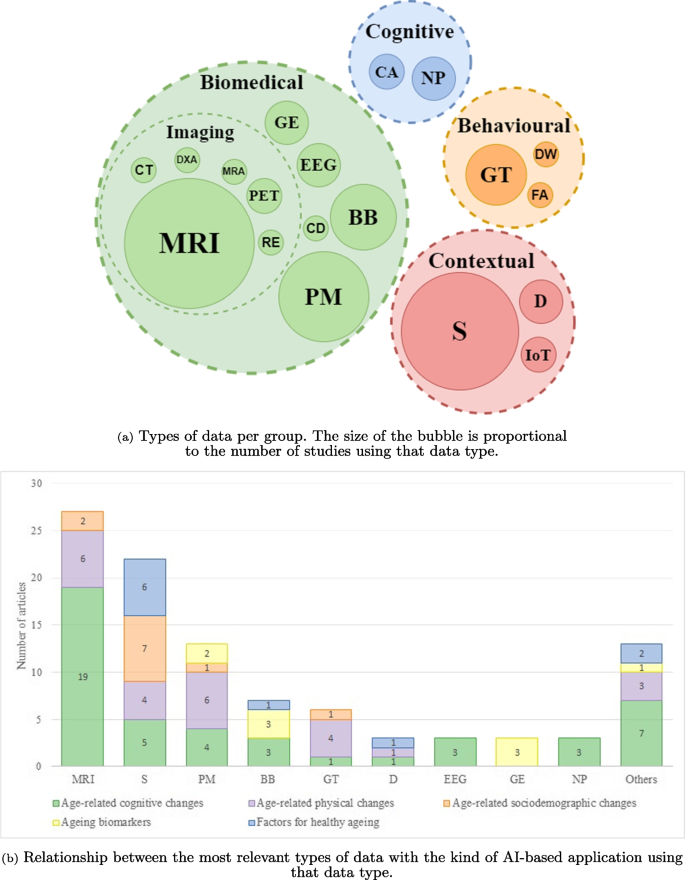
Summary of the types of data used in ageing research. Legend: {BB: Blood biochemistry, CA: Cognitive assessment test, CD: Clinical data, CT: Computed tomography scan, D: Demographic, DW: Drawing, DXA: Dual-energy X-ray absorptiometry measurements, EEG: Electroencephalogram, FA: Functional assessment, GE: Gene expression profile, GT: Gait test, IoT: Internet of Things sensors, MRA: Magnetic resonance angiography scan, MRI: Magnetic resonance imaging scan, NP: Neuropsychological test, PET: Positron emission tomography scan, PM: Physical measures, RE: Retinal photographs, S: Survey}
Biomedical data are the most prominent group of data types by providing health parameters and physiological markers that help study ageing phenomena at a microscopic scale. In this group, we highlight the different types of techniques to produce in-body images, such as MRI scans [ 27 , 30 , 31 , 44 , 45 , 48 , 51 , 53 , 54 , 56 , 58 , 59 , 61 , 68 , 70 , 73 , 75 , 77 , 79 , 80 , 81 , 83 , 85 , 92 , 94 , 96 ], positron emission tomography (PET) scans [ 34 , 71 ], and computed tomography (CT) scans [ 41 ]. In this context, most MRI images are brain scans to identify cognitive changes by determining the functional activity of brain regions along ageing as well as discover novel biomarkers. Besides images, biochemical blood measurements [ 23 , 38 , 40 , 45 , 72 , 73 , 83 ] and genetic data [ 39 , 42 , 60 ] are usually used in addition to more generic clinical and physical information (e.g., muscle strength, balance, endurance...).
Cognitive data help interpret the complexity of mental and neuropsychological dimensions of individuals by means of cognitive assessment tests (e.g., Mini-Mental State Examination) [ 47 , 93 ] and neuropsychological tests [ 40 , 48 , 83 ]. Another commonly used kind of data is behavioural data, which provide observable patterns of individuals. These data are generally used to detect and predict pattern changes over time due to ageing. In this context, gait analysis uses spatiotemporal, kinetic, and kinematic measurements collected from inertial sensors and motion cameras [ 25 , 43 , 55 , 66 , 85 , 96 ]. Lastly, contextual data refer to background information providing perspective and a broader understanding of individuals, such as social, economic and demographic indicators [ 32 , 43 , 47 ], wearable- and smart home IoT-collected data [ 46 , 69 ], and other data collected through surveys [ 22 , 23 , 28 , 29 , 33 , 37 , 49 , 50 , 52 , 55 , 62 , 64 , 65 , 74 , 76 , 78 , 83 , 84 , 88 , 89 , 91 , 95 ].
Some studies do not only use a single type of data but combine and integrate multiple types of data [ 23 , 32 , 38 , 40 , 43 , 45 , 47 , 48 , 52 , 54 , 55 , 69 , 73 , 76 , 83 , 85 , 89 , 93 , 96 ]. Particularly, imaging data are usually combined with other data types, such as blood biochemistry, physical measurements, neuropsychological tests, and surveys. This multidimensional approach enriches the understanding of factors influencing ageing, their impact on health, and the development of targeted interventions to promote healthy ageing and the well-being of older populations.
To foster research on ageing, different populations have been studied through longitudinal ageing datasets. These datasets have been analysed using AI techniques by some of these works, such as the China Health and Retirement Longitudinal Study (CHARLS) [ 95 ], the Chinese Longitudinal Healthy Longevity Survey (CLHLS) [ 88 ], the Colombian Survey on Health, Well-Being, and Aging (SABE) [ 78 ], the English Longitudinal Study of Aging (ELSA) [ 28 , 33 , 37 , 49 , 74 ], the Korean Longitudinal Study of Aging (KLoSA) [ 65 ], the Health and Retirement Study (HRS) [ 29 , 49 , 50 ], and the Genotype-Tissue Expression (GTEx) [ 60 ], among others. A description of the most well-known public datasets intended for research on ageing is provided in Table 4 . As observed, these datasets consider small populations (thousands of individuals), which hinders the ability to extrapolate results to larger scales, e.g., at a national level. The high cost to create these datasets (technical infrastructure, human resources...) is certainly a limiting factor.
3.3 AI techniques: approaches and classification
Research on ageing leverages AI techniques, particularly ML techniques, to extract meaningful knowledge from vast amounts of data (see Table 2 and Fig. 4 ). This section aims to identify and classify the most commonly used AI techniques (RQ3).
ML is a discipline within the field of AI which, by means of algorithms, provides computers with the ability to identify complex patterns from large amounts of data. Contrary to rule-based systems or expert systems, which require a set of predefined rules and domain knowledge introduced by experts, ML algorithms imitate the way that humans learn without being explicitly programmed. ML models are hence useful to explore associations and make more accurate classifications and predictions than their statistical counterparts and confer greater flexibility when modelling unstructured and nonlinear data. However, ML models require large data samples, which are not always possible, to achieve high accuracy and have been criticised in the latest years for their lack of interpretability and explainability [ 99 ].
The studies of this systematic review leverage ML techniques for different purposes, namely prediction (i.e., estimating or forecasting future values or outcomes using historical data or observed characteristics), detection (i.e., identifying the presence or absence of specific events or patterns in datasets), classification (i.e., assigning data to groups or predefined classes based on their characteristics), and association (i.e., identifying relationships and connections among elements in datasets). As reported in Table 2 , more than half of the studies reviewed concentrate on prediction tasks (58%), followed by detection and classification tasks (20% and 18%, respectively), whereas association tasks have little relevance (4%).
Supervised and unsupervised learning are examples of two different types of ML approaches. On the one hand, supervised learning algorithms are trained on a set of labelled data, i.e., the input data is paired with the desired output, so they learn to predict the output from new input data. Supervised learning is often used for classification, regression, and object detection tasks. On the other hand, unsupervised learning algorithms are trained on a set of unlabelled data, i.e., the input data is not paired with the desired output, so they learn to find patterns and relationships in the data. Unsupervised learning is often used for tasks such as clustering, dimensionality reduction, feature selection, and anomaly detection.
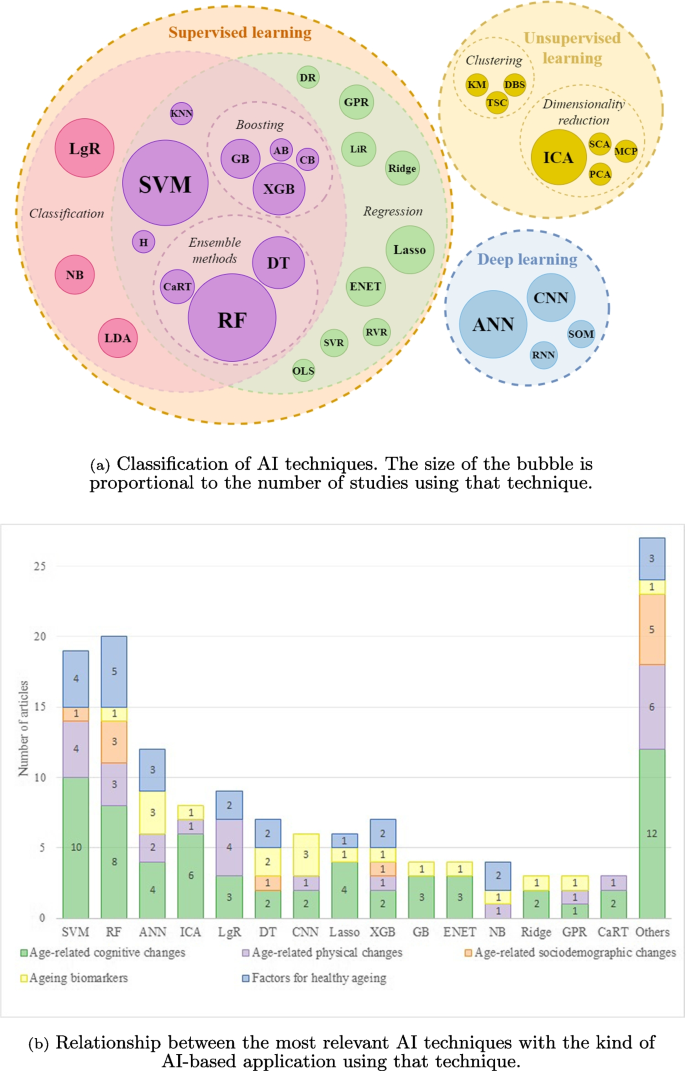
Summary of the AI techniques used in ageing research. Legend: {AB: AdaBoost, ANN: Artificial neural network, CaRT: Classification and regression tree, CB: CatBoost, CNN: Convolutional neural network, DBS: DBSCAN, DR: Dual regression, DT: Decision tree, ENET: Elastic net, GB: GradientBoost, GPR: Gaussian process regression, H: Holistic atlases of functional networks and interactions, ICA: Independent component analysis, KM: k -means, KNN: k -nearest neighbours, Lasso: Least absolute shrinkage and selection operator, LDA: Linear discriminant analysis, LgR: Logistic regression, LiR: Linear regression, MCP: Minimax concave penalty, NB: Naive Bayes, OLS: Ordinary least squares regression, PCA: Principal component analysis, RF: Random forest, Ridge: Ridge regression, RNN: Recurrent neural network, RVR: Relevance vector regression, SCA: Smoothly clipped absolute deviation, SOM: Self-organised maps, SVM: Support vector machines, SVR: Support vector regression, TSC: Two-step clustering, XGB: Extreme gradient boosting}
One of the most extensively used supervised ML algorithms is support vector machines (SVM), useful to solve both complex classification and regression problems by performing optimal data transformations that determine boundaries between data points based on predefined classes in a multidimensional space. In ageing studies, SVMs have been helpful in automatically recognising ageing-related changes in gait patterns between young and elderly walking [ 25 ], distinguishing age-related differences in biomedical data like images [ 61 , 63 ], as well as predicting cognitive impairment [ 66 , 70 ], frailty [ 33 ], and quality of life indicators [ 55 ]. Similarly, classification and regression problems are also commonly addressed using random forest (RF) and decision tree (DT) algorithms, which can handle both categorical and continuous data. DTs are a non-parametric method that predicts the value of a target variable by learning simple decision rules inferred from the data features. Ageing studies have used DTs to develop ageing predictor models from highly-dimensional datasets [ 22 , 42 ], predict cognitive decline in adults using physical and imaging data [ 66 , 82 ], and classify longevity profiles from longitudinal data [ 74 ]. However, DTs are prone to overfitting the training data, especially when trees become very deep or complex, resulting in poor performance on new, unseen data. To solve this problem, RFs are a combination of DTs whose results are accumulated into a final output. Hence, most studies prefer using RF rather than DT algorithms. For instance, RF methods have been used to predict cognitive function and changes [ 29 , 47 , 48 , 50 , 88 , 93 ], classify and predict factors related to successful ageing and quality of life [ 22 , 28 , 76 , 95 ], and detect undesirable age-related patterns [ 84 ].
In the realm of pure ML classification algorithms, the logistic regression (LgR) algorithm, naive Bayes (NB) algorithm, and linear discriminant analysis (LDA) stand out. These algorithms help predict discrete outputs, distinguish between different categories, or identify which of several classes an observation belongs to. LgR is a fast, scalable algorithm for binary classifications, especially when there are no complex relationships among data features, to e.g., analyse sarcopenia associations [ 52 ], and identify the main characteristics linked to centenarians [ 32 ]. NB is a probabilistic classifier based on the Bayes theorem with the “naive” assumption of independence among features/predictors, i.e., the presence of one particular feature does not affect the other, which is not always true in the real world. For instance, NB classifiers were used to discriminate between ageing-related and non-ageing related DNA repair genes [ 39 ]. Multi-class classification problems are solved using LDA, aimed at finding a linear combination of features that maximises the separation between two or more classes. LDA is a fundamental tool in pattern recognition in order to recognise recurring patterns or structures in data [ 75 ].
Whereas ML classification algorithms are intended to predict or classify discrete values, ML regression algorithms provide prediction tools to investigate the relationship between a dependent, continuous variable and independent variables (predictors). The most basic regression model is linear regression (LiR), which has been successfully used to predict the physical and mental components associated with health-related quality of life [ 55 ]. However, LiR fails to identify the most relevant predictor variables, especially when data is highly dimensional. To overcome this, LiR models have been adapted to improve variable selection and model regularisation. Among others, the most popular ones are Lasso regression, Ridge regression, and Elastic Net (ENET) regression which combines the Lasso and Ridge regression models. For instance, these three algorithms performed similarly in predicting brain age [ 51 ].
Unsupervised learning algorithms are suited for analysing complex, highly correlated, unlabelled data. One of the most popular algorithms is independent component analysis (ICA), which aims to separate and identify the underlying independent sources in large, multivariate datasets. Hence, ICA helps extract the most relevant features from data and understand hidden structures. This technique is suitable for understanding age-related impairments and ageing factors by analysing brain signals and brain images [ 44 , 45 , 68 , 71 , 77 , 96 ]. Also, unsupervised learning enables discovering natural groups within data without any prior knowledge. For instance, groups of similar individuals based on health-related factors [ 52 ] or longevity profiles [ 74 ] were identified using clustering algorithms, such as k -means (KM) and DBSCAN (DBS) algorithms, respectively.
In recent years, DL, a branch within the ML field, has gained increasing attention. DL relies on artificial neural networks (ANN), capable of learning complex and intricate patterns and relationships within data and, then, using these patterns to make predictions or decisions about new data. ANNs are designed to imitate how humans think and learn by means of layers of interconnected nodes (called neurons) that work together to apply nonlinear data transformations. By building computational models with multiple processing layers, networks can create multiple levels of abstraction to represent the data. Ageing studies have taken advantage of ANNs to predict sarcopenia [ 24 ] and ageing-related cognitive variables [ 53 ], as well as to develop ageing biomarkers [ 58 , 60 , 72 ]. There are different types of ANNs for solving specific tasks. On the one hand, convolutional neural networks (CNN) are prevalent in image processing, such as MRI and CT scans, for predicting brain age [ 30 , 73 ], biological age [ 67 ] and sarcopenia [ 41 ], and detecting brain changes [ 79 , 94 ]. On the other hand, recurrent neural networks (RNN) work with sequential or temporal data to estimate, for instance, mortality using the history of previous health states [ 37 ], or physical activity energy expenditure using sensor data [ 69 ].
Most research studies employing AI techniques, including those reviewed in this systematic review, only focus on the performance of their models, often neglecting the interpretability and explainability of such AI models. The black-box nature of ML models, which are unable to provide clear explanations for their decisions, results in a lack of transparency. This has prompted the emergence of explainable AI (XAI) [ 100 ], the next frontier in AI research. XAI encompasses a set of processes and methods designed to enable humans to comprehend and trust the outcomes generated by ML algorithms. In this review, only four studies have considered this approach. Concretely, Bae et al. [ 24 ] employed the SHapley Additive exPlanations (SHAP) and the Local Interpretable Model-Agnostic Explanations (LIME) methods to assess the functioning of the AI model’s predictions, which helped the authors understand which characteristics contributed most significantly to predicting sarcopenia. Similarly, [ 89 , 93 ] evaluated their resulting AI models using the SHAP method. Finally, Khodabandehloo et al. [ 46 ] presented a novel architecture with a built-in AI explanatory module designed to detect symptoms of cognitive decline, thereby enhancing support for clinicians in diagnostic decision-making.
4 Discussion
Despite the progressive research advances in ageing studies, there is still a long road ahead to foster effective active ageing initiatives and promote healthy lifestyles among older adults. Building upon the main findings from the literature, we next discuss the main challenges in the field (RQ4) and outline future opportunities for ageing research leveraging the full potential of AI (RQ5).
4.1 Main findings
As the world’s population ages, the pursuit of a longer and healthier life has become a priority for scientists and researchers worldwide. Ageing involves complex biological processes influenced by various factors, including genetics, diseases, disorders, social habits, lifestyle, and environmental exposures [ 101 ]. Due to the complexity inherent in these processes, traditional computing methods are insufficient in identifying underlying ageing phenomena. In this context, AI has emerged as a key tool capable of discovering hidden patterns within large, complex, heterogeneous, and nonlinear datasets to make accurate age-related predictions. The integration of AI into the study of human ageing not only yields a deeper understanding of these processes but also enables the development of long-term strategies for building preventative and sustainable healthcare systems. The main findings of our review are enumerated below.
AI enhances cognitive ageing assessments
AI has been predominantly used to study cognitive changes associated with ageing, particularly in identifying subtle patterns and changes in the structure and function of the human brain. ML techniques demonstrate proficiency in developing complex models from imaging data, especially MRI and PET scans, to detect or predict abnormal cognitive ageing trajectories. Specifically, affected brain regions can be assessed with high precision, and brain biomarkers can be later developed for the early detection of potential cognitive impairments, such as dementia. Thus, AI improves cognitive ageing assessment and enables the implementation of personalised and advanced intervention strategies to promote proper cognitive health.
AI facilitates early detection of abnormal physical conditions
AI has proven useful in evaluating age-related physical changes. ML algorithms are employed to identify patterns in physical health status and detect early indicators of abnormal conditions such as mobility impairments, sarcopenia, or frailty, which may increase the risk of falls or functional decline. Given the predictive nature of such studies, these insights are crucial for clinicians to intervene at early stages and implement strategies to mitigate or delay their effects.
Contextual factors influence ageing
Beyond physical and cognitive conditions, contextual factors such as social, economic, demographic, and lifestyle variables significantly influence ageing patterns. Although these aspects were not traditionally considered in early studies, they are increasingly being taken into account in more recent research. Adopting positivist perspectives towards old age enables the development of digital health interventions aimed at assisting individuals in modifying their daily habits to promote healthy ageing. By leveraging data from wearable devices and other IoT sources, AI provides personalised recommendations for exercise, diet, and other lifestyle factors that could affect the ageing processes.
DL techniques facilitate biomarkers development
As computational capabilities have increased, AI models have evolved towards more complex structures, mainly using DL approaches with neural network architectures. These models can analyse genetic, haematological, molecular, and imaging data, among other sources, to identify biomarkers aimed at predicting aspects of functional ageing and even facilitating specific longevity interventions. Although biomarkers are objective indicators of the biological ageing process, their standardisation, validation, and reproducibility are hindered by biological diversity [ 102 ]. Overcoming these obstacles requires not only sophisticated DL-based models but also a concerted effort to collect and provide inclusive, diverse, and longitudinal data to advance research in this arena.
Need for longitudinal datasets
Research on ageing requires longitudinal datasets that track individuals’ health trajectories, lifestyle changes, and socioeconomic circumstances across multiple waves. These datasets are indispensable for understanding ageing dynamics upon which AI models are built to understand ageing processes. While datasets like the HRS, ELSA, and CHARLS encompass data from thousands of individuals over decades, most datasets are constrained by shorter duration and smaller sample sizes due to the elevated costs involved in their generation.
4.2 Challenges
While AI holds promising potential, several challenges must be addressed to grasp the full potential of these AI-based systems for research on ageing. These challenges have been classified into (i) data challenges, (ii) algorithmic and modelling challenges, and (iii) operational challenges. Regarding data challenges, the following aspects have been identified:
Data quality and completeness
ML algorithms rely heavily on large amounts of high-quality, complete, consistent, and noise-free data. Data quality issues and inadequate sample sizes may not accurately reflect reality, leading to model inefficiencies when applied to new data samples. More extensive longitudinal datasets are needed to mitigate these limitations because current publicly available data repositories often comprise limited population samples.
Data labelling
Supervised ML algorithms require labelled data, which involves standardised assessments and objective annotation. For example, labelling brain images to distinguish between those of healthy adults and those of adults with cognitive impairments. This annotation process is often manual and time-consuming, often requiring the expertise of multiple specialists. Incorporating human knowledge into AI models (human-in-the-loop [ 103 ]) helps identify and correct data problems, algorithmic biases, and potential erroneous results.
Accessibility to electronic health records (EHR)
EHRs are a suitable tool to provide standardised, reliable, and interoperable health records that facilitate the analysis of health and physiological parameters, as well as the tracking of diagnoses, procedures, and interventions across large populations. The adoption of EHRs in ageing research would significantly alleviate data integration and management issues. However, accessibility to EHRs may be limited due to bureaucracy and regulatory obstacles along with high implementation and maintenance costs, posing serious challenges in obtaining complete health histories.
Reliability of self-reported data
Self-reported surveyed data, including sociodemographic information, habits, diet, and test evaluations, allow for frequent measurements and precise projections of ageing’s impact. However, self-reported data can be non-representative and biased, relying on individuals’ memory and self-perception.
Next, the following challenges related to AI algorithmic and modelling aspects have been identified:
Lack of generalisation in AI ageing-related models
Most AI-generated models for ageing are ad-hoc solutions trained on concrete data specific to particular regions and cohorts. Recent research by Chekroud et al. [ 104 ] revealed that ML models often perform well on one dataset but poorly on other datasets, indicating a lack of generalisation. Validating ML models across different contexts is crucial to ensure their reliability.
Multimodal learning
Combining data from various sources and types, such as biomedical, imaging, demographic, and contextual data, can achieve a comprehensive understanding of ageing-related processes. Unfortunately, this is uncommon. Data fusion enables multimodal learning [ 105 ] to improve model performance and reduce dependency on large datasets. However, this presents further challenges including data quality, granularity, interoperability, and temporal misalignment issues.
Continuous re-evaluation of models
As new data waves are introduced into longitudinal datasets, previously unobserved patterns or shifts in population characteristics may emerge, revealing behaviours not detected in the original datasets. This involves periodic retraining and revalidation of ML models to ensure their accuracy and relevance. Consequently, models must adapt to these potential changes to maintain their performance across evolving datasets. The maintenance of these models requires substantial computational resources.
Trustworthiness and interpretability
Enhancing the trustworthiness of AI algorithms is essential. In line with the XAI paradigm, efforts should concentrate on developing novel methods for interpreting AI models to reduce their black-box nature. Making AI decisions comprehensible and explainable will significantly increase trust in this technology.
Last, the following operational challenges have been identified within this context:
Computational cost
DL-based approaches are computationally intensive, requiring high-performance computing infrastructures with elevated computational, memory, storage, and energy consumption costs. While these approaches are suitable for automatically extracting features and estimating millions of parameters within models, they also require extensive amounts of labelled data to achieve high performance, which limits their scalability in real-life ageing applications.
Data protection and ethical considerations
Both data protection and ethical considerations are fundamental in human ageing studies. Practices such as anonymising personally identifiable information, obtaining informed consent, and implementing strict access controls, as outlined in the EU General Data Protection Regulation, are necessary. However, as data collection becomes more opportunistically and ubiquitous through wearables, sensors, and IoT devices, these aspects must be further emphasised. From the ethics perspective, discriminatory models must be avoided and minority profiles cannot be disregarded during AI training [ 106 ]. In this context, the recently adopted AI Act within the EU represents an initial but very important step toward regulating the use of AI [ 107 , 108 ].
4.3 Opportunities and promising applications
The intersection of AI and ageing research represents a promising frontier capable of revolutionising our understanding of ageing processes and developing innovative solutions to promote healthy ageing. Encouraging interdisciplinary collaboration among computer scientists, AI specialists, physicians, ageing researchers, and other professionals is crucial for overcoming the aforementioned challenges. Efforts must be devoted to developing proactive initiatives and preparing healthcare systems to minimise the impact of severe age-related diseases and unhealthy ageing in the years to come. To this end, the main opportunities and promising application in the field are described below.
Understanding ageing traits
The complexity of biological processes along with the lack of appropriate analytical tools hamper the understanding of the interplay of ageing traits and their role in age-related dysfunction. Although age-related traits have been identified [ 109 ], understanding their interrelationship across biological, environmental, and genetic domains remains open. Specifically, DL techniques are promising in uncovering relationships with cellular function, tissue-specific physiology, and biomarker development through the analysis of omics data.
Contextualisation of ageing processes
While age-related factors have traditionally been considered intrinsic, such as genetics and epigenetic changes, contextual factors like diet, exercise, lifestyle, and smoking and drinking habits are now recognised for their influence in accelerating the ageing process [ 110 ]. Analysing these habits, behaviours, and sociodemographic aspects can reveal different patterns and dynamics related to human ageing. Continuous collection and monitoring of these variables are essential for studying changes at an individual level over time. Promoting studies that incorporate the analysis of extrinsic and contextual variables to complement findings from current ageing studies is desirable.
Augment data collection with Wearable and mobile technology
User-centred biomedical data collected from wearable and mobile devices could augment traditional health records. For instance, these technology can support the automatic monitoring and assessment of intrinsic capacity in older adults following the practices outlined in the ICOPE guidelines [ 111 ]. This automation enables closer tracking of patients evolution, beyond traditional physician visits. This mass sensorisation paves the way to study digital biomarkers, opening new avenues for research in ageing and healthcare [ 112 ].
Towards context-aware health paradigms
Context-aware paradigms, such as smart health, offer numerous ageing-oriented solutions. Leveraging data gathered from the user’s environment (e.g., smart homes, nursing homes...) through sensors and IoT devices, diverse routines, habits, and patterns can be detected. These insights enable the provision of personalised services to older adults, encompassing health status monitoring, prediction of health trajectories, early detection of cognitive or physical decline, healthy habits recommendation, and assessment of quality of life and well-being.
Crowdsourcing-based solutions
Crowdsourcing-based data solutions present exciting opportunities for studying human ageing. This approach involves collecting, labelling, and classifying large datasets with the help of mass collaboration, aiding in the training, validation, and improvement of ML algorithms. Active participation from the global community would provide a diverse and extensive dataset. Addressing data quality issues could help identify risk factors across different cohorts and develop large-scale biomarkers.
5 Conclusion
Population ageing is a global phenomenon that profoundly impacts economic, social, and health conditions worldwide. AI is shifting many sectors with groundbreaking technological advances, and research on ageing is no exception. The adoption of AI tools is gradually contributing to improving our understanding of the ageing processes. However, the rapid evolution of AI techniques along with the steady advances in ageing research foster the need for a timely review of the state-of-the-art in this field. By following a rigorous review methodology, this article has presented a systematic literature review of the current knowledge on AI for ageing research. The research questions outlined in Table 1 summarise the main goals of our research, which include summarising the main applications, resources, and techniques employed in these studies, as well as highlighting the challenges and promising opportunities in this domain. These research questions are discussed in order next:
RQ1: How has AI currently been used for the study of human ageing?
The potential of AI in studying human ageing is vast, but its application must be justified. In Section 3.1 , we have identified the main goals and scope in which AI has been adopted to research on ageing. Our analysis categorises studies into five main areas: (i) age-related cognitive changes, (ii) age-related physical changes, (iii) age-related sociodemographic changes, (iv) ageing biomarkers, and (v) factors for healthy ageing. This taxonomy helps identify the main research areas where AI can make significant contributions. While the majority of studies fall under the first category, focusing on using AI to understand brain function and predict cognitive decline, the rise of DL techniques holds promise for developing ageing biomarkers. Each article has been thoroughly described by carefully emphasising its objectives and contributions.
RQ2: What data sources and data types have been used?
Research on ageing is evidence-based, requiring enormous datasets to derive meaningful knowledge. In Section 3.2 , we have observed a wide diversity and heterogeneity in the datasets used for ageing research. Biomedical-related data is predominant, as expected, but it is worth noting the relevance of imaging data, especially from brain regions taken from MRI, PET or CT scans. The analysis of physical measurements, genetic data, and blood measurements is relevant as well. Notwithstanding, there is a growing recognition of the importance of contextual data, which helps understanding ageing considering, not only biomedical data, but also social, economic, and demographic factors as well as lifestyle and daily habits. Understanding ageing within its proper context is essential for developing targeted ageing initiatives tailored to specific populations. Moreover, to foster research on ageing, there exist some public datasets from longitudinal studies. Despite that, these datasets are relatively small (considering only thousands of individuals), region-specific (mostly from China and the United States), and time-constrained (data collected within a decade or less). Addressing these limitations and promoting more comprehensive longitudinal studies, despite their associated costs, is critical to accelerating the pace of ageing research.
RQ3: How AI techniques have been used in current research on ageing?
The number of AI techniques employed for ageing research is vast, as observed in Section 3.3 . While ML models are well-suited for classification, prediction, and clustering tasks, DL models are gaining popularity due to their increased efficiency, but at the price of higher computational costs. In a nutshell, SVM, RF, and ANN emerge as the most prominent techniques in the field. However, these methods generally lack interpretability and explainability. To improve the transparency of AI-made decisions, studies are starting to integrate XAI approaches, although their adoption will be pivotal in the years ahead.
RQ4: Which are the main challenges in the field?
As summarised in Section 4.2 , continuous efforts are directed towards refining AI models to better comprehend ageing processes. However, challenges such as insufficient data training, data quality issues, and the lack of worldwide longitudinal datasets demonstrate that there is still a long road ahead to overcome all data challenges. Relying on data is first and foremost when adopting AI strategies. Leveraging the context-aware IoT network could enable seamless collection of continuous health data from large populations, though this must be accompanied by efforts to address privacy and ethical issues. Moreover, there is a pressing need to develop interpretable and explainable AI methods to reduce their current black-box nature. Building trust in these systems will be crucial for their successful deployment in real-life scenarios.
RQ5: Which opportunities remain open for future research directions?
Despite the steady progress in the field, research on ageing can still evolve rapidly with the latest AI enhancements. As discussed in Section 4.3 , we foresee numerous promising opportunities within the smart healthcare paradigm by leveraging the sensing and communication network of context-aware environments at the time of collecting health information. This information can help develop personalised AI-driven healthcare services and promote active ageing initiatives to improve the quality of life and well-being of older people.
We believe that the information analysed and the research questions answered in this review reflect the current state-of-the-art with high fidelity. As such, this article provides a fruitful and interdisciplinary ground of research and a clear overview of the challenges and opportunities that may need attention in the future. As a final note, we sustain that research on ageing should not focus on adding years to life, but on adding life to years.
Data Availibility
For the sake of transparency, the screening files used in this systematic literature review as well as the PRISMA checklists are available at the following link: https://smarttechresearch.com/opendata/AIageing .
Code Availability
Not applicable.
United Nations (2022) World Population Prospects 2022: Summary of Results. Technical Report UN DESA/POP/2022/TR/NO. 3, United Nations Department of Economic and Social Affairs, Population Division, New York, USA
United Nations (2023) The Sustainable Development Goals Report 2023, Special. Technical report, United Nations Department of Economic and Social Affairs, New York, USA
Google Scholar
World Health Organization (2020) Decade of healthy ageing: baseline report. Technical report, World Health Organization, Geneva, Switzerland
Beard JR, Officer A, De Carvalho IA, Sadana R, Pot AM, Michel J-P, Lloyd-Sherlock P, Epping-Jordan JE, Peeters GG, Mahanani WR et al (2016) The World report on ageing and health: a policy framework for healthy ageing. The Lancet. 387(10033):2145–2154
Article Google Scholar
Jaul E, Barron J (2017) Age-related diseases and clinical and public health implications for the 85 years old and over population. Front Public Health 5:335
Solanas A, Patsakis C, Conti M, Vlachos IS, Ramos V, Falcone F, Postolache O, Pérez-Martínez PA, Di Pietro R, Perrea DN et al (2014) Smart health: a context-aware health paradigm within smart cities. IEEE Commun Mag 52(8):74–81
Batista E, Borràs F, Martínez-Ballesté A (2015) Monitoring People with MCI: Deployment in a Real Scenario for Low-Budget Smartphones. In: Proc. 6th International Conference on Information, Intelligence, Systems and Applications (IISA), Corfú, Greece, pp. 1–6. IEEE
Casino F, Patsakis C, Batista E, Borras F, Martinez-Balleste A (2017) Healthy routes in the smart city: a context-aware mobile recommender. IEEE Softw 34(6):42–47
Maresova P, Krejcar O, Barakovic S, Husic JB, Lameski P, Zdravevski E, Chorbev I, Trajkovik V (2020) Health-related ICT solutions of smart environments for elderly - systematic review. IEEE Access. 8:54574–54600
Ferre M, Batista E, Solanas A, Martínez-Ballesté A (2021) Smart health-enhanced early mobilisation in intensive care units. Sensors. 21(16):5408
Batista E, Moncusi MA, López-Aguilar P, Martínez-Ballesté A, Solanas A (2021) Sensors for context-aware smart healthcare: a security perspective. Sensors. 21(20):6886
Batista E, Lopez-Aguilar P, Solanas A (2023) Smart health in the 6G era: bringing security to future smart health services. IEEE Communications Magazine 1–7
Secinaro S, Calandra D, Secinaro A, Muthurangu V, Biancone P (2021) The role of artificial intelligence in healthcare: a structured literature review. BMC Med Inform Decis Mak 21(125):1–23
Meskó B, Drobni Z, Bényei É, Gergely B, Győrffy Z (2017) Digital health is a cultural transformation of traditional healthcare. Mhealth 3
Solanas A, Casino F, Batista E, Rallo R (2017) Trends and challenges in smart healthcare research: a journey from data to wisdom. In: Proc. IEEE 3rd international forum on Research and Technologies for Society and Industry (RTSI), pp 1–6. IEEE
Bernal MC, Martínez-Ballesté A, Solanas A (2021) A review of data sources for the study of ageing. In: Proc. IEEE 45th annual Computers, Software, and Applications Conference (COMPSAC), pp 1843–1848. IEEE
Chu CH, Nyrup R, Leslie K, Shi J, Bianchi A, Lyn A, McNicholl M, Khan S, Rahimi S, Grenier A (2022) Digital ageism: challenges and opportunities in artificial intelligence for older adults. Gerontologist 62(7):947–955
Brocke J, Simons A, Niehaves B, Riemer K, Plattfaut R, Cleven, A (2009) Reconstructing the giant: on the importance of rigour in documenting the literature search process. In: Proc. 17th European Conference on Information Systems (ECIS), Verona, Italy, pp. 2206–2217
Moher, D., Liberati, A., Tetzlaff, J., Altman, D.G., Group, P. (2009) Preferred reporting items for systematic reviews and meta-analyses: the PRISMA statement. Ann Intern Med 151(4):264–269
Institute of Electrical and Electronics Engineers: IEEE Thesaurus (2024). https://www.ieee.org/publications/services/thesaurus.html
National Library of Medicine: Medical Subject Headings (MeSH) (2024). https://www.nlm.nih.gov/mesh
Ahmadi M, Nopour R, Nasiri S (2023) Developing a prediction model for successful aging among the elderly using machine learning algorithms. Digital Health. 9:20552076231178424
Ala-Korpela M, Lehtimäki T, Kähönen M, Viikari J, Perola M, Salomaa V, Kettunen J, Raitakari OT, Mäkinen V-P (2023) Cross-sectionally calculated metabolic aging does not relate to longitudinal metabolic changes—support for stratified aging models. The Journal of Clinical Endocrinology & Metabolism. 108(8):2099–2104
Bae J-H, Seo J-W, Kim DY (2023) Deep-learning model for predicting physical fitness in possible sarcopenia: analysis of the Korean physical fitness award from 2010 to 2023. Front Public Health 11:1241388
Begg R, Kamruzzaman J (2005) A machine learning approach for automated recognition of movement patterns using basic, kinetic and kinematic gait data. J Biomech 38(3):401–408
Bidelman GM, Mahmud MS, Yeasin M, Shen D, Arnott SR, Alain C (2019) Age-related hearing loss increases full-brain connectivity while reversing directed signaling within the dorsal-ventral pathway for speech. Brain Struct Funct 224(8):2661–2676
Boyle R, Jollans L, Rueda-Delgado LM, Rizzo R, Yener GG, McMorrow JP, Knight SP, Carey D, Robertson IH, Emek-Savaş DD et al (2021) Brain-predicted age difference score is related to specific cognitive functions: a multi-site replication analysis. Brain Imaging Behav 15(1):327–345
Caballero FF, Soulis G, Engchuan W, Sánchez-Niubó A, Arndt H, Ayuso-Mateos JL, Haro JM, Chatterji S, Panagiotakos DB (2017) Advanced analytical methodologies for measuring healthy ageing and its determinants, using factor analysis and machine learning techniques: the ATHLOS project. Sci Rep 7(1):43955
Casanova R, Saldana S, Lutz MW, Plassman BL, Kuchibhatla M, Hayden KM (2020) Investigating predictors of cognitive decline using machine learning. The Journals of Gerontology: Series B - Psychological Sciences and Social Sciences. 75(4):733–742
Cole JH, Poudel RPK, Tsagkrasoulis D, Caan MWA, Steves C, Spector TD, Montana G (2017) Predicting brain age with deep learning from raw imaging data results in a reliable and heritable biomarker. Neuroimage 163:115–124
Cole JH, Ritchie SJ, Bastin ME, Valdés Hernández MC, Maniega SM, Royle N, Corley J, Pattie A, Harris SE, Zhang Q et al (2018) Brain age predicts mortality. Mol Psychiatry 23(5):1385–1392
Cruces-Salguero S, Larrañaga I, Mar J, Matheu A (2023) Descriptive and predictive analysis identify centenarians’ characteristics from the Basque population. Front Public Health 10:1096837
Cunha Leme DE, De Oliveira C (2023) Machine learning models to predict future frailty in community-dwelling middle-aged and older adults: the ELSA cohort study. Journals of Gerontology: Series A - Biological Sciences and Medical Sciences. 78(11):2176–2184
Di X, Wölfer M, Amend M, Wehrl H, Ionescu TM, Pichler BJ, Biswal BB (2019) The Alzheimer’s disease neuroimaging initiative: interregional causal influences of brain metabolic activity reveal the spread of aging effects during normal aging. Hum Brain Mapp 40(16):4657–4668
Fabris F, Magalhães JPD, Freitas AA (2017) A review of supervised machine learning applied to ageing research. Biogerontology 18(2):171–188
Farrell S, Stubbings G, Rockwood K, Mitnitski A, Rutenberg A (2021) The potential for complex computational models of aging. Mech Ageing Dev 193:111403
Farrell S, Mitnitski A, Rockwood K, Rutenberg AD (2022) Interpretable machine learning for high-dimensional trajectories of aging health. PLoS Comput Biol 18(1):1009746
Fielding RA, Atkinson EJ, Aversa Z, White TA, Heeren AA, Achenbach SJ, Mielke MM, Cummings SR, Pahor M, Leeuwenburgh C, LeBrasseur NK (2022) Associations between biomarkers of cellular senescence and physical function in humans: observations from the lifestyle interventions for elders (LIFE) study. GeroScience. 44(6):2757–2770
Freitas AA, Vasieva O, Magalhães JP (2011) A data mining approach for classifying DNA repair genes into ageing-related or non-ageing-related. BMC Genomics 12(1):1–11
Ganguli M, Beer JC, Zmuda JM, Ryan CM, Sullivan KJ, Chang C-CH, Rao RH (2020) Aging, diabetes, obesity, and cognitive decline: a population-based study. J Am Geriatr Soc 68(5):991–998
Gu S, Wang L, Han R, Liu X, Wang Y, Chen T, Zheng Z (2023) Detection of sarcopenia using deep learning-based artificial intelligence body part measure system (AIBMS). Front Physiol 14:46
Gullett JM, Chen Z, O’Shea A, Akbar M, Bian J, Rani A, Porges EC, Foster TC, Woods AJ, Modave F et al (2020) MicroRNA predicts cognitive performance in healthy older adults. Neurobiol Aging 95:186–194
Hicks GE, Shardell M, Alley DE, Miller RR, Bandinelli S, Guralnik J, Lauretani F, Simonsick EM, Ferrucci L (2012) Absolute strength and loss of strength as predictors of mobility decline in older adults: the InCHIANTI study. Journals of Gerontology: Series A - Biological Sciences and Medical Sciences. 67(1):66–73
Huang C-C, Hsieh W-J, Lee P-L, Peng L-N, Liu L-K, Lee W-J, Huang J-K, Chen L-K, Lin C-P (2015) Age-related changes in resting-state networks of a large sample size of healthy elderly. CNS Neuroscience & Therapeutics. 21(10):817–825
Kharabian Masouleh S, Beyer F, Lampe L, Loeffler M, Luck T, Riedel-Heller SG, Schroeter ML, Stumvoll M, Villringer A, Witte AV (2018) Gray matter structural networks are associated with cardiovascular risk factors in healthy older adults. Journal of Cerebral Blood Flow & Metabolism. 38(2):360–372
Khodabandehloo E, Riboni D, Alimohammadi A (2021) HealthXAI: collaborative and explainable AI for supporting early diagnosis of cognitive decline. Futur Gener Comput Syst 116:168–189
Kimura N, Aso Y, Yabuuchi K, Ishibashi M, Hori D, Sasaki Y, Nakamichi A, Uesugi S, Fujioka H, Iwao S et al (2019) Modifiable lifestyle factors and cognitive function in older people: a cross-sectional observational study. Front Neurol 10:401
Koini M, Duering M, Gesierich BG, Rombouts SARB, Ropele S, Wagner F, Enzinger C, Schmidt R (2018) Grey-matter network disintegration as predictor of cognitive and motor function with aging. Brain Struct Funct 223:2475–2487
Komura T, Cowden RG, Chen R, Andrews RM, Shiba K (2023) Estimating the heterogeneous effect of life satisfaction on cognitive functioning among older adults: evidence of US and UK national surveys. SSM - Mental Health. 4:100260
Krakovska O, Christie GJ, Farzan F, Sixsmith A, Ester M, Moreno S (2021) Healthy memory aging - the benefits of regular daily activities increase with age. Aging (Albany NY). 13(24):25643
Kuo C-Y, Lee P-L, Hung S-C, Liu L-K, Lee W-J, Chung C-P, Yang AC, Tsai S-J, Wang P-N, Chen L-K et al (2020) Large-scale structural covariance networks predict age in middle-to-late adulthood: a novel brain aging biomarker. Cereb Cortex 30(11):5844–5862
Kwon Y-J, Kim HS, Jung D-H, Kim J-K (2020) Cluster analysis of nutritional factors associated with low muscle mass index in middle-aged and older adults. Clin Nutr 39(11):3369–3376
La Corte V, Sperduti M, Malherbe C, Vialatte F, Lion S, Gallarda T, Oppenheim C, Piolino P (2016) Cognitive decline and reorganization of functional connectivity in healthy aging: the pivotal role of the salience network in the prediction of age and cognitive performances. Frontiers in Aging Neuroscience. 8:204
Lange A-MG, Anatürk M, Suri S, Kaufmann T, Cole JH, Griffanti L, Zsoldos E, Jensen DEA, Filippini N, Singh-Manoux A et al (2020) Multimodal brain-age prediction and cardiovascular risk: the Whitehall II MRI sub-study. Neuroimage 222:117292
Lee M, Noh Y, Youm C, Kim S, Park H, Noh B, Kim B, Choi H, Yoon H (2021) Estimating health-related quality of life based on demographic characteristics, questionnaires, gait ability, and physical fitness in Korean elderly adults. Int J Environ Res Public Health 18(22):11816
Lee WH (2023) The choice of machine learning algorithms impacts the association between brain-predicted age difference and cognitive function. Mathematics. 11(5):1229
Leghissa M, Carrera Á, Iglesias CA (2023) Machine learning approaches for frailty detection, prediction and classification in elderly people: a systematic review. Int J Med Informatics 178:105172
Lin L, Jin C, Fu Z, Zhang B, Bin G, Wu S (2016) Predicting healthy older adult’s brain age based on structural connectivity networks using artificial neural networks. Comput Methods Programs Biomed 125:8–17
Lindbergh CA, Zhao Y, Lv J, Mewborn CM, Puente AN, Terry DP, Renzi-Hammond LM, Hammond BR, Liu T, Miller LS (2019) Intelligence moderates the relationship between age and inter-connectivity of resting state networks in older adults. Neurobiol Aging 78:121–129
Mamoshina P, Volosnikova M, Ozerov IV, Putin E, Skibina E, Cortese F, Zhavoronkov A (2018) Machine learning on human muscle transcriptomic data for biomarker discovery and tissue-specific drug target identification. Front Genet 9:242
Meier TB, Desphande AS, Vergun S, Nair VA, Song J, Biswal BB, Meyerand ME, Birn RM, Prabhakaran V (2012) Support vector machine classification and characterization of age-related reorganization of functional brain networks. Neuroimage 60(1):601–613
Mirzaeian R, Nopour R, Asghari Varzaneh Z, Shafiee M, Shanbehzadeh M, Kazemi-Arpanahi H (2023) Which are best for successful aging prediction? Bagging, boosting, or simple machine learning algorithms? Biomed Eng Online 22(1):85
Moezzi B, Pratti LM, Hordacre B, Graetz L, Berryman C, Lavrencic LM, Ridding MC, Keage HAD, McDonnell MD, Goldsworthy MR (2019) Characterization of young and old adult brains: an EEG functional connectivity analysis. Neuroscience 422:230–239
Molassiotis A, Kwok SWH, Leung AYM, Tyrovolas S (2022) Associations between sociodemographic factors, health spending, disease burden, and life expectancy of older adults (70+ years old) in 22 countries in the Western Pacific Region, 1995–2019: estimates from the Global Burden of Disease (GBD) Study 2019. GeroScience. 44(2):925–951
Na K-S (2019) Prediction of future cognitive impairment among the community elderly: a machine-learning based approach. Sci Rep 9(1):3335
Noh B, Yoon H, Youm C, Kim S, Lee M, Park H, Kim B, Choi H, Noh Y (2021) Prediction of decline in global cognitive function using machine learning with feature ranking of gait and physical fitness outcomes in older adults. Int J Environ Res Public Health 18(21):11347
Nusinovici S, Rim TH, Yu M, Lee G, Tham Y-C, Cheung N, Chong CCY, Da Soh Z, Thakur S, Lee CJ et al (2022) Retinal photograph-based deep learning predicts biological age, and stratifies morbidity and mortality risk. Age Ageing 51(4):065
Onoda K, Ishihara M, Yamaguchi S (2012) Decreased functional connectivity by aging is associated with cognitive decline. J Cogn Neurosci 24(11):2186–2198
Paraschiakos S, Sá CR, Okai J, Slagboom PE, Beekman M, Knobbe A (2022) A recurrent neural network architecture to model physical activity energy expenditure in older people. Data Min Knowl Disc 36(1):477–512
Park J, Carp J, Hebrank A, Park DC, Polk TA (2010) Neural specificity predicts fluid processing ability in older adults. J Neurosci 30(27):9253–9259
Pereira JB, Harrison TM, La Joie R, Baker SL, Jagust WJ (2020) Spatial patterns of tau deposition are associated with amyloid, ApoE, sex, and cognitive decline in older adults. Eur J Nucl Med Mol Imaging 47(9):2155–2164
Putin E, Mamoshina P, Aliper A, Korzinkin M, Moskalev A, Kolosov A, Ostrovskiy A, Cantor C, Vijg J, Zhavoronkov A (2016) Deep biomarkers of human aging: application of deep neural networks to biomarker development. Aging (Albany NY). 8(5):1021
Ren B, Wu Y, Huang L, Zhang Z, Huang B, Zhang H, Ma J, Li B, Liu X, Wu G et al (2022) Deep transfer learning of structural magnetic resonance imaging fused with blood parameters improves brain age prediction. Hum Brain Mapp 43(5):1640–1656
Ribeiro CE, Zárate LE (2019) Classifying longevity profiles through longitudinal data mining. Expert Syst Appl 117:75–89
Robinson EC, Hammers A, Ericsson A, Edwards AD, Rueckert D (2010) Identifying population differences in whole-brain structural networks: a machine learning approach. Neuroimage 50(3):910–919
Sajeev S, Champion S, Maeder A, Gordon S (2022) Machine learning models for identifying pre-frailty in community dwelling older adults. BMC Geriatr 22(1):794
Salami A, Wåhlin A, Kaboodvand N, Lundquist A, Nyberg L (2016) Longitudinal evidence for dissociation of anterior and posterior MTL resting-state connectivity in aging: links to perfusion and memory. Cereb Cortex 26(10):3953–3963
Santamaria-Garcia H, Moguilner S, Rodriguez-Villagra OA, Botero-Rodriguez F, Pina-Escudero SD, O’Donovan G, Albala C, Matallana D, Schulte M, Slachevsky A et al (2023) The impacts of social determinants of health and cardiometabolic factors on cognitive and functional aging in Colombian underserved populations. GeroScience 1–19
Schilling KG, Archer D, Yeh F-C, Rheault F, Cai LY, Hansen C, Yang Q, Ramdass K, Shafer AT, Resnick SM et al (2022) Aging and white matter microstructure and macrostructure: a longitudinal multi-site diffusion MRI study of 1218 participants. Brain Struct Funct 227(6):2111–2125
Sone D, Beheshti I, Shinagawa S, Niimura H, Kobayashi N, Kida H, Shikimoto R, Noda Y, Nakajima S, Bun S et al (2022) Neuroimaging-derived brain age is associated with life satisfaction in cognitively unimpaired elderly: a community-based study. Transl Psychiatry 12(25):1–6
Statsenko Y, Habuza T, Smetanina D, Simiyu GL, Uzianbaeva L, Neidl-Van Gorkom K, Zaki N, Charykova I, Al Koteesh J, Almansoori TM et al (2022) Brain morphometry and cognitive performance in normal brain aging: age-and sex-related structural and functional changes. Frontiers in Aging Neuroscience. 13:713680
Sun S, Liu D, Zhou Y, Yang G, Cui L-B, Xu X, Guo Y, Sun T, Jiang J, Li N et al (2023) Longitudinal real world correlation study of blood pressure and novel features of cerebral magnetic resonance angiography by artificial intelligence analysis on elderly cognitive impairment. Frontiers in Aging Neuroscience. 15:1121152
Tan WY, Hargreaves C, Chen C, Hilal S (2023) A machine learning approach for early diagnosis of cognitive impairment using population-based data. J Alzheimers Dis 91(1):449–461
Tatoli R, Lampignano L, Donghia R, Castellana F, Zupo R, Bortone I, De Nucci S, Campanile G, Lofù D, Vimercati L et al (2022) Dietary customs and social deprivation in an aging population from Southern Italy: a machine learning approach. Front Nutr 9:811076
Vaughan BA, Simon JE, Grooms DR, Clark LA, Wages NP, Clark BC (2022) Brain-predicted age difference moderates the association between muscle strength and mobility. Frontiers in Aging Neuroscience. 14:808022
Vergun S, Deshpande AS, Meier TB, Song J, Tudorascu DL, Nair VA, Singh V, Biswal BB, Meyerand ME, Birn RM et al (2013) Characterizing functional connectivity differences in aging adults using machine learning on resting state fMRI data. Front Comput Neurosci 7:38
Vysata O, Kukal J, Prochazka A, Pazdera L, Simko J, Valis M (2014) Age-related changes in EEG coherence. Neurol Neurochir Pol 48(1):35–38
Wang S, Wang W, Li X, Liu Y, Wei J, Zheng J, Wang Y, Ye B, Zhao R, Huang Y et al (2022) Using machine learning algorithms for predicting cognitive impairment and identifying modifiable factors among Chinese elderly people. Frontiers in Aging Neuroscience. 14:977034
Wei M, He S, Meng D, Yang G, Wang Z (2023) Hybrid exercise program enhances physical fitness and reverses frailty in older adults: insights and predictions from machine learning. J Nutr Health Aging 27(10):894–902
Woodman RJ, Mangoni AA (2023) A comprehensive review of machine learning algorithms and their application in geriatric medicine: present and future. Aging Clin Exp Res 35(11):2363–2397
Wu Y, Su B, Chen C, Zhao Y, Zhong P, Zheng X (2023) Urban-rural disparities in the prevalence and trends of depressive symptoms among Chinese elderly and their associated factors. J Affect Disord 340:258–268
Xiong M, Lin L, Jin Y, Kang W, Wu S, Sun S (2023) Comparison of machine learning models for brain age prediction using six imaging modalities on middle-aged and older adults. Sensors. 23(7):3622
Yamada Y, Shinkawa K, Kobayashi M, Badal VD, Glorioso D, Lee EE, Daly R, Nebeker C, Twamley EW, Depp C et al (2022) Automated analysis of drawing process to estimate global cognition in older adults: preliminary international validation on the US and Japan data sets. JMIR Formative Research. 6(5):37014
Yamada S, Otani T, Ii S, Kawano H, Nozaki K, Wada S, Oshima M, Watanabe Y (2023) Aging-related volume changes in the brain and cerebrospinal fluid using artificial intelligence-automated segmentation. Eur Radiol 33(10):7099–7112
Yu J, Huang W, Kahana E (2022) Investigating factors of active aging among Chinese older adults: a machine learning approach. Gerontologist 62(3):332–341
Yuan J, Blumen HM, Verghese J, Holtzer R (2015) Functional connectivity associated with gait velocity during walking and walking-while-talking in aging: a resting-state fMRI study. Hum Brain Mapp 36(4):1484–1493
Zhavoronkov A, Mamoshina P, Vanhaelen Q, Scheibye-Knudsen M, Moskalev A, Aliper A (2019) Artificial intelligence for aging and longevity research: recent advances and perspectives. Ageing Res Rev 49:49–66
Zhavoronkov A, Mamoshina P (2019) Deep aging clocks: the emergence of AI-based biomarkers of aging and longevity. Trends Pharmacol Sci 40(8):546–549
Black JE, Kueper JK, Williamson TS (2023) An introduction to machine learning for classification and prediction. Fam Pract 40(1):200–204
Minh D, Wang HX, Li YF, Nguyen TN (2022) Explainable artificial intelligence: a comprehensive review. Artif Intell Rev 55(1):3503–3568
MacNee W, Rabinovich RA, Choudhury G (2014) Ageing and the border between health and disease. Eur Respir J 44(5):1332–1352
Moqri M, Herzog C, Poganik JR, Justice J, Belsky DW, Higgins-Chen A, Moskalev A, Fuellen G, Cohen AA, Bautmans I et al (2023) Biomarkers of aging for the identification and evaluation of longevity interventions. Cell 186(18):3758–3775
Wu X, Xiao L, Sun Y, Zhang J, Ma T, He L (2022) A survey of human-in-the-loop for machine learning. Futur Gener Comput Syst 135:364–381
Chekroud AM, Hawrilenko M, Loho H, Bondar J, Gueorguieva R, Hasan A, Kambeitz J, Corlett PR, Koutsouleris N, Krumholz HM et al (2024) Illusory generalizability of clinical prediction models. Science 383(6679):164–167
Baltrušaitis T, Ahuja C, Morency L-P (2018) Multimodal machine learning: a survey and taxonomy. IEEE Trans Pattern Anal Mach Intell 41(2):423–443
World Health Organization (2021) Ethics and governance of artificial intelligence for health: WHO guidance. Technical report, World Health Organization, Geneva, Switzerland
European Union (2021) Proposal for a regulation of the European Parliament and the Council laying down harmonised rules on Artificial Intelligence (Artificial Intelligence Act) and amending certain Union legislative acts. EUR-Lex 52021PC0206:1–108
European Union (2023) Artificial Intelligence Act: deal on comprehensive rules for trustworthy AI. Document 20231206IPR15699, Press Release
López-Otín C, Blasco MA, Partridge L, Serrano M, Kroemer G (2023) Hallmarks of aging: an expanding universe. Cell 186(2):243–278
Navarro C, Salazar J, Díaz MP, Chacin M, Santeliz R, Vera I, Parra H, Bernal MC, Castro A, Escalona D et al (2023) Intrinsic and environmental basis of aging: a narrative review. Heliyon. 9(8):18239
World Health Organization (2019) Integrated care for older people (ICOPE): guidance for person-centred assessment and pathways in primary care. Technical report, World Health Organization, Geneva, Switzerland
Piau A, Steinmeyer Z, Cesari M, Kornfeld J, Beattie Z, Kaye J, Vellas B, Nourhashemi F (2021) Intrinsic capacitiy monitoring by digital biomarkers in Integrated Care For Older People (ICOPE). The Journal of Frailty & Aging. 10:132–138
Download references
Open Access funding provided thanks to the CRUE-CSIC agreement with Springer Nature. This work is supported by Ministerio de Ciencia, Innovación y Universidades, Gobierno de España (Agencia Estatal de Investigación, Fondo Europeo de Desarrollo Regional -FEDER-, European Union) under the research grant PID2021-127409OB-C33 CONDOR. Also, this work is partially founded by AGAUR research group 2021SGR-00111 “ASCLEPIUS: Smart Technology for Smart Healthcare”. This work is also supported by International Experts Program promoted by the Ministerio de Ciencia, Tecnología e Innovación through Instituto Colombiano de Crédito Educativo y Estudios Técnicos en el Exterior - ICETEX, and internal funds for research strengthening from Universidad Simón Bolívar, Vicerrectoría de Investigación, Extensión e Innovación, Colombia.
Author information
Authors and affiliations.
Smart Technologies Research Group, Department of Computer Engineering and Mathematics, Universitat Rovira i Virgili, Av. Països Catalans 26, 43007, Tarragona, Spain
Mary Carlota Bernal, Edgar Batista, Antoni Martínez-Ballesté & Agusti Solanas
Facultad de Ingenierías, Centro de Crecimiento Empresarial - MACONDOLAB, Universidad Simón Bolívar, Av. 3, 13-34, Cúcuta, Colombia
Mary Carlota Bernal
You can also search for this author in PubMed Google Scholar
Contributions
Mary Carlota Bernal : Conceptualisation, Methodology, Validation, Formal analysis, Visualisation, Data curation, Writing - Original Draft. Edgar Batista : Methodology, Formal analysis, Visualisation, Writing - Original Draft, Writing - Review & Editing. Antoni Martínez-Ballesté : Methodology, Formal analysis, Writing - Review & Editing, Supervision, Funding acquisition. Agusti Solanas : Conceptualisation, Methodology, Formal analysis, Writing - Review & Editing, Supervision, Funding acquisition.
Corresponding author
Correspondence to Edgar Batista .
Ethics declarations
Conflict of interest.
The authors declare that they have no known competing financial interests or personal relationships that could have appeared to influence the work reported in this paper.
Ethics Approval and Consent to Participate
Consent for publication, materials availability, additional information, publisher's note.
Springer Nature remains neutral with regard to jurisdictional claims in published maps and institutional affiliations.
Rights and permissions
Open Access This article is licensed under a Creative Commons Attribution 4.0 International License, which permits use, sharing, adaptation, distribution and reproduction in any medium or format, as long as you give appropriate credit to the original author(s) and the source, provide a link to the Creative Commons licence, and indicate if changes were made. The images or other third party material in this article are included in the article’s Creative Commons licence, unless indicated otherwise in a credit line to the material. If material is not included in the article’s Creative Commons licence and your intended use is not permitted by statutory regulation or exceeds the permitted use, you will need to obtain permission directly from the copyright holder. To view a copy of this licence, visit http://creativecommons.org/licenses/by/4.0/ .
Reprints and permissions
About this article
Bernal, M., Batista, E., Martínez-Ballesté, A. et al. Artificial intelligence for the study of human ageing: a systematic literature review. Appl Intell (2024). https://doi.org/10.1007/s10489-024-05817-z
Download citation
Accepted : 24 August 2024
Published : 06 September 2024
DOI : https://doi.org/10.1007/s10489-024-05817-z
Share this article
Anyone you share the following link with will be able to read this content:
Sorry, a shareable link is not currently available for this article.
Provided by the Springer Nature SharedIt content-sharing initiative
- Artificial intelligence
- Machine learning
- Ageing datasets
- Older adults
- Find a journal
- Publish with us
- Track your research

An official website of the United States government
The .gov means it’s official. Federal government websites often end in .gov or .mil. Before sharing sensitive information, make sure you’re on a federal government site.
The site is secure. The https:// ensures that you are connecting to the official website and that any information you provide is encrypted and transmitted securely.
- Publications
- Account settings
Preview improvements coming to the PMC website in October 2024. Learn More or Try it out now .
- Advanced Search
- Journal List
- Perspect Clin Res
- v.11(2); Apr-Jun 2020
Study designs: Part 7 – Systematic reviews
Priya ranganathan.
Department of Anaesthesiology, Tata Memorial Centre, Homi Bhabha National Institute, Mumbai, Maharashtra, India
Rakesh Aggarwal
1 Director, Jawaharlal Institute of Postgraduate Medical Education and Research, Puducherry, India
In this series on research study designs, we have so far looked at different types of primary research designs which attempt to answer a specific question. In this segment, we discuss systematic review, which is a study design used to summarize the results of several primary research studies. Systematic reviews often also use meta-analysis, which is a statistical tool to mathematically collate the results of various research studies to obtain a pooled estimate of treatment effect; this will be discussed in the next article.
In the previous six articles in this series on study designs, we have looked at different types of primary research study designs which are used to answer research questions. In this article, we describe the systematic review, a type of secondary research design that is used to summarize the results of prior primary research studies. Systematic reviews are considered the highest level of evidence for a particular research question.[ 1 ]
SYSTEMATIC REVIEWS
As defined in the Cochrane Handbook for Systematic Reviews of Interventions , “Systematic reviews seek to collate evidence that fits pre-specified eligibility criteria in order to answer a specific research question. They aim to minimize bias by using explicit, systematic methods documented in advance with a protocol.”[ 2 ]
NARRATIVE VERSUS SYSTEMATIC REVIEWS
Review of available data has been done since times immemorial. However, the traditional narrative reviews (“expert reviews”) do not involve a systematic search of the literature. Instead, the author of the review, usually an expert on the subject, used informal methods to identify (what he or she thinks are) the key studies on the topic. The final review thus is a summary of these “selected” studies. Since studies are chosen at will (haphazardly!) and without clearly defined criteria, such reviews preferentially include those studies that favor the author's views, leading to a potential for subjectivity or selection bias.
In contrast, systematic reviews involve a formal prespecified protocol with explicit, transparent criteria for the inclusion and exclusion of studies, thereby ensuring completeness of coverage of the available evidence, and providing a more objective, replicable, and comprehensive overview it.
META-ANALYSIS
Many systematic reviews use an additional tool, known as meta-analysis, which is a statistical technique for combining the results of multiple studies in a systematic review in a mathematically appropriate way, to create a single (pooled) and more precise estimate of treatment effect. The feasibility of performing a meta-analysis in a systematic review depends on the number of studies included in the final review and the degree of heterogeneity in the inclusion criteria as well as the results between the included studies. Meta-analysis will be discussed in detail in the next article in this series.
THE PROCESS OF A SYSTEMATIC REVIEW
The conduct of a systematic review involves several sequential key steps.[ 3 , 4 ] As in other research study designs, a clearly stated research question and a well-written research protocol are essential before commencing a systematic review.
Step 1: Stating the review question
Systematic reviews can be carried out in any field of medical research, e.g. efficacy or safety of interventions, diagnostics, screening or health economics. In this article, we focus on systematic reviews of studies looking at the efficacy of interventions. As for the other study designs, for a systematic review too, the question is best framed using the Population, Intervention, Comparator, and Outcome (PICO) format.
For example, Safi et al . carried out a systematic review on the effect of beta-blockers on the outcomes of patients with myocardial infarction.[ 5 ] In this review, the Population was patients with suspected or confirmed myocardial infarction, the Intervention was beta-blocker therapy, the Comparator was either placebo or no intervention, and the Outcomes were all-cause mortality and major adverse cardiovascular events. The review question was “ In patients with suspected or confirmed myocardial infarction, does the use of beta-blockers affect mortality or major adverse cardiovascular outcomes? ”
Step 2: Listing the eligibility criteria for studies to be included
It is essential to explicitly define a priori the criteria for selection of studies which will be included in the review. Besides the PICO components, some additional criteria used frequently for this purpose include language of publication (English versus non-English), publication status (published as full paper versus unpublished), study design (randomized versus quasi-experimental), age group (adults versus children), and publication year (e.g. in the last 5 years, or since a particular date). The PICO criteria used may not be very specific, e.g. it is possible to include studies that use one or the other drug belonging to the same group. For instance, the systematic review by Safi et al . included all randomized clinical trials, irrespective of setting, blinding, publication status, publication year, or language, and reported outcomes, that had used any beta-blocker and in a broad range of doses.[ 5 ]
Step 3: Comprehensive search for studies that meet the eligibility criteria
A thorough literature search is essential to identify all articles related to the research question and to ensure that no relevant article is left out. The search may include one or more electronic databases and trial registries; in addition, it is common to hand-search the cross-references in the articles identified through such searches. One could also plan to reach out to experts in the field to identify unpublished data, and to search the grey literature non-peer-reviewednon-peer-reviewed. This last option is particularly helpful non-pharmacologic (theses, conference abstracts, and non-peer-reviewed journals). These sources are particularly helpful when the intervention is relatively new, since data on these may not yet have been published as full papers and hence are unlikely to be found in literature databases. In the review by Safi et al ., the search strategy included not only several electronic databases (Cochrane, MEDLINE, EMBASE, LILACS, etc.) but also other resources (e.g. Google Scholar, WHO International Clinical Trial Registry Platform, and reference lists of identified studies).[ 5 ] It is not essential to include all the above databases in one's search. However, it is mandatory to define in advance which of these will be searched.
Step 4: Identifying and selecting relevant studies
Once the search strategy defined in the previous step has been run to identify potentially relevant studies, a two-step process is followed. First, the titles and abstracts of the identified studies are processed to exclude any duplicates and to discard obviously irrelevant studies. In the next step, full-text papers of the remaining articles are retrieved and closely reviewed to identify studies that meet the eligibility criteria. To minimize bias, these selection steps are usually performed independently by at least two reviewers, who also assign a reason for non-selection to each discarded study. Any discrepancies are then resolved either by an independent reviewer or by mutual consensus of the original reviewers. In the Cochrane review on beta-blockers referred to above, two review authors independently screened the titles for inclusion, and then, four review authors independently reviewed the screen-positive studies to identify the trials to be included in the final review.[ 5 ] Disagreements were resolved by discussion or by taking the opinion of a separate reviewer. A summary of this selection process, showing the degree of agreement between reviewers, and a flow diagram that depicts the numbers of screened, included and excluded (with reason for exclusion) studies are often included in the final review.
Step 5: Data extraction
In this step, from each selected study, relevant data are extracted. This should be done by at least two reviewers independently, and the data then compared to identify any errors in extraction. Standard data extraction forms help in objective data extraction. The data extracted usually contain the name of the author, the year of publication, details of intervention and control treatments, and the number of participants and outcome data in each group. In the review by Safi et al ., four review authors independently extracted data and resolved any differences by discussion.[ 5 ]
Handling missing data
Some of the studies included in the review may not report outcomes in accordance with the review methodology. Such missing data can be handled in two ways – by contacting authors of the original study to obtain the necessary data and by using data imputation techniques. Safi et al . used both these approaches – they tried to get data from the trial authors; however, where that failed, they analyzed the primary outcome (mortality) using the best case (i.e. presuming that all the participants in the experimental arm with missing data had survived and those in the control arm with missing mortality data had died – representing the maximum beneficial effect of the intervention) and the worst case (all the participants with missing data in the experimental arm assumed to have died and those in the control arm to have survived – representing the least beneficial effect of the intervention) scenarios.
Evaluating the quality (or risk of bias) in the included studies
The overall quality of a systematic review depends on the quality of each of the included studies. Quality of a study is inversely proportional to the potential for bias in its design. In our previous articles on interventional study design in this series, we discussed various methods to reduce bias – such as randomization, allocation concealment, participant and assessor blinding, using objective endpoints, minimizing missing data, the use of intention-to-treat analysis, and complete reporting of all outcomes.[ 6 , 7 ] These features form the basis of the Cochrane Risk of Bias Tool (RoB 2), which is a commonly used instrument to assess the risk of bias in the studies included in a systematic review.[ 8 ] Based on this tool, one can classify each study in a review as having low risk of bias, having some concerns regarding bias, or at high risk of bias. Safi et al . used this tool to classify the included studies as having low or high risk of bias and presented these data in both tabular and graphical formats.[ 5 ]
In some reviews, the authors decide to summarize only studies with a low risk of bias and to exclude those with a high risk of bias. Alternatively, some authors undertake a separate analysis of studies with low risk of bias, besides an analysis of all the studies taken together. The conclusions from such analyses of only high-quality studies may be more robust.
Step 6: Synthesis of results
The data extracted from various studies are pooled quantitatively (known as a meta-analysis) or qualitatively (if pooling of results is not considered feasible). For qualitative reviews, data are usually presented in the tabular format, showing the characteristics of each included study, to allow for easier interpretation.
Sensitivity analyses
Sensitivity analyses are used to test the robustness of the results of a systematic review by examining the impact of excluding or including studies with certain characteristics. As referred to above, this can be based on the risk of bias (methodological quality), studies with a specific study design, studies with a certain dosage or schedule, or sample size. If results of these different analyses are more-or-less the same, one can be more certain of the validity of the findings of the review. Furthermore, such analyses can help identify whether the effect of the intervention could vary across different levels of another factor. In the beta-blocker review, sensitivity analysis was performed depending on the risk of bias of included studies.[ 5 ]
IMPORTANT RESOURCES FOR CARRYING OUT SYSTEMATIC REVIEWS AND META-ANALYSES
Cochrane is an organization that works to produce good-quality, updated systematic reviews related to human healthcare and policy, which are accessible to people across the world.[ 9 ] There are more than 7000 Cochrane reviews on various topics. One of its main resources is the Cochrane Library (available at https://www.cochranelibrary.com/ ), which incorporates several databases with different types of high-quality evidence to inform healthcare decisions, including the Cochrane Database of Systematic Reviews, Cochrane Central Register of Controlled Trials (CENTRAL), and Cochrane Clinical Answers.
The Cochrane Handbook for Systematic Reviews of Interventions
The Cochrane handbook is an official guide, prepared by the Cochrane Collaboration, to the process of preparing and maintaining Cochrane systematic reviews.[ 10 ]
Review Manager software
Review Manager (RevMan) is a software developed by Cochrane to support the preparation and maintenance of systematic reviews, including tools for performing meta-analysis.[ 11 ] It is freely available in both online (RevMan Web) and offline (RevMan 5.3) versions.
Preferred Reporting Items for Systematic Reviews and Meta-Analyses statement
The Preferred Reporting Items for Systematic Reviews and Meta-Analyses (PRISMA) statement is an evidence-based minimum set of items for reporting of systematic reviews and meta-analyses of randomized trials.[ 12 ] It can be used both by authors of such studies to improve the completeness of reporting and by reviewers and readers to critically appraise a systematic review. There are several extensions to the PRISMA statement for specific types of reviews. An update is currently underway.
Meta-analysis of Observational Studies in Epidemiology statement
The Meta-analysis of Observational Studies in Epidemiology statement summarizes the recommendations for reporting of meta-analyses in epidemiology.[ 13 ]
PROSPERO is an international database for prospective registration of protocols for systematic reviews in healthcare.[ 14 ] It aims to avoid duplication of and to improve transparency in reporting of results of such reviews.
Financial support and sponsorship
Conflicts of interest.
There are no conflicts of interest.
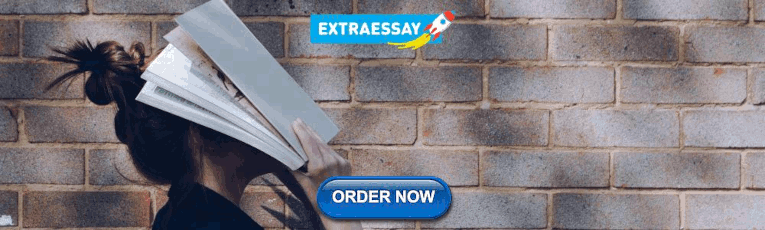
IMAGES
VIDEO
COMMENTS
If the included studies in a systematic review measure an outcome differently, their reported results may be ... Tetzlaff J, Sampson M, Tricco AC, et al. Epidemiology and reporting characteristics of systematic reviews of biomedical research: a cross-sectional study. PLoS Med. 2016; 13 (5):1-31. doi: 10.1371/journal.pmed.1002028. [PMC free ...
Topic selection and planning. In recent years, there has been an explosion in the number of systematic reviews conducted and published (Chalmers & Fox 2016, Fontelo & Liu 2018, Page et al 2015) - although a systematic review may be an inappropriate or unnecessary research methodology for answering many research questions.Systematic reviews can be inadvisable for a variety of reasons.
Systematic reviews are characterized by a methodical and replicable methodology and presentation. They involve a comprehensive search to locate all relevant published and unpublished work on a subject; a systematic integration of search results; and a critique of the extent, nature, and quality of evidence in relation to a particular research question.
A systematic review is a type of review that uses repeatable methods to find, select, and synthesize all available evidence. It answers a clearly formulated research question and explicitly states the methods used to arrive at the answer. Example: Systematic review. In 2008, Dr. Robert Boyle and his colleagues published a systematic review in ...
In recent years, there has been an explosion in the number of systematic reviews conducted and published (Chalmers & Fox 2016, Fontelo & Liu 2018, Page et al 2015) - although a systematic review may be an inappropriate or unnecessary research methodology for answering many research questions.Systematic reviews can be inadvisable for a variety of reasons.
Systematic reviews involve the application of scientific methods to reduce bias in review of literature. The key components of a systematic review are a well-defined research question, comprehensive literature search to identify all studies that potentially address the question, systematic assembly of the studies that answer the question, critical appraisal of the methodological quality of the ...
Guidelines for writing a systematic review. 1. Introduction. A key feature of any academic activity is to have a sufficient understanding of the subject area under investigation and thus an awareness of previous research. Undertaking a literature review with an analysis of the results on a specific issue is required to demonstrate sufficient ...
A systematic review is a scholarly synthesis of the evidence on a clearly presented topic using critical methods to identify, define and assess research on the topic. [1] A systematic review extracts and interprets data from published studies on the topic (in the scientific literature), then analyzes, describes, critically appraises and ...
A systematic review aims to bring evidence together to answer a pre-defined research question. This involves the identification of all primary research relevant to the defined review question, the critical appraisal of this research, and the synthesis of the findings.13 Systematic reviews may combine data from different.
A systematic review identifies and synthesizes all relevant studies that fit prespecified criteria to answer a research question (Lasserson et al. 2019; IOM 2011).What sets a systematic review apart from a narrative review is that it follows consistent, rigorous, and transparent methods established in a protocol in order to minimize bias and errors.
Systematic reviews are characterized by a methodical and replicable methodology and presentation. They involve a comprehensive search to locate all relevant published and unpublished work on a subject; a systematic integration of search results; and a critique of the extent, nature, and quality of evidence in relation to a particular research question. The best reviews synthesize studies to ...
Systematic Review | Definition, Examples & Guide - Scribbr
Systematic reviews can help us know what we know about a topic, and what is not yet known, often to a greater extent than the findings of a single study. 4 The process is comprehensive enough to establish consistency and generalizability of research findings across settings and populations. 3 A meta-analysis is a type of systematic review that ...
A systematic review is a type of study that synthesises research that has been conducted on a particular topic. Systematic reviews are considered to provide the highest level of evidence on the hierarchy of evidence pyramid. Systematic reviews are conducted following rigorous research methodology. To minimise bias, systematic reviews utilise a ...
A systematic review attempts to collate all empirical evidence that fits pre-specified eligibility criteria in order to answer a specific research question. ... a systematic presentation, and synthesis, of the characteristics and findings of the included studies. Many systematic reviews contain meta-analyses. Meta-analysis is the use of ...
To review systematically research studies, which have investigated the impact of gray literature in meta‐analyses of randomized trials of health care interventions. ... To investigate the effectiveness of checking reference lists for the identification of additional, relevant studies for systematic reviews. Effectiveness is defined as the ...
Several CDC librarians have special training in conducting literature searches for systematic reviews. Literature searches for systematic reviews can take a few weeks to several months from planning to delivery. Fill out a search request form or contact the Stephen B. Thacker CDC Library by email [email protected] or telephone 404-639-1717.
an explicit, reproducible methodology. a systematic search that attempts to identify all studies that would meet the eligibility criteria. an assessment of the validity of the findings of the included studies, for example through the assessment of the risk of bias. a systematic presentation, and synthesis, of the characteristics and findings of ...
Literature reviews establish the foundation of academic inquires. However, in the planning field, we lack rigorous systematic reviews. In this article, through a systematic search on the methodology of literature review, we categorize a typology of literature reviews, discuss steps in conducting a systematic literature review, and provide suggestions on how to enhance rigor in literature ...
This systematic review was interested in comparing the diet quality of vegetarian and non-vegetarian diets. Twelve studies were included. Vegetarians more closely met recommendations for total fruit, whole grains, seafood and plant protein, and sodium intake. In nine of the twelve studies, vegetarians had higher overall diet quality compared to ...
In this re-review of 133 studies from two systematic reviews assessing effectiveness of water, sanitation and/or handwashing with soap interventions on diarrhoeal disease 1 and ARIs 2, we ...
Over the last few decades, a growing number of studies have used wearable technologies, such as inertial and pressure sensors, to investigate various domains of music experience, from performance to education. In this paper, we systematically review this body of literature using the PRISMA (Preferred Reporting Items for Systematic Reviews and Meta-Analyses) method. The initial search yielded a ...
With the view to address this challenge, the systematic review method was developed. Systematic reviews aim to inform and facilitate this process through research synthesis of multiple studies, enabling increased and efficient access to evidence.[1,3,4] Systematic reviews and meta-analyses have become increasingly important in healthcare settings.
Integrative Reviews: Similar to systematic reviews, but they aim to generate new knowledge by integrating findings from different studies to develop new theories or frameworks. Importance of Literature Reviews. Foundation for Research: They provide a solid background for new research projects, helping to justify the research question and ...
Objective:There are significant temporal and financial barriers for individuals with personality disorders (PD) receiving evidence-based psychological treatments. Emerging research indicates Group Schema Therapy (GST) may be an accessible, efficient, and cost-effective PD intervention, however, there has been no synthesis of the available evidence to date. This review therefore aimed to ...
It is easy to confuse systematic reviews and meta-analyses. A systematic review is an objective, reproducible method to find answers to a certain research question, by collecting all available studies related to that question and reviewing and analyzing their results. A meta-analysis differs from a systematic review in that it uses statistical ...
The studies of this systematic review leverage ML techniques for different purposes, namely prediction (i.e., estimating or forecasting future values or outcomes using historical data or observed characteristics), detection (i.e., identifying the presence or absence of specific events or patterns in datasets), classification (i.e., assigning ...
This new systematic review of human observational studies is based on a much larger data set compared to what the IARC examined in 2011. It includes more recent and more comprehensive studies.
Study designs: Part 7 - Systematic reviews. In this series on research study designs, we have so far looked at different types of primary research designs which attempt to answer a specific question. In this segment, we discuss systematic review, which is a study design used to summarize the results of several primary research studies.