- Privacy Policy

Home » Validity – Types, Examples and Guide
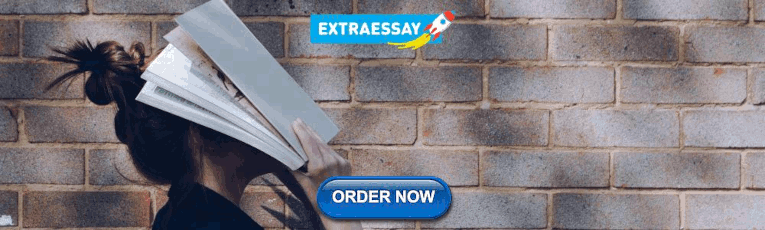
Validity – Types, Examples and Guide
Table of Contents

Validity is a fundamental concept in research, referring to the extent to which a test, measurement, or study accurately reflects or assesses the specific concept that the researcher is attempting to measure. Ensuring validity is crucial as it determines the trustworthiness and credibility of the research findings.
Research Validity
Research validity pertains to the accuracy and truthfulness of the research. It examines whether the research truly measures what it claims to measure. Without validity, research results can be misleading or erroneous, leading to incorrect conclusions and potentially flawed applications.
How to Ensure Validity in Research
Ensuring validity in research involves several strategies:
- Clear Operational Definitions : Define variables clearly and precisely.
- Use of Reliable Instruments : Employ measurement tools that have been tested for reliability.
- Pilot Testing : Conduct preliminary studies to refine the research design and instruments.
- Triangulation : Use multiple methods or sources to cross-verify results.
- Control Variables : Control extraneous variables that might influence the outcomes.
Types of Validity
Validity is categorized into several types, each addressing different aspects of measurement accuracy.
Internal Validity
Internal validity refers to the degree to which the results of a study can be attributed to the treatments or interventions rather than other factors. It is about ensuring that the study is free from confounding variables that could affect the outcome.
External Validity
External validity concerns the extent to which the research findings can be generalized to other settings, populations, or times. High external validity means the results are applicable beyond the specific context of the study.
Construct Validity
Construct validity evaluates whether a test or instrument measures the theoretical construct it is intended to measure. It involves ensuring that the test is truly assessing the concept it claims to represent.
Content Validity
Content validity examines whether a test covers the entire range of the concept being measured. It ensures that the test items represent all facets of the concept.
Criterion Validity
Criterion validity assesses how well one measure predicts an outcome based on another measure. It is divided into two types:
- Predictive Validity : How well a test predicts future performance.
- Concurrent Validity : How well a test correlates with a currently existing measure.
Face Validity
Face validity refers to the extent to which a test appears to measure what it is supposed to measure, based on superficial inspection. While it is the least scientific measure of validity, it is important for ensuring that stakeholders believe in the test’s relevance.
Importance of Validity
Validity is crucial because it directly affects the credibility of research findings. Valid results ensure that conclusions drawn from research are accurate and can be trusted. This, in turn, influences the decisions and policies based on the research.
Examples of Validity
- Internal Validity : A randomized controlled trial (RCT) where the random assignment of participants helps eliminate biases.
- External Validity : A study on educational interventions that can be applied to different schools across various regions.
- Construct Validity : A psychological test that accurately measures depression levels.
- Content Validity : An exam that covers all topics taught in a course.
- Criterion Validity : A job performance test that predicts future job success.
Where to Write About Validity in A Thesis
In a thesis, the methodology section should include discussions about validity. Here, you explain how you ensured the validity of your research instruments and design. Additionally, you may discuss validity in the results section, interpreting how the validity of your measurements affects your findings.
Applications of Validity
Validity has wide applications across various fields:
- Education : Ensuring assessments accurately measure student learning.
- Psychology : Developing tests that correctly diagnose mental health conditions.
- Market Research : Creating surveys that accurately capture consumer preferences.
Limitations of Validity
While ensuring validity is essential, it has its limitations:
- Complexity : Achieving high validity can be complex and resource-intensive.
- Context-Specific : Some validity types may not be universally applicable across all contexts.
- Subjectivity : Certain types of validity, like face validity, involve subjective judgments.
By understanding and addressing these aspects of validity, researchers can enhance the quality and impact of their studies, leading to more reliable and actionable results.
About the author
Muhammad Hassan
Researcher, Academic Writer, Web developer
You may also like

External Validity – Threats, Examples and Types

Internal Consistency Reliability – Methods...

Split-Half Reliability – Methods, Examples and...

Criterion Validity – Methods, Examples and...

Construct Validity – Types, Threats and Examples

Internal Validity – Threats, Examples and Guide
Validity in research: a guide to measuring the right things
Last updated
27 February 2023
Reviewed by
Cathy Heath
Short on time? Get an AI generated summary of this article instead
Validity is necessary for all types of studies ranging from market validation of a business or product idea to the effectiveness of medical trials and procedures. So, how can you determine whether your research is valid? This guide can help you understand what validity is, the types of validity in research, and the factors that affect research validity.
Make research less tedious
Dovetail streamlines research to help you uncover and share actionable insights
- What is validity?
In the most basic sense, validity is the quality of being based on truth or reason. Valid research strives to eliminate the effects of unrelated information and the circumstances under which evidence is collected.
Validity in research is the ability to conduct an accurate study with the right tools and conditions to yield acceptable and reliable data that can be reproduced. Researchers rely on carefully calibrated tools for precise measurements. However, collecting accurate information can be more of a challenge.
Studies must be conducted in environments that don't sway the results to achieve and maintain validity. They can be compromised by asking the wrong questions or relying on limited data.
Why is validity important in research?
Research is used to improve life for humans. Every product and discovery, from innovative medical breakthroughs to advanced new products, depends on accurate research to be dependable. Without it, the results couldn't be trusted, and products would likely fail. Businesses would lose money, and patients couldn't rely on medical treatments.
While wasting money on a lousy product is a concern, lack of validity paints a much grimmer picture in the medical field or producing automobiles and airplanes, for example. Whether you're launching an exciting new product or conducting scientific research, validity can determine success and failure.
- What is reliability?
Reliability is the ability of a method to yield consistency. If the same result can be consistently achieved by using the same method to measure something, the measurement method is said to be reliable. For example, a thermometer that shows the same temperatures each time in a controlled environment is reliable.
While high reliability is a part of measuring validity, it's only part of the puzzle. If the reliable thermometer hasn't been properly calibrated and reliably measures temperatures two degrees too high, it doesn't provide a valid (accurate) measure of temperature.
Similarly, if a researcher uses a thermometer to measure weight, the results won't be accurate because it's the wrong tool for the job.
- How are reliability and validity assessed?
While measuring reliability is a part of measuring validity, there are distinct ways to assess both measurements for accuracy.
How is reliability measured?
These measures of consistency and stability help assess reliability, including:
Consistency and stability of the same measure when repeated multiple times and conditions
Consistency and stability of the measure across different test subjects
Consistency and stability of results from different parts of a test designed to measure the same thing
How is validity measured?
Since validity refers to how accurately a method measures what it is intended to measure, it can be difficult to assess the accuracy. Validity can be estimated by comparing research results to other relevant data or theories.
The adherence of a measure to existing knowledge of how the concept is measured
The ability to cover all aspects of the concept being measured
The relation of the result in comparison with other valid measures of the same concept
- What are the types of validity in a research design?
Research validity is broadly gathered into two groups: internal and external. Yet, this grouping doesn't clearly define the different types of validity. Research validity can be divided into seven distinct groups.
Face validity : A test that appears valid simply because of the appropriateness or relativity of the testing method, included information, or tools used.
Content validity : The determination that the measure used in research covers the full domain of the content.
Construct validity : The assessment of the suitability of the measurement tool to measure the activity being studied.
Internal validity : The assessment of how your research environment affects measurement results. This is where other factors can’t explain the extent of an observed cause-and-effect response.
External validity : The extent to which the study will be accurate beyond the sample and the level to which it can be generalized in other settings, populations, and measures.
Statistical conclusion validity: The determination of whether a relationship exists between procedures and outcomes (appropriate sampling and measuring procedures along with appropriate statistical tests).
Criterion-related validity : A measurement of the quality of your testing methods against a criterion measure (like a “gold standard” test) that is measured at the same time.
- Examples of validity
Like different types of research and the various ways to measure validity, examples of validity can vary widely. These include:
A questionnaire may be considered valid because each question addresses specific and relevant aspects of the study subject.
In a brand assessment study, researchers can use comparison testing to verify the results of an initial study. For example, the results from a focus group response about brand perception are considered more valid when the results match that of a questionnaire answered by current and potential customers.
A test to measure a class of students' understanding of the English language contains reading, writing, listening, and speaking components to cover the full scope of how language is used.
- Factors that affect research validity
Certain factors can affect research validity in both positive and negative ways. By understanding the factors that improve validity and those that threaten it, you can enhance the validity of your study. These include:
Random selection of participants vs. the selection of participants that are representative of your study criteria
Blinding with interventions the participants are unaware of (like the use of placebos)
Manipulating the experiment by inserting a variable that will change the results
Randomly assigning participants to treatment and control groups to avoid bias
Following specific procedures during the study to avoid unintended effects
Conducting a study in the field instead of a laboratory for more accurate results
Replicating the study with different factors or settings to compare results
Using statistical methods to adjust for inconclusive data
What are the common validity threats in research, and how can their effects be minimized or nullified?
Research validity can be difficult to achieve because of internal and external threats that produce inaccurate results. These factors can jeopardize validity.
History: Events that occur between an early and later measurement
Maturation: The passage of time in a study can include data on actions that would have naturally occurred outside of the settings of the study
Repeated testing: The outcome of repeated tests can change the outcome of followed tests
Selection of subjects: Unconscious bias which can result in the selection of uniform comparison groups
Statistical regression: Choosing subjects based on extremes doesn't yield an accurate outcome for the majority of individuals
Attrition: When the sample group is diminished significantly during the course of the study
Maturation: When subjects mature during the study, and natural maturation is awarded to the effects of the study
While some validity threats can be minimized or wholly nullified, removing all threats from a study is impossible. For example, random selection can remove unconscious bias and statistical regression.
Researchers can even hope to avoid attrition by using smaller study groups. Yet, smaller study groups could potentially affect the research in other ways. The best practice for researchers to prevent validity threats is through careful environmental planning and t reliable data-gathering methods.
- How to ensure validity in your research
Researchers should be mindful of the importance of validity in the early planning stages of any study to avoid inaccurate results. Researchers must take the time to consider tools and methods as well as how the testing environment matches closely with the natural environment in which results will be used.
The following steps can be used to ensure validity in research:
Choose appropriate methods of measurement
Use appropriate sampling to choose test subjects
Create an accurate testing environment
How do you maintain validity in research?
Accurate research is usually conducted over a period of time with different test subjects. To maintain validity across an entire study, you must take specific steps to ensure that gathered data has the same levels of accuracy.
Consistency is crucial for maintaining validity in research. When researchers apply methods consistently and standardize the circumstances under which data is collected, validity can be maintained across the entire study.
Is there a need for validation of the research instrument before its implementation?
An essential part of validity is choosing the right research instrument or method for accurate results. Consider the thermometer that is reliable but still produces inaccurate results. You're unlikely to achieve research validity without activities like calibration, content, and construct validity.
- Understanding research validity for more accurate results
Without validity, research can't provide the accuracy necessary to deliver a useful study. By getting a clear understanding of validity in research, you can take steps to improve your research skills and achieve more accurate results.
Should you be using a customer insights hub?
Do you want to discover previous research faster?
Do you share your research findings with others?
Do you analyze research data?
Start for free today, add your research, and get to key insights faster
Editor’s picks
Last updated: 18 April 2023
Last updated: 27 February 2023
Last updated: 22 August 2024
Last updated: 5 February 2023
Last updated: 16 August 2024
Last updated: 9 March 2023
Last updated: 30 April 2024
Last updated: 12 December 2023
Last updated: 11 March 2024
Last updated: 4 July 2024
Last updated: 6 March 2024
Last updated: 5 March 2024
Last updated: 13 May 2024
Latest articles
Related topics, .css-je19u9{-webkit-align-items:flex-end;-webkit-box-align:flex-end;-ms-flex-align:flex-end;align-items:flex-end;display:-webkit-box;display:-webkit-flex;display:-ms-flexbox;display:flex;-webkit-flex-direction:row;-ms-flex-direction:row;flex-direction:row;-webkit-box-flex-wrap:wrap;-webkit-flex-wrap:wrap;-ms-flex-wrap:wrap;flex-wrap:wrap;-webkit-box-pack:center;-ms-flex-pack:center;-webkit-justify-content:center;justify-content:center;row-gap:0;text-align:center;max-width:671px;}@media (max-width: 1079px){.css-je19u9{max-width:400px;}.css-je19u9>span{white-space:pre;}}@media (max-width: 799px){.css-je19u9{max-width:400px;}.css-je19u9>span{white-space:pre;}} decide what to .css-1kiodld{max-height:56px;display:-webkit-box;display:-webkit-flex;display:-ms-flexbox;display:flex;-webkit-align-items:center;-webkit-box-align:center;-ms-flex-align:center;align-items:center;}@media (max-width: 1079px){.css-1kiodld{display:none;}} build next, decide what to build next, log in or sign up.
Get started for free
Have a language expert improve your writing
Run a free plagiarism check in 10 minutes, automatically generate references for free.
- Knowledge Base
- Methodology
- Reliability vs Validity in Research | Differences, Types & Examples
Reliability vs Validity in Research | Differences, Types & Examples
Published on 3 May 2022 by Fiona Middleton . Revised on 10 October 2022.
Reliability and validity are concepts used to evaluate the quality of research. They indicate how well a method , technique, or test measures something. Reliability is about the consistency of a measure, and validity is about the accuracy of a measure.
It’s important to consider reliability and validity when you are creating your research design , planning your methods, and writing up your results, especially in quantitative research .
Reliability | Validity | |
---|---|---|
What does it tell you? | The extent to which the results can be reproduced when the research is repeated under the same conditions. | The extent to which the results really measure what they are supposed to measure. |
How is it assessed? | By checking the consistency of results across time, across different observers, and across parts of the test itself. | By checking how well the results correspond to established theories and other measures of the same concept. |
How do they relate? | A reliable measurement is not always valid: the results might be reproducible, but they’re not necessarily correct. | A valid measurement is generally reliable: if a test produces accurate results, they should be . |
Table of contents
Understanding reliability vs validity, how are reliability and validity assessed, how to ensure validity and reliability in your research, where to write about reliability and validity in a thesis.
Reliability and validity are closely related, but they mean different things. A measurement can be reliable without being valid. However, if a measurement is valid, it is usually also reliable.
What is reliability?
Reliability refers to how consistently a method measures something. If the same result can be consistently achieved by using the same methods under the same circumstances, the measurement is considered reliable.
What is validity?
Validity refers to how accurately a method measures what it is intended to measure. If research has high validity, that means it produces results that correspond to real properties, characteristics, and variations in the physical or social world.
High reliability is one indicator that a measurement is valid. If a method is not reliable, it probably isn’t valid.
However, reliability on its own is not enough to ensure validity. Even if a test is reliable, it may not accurately reflect the real situation.
Validity is harder to assess than reliability, but it is even more important. To obtain useful results, the methods you use to collect your data must be valid: the research must be measuring what it claims to measure. This ensures that your discussion of the data and the conclusions you draw are also valid.
Prevent plagiarism, run a free check.
Reliability can be estimated by comparing different versions of the same measurement. Validity is harder to assess, but it can be estimated by comparing the results to other relevant data or theory. Methods of estimating reliability and validity are usually split up into different types.
Types of reliability
Different types of reliability can be estimated through various statistical methods.
Type of reliability | What does it assess? | Example |
---|---|---|
The consistency of a measure : do you get the same results when you repeat the measurement? | A group of participants complete a designed to measure personality traits. If they repeat the questionnaire days, weeks, or months apart and give the same answers, this indicates high test-retest reliability. | |
The consistency of a measure : do you get the same results when different people conduct the same measurement? | Based on an assessment criteria checklist, five examiners submit substantially different results for the same student project. This indicates that the assessment checklist has low inter-rater reliability (for example, because the criteria are too subjective). | |
The consistency of : do you get the same results from different parts of a test that are designed to measure the same thing? | You design a questionnaire to measure self-esteem. If you randomly split the results into two halves, there should be a between the two sets of results. If the two results are very different, this indicates low internal consistency. |
Types of validity
The validity of a measurement can be estimated based on three main types of evidence. Each type can be evaluated through expert judgement or statistical methods.
Type of validity | What does it assess? | Example |
---|---|---|
The adherence of a measure to of the concept being measured. | A self-esteem questionnaire could be assessed by measuring other traits known or assumed to be related to the concept of self-esteem (such as social skills and optimism). Strong correlation between the scores for self-esteem and associated traits would indicate high construct validity. | |
The extent to which the measurement of the concept being measured. | A test that aims to measure a class of students’ level of Spanish contains reading, writing, and speaking components, but no listening component. Experts agree that listening comprehension is an essential aspect of language ability, so the test lacks content validity for measuring the overall level of ability in Spanish. | |
The extent to which the result of a measure corresponds to of the same concept. | A is conducted to measure the political opinions of voters in a region. If the results accurately predict the later outcome of an election in that region, this indicates that the survey has high criterion validity. |
To assess the validity of a cause-and-effect relationship, you also need to consider internal validity (the design of the experiment ) and external validity (the generalisability of the results).
The reliability and validity of your results depends on creating a strong research design , choosing appropriate methods and samples, and conducting the research carefully and consistently.
Ensuring validity
If you use scores or ratings to measure variations in something (such as psychological traits, levels of ability, or physical properties), it’s important that your results reflect the real variations as accurately as possible. Validity should be considered in the very earliest stages of your research, when you decide how you will collect your data .
- Choose appropriate methods of measurement
Ensure that your method and measurement technique are of high quality and targeted to measure exactly what you want to know. They should be thoroughly researched and based on existing knowledge.
For example, to collect data on a personality trait, you could use a standardised questionnaire that is considered reliable and valid. If you develop your own questionnaire, it should be based on established theory or the findings of previous studies, and the questions should be carefully and precisely worded.
- Use appropriate sampling methods to select your subjects
To produce valid generalisable results, clearly define the population you are researching (e.g., people from a specific age range, geographical location, or profession). Ensure that you have enough participants and that they are representative of the population.
Ensuring reliability
Reliability should be considered throughout the data collection process. When you use a tool or technique to collect data, it’s important that the results are precise, stable, and reproducible.
- Apply your methods consistently
Plan your method carefully to make sure you carry out the same steps in the same way for each measurement. This is especially important if multiple researchers are involved.
For example, if you are conducting interviews or observations, clearly define how specific behaviours or responses will be counted, and make sure questions are phrased the same way each time.
- Standardise the conditions of your research
When you collect your data, keep the circumstances as consistent as possible to reduce the influence of external factors that might create variation in the results.
For example, in an experimental setup, make sure all participants are given the same information and tested under the same conditions.
It’s appropriate to discuss reliability and validity in various sections of your thesis or dissertation or research paper. Showing that you have taken them into account in planning your research and interpreting the results makes your work more credible and trustworthy.
Section | Discuss |
---|---|
What have other researchers done to devise and improve methods that are reliable and valid? | |
How did you plan your research to ensure reliability and validity of the measures used? This includes the chosen sample set and size, sample preparation, external conditions, and measuring techniques. | |
If you calculate reliability and validity, state these values alongside your main results. | |
This is the moment to talk about how reliable and valid your results actually were. Were they consistent, and did they reflect true values? If not, why not? | |
If reliability and validity were a big problem for your findings, it might be helpful to mention this here. |
Cite this Scribbr article
If you want to cite this source, you can copy and paste the citation or click the ‘Cite this Scribbr article’ button to automatically add the citation to our free Reference Generator.
Middleton, F. (2022, October 10). Reliability vs Validity in Research | Differences, Types & Examples. Scribbr. Retrieved 3 September 2024, from https://www.scribbr.co.uk/research-methods/reliability-or-validity/
Is this article helpful?
Fiona Middleton
Other students also liked, the 4 types of validity | types, definitions & examples, a quick guide to experimental design | 5 steps & examples, sampling methods | types, techniques, & examples.

Validity & Reliability In Research
A Plain-Language Explanation (With Examples)
By: Derek Jansen (MBA) | Expert Reviewer: Kerryn Warren (PhD) | September 2023
Validity and reliability are two related but distinctly different concepts within research. Understanding what they are and how to achieve them is critically important to any research project. In this post, we’ll unpack these two concepts as simply as possible.
This post is based on our popular online course, Research Methodology Bootcamp . In the course, we unpack the basics of methodology using straightfoward language and loads of examples. If you’re new to academic research, you definitely want to use this link to get 50% off the course (limited-time offer).
Overview: Validity & Reliability
- The big picture
- Validity 101
- Reliability 101
- Key takeaways
First, The Basics…
First, let’s start with a big-picture view and then we can zoom in to the finer details.
Validity and reliability are two incredibly important concepts in research, especially within the social sciences. Both validity and reliability have to do with the measurement of variables and/or constructs – for example, job satisfaction, intelligence, productivity, etc. When undertaking research, you’ll often want to measure these types of constructs and variables and, at the simplest level, validity and reliability are about ensuring the quality and accuracy of those measurements .
As you can probably imagine, if your measurements aren’t accurate or there are quality issues at play when you’re collecting your data, your entire study will be at risk. Therefore, validity and reliability are very important concepts to understand (and to get right). So, let’s unpack each of them.
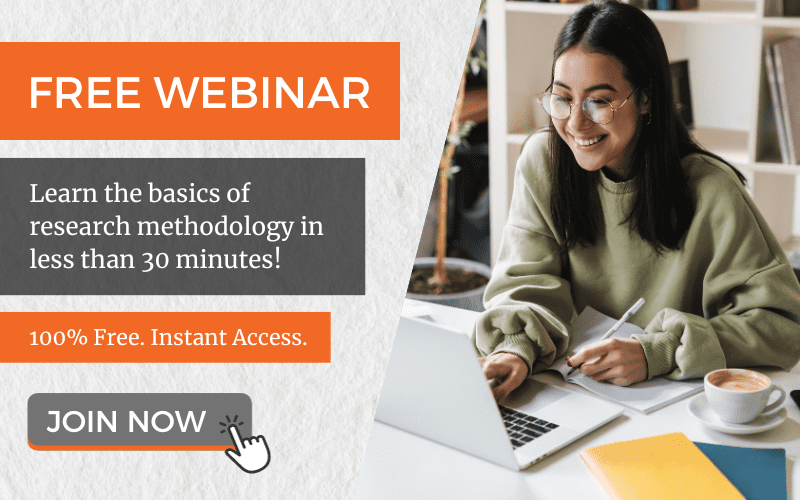
What Is Validity?
In simple terms, validity (also called “construct validity”) is all about whether a research instrument accurately measures what it’s supposed to measure .
For example, let’s say you have a set of Likert scales that are supposed to quantify someone’s level of overall job satisfaction. If this set of scales focused purely on only one dimension of job satisfaction, say pay satisfaction, this would not be a valid measurement, as it only captures one aspect of the multidimensional construct. In other words, pay satisfaction alone is only one contributing factor toward overall job satisfaction, and therefore it’s not a valid way to measure someone’s job satisfaction.
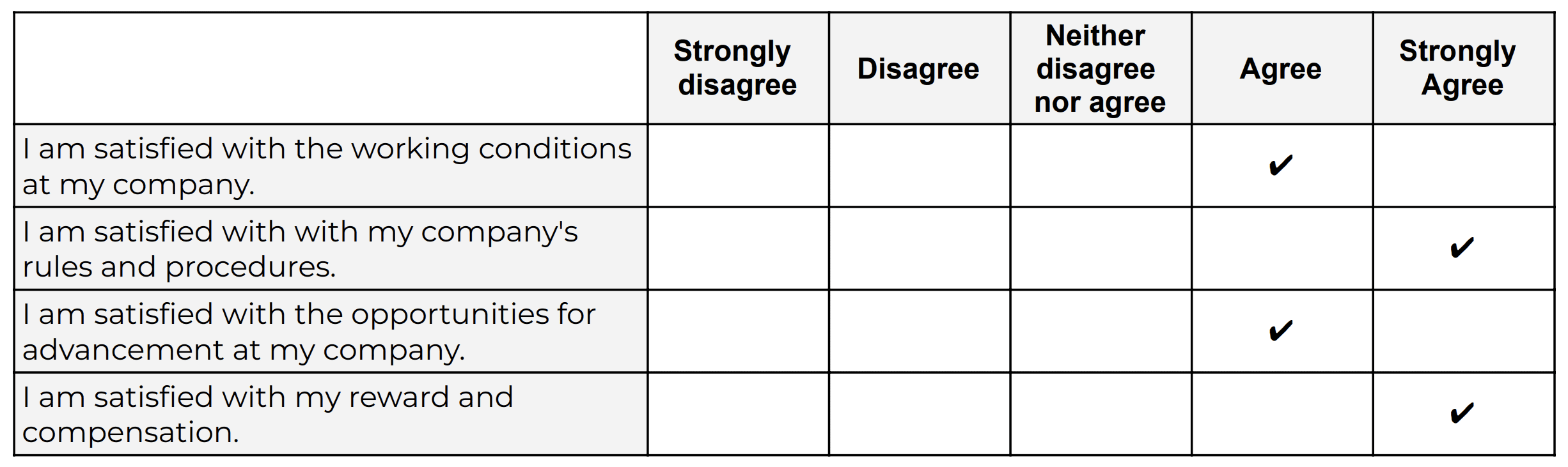
Oftentimes in quantitative studies, the way in which the researcher or survey designer interprets a question or statement can differ from how the study participants interpret it . Given that respondents don’t have the opportunity to ask clarifying questions when taking a survey, it’s easy for these sorts of misunderstandings to crop up. Naturally, if the respondents are interpreting the question in the wrong way, the data they provide will be pretty useless . Therefore, ensuring that a study’s measurement instruments are valid – in other words, that they are measuring what they intend to measure – is incredibly important.
There are various types of validity and we’re not going to go down that rabbit hole in this post, but it’s worth quickly highlighting the importance of making sure that your research instrument is tightly aligned with the theoretical construct you’re trying to measure . In other words, you need to pay careful attention to how the key theories within your study define the thing you’re trying to measure – and then make sure that your survey presents it in the same way.
For example, sticking with the “job satisfaction” construct we looked at earlier, you’d need to clearly define what you mean by job satisfaction within your study (and this definition would of course need to be underpinned by the relevant theory). You’d then need to make sure that your chosen definition is reflected in the types of questions or scales you’re using in your survey . Simply put, you need to make sure that your survey respondents are perceiving your key constructs in the same way you are. Or, even if they’re not, that your measurement instrument is capturing the necessary information that reflects your definition of the construct at hand.
If all of this talk about constructs sounds a bit fluffy, be sure to check out Research Methodology Bootcamp , which will provide you with a rock-solid foundational understanding of all things methodology-related. Remember, you can take advantage of our 60% discount offer using this link.
Need a helping hand?
What Is Reliability?
As with validity, reliability is an attribute of a measurement instrument – for example, a survey, a weight scale or even a blood pressure monitor. But while validity is concerned with whether the instrument is measuring the “thing” it’s supposed to be measuring, reliability is concerned with consistency and stability . In other words, reliability reflects the degree to which a measurement instrument produces consistent results when applied repeatedly to the same phenomenon , under the same conditions .
As you can probably imagine, a measurement instrument that achieves a high level of consistency is naturally more dependable (or reliable) than one that doesn’t – in other words, it can be trusted to provide consistent measurements . And that, of course, is what you want when undertaking empirical research. If you think about it within a more domestic context, just imagine if you found that your bathroom scale gave you a different number every time you hopped on and off of it – you wouldn’t feel too confident in its ability to measure the variable that is your body weight 🙂
It’s worth mentioning that reliability also extends to the person using the measurement instrument . For example, if two researchers use the same instrument (let’s say a measuring tape) and they get different measurements, there’s likely an issue in terms of how one (or both) of them are using the measuring tape. So, when you think about reliability, consider both the instrument and the researcher as part of the equation.
As with validity, there are various types of reliability and various tests that can be used to assess the reliability of an instrument. A popular one that you’ll likely come across for survey instruments is Cronbach’s alpha , which is a statistical measure that quantifies the degree to which items within an instrument (for example, a set of Likert scales) measure the same underlying construct . In other words, Cronbach’s alpha indicates how closely related the items are and whether they consistently capture the same concept .
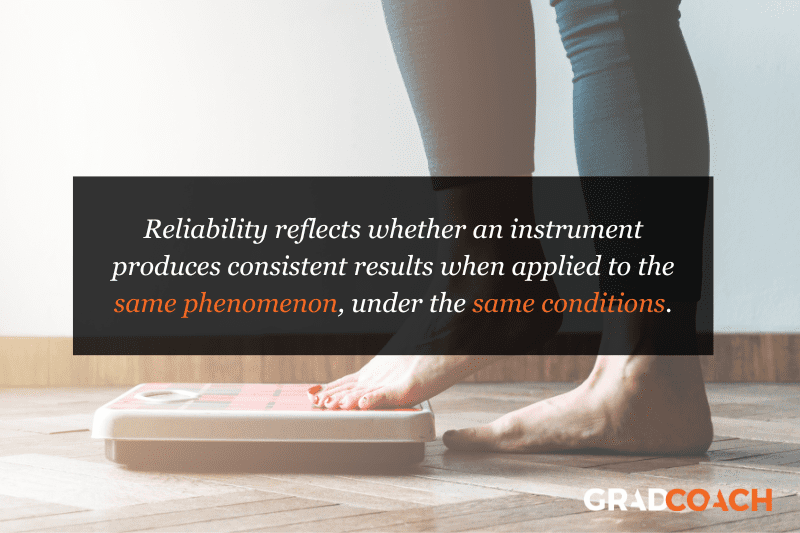
Recap: Key Takeaways
Alright, let’s quickly recap to cement your understanding of validity and reliability:
- Validity is concerned with whether an instrument (e.g., a set of Likert scales) is measuring what it’s supposed to measure
- Reliability is concerned with whether that measurement is consistent and stable when measuring the same phenomenon under the same conditions.
In short, validity and reliability are both essential to ensuring that your data collection efforts deliver high-quality, accurate data that help you answer your research questions . So, be sure to always pay careful attention to the validity and reliability of your measurement instruments when collecting and analysing data. As the adage goes, “rubbish in, rubbish out” – make sure that your data inputs are rock-solid.
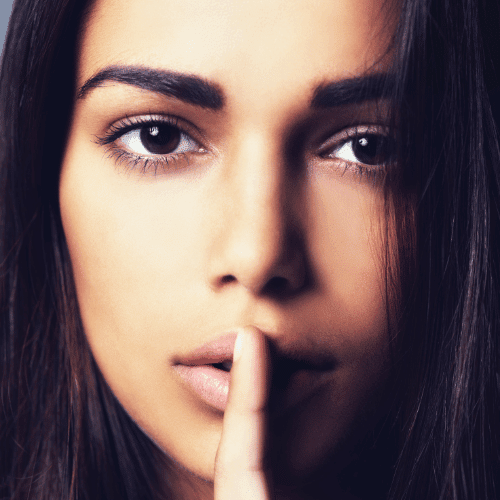
Psst… there’s more!
This post is an extract from our bestselling short course, Methodology Bootcamp . If you want to work smart, you don't want to miss this .
THE MATERIAL IS WONDERFUL AND BENEFICIAL TO ALL STUDENTS.
THE MATERIAL IS WONDERFUL AND BENEFICIAL TO ALL STUDENTS AND I HAVE GREATLY BENEFITED FROM THE CONTENT.
Submit a Comment Cancel reply
Your email address will not be published. Required fields are marked *
Save my name, email, and website in this browser for the next time I comment.
- Print Friendly

Understanding Reliability and Validity
These related research issues ask us to consider whether we are studying what we think we are studying and whether the measures we use are consistent.
Reliability
Reliability is the extent to which an experiment, test, or any measuring procedure yields the same result on repeated trials. Without the agreement of independent observers able to replicate research procedures, or the ability to use research tools and procedures that yield consistent measurements, researchers would be unable to satisfactorily draw conclusions, formulate theories, or make claims about the generalizability of their research. In addition to its important role in research, reliability is critical for many parts of our lives, including manufacturing, medicine, and sports.
Reliability is such an important concept that it has been defined in terms of its application to a wide range of activities. For researchers, four key types of reliability are:
Equivalency Reliability
Equivalency reliability is the extent to which two items measure identical concepts at an identical level of difficulty. Equivalency reliability is determined by relating two sets of test scores to one another to highlight the degree of relationship or association. In quantitative studies and particularly in experimental studies, a correlation coefficient, statistically referred to as r , is used to show the strength of the correlation between a dependent variable (the subject under study), and one or more independent variables , which are manipulated to determine effects on the dependent variable. An important consideration is that equivalency reliability is concerned with correlational, not causal, relationships.
For example, a researcher studying university English students happened to notice that when some students were studying for finals, their holiday shopping began. Intrigued by this, the researcher attempted to observe how often, or to what degree, this these two behaviors co-occurred throughout the academic year. The researcher used the results of the observations to assess the correlation between studying throughout the academic year and shopping for gifts. The researcher concluded there was poor equivalency reliability between the two actions. In other words, studying was not a reliable predictor of shopping for gifts.
Stability Reliability
Stability reliability (sometimes called test, re-test reliability) is the agreement of measuring instruments over time. To determine stability, a measure or test is repeated on the same subjects at a future date. Results are compared and correlated with the initial test to give a measure of stability.
An example of stability reliability would be the method of maintaining weights used by the U.S. Bureau of Standards. Platinum objects of fixed weight (one kilogram, one pound, etc...) are kept locked away. Once a year they are taken out and weighed, allowing scales to be reset so they are "weighing" accurately. Keeping track of how much the scales are off from year to year establishes a stability reliability for these instruments. In this instance, the platinum weights themselves are assumed to have a perfectly fixed stability reliability.
Internal Consistency
Internal consistency is the extent to which tests or procedures assess the same characteristic, skill or quality. It is a measure of the precision between the observers or of the measuring instruments used in a study. This type of reliability often helps researchers interpret data and predict the value of scores and the limits of the relationship among variables.
For example, a researcher designs a questionnaire to find out about college students' dissatisfaction with a particular textbook. Analyzing the internal consistency of the survey items dealing with dissatisfaction will reveal the extent to which items on the questionnaire focus on the notion of dissatisfaction.
Interrater Reliability
Interrater reliability is the extent to which two or more individuals (coders or raters) agree. Interrater reliability addresses the consistency of the implementation of a rating system.
A test of interrater reliability would be the following scenario: Two or more researchers are observing a high school classroom. The class is discussing a movie that they have just viewed as a group. The researchers have a sliding rating scale (1 being most positive, 5 being most negative) with which they are rating the student's oral responses. Interrater reliability assesses the consistency of how the rating system is implemented. For example, if one researcher gives a "1" to a student response, while another researcher gives a "5," obviously the interrater reliability would be inconsistent. Interrater reliability is dependent upon the ability of two or more individuals to be consistent. Training, education and monitoring skills can enhance interrater reliability.
Related Information: Reliability Example
An example of the importance of reliability is the use of measuring devices in Olympic track and field events. For the vast majority of people, ordinary measuring rulers and their degree of accuracy are reliable enough. However, for an Olympic event, such as the discus throw, the slightest variation in a measuring device -- whether it is a tape, clock, or other device -- could mean the difference between the gold and silver medals. Additionally, it could mean the difference between a new world record and outright failure to qualify for an event. Olympic measuring devices, then, must be reliable from one throw or race to another and from one competition to another. They must also be reliable when used in different parts of the world, as temperature, air pressure, humidity, interpretation, or other variables might affect their readings.
Validity refers to the degree to which a study accurately reflects or assesses the specific concept that the researcher is attempting to measure. While reliability is concerned with the accuracy of the actual measuring instrument or procedure, validity is concerned with the study's success at measuring what the researchers set out to measure.
Researchers should be concerned with both external and internal validity. External validity refers to the extent to which the results of a study are generalizable or transferable. (Most discussions of external validity focus solely on generalizability; see Campbell and Stanley, 1966. We include a reference here to transferability because many qualitative research studies are not designed to be generalized.)
Internal validity refers to (1) the rigor with which the study was conducted (e.g., the study's design, the care taken to conduct measurements, and decisions concerning what was and wasn't measured) and (2) the extent to which the designers of a study have taken into account alternative explanations for any causal relationships they explore (Huitt, 1998). In studies that do not explore causal relationships, only the first of these definitions should be considered when assessing internal validity.
Scholars discuss several types of internal validity. For brief discussions of several types of internal validity, click on the items below:
Face Validity
Face validity is concerned with how a measure or procedure appears. Does it seem like a reasonable way to gain the information the researchers are attempting to obtain? Does it seem well designed? Does it seem as though it will work reliably? Unlike content validity, face validity does not depend on established theories for support (Fink, 1995).
Criterion Related Validity
Criterion related validity, also referred to as instrumental validity, is used to demonstrate the accuracy of a measure or procedure by comparing it with another measure or procedure which has been demonstrated to be valid.
For example, imagine a hands-on driving test has been shown to be an accurate test of driving skills. By comparing the scores on the written driving test with the scores from the hands-on driving test, the written test can be validated by using a criterion related strategy in which the hands-on driving test is compared to the written test.
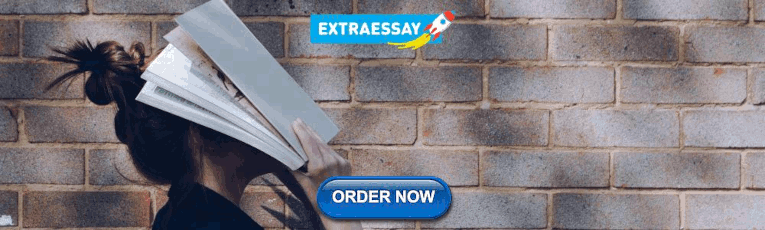
Construct Validity
Construct validity seeks agreement between a theoretical concept and a specific measuring device or procedure. For example, a researcher inventing a new IQ test might spend a great deal of time attempting to "define" intelligence in order to reach an acceptable level of construct validity.
Construct validity can be broken down into two sub-categories: Convergent validity and discriminate validity. Convergent validity is the actual general agreement among ratings, gathered independently of one another, where measures should be theoretically related. Discriminate validity is the lack of a relationship among measures which theoretically should not be related.
To understand whether a piece of research has construct validity, three steps should be followed. First, the theoretical relationships must be specified. Second, the empirical relationships between the measures of the concepts must be examined. Third, the empirical evidence must be interpreted in terms of how it clarifies the construct validity of the particular measure being tested (Carmines & Zeller, p. 23).
Content Validity
Content Validity is based on the extent to which a measurement reflects the specific intended domain of content (Carmines & Zeller, 1991, p.20).
Content validity is illustrated using the following examples: Researchers aim to study mathematical learning and create a survey to test for mathematical skill. If these researchers only tested for multiplication and then drew conclusions from that survey, their study would not show content validity because it excludes other mathematical functions. Although the establishment of content validity for placement-type exams seems relatively straight-forward, the process becomes more complex as it moves into the more abstract domain of socio-cultural studies. For example, a researcher needing to measure an attitude like self-esteem must decide what constitutes a relevant domain of content for that attitude. For socio-cultural studies, content validity forces the researchers to define the very domains they are attempting to study.
Related Information: Validity Example
Many recreational activities of high school students involve driving cars. A researcher, wanting to measure whether recreational activities have a negative effect on grade point average in high school students, might conduct a survey asking how many students drive to school and then attempt to find a correlation between these two factors. Because many students might use their cars for purposes other than or in addition to recreation (e.g., driving to work after school, driving to school rather than walking or taking a bus), this research study might prove invalid. Even if a strong correlation was found between driving and grade point average, driving to school in and of itself would seem to be an invalid measure of recreational activity.
The challenges of achieving reliability and validity are among the most difficult faced by researchers. In this section, we offer commentaries on these challenges.
Difficulties of Achieving Reliability
It is important to understand some of the problems concerning reliability which might arise. It would be ideal to reliably measure, every time, exactly those things which we intend to measure. However, researchers can go to great lengths and make every attempt to ensure accuracy in their studies, and still deal with the inherent difficulties of measuring particular events or behaviors. Sometimes, and particularly in studies of natural settings, the only measuring device available is the researcher's own observations of human interaction or human reaction to varying stimuli. As these methods are ultimately subjective in nature, results may be unreliable and multiple interpretations are possible. Three of these inherent difficulties are quixotic reliability, diachronic reliability and synchronic reliability.
Quixotic reliability refers to the situation where a single manner of observation consistently, yet erroneously, yields the same result. It is often a problem when research appears to be going well. This consistency might seem to suggest that the experiment was demonstrating perfect stability reliability. This, however, would not be the case.
For example, if a measuring device used in an Olympic competition always read 100 meters for every discus throw, this would be an example of an instrument consistently, yet erroneously, yielding the same result. However, quixotic reliability is often more subtle in its occurrences than this. For example, suppose a group of German researchers doing an ethnographic study of American attitudes ask questions and record responses. Parts of their study might produce responses which seem reliable, yet turn out to measure felicitous verbal embellishments required for "correct" social behavior. Asking Americans, "How are you?" for example, would in most cases, elicit the token, "Fine, thanks." However, this response would not accurately represent the mental or physical state of the respondents.
Diachronic reliability refers to the stability of observations over time. It is similar to stability reliability in that it deals with time. While this type of reliability is appropriate to assess features that remain relatively unchanged over time, such as landscape benchmarks or buildings, the same level of reliability is more difficult to achieve with socio-cultural phenomena.
For example, in a follow-up study one year later of reading comprehension in a specific group of school children, diachronic reliability would be hard to achieve. If the test were given to the same subjects a year later, many confounding variables would have impacted the researchers' ability to reproduce the same circumstances present at the first test. The final results would almost assuredly not reflect the degree of stability sought by the researchers.
Synchronic reliability refers to the similarity of observations within the same time frame; it is not about the similarity of things observed. Synchronic reliability, unlike diachronic reliability, rarely involves observations of identical things. Rather, it concerns itself with particularities of interest to the research.
For example, a researcher studies the actions of a duck's wing in flight and the actions of a hummingbird's wing in flight. Despite the fact that the researcher is studying two distinctly different kinds of wings, the action of the wings and the phenomenon produced is the same.
Comments on a Flawed, Yet Influential Study
An example of the dangers of generalizing from research that is inconsistent, invalid, unreliable, and incomplete is found in the Time magazine article, "On A Screen Near You: Cyberporn" (De Witt, 1995). This article relies on a study done at Carnegie Mellon University to determine the extent and implications of online pornography. Inherent to the study are methodological problems of unqualified hypotheses and conclusions, unsupported generalizations and a lack of peer review.
Ignoring the functional problems that manifest themselves later in the study, it seems that there are a number of ethical problems within the article. The article claims to be an exhaustive study of pornography on the Internet, (it was anything but exhaustive), it resembles a case study more than anything else. Marty Rimm, author of the undergraduate paper that Time used as a basis for the article, claims the paper was an "exhaustive study" of online pornography when, in fact, the study based most of its conclusions about pornography on the Internet on the "descriptions of slightly more than 4,000 images" (Meeks, 1995, p. 1). Some USENET groups see hundreds of postings in a day.
Considering the thousands of USENET groups, 4,000 images no longer carries the authoritative weight that its author intended. The real problem is that the study (an undergraduate paper similar to a second-semester composition assignment) was based not on pornographic images themselves, but on the descriptions of those images. This kind of reduction detracts significantly from the integrity of the final claims made by the author. In fact, this kind of research is commensurate with doing a study of the content of pornographic movies based on the titles of the movies, then making sociological generalizations based on what those titles indicate. (This is obviously a problem with a number of types of validity, because Rimm is not studying what he thinks he is studying, but instead something quite different. )
The author of the Time article, Philip Elmer De Witt writes, "The research team at CMU has undertaken the first systematic study of pornography on the Information Superhighway" (Godwin, 1995, p. 1). His statement is problematic in at least three ways. First, the research team actually consisted of a few of Rimm's undergraduate friends with no methodological training whatsoever. Additionally, no mention of the degree of interrater reliability is made. Second, this systematic study is actually merely a "non-randomly selected subset of commercial bulletin-board systems that focus on selling porn" (Godwin, p. 6). As pornography vending is actually just a small part of the whole concerning the use of pornography on the Internet, the entire premise of this study's content validity is firmly called into question. Finally, the use of the term "Information Superhighway" is a false assessment of what in actuality is only a few USENET groups and BBSs (Bulletin Board System), which make up only a small fraction of the entire "Information Superhighway" traffic. Essentially, what is here is yet another violation of content validity.
De Witt is quoted as saying: "In an 18-month study, the team surveyed 917,410 sexually-explicit pictures, descriptions, short-stories and film clips. On those USENET newsgroups where digitized images are stored, 83.5 percent of the pictures were pornographic" (De Witt 40).
Statistically, some interesting contradictions arise. The figure 917,410 was taken from adult-oriented BBSs--none came from actual USENET groups or the Internet itself. This is a glaring discrepancy. Out of the 917,410 files, 212,114 are only descriptions (Hoffman & Novak, 1995, p.2). The question is, how many actual images did the "researchers" see?
"Between April and July 1994, the research team downloaded all available images (3,254)...the team encountered technical difficulties with 13 percent of these images...This left a total of 2,830 images for analysis" (p. 2). This means that out of 917,410 files discussed in this study, 914,580 of them were not even pictures! As for the 83.5 percent figure, this is actually based on "17 alt.binaries groups that Rimm considered pornographic" (p. 2).
In real terms, 17 USENET groups is a fraction of a percent of all USENET groups available. Worse yet, Time claimed that "...only about 3 percent of all messages on the USENET [represent pornographic material], while the USENET itself represents 11.5 percent of the traffic on the Internet" (De Witt, p. 40).
Time neglected to carry the interpretation of this data out to its logical conclusion, which is that less than half of 1 percent (3 percent of 11 percent) of the images on the Internet are associated with newsgroups that contain pornographic imagery. Furthermore, of this half percent, an unknown but even smaller percentage of the messages in newsgroups that are 'associated with pornographic imagery', actually contained pornographic material (Hoffman & Novak, p. 3).
Another blunder can be seen in the avoidance of peer-review, which suggests that there was some political interests being served in having the study become a Time cover story. Marty Rimm contracted the Georgetown Law Review and Time in an agreement to publish his study as long as they kept it under lock and key. During the months before publication, many interested scholars and professionals tried in vain to obtain a copy of the study in order to check it for flaws. De Witt justified not letting such peer-review take place, and also justified the reliability and validity of the study, on the grounds that because the Georgetown Law Review had accepted it, it was therefore reliable and valid, and needed no peer-review. What he didn't know, was that law reviews are not edited by professionals, but by "third year law students" (Godwin, p. 4).
There are many consequences of the failure to subject such a study to the scrutiny of peer review. If it was Rimm's desire to publish an article about on-line pornography in a manner that legitimized his article, yet escaped the kind of critical review the piece would have to undergo if published in a scholarly journal of computer-science, engineering, marketing, psychology, or communications. What better venue than a law journal? A law journal article would have the added advantage of being taken seriously by law professors, lawyers, and legally-trained policymakers. By virtue of where it appeared, it would automatically be catapulted into the center of the policy debate surrounding online censorship and freedom of speech (Godwin).
Herein lies the dangerous implication of such a study: Because the questions surrounding pornography are of such immediate political concern, the study was placed in the forefront of the U.S. domestic policy debate over censorship on the Internet, (an integral aspect of current anti-First Amendment legislation) with little regard for its validity or reliability.
On June 26, the day the article came out, Senator Grassley, (co-sponsor of the anti-porn bill, along with Senator Dole) began drafting a speech that was to be delivered that very day in the Senate, using the study as evidence. The same day, at the same time, Mike Godwin posted on WELL (Whole Earth 'Lectronic Link, a forum for professionals on the Internet) what turned out to be the overstatement of the year: "Philip's story is an utter disaster, and it will damage the debate about this issue because we will have to spend lots of time correcting misunderstandings that are directly attributable to the story" (Meeks, p. 7).
As Godwin was writing this, Senator Grassley was speaking to the Senate: "Mr. President, I want to repeat that: 83.5 percent of the 900,000 images reviewed--these are all on the Internet--are pornographic, according to the Carnegie-Mellon study" ( p. 7). Several days later, Senator Dole was waving the magazine in front of the Senate like a battle flag.
Donna Hoffman, professor at Vanderbilt University, summed up the dangerous political implications by saying, "The critically important national debate over First Amendment rights and restrictions of information on the Internet and other emerging media requires facts and informed opinion, not hysteria" (p.1).
In addition to the hysteria, Hoffman sees a plethora of other problems with the study. "Because the content analysis and classification scheme are 'black boxes,'" Hoffman said, "because no reliability and validity results are presented, because no statistical testing of the differences both within and among categories for different types of listings has been performed, and because not a single hypothesis has been tested, formally or otherwise, no conclusions should be drawn until the issues raised in this critique are resolved" (p. 4).
However, the damage has already been done. This questionable research by an undergraduate engineering major has been generalized to such an extent that even the U.S. Senate, and in particular Senators Grassley and Dole, have been duped, albeit through the strength of their own desires to see only what they wanted to see.
Annotated Bibliography
American Psychological Association. (1985). Standards for educational and psychological testing. Washington, DC: Author.
This work on focuses on reliability, validity and the standards that testers need to achieve in order to ensure accuracy.
Babbie, E.R. & Huitt, R.E. (1979). The practice of social research 2nd ed . Belmont, CA: Wadsworth Publishing.
An overview of social research and its applications.
Beauchamp, T. L., Faden, R.R., Wallace, Jr., R.J. & Walters, L . ( 1982). Ethical issues in social science research. Baltimore and London: The Johns Hopkins University Press.
A systematic overview of ethical issues in Social Science Research written by researchers with firsthand familiarity with the situations and problems researchers face in their work. This book raises several questions of how reliability and validity can be affected by ethics.
Borman, K.M. et al. (1986). Ethnographic and qualitative research design and why it doesn't work. American behavioral scientist 30 , 42-57.
The authors pose questions concerning threats to qualitative research and suggest solutions.
Bowen, K. A. (1996, Oct. 12). The sin of omission -punishable by death to internal validity: An argument for integration of quantitative research methods to strengthen internal validity. Available: http://trochim.human.cornell.edu/gallery/bowen/hss691.htm
An entire Web site that examines the merits of integrating qualitative and quantitative research methodologies through triangulation. The author argues that improving the internal validity of social science will be the result of such a union.
Brinberg, D. & McGrath, J.E. (1985). Validity and the research process . Beverly Hills: Sage Publications.
The authors investigate validity as value and propose the Validity Network Schema, a process by which researchers can infuse validity into their research.
Bussières, J-F. (1996, Oct.12). Reliability and validity of information provided by museum Web sites. Available: http://www.oise.on.ca/~jfbussieres/issue.html
This Web page examines the validity of museum Web sites which calls into question the validity of Web-based resources in general. Addresses the issue that all Websites should be examined with skepticism about the validity of the information contained within them.
Campbell, D. T. & Stanley, J.C. (1963). Experimental and quasi-experimental designs for research. Boston: Houghton Mifflin.
An overview of experimental research that includes pre-experimental designs, controls for internal validity, and tables listing sources of invalidity in quasi-experimental designs. Reference list and examples.
Carmines, E. G. & Zeller, R.A. (1991). Reliability and validity assessment . Newbury Park: Sage Publications.
An introduction to research methodology that includes classical test theory, validity, and methods of assessing reliability.
Carroll, K. M. (1995). Methodological issues and problems in the assessment of substance use. Psychological Assessment, Sep. 7 n3 , 349-58.
Discusses methodological issues in research involving the assessment of substance abuse. Introduces strategies for avoiding problems with the reliability and validity of methods.
Connelly, F. M. & Clandinin, D.J. (1990). Stories of experience and narrative inquiry. Educational Researcher 19:5 , 2-12.
A survey of narrative inquiry that outlines criteria, methods, and writing forms. It includes a discussion of risks and dangers in narrative studies, as well as a research agenda for curricula and classroom studies.
De Witt, P.E. (1995, July 3). On a screen near you: Cyberporn. Time, 38-45.
This is an exhaustive Carnegie Mellon study of online pornography by Marty Rimm, electrical engineering student.
Fink, A., ed. (1995). The survey Handbook, v.1 .Thousand Oaks, CA: Sage.
A guide to survey; this is the first in a series referred to as the "survey kit". It includes bibliograpgical references. Addresses survey design, analysis, reporting surveys and how to measure the validity and reliability of surveys.
Fink, A., ed. (1995). How to measure survey reliability and validity v. 7 . Thousand Oaks, CA: Sage.
This volume seeks to select and apply reliability criteria and select and apply validity criteria. The fundamental principles of scaling and scoring are considered.
Godwin, M. (1995, July). JournoPorn, dissection of the Time article. Available: http://www.hotwired.com
A detailed critique of Time magazine's Cyberporn , outlining flaws of methodology as well as exploring the underlying assumptions of the article.
Hambleton, R.K. & Zaal, J.N., eds. (1991). Advances in educational and psychological testing . Boston: Kluwer Academic.
Information on the concepts of reliability and validity in psychology and education.
Harnish, D.L. (1992). Human judgment and the logic of evidence: A critical examination of research methods in special education transition literature . In D.L. Harnish et al. eds., Selected readings in transition.
This article investigates threats to validity in special education research.
Haynes, N. M. (1995). How skewed is 'the bell curve'? Book Product Reviews . 1-24.
This paper claims that R.J. Herrnstein and C. Murray's The Bell Curve: Intelligence and Class Structure in American Life does not have scientific merit and claims that the bell curve is an unreliable measure of intelligence.
Healey, J. F. (1993). Statistics: A tool for social research, 3rd ed . Belmont: Wadsworth Publishing.
Inferential statistics, measures of association, and multivariate techniques in statistical analysis for social scientists are addressed.
Helberg, C. (1996, Oct.12). Pitfalls of data analysis (or how to avoid lies and damned lies). Available: http//maddog/fammed.wisc.edu/pitfalls/
A discussion of things researchers often overlook in their data analysis and how statistics are often used to skew reliability and validity for the researchers purposes.
Hoffman, D. L. and Novak, T.P. (1995, July). A detailed critique of the Time article: Cyberporn. Available: http://www.hotwired.com
A methodological critique of the Time article that uncovers some of the fundamental flaws in the statistics and the conclusions made by De Witt.
Huitt, William G. (1998). Internal and External Validity . http://www.valdosta.peachnet.edu/~whuitt/psy702/intro/valdgn.html
A Web document addressing key issues of external and internal validity.
Jones, J. E. & Bearley, W.L. (1996, Oct 12). Reliability and validity of training instruments. Organizational Universe Systems. Available: http://ous.usa.net/relval.htm
The authors discuss the reliability and validity of training design in a business setting. Basic terms are defined and examples provided.
Cultural Anthropology Methods Journal. (1996, Oct. 12). Available: http://www.lawrence.edu/~bradleyc/cam.html
An online journal containing articles on the practical application of research methods when conducting qualitative and quantitative research. Reliability and validity are addressed throughout.
Kirk, J. & Miller, M. M. (1986). Reliability and validity in qualitative research. Beverly Hills: Sage Publications.
This text describes objectivity in qualitative research by focusing on the issues of validity and reliability in terms of their limitations and applicability in the social and natural sciences.
Krakower, J. & Niwa, S. (1985). An assessment of validity and reliability of the institutinal perfarmance survey . Boulder, CO: National center for higher education management systems.
Educational surveys and higher education research and the efeectiveness of organization.
Lauer, J. M. & Asher, J.W. (1988). Composition Research. New York: Oxford University Press.
A discussion of empirical designs in the context of composition research as a whole.
Laurent, J. et al. (1992, Mar.) Review of validity research on the stanford-binet intelligence scale: 4th Ed. Psychological Assessment . 102-112.
This paper looks at the results of construct and criterion- related validity studies to determine if the SB:FE is a valid measure of intelligence.
LeCompte, M. D., Millroy, W.L., & Preissle, J. eds. (1992). The handbook of qualitative research in education. San Diego: Academic Press.
A compilation of the range of methodological and theoretical qualitative inquiry in the human sciences and education research. Numerous contributing authors apply their expertise to discussing a wide variety of issues pertaining to educational and humanities research as well as suggestions about how to deal with problems when conducting research.
McDowell, I. & Newell, C. (1987). Measuring health: A guide to rating scales and questionnaires . New York: Oxford University Press.
This gives a variety of examples of health measurement techniques and scales and discusses the validity and reliability of important health measures.
Meeks, B. (1995, July). Muckraker: How Time failed. Available: http://www.hotwired.com
A step-by-step outline of the events which took place during the researching, writing, and negotiating of the Time article of 3 July, 1995 titled: On A Screen Near You: Cyberporn .
Merriam, S. B. (1995). What can you tell from an N of 1?: Issues of validity and reliability in qualitative research. Journal of Lifelong Learning v4 , 51-60.
Addresses issues of validity and reliability in qualitative research for education. Discusses philosophical assumptions underlying the concepts of internal validity, reliability, and external validity or generalizability. Presents strategies for ensuring rigor and trustworthiness when conducting qualitative research.
Morris, L.L, Fitzgibbon, C.T., & Lindheim, E. (1987). How to measure performance and use tests. In J.L. Herman (Ed.), Program evaluation kit (2nd ed.). Newbury Park, CA: Sage.
Discussion of reliability and validity as it pertyains to measuring students' performance.
Murray, S., et al. (1979, April). Technical issues as threats to internal validity of experimental and quasi-experimental designs. San Francisco: University of California. 8-12.
(From Yang et al. bibliography--unavailable as of this writing.)
Russ-Eft, D. F. (1980). Validity and reliability in survey research. American Institutes for Research in the Behavioral Sciences August , 227 151.
An investigation of validity and reliability in survey research with and overview of the concepts of reliability and validity. Specific procedures for measuring sources of error are suggested as well as general suggestions for improving the reliability and validity of survey data. A extensive annotated bibliography is provided.
Ryser, G. R. (1994). Developing reliable and valid authentic assessments for the classroom: Is it possible? Journal of Secondary Gifted Education Fall, v6 n1 , 62-66.
Defines the meanings of reliability and validity as they apply to standardized measures of classroom assessment. This article defines reliability as scorability and stability and validity is seen as students' ability to use knowledge authentically in the field.
Schmidt, W., et al. (1982). Validity as a variable: Can the same certification test be valid for all students? Institute for Research on Teaching July, ED 227 151.
A technical report that presents specific criteria for judging content, instructional and curricular validity as related to certification tests in education.
Scholfield, P. (1995). Quantifying language. A researcher's and teacher's guide to gathering language data and reducing it to figures . Bristol: Multilingual Matters.
A guide to categorizing, measuring, testing, and assessing aspects of language. A source for language-related practitioners and researchers in conjunction with other resources on research methods and statistics. Questions of reliability, and validity are also explored.
Scriven, M. (1993). Hard-Won Lessons in Program Evaluation . San Francisco: Jossey-Bass Publishers.
A common sense approach for evaluating the validity of various educational programs and how to address specific issues facing evaluators.
Shou, P. (1993, Jan.). The singer loomis inventory of personality: A review and critique. [Paper presented at the Annual Meeting of the Southwest Educational Research Association.]
Evidence for reliability and validity are reviewed. A summary evaluation suggests that SLIP (developed by two Jungian analysts to allow examination of personality from the perspective of Jung's typology) appears to be a useful tool for educators and counselors.
Sutton, L.R. (1992). Community college teacher evaluation instrument: A reliability and validity study . Diss. Colorado State University.
Studies of reliability and validity in occupational and educational research.
Thompson, B. & Daniel, L.G. (1996, Oct.). Seminal readings on reliability and validity: A "hit parade" bibliography. Educational and psychological measurement v. 56 , 741-745.
Editorial board members of Educational and Psychological Measurement generated bibliography of definitive publications of measurement research. Many articles are directly related to reliability and validity.
Thompson, E. Y., et al. (1995). Overview of qualitative research . Diss. Colorado State University.
A discussion of strengths and weaknesses of qualitative research and its evolution and adaptation. Appendices and annotated bibliography.
Traver, C. et al. (1995). Case Study . Diss. Colorado State University.
This presentation gives an overview of case study research, providing definitions and a brief history and explanation of how to design research.
Trochim, William M. K. (1996) External validity. (. Available: http://trochim.human.cornell.edu/kb/EXTERVAL.htm
A comprehensive treatment of external validity found in William Trochim's online text about research methods and issues.
Trochim, William M. K. (1996) Introduction to validity. (. Available: hhttp://trochim.human.cornell.edu/kb/INTROVAL.htm
An introduction to validity found in William Trochim's online text about research methods and issues.
Trochim, William M. K. (1996) Reliability. (. Available: http://trochim.human.cornell.edu/kb/reltypes.htm
A comprehensive treatment of reliability found in William Trochim's online text about research methods and issues.
Validity. (1996, Oct. 12). Available: http://vislab-www.nps.navy.mil/~haga/validity.html
A source for definitions of various forms and types of reliability and validity.
Vinsonhaler, J. F., et al. (1983, July). Improving diagnostic reliability in reading through training. Institute for Research on Teaching ED 237 934.
This technical report investigates the practical application of a program intended to improve the diagnoses of reading deficient students. Here, reliability is assumed and a pragmatic answer to a specific educational problem is suggested as a result.
Wentland, E. J. & Smith, K.W. (1993). Survey responses: An evaluation of their validity . San Diego: Academic Press.
This book looks at the factors affecting response validity (or the accuracy of self-reports in surveys) and provides several examples with varying accuracy levels.
Wiget, A. (1996). Father juan greyrobe: Reconstructing tradition histories, and the reliability and validity of uncorroborated oral tradition. Ethnohistory 43:3 , 459-482.
This paper presents a convincing argument for the validity of oral histories in ethnographic research where at least some of the evidence can be corroborated through written records.
Yang, G. H., et al. (1995). Experimental and quasi-experimental educational research . Diss. Colorado State University.
This discussion defines experimentation and considers the rhetorical issues and advantages and disadvantages of experimental research. Annotated bibliography.
Yarroch, W. L. (1991, Sept.). The Implications of content versus validity on science tests. Journal of Research in Science Teaching , 619-629.
The use of content validity as the primary assurance of the measurement accuracy for science assessment examinations is questioned. An alternative accuracy measure, item validity, is proposed to look at qualitative comparisons between different factors.
Yin, R. K. (1989). Case study research: Design and methods . London: Sage Publications.
This book discusses the design process of case study research, including collection of evidence, composing the case study report, and designing single and multiple case studies.
Related Links
Internal Validity Tutorial. An interactive tutorial on internal validity.
http://server.bmod.athabascau.ca/html/Validity/index.shtml
Howell, Jonathan, Paul Miller, Hyun Hee Park, Deborah Sattler, Todd Schack, Eric Spery, Shelley Widhalm, & Mike Palmquist. (2005). Reliability and Validity. Writing@CSU . Colorado State University. https://writing.colostate.edu/guides/guide.cfm?guideid=66
- How it works
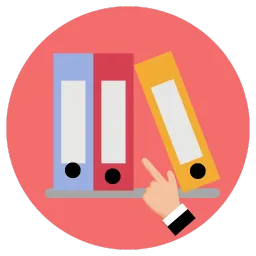
Reliability and Validity – Definitions, Types & Examples
Published by Alvin Nicolas at August 16th, 2021 , Revised On October 26, 2023
A researcher must test the collected data before making any conclusion. Every research design needs to be concerned with reliability and validity to measure the quality of the research.
What is Reliability?
Reliability refers to the consistency of the measurement. Reliability shows how trustworthy is the score of the test. If the collected data shows the same results after being tested using various methods and sample groups, the information is reliable. If your method has reliability, the results will be valid.
Example: If you weigh yourself on a weighing scale throughout the day, you’ll get the same results. These are considered reliable results obtained through repeated measures.
Example: If a teacher conducts the same math test of students and repeats it next week with the same questions. If she gets the same score, then the reliability of the test is high.
What is the Validity?
Validity refers to the accuracy of the measurement. Validity shows how a specific test is suitable for a particular situation. If the results are accurate according to the researcher’s situation, explanation, and prediction, then the research is valid.
If the method of measuring is accurate, then it’ll produce accurate results. If a method is reliable, then it’s valid. In contrast, if a method is not reliable, it’s not valid.
Example: Your weighing scale shows different results each time you weigh yourself within a day even after handling it carefully, and weighing before and after meals. Your weighing machine might be malfunctioning. It means your method had low reliability. Hence you are getting inaccurate or inconsistent results that are not valid.
Example: Suppose a questionnaire is distributed among a group of people to check the quality of a skincare product and repeated the same questionnaire with many groups. If you get the same response from various participants, it means the validity of the questionnaire and product is high as it has high reliability.
Most of the time, validity is difficult to measure even though the process of measurement is reliable. It isn’t easy to interpret the real situation.
Example: If the weighing scale shows the same result, let’s say 70 kg each time, even if your actual weight is 55 kg, then it means the weighing scale is malfunctioning. However, it was showing consistent results, but it cannot be considered as reliable. It means the method has low reliability.
Internal Vs. External Validity
One of the key features of randomised designs is that they have significantly high internal and external validity.
Internal validity is the ability to draw a causal link between your treatment and the dependent variable of interest. It means the observed changes should be due to the experiment conducted, and any external factor should not influence the variables .
Example: age, level, height, and grade.
External validity is the ability to identify and generalise your study outcomes to the population at large. The relationship between the study’s situation and the situations outside the study is considered external validity.
Also, read about Inductive vs Deductive reasoning in this article.
Looking for reliable dissertation support?
We hear you.
- Whether you want a full dissertation written or need help forming a dissertation proposal, we can help you with both.
- Get different dissertation services at ResearchProspect and score amazing grades!
Threats to Interval Validity
Threat | Definition | Example |
---|---|---|
Confounding factors | Unexpected events during the experiment that are not a part of treatment. | If you feel the increased weight of your experiment participants is due to lack of physical activity, but it was actually due to the consumption of coffee with sugar. |
Maturation | The influence on the independent variable due to passage of time. | During a long-term experiment, subjects may feel tired, bored, and hungry. |
Testing | The results of one test affect the results of another test. | Participants of the first experiment may react differently during the second experiment. |
Instrumentation | Changes in the instrument’s collaboration | Change in the may give different results instead of the expected results. |
Statistical regression | Groups selected depending on the extreme scores are not as extreme on subsequent testing. | Students who failed in the pre-final exam are likely to get passed in the final exams; they might be more confident and conscious than earlier. |
Selection bias | Choosing comparison groups without randomisation. | A group of trained and efficient teachers is selected to teach children communication skills instead of randomly selecting them. |
Experimental mortality | Due to the extension of the time of the experiment, participants may leave the experiment. | Due to multi-tasking and various competition levels, the participants may leave the competition because they are dissatisfied with the time-extension even if they were doing well. |
Threats of External Validity
Threat | Definition | Example |
---|---|---|
Reactive/interactive effects of testing | The participants of the pre-test may get awareness about the next experiment. The treatment may not be effective without the pre-test. | Students who got failed in the pre-final exam are likely to get passed in the final exams; they might be more confident and conscious than earlier. |
Selection of participants | A group of participants selected with specific characteristics and the treatment of the experiment may work only on the participants possessing those characteristics | If an experiment is conducted specifically on the health issues of pregnant women, the same treatment cannot be given to male participants. |
How to Assess Reliability and Validity?
Reliability can be measured by comparing the consistency of the procedure and its results. There are various methods to measure validity and reliability. Reliability can be measured through various statistical methods depending on the types of validity, as explained below:
Types of Reliability
Type of reliability | What does it measure? | Example |
---|---|---|
Test-Retests | It measures the consistency of the results at different points of time. It identifies whether the results are the same after repeated measures. | Suppose a questionnaire is distributed among a group of people to check the quality of a skincare product and repeated the same questionnaire with many groups. If you get the same response from a various group of participants, it means the validity of the questionnaire and product is high as it has high test-retest reliability. |
Inter-Rater | It measures the consistency of the results at the same time by different raters (researchers) | Suppose five researchers measure the academic performance of the same student by incorporating various questions from all the academic subjects and submit various results. It shows that the questionnaire has low inter-rater reliability. |
Parallel Forms | It measures Equivalence. It includes different forms of the same test performed on the same participants. | Suppose the same researcher conducts the two different forms of tests on the same topic and the same students. The tests could be written and oral tests on the same topic. If results are the same, then the parallel-forms reliability of the test is high; otherwise, it’ll be low if the results are different. |
Inter-Term | It measures the consistency of the measurement. | The results of the same tests are split into two halves and compared with each other. If there is a lot of difference in results, then the inter-term reliability of the test is low. |
Types of Validity
As we discussed above, the reliability of the measurement alone cannot determine its validity. Validity is difficult to be measured even if the method is reliable. The following type of tests is conducted for measuring validity.
Type of reliability | What does it measure? | Example |
---|---|---|
Content validity | It shows whether all the aspects of the test/measurement are covered. | A language test is designed to measure the writing and reading skills, listening, and speaking skills. It indicates that a test has high content validity. |
Face validity | It is about the validity of the appearance of a test or procedure of the test. | The type of included in the question paper, time, and marks allotted. The number of questions and their categories. Is it a good question paper to measure the academic performance of students? |
Construct validity | It shows whether the test is measuring the correct construct (ability/attribute, trait, skill) | Is the test conducted to measure communication skills is actually measuring communication skills? |
Criterion validity | It shows whether the test scores obtained are similar to other measures of the same concept. | The results obtained from a prefinal exam of graduate accurately predict the results of the later final exam. It shows that the test has high criterion validity. |
Does your Research Methodology Have the Following?
- Great Research/Sources
- Perfect Language
- Accurate Sources
If not, we can help. Our panel of experts makes sure to keep the 3 pillars of Research Methodology strong.
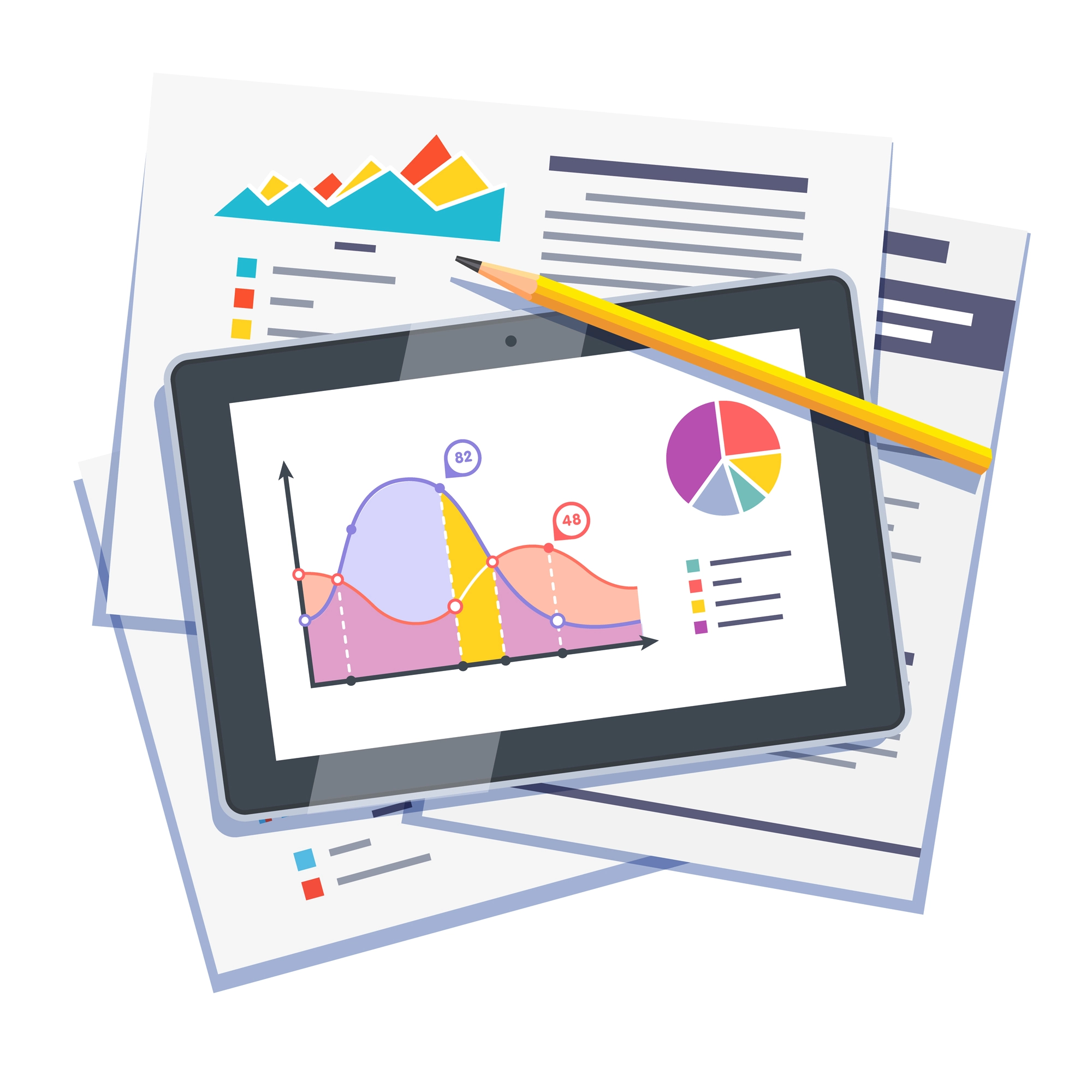
How to Increase Reliability?
- Use an appropriate questionnaire to measure the competency level.
- Ensure a consistent environment for participants
- Make the participants familiar with the criteria of assessment.
- Train the participants appropriately.
- Analyse the research items regularly to avoid poor performance.
How to Increase Validity?
Ensuring Validity is also not an easy job. A proper functioning method to ensure validity is given below:
- The reactivity should be minimised at the first concern.
- The Hawthorne effect should be reduced.
- The respondents should be motivated.
- The intervals between the pre-test and post-test should not be lengthy.
- Dropout rates should be avoided.
- The inter-rater reliability should be ensured.
- Control and experimental groups should be matched with each other.
How to Implement Reliability and Validity in your Thesis?
According to the experts, it is helpful if to implement the concept of reliability and Validity. Especially, in the thesis and the dissertation, these concepts are adopted much. The method for implementation given below:
Segments | Explanation | |||||||||||||||||||||||||||||||||||||
---|---|---|---|---|---|---|---|---|---|---|---|---|---|---|---|---|---|---|---|---|---|---|---|---|---|---|---|---|---|---|---|---|---|---|---|---|---|---|
All the planning about reliability and validity will be discussed here, including the chosen samples and size and the techniques used to measure reliability and validity. | ||||||||||||||||||||||||||||||||||||||
Please talk about the level of reliability and validity of your results and their influence on values. | ||||||||||||||||||||||||||||||||||||||
Frequently Asked QuestionsWhat is reliability and validity in research. Reliability in research refers to the consistency and stability of measurements or findings. Validity relates to the accuracy and truthfulness of results, measuring what the study intends to. Both are crucial for trustworthy and credible research outcomes. What is validity?Validity in research refers to the extent to which a study accurately measures what it intends to measure. It ensures that the results are truly representative of the phenomena under investigation. Without validity, research findings may be irrelevant, misleading, or incorrect, limiting their applicability and credibility. What is reliability?Reliability in research refers to the consistency and stability of measurements over time. If a study is reliable, repeating the experiment or test under the same conditions should produce similar results. Without reliability, findings become unpredictable and lack dependability, potentially undermining the study’s credibility and generalisability. What is reliability in psychology?In psychology, reliability refers to the consistency of a measurement tool or test. A reliable psychological assessment produces stable and consistent results across different times, situations, or raters. It ensures that an instrument’s scores are not due to random error, making the findings dependable and reproducible in similar conditions. What is test retest reliability?Test-retest reliability assesses the consistency of measurements taken by a test over time. It involves administering the same test to the same participants at two different points in time and comparing the results. A high correlation between the scores indicates that the test produces stable and consistent results over time. How to improve reliability of an experiment?
What is the difference between reliability and validity?Reliability refers to the consistency and repeatability of measurements, ensuring results are stable over time. Validity indicates how well an instrument measures what it’s intended to measure, ensuring accuracy and relevance. While a test can be reliable without being valid, a valid test must inherently be reliable. Both are essential for credible research. Are interviews reliable and valid?Interviews can be both reliable and valid, but they are susceptible to biases. The reliability and validity depend on the design, structure, and execution of the interview. Structured interviews with standardised questions improve reliability. Validity is enhanced when questions accurately capture the intended construct and when interviewer biases are minimised. Are IQ tests valid and reliable?IQ tests are generally considered reliable, producing consistent scores over time. Their validity, however, is a subject of debate. While they effectively measure certain cognitive skills, whether they capture the entirety of “intelligence” or predict success in all life areas is contested. Cultural bias and over-reliance on tests are also concerns. Are questionnaires reliable and valid?Questionnaires can be both reliable and valid if well-designed. Reliability is achieved when they produce consistent results over time or across similar populations. Validity is ensured when questions accurately measure the intended construct. However, factors like poorly phrased questions, respondent bias, and lack of standardisation can compromise their reliability and validity. You May Also LikeIn historical research, a researcher collects and analyse the data, and explain the events that occurred in the past to test the truthfulness of observations. In correlational research, a researcher measures the relationship between two or more variables or sets of scores without having control over the variables. Struggling to figure out “whether I should choose primary research or secondary research in my dissertation?” Here are some tips to help you decide. USEFUL LINKS LEARNING RESOURCES ![]() COMPANY DETAILS ![]()
![]() Research validity in surveys relates to the extent at which the survey measures right elements that need to be measured. In simple terms, validity refers to how well an instrument as measures what it is intended to measure. Reliability alone is not enough, measures need to be reliable, as well as, valid. For example, if a weight measuring scale is wrong by 4kg (it deducts 4 kg of the actual weight), it can be specified as reliable, because the scale displays the same weight every time we measure a specific item. However, the scale is not valid because it does not display the actual weight of the item. Research validity can be divided into two groups: internal and external. It can be specified that “internal validity refers to how the research findings match reality, while external validity refers to the extend to which the research findings can be replicated to other environments” (Pelissier, 2008, p.12). Moreover, validity can also be divided into five types: 1. Face Validity is the most basic type of validity and it is associated with a highest level of subjectivity because it is not based on any scientific approach. In other words, in this case a test may be specified as valid by a researcher because it may seem as valid, without an in-depth scientific justification. Example: questionnaire design for a study that analyses the issues of employee performance can be assessed as valid because each individual question may seem to be addressing specific and relevant aspects of employee performance. 2. Construct Validity relates to assessment of suitability of measurement tool to measure the phenomenon being studied. Application of construct validity can be effectively facilitated with the involvement of panel of ‘experts’ closely familiar with the measure and the phenomenon. Example: with the application of construct validity the levels of leadership competency in any given organisation can be effectively assessed by devising questionnaire to be answered by operational level employees and asking questions about the levels of their motivation to do their duties in a daily basis. 3. Criterion-Related Validity involves comparison of tests results with the outcome. This specific type of validity correlates results of assessment with another criterion of assessment. Example: nature of customer perception of brand image of a specific company can be assessed via organising a focus group. The same issue can also be assessed through devising questionnaire to be answered by current and potential customers of the brand. The higher the level of correlation between focus group and questionnaire findings, the high the level of criterion-related validity. 4. Formative Validity refers to assessment of effectiveness of the measure in terms of providing information that can be used to improve specific aspects of the phenomenon. Example: when developing initiatives to increase the levels of effectiveness of organisational culture if the measure is able to identify specific weaknesses of organisational culture such as employee-manager communication barriers, then the level of formative validity of the measure can be assessed as adequate. 5. Sampling Validity (similar to content validity) ensures that the area of coverage of the measure within the research area is vast. No measure is able to cover all items and elements within the phenomenon, therefore, important items and elements are selected using a specific pattern of sampling method depending on aims and objectives of the study. Example: when assessing a leadership style exercised in a specific organisation, assessment of decision-making style would not suffice, and other issues related to leadership style such as organisational culture, personality of leaders, the nature of the industry etc. need to be taken into account as well. My e-book, The Ultimate Guide to Writing a Dissertation in Business Studies: a step by step assistance offers practical assistance to complete a dissertation with minimum or no stress. The e-book covers all stages of writing a dissertation starting from the selection to the research area to submitting the completed version of the work within the deadline. John Dudovskiy ![]() ![]() An official website of the United States government The .gov means it’s official. Federal government websites often end in .gov or .mil. Before sharing sensitive information, make sure you’re on a federal government site. The site is secure. The https:// ensures that you are connecting to the official website and that any information you provide is encrypted and transmitted securely.
Save citation to fileEmail citation, add to collections.
Add to My BibliographyYour saved search, create a file for external citation management software, your rss feed.
Reliability and validity: Importance in Medical ResearchAffiliations.
Reliability and validity are among the most important and fundamental domains in the assessment of any measuring methodology for data-collection in a good research. Validity is about what an instrument measures and how well it does so, whereas reliability concerns the truthfulness in the data obtained and the degree to which any measuring tool controls random error. The current narrative review was planned to discuss the importance of reliability and validity of data-collection or measurement techniques used in research. It describes and explores comprehensively the reliability and validity of research instruments and also discusses different forms of reliability and validity with concise examples. An attempt has been taken to give a brief literature review regarding the significance of reliability and validity in medical sciences. Keywords: Validity, Reliability, Medical research, Methodology, Assessment, Research tools.. PubMed Disclaimer Similar articles
Publication types
LinkOut - more resourcesFull text sources.
![]()
NCBI Literature Resources MeSH PMC Bookshelf Disclaimer The PubMed wordmark and PubMed logo are registered trademarks of the U.S. Department of Health and Human Services (HHS). Unauthorized use of these marks is strictly prohibited. Have a language expert improve your writingRun a free plagiarism check in 10 minutes, generate accurate citations for free.
Methodology
External Validity | Definition, Types, Threats & ExamplesPublished on May 8, 2020 by Pritha Bhandari . Revised on December 18, 2023. External validity is the extent to which you can generalize the findings of a study to other situations, people, settings, and measures. In other words, can you apply the findings of your study to a broader context? The aim of scientific research is to produce generalizable knowledge about the real world. Without high external validity, you cannot apply results from the laboratory to other people or the real world. These results will suffer from research biases like undercoverage bias . In qualitative studies , external validity is referred to as transferability. Table of contentsTypes of external validity, trade-off between external and internal validity, threats to external validity and how to counter them, other interesting articles, frequently asked questions about external validity. There are two main types of external validity: population validity and ecological validity. ![]() Population validityPopulation validity refers to whether you can reasonably generalize the findings from your sample to a larger group of people (the population). Population validity depends on the choice of population and on the extent to which the study sample mirrors that population. Non-probability sampling methods are often used for convenience. With this type of sampling, the generalizability of results is limited to populations that share similar characteristics with the sample. You recruit over 200 participants. They are science and engineering majors; most of them are American, male, 18–20 years old and from a high socioeconomic background. In a laboratory setting, you administer a mathematics and science test and then ask them to rate how well they think performed. You find that the average participant believes they are smarter than 66% of their peers. Here, your sample is not representative of the whole population of students at your university. The findings can only reasonably be generalized to populations that share characteristics with the participants, e.g. college-educated men and STEM majors. For higher population validity, your sample would need to include people with different characteristics (e.g., women, non-binary people, and students from different majors, countries, and socioeconomic backgrounds). Samples like this one, from Western, Educated, Industrialized, Rich and Democratic (WEIRD) countries, are used in an estimated 96% of psychology studies , even though they represent only 12% of the world’s population. Since they are outliers in terms of visual perception, moral reasoning and categorization (among many other topics), WEIRD samples limit broad population validity in the social sciences.
Ecological validity refers to whether you can reasonably generalize the findings of a study to other situations and settings in the ‘real world’. In a laboratory setting, you set up a simple computer-based task to measure reaction times. Participants are told to imagine themselves driving around the racetrack and double-click the mouse whenever they see an orange cat on the screen. For one round, participants listen to a podcast. In the other round, they do not need to listen to anything. After assessing the results, you find that reaction times are much slower when listening to the podcast. In the example above, it is difficult to generalize the findings to real-life driving conditions. A computer-based task using a mouse does not resemble real-life driving conditions with a steering wheel. Additionally, a static image of an orange cat may not represent common real-life hurdles when driving. To improve ecological validity in a lab setting, you could use an immersive driving simulator with a steering wheel and foot pedal instead of a computer and mouse. This increases psychological realism by more closely mirroring the experience of driving in the real world. Alternatively, for higher ecological validity, you could conduct the experiment using a real driving course. Here's why students love Scribbr's proofreading servicesDiscover proofreading & editing Internal validity is the extent to which you can be confident that the causal relationship established in your experiment cannot be explained by other factors. There is an inherent trade-off between external and internal validity ; the more applicable you make your study to a broader context, the less you can control extraneous factors in your study. Threats to external validity are important to recognize and counter in a research design for a robust study. Participants are given a pretest and a post-test measuring how often they experienced anxiety in the past week. During the study, all participants are given an individual mindfulness training and asked to practice mindfulness daily for 15 minutes in the morning.
How to counter threats to external validityThere are several ways to counter threats to external validity:
If you want to know more about statistics , methodology , or research bias , make sure to check out some of our other articles with explanations and examples.
Research bias
Receive feedback on language, structure, and formattingProfessional editors proofread and edit your paper by focusing on:
See an example ![]() The external validity of a study is the extent to which you can generalize your findings to different groups of people, situations, and measures. I nternal validity is the degree of confidence that the causal relationship you are testing is not influenced by other factors or variables . External validity is the extent to which your results can be generalized to other contexts. The validity of your experiment depends on your experimental design . There are seven threats to external validity : selection bias , history, experimenter effect, Hawthorne effect , testing effect, aptitude-treatment and situation effect. The two types of external validity are population validity (whether you can generalize to other groups of people) and ecological validity (whether you can generalize to other situations and settings). Cite this Scribbr articleIf you want to cite this source, you can copy and paste the citation or click the “Cite this Scribbr article” button to automatically add the citation to our free Citation Generator. Bhandari, P. (2023, December 18). External Validity | Definition, Types, Threats & Examples. Scribbr. Retrieved September 3, 2024, from https://www.scribbr.com/methodology/external-validity/ Is this article helpful?![]() Pritha BhandariOther students also liked, internal vs. external validity | understanding differences & threats, internal validity in research | definition, threats, & examples, sampling bias and how to avoid it | types & examples, get unlimited documents corrected. ✔ Free APA citation check included ✔ Unlimited document corrections ✔ Specialized in correcting academic texts ![]() An official website of the United States government The .gov means it’s official. Federal government websites often end in .gov or .mil. Before sharing sensitive information, make sure you’re on a federal government site. The site is secure. The https:// ensures that you are connecting to the official website and that any information you provide is encrypted and transmitted securely.
Preview improvements coming to the PMC website in October 2024. Learn More or Try it out now .
![]() Construct Validity: Advances in Theory and MethodologyMilton e. strauss. i Department of Psychology, University of New Mexico, MSC03 2220 1 University of New Mexico Albuquerque, NM 87131−0001 [email protected] Gregory T. Smithii Department of Psychology, University of Kentucky Lexington, KY 40506−0044 ude.yku.liame@htimsg Measures of psychological constructs are validated by testing whether they relate to measures of other constructs as specified by theory. Each test of relations between measures reflects on the validity of both the measures and the theory driving the test. Construct validation concerns the simultaneous process of measure and theory validation. In this chapter, we review the recent history of validation efforts in clinical psychological science that has led to this perspective, and we review five recent advances in validation theory and methodology of importance for clinical researchers. These are: the emergence of nonjustificationist philosophy of science; an increasing appreciation for theory and the need for informative tests of construct validity; valid construct representation in experimental psychopathology; the need to avoid representing multidimensional constructs with a single score; and the emergence of effective new statistical tools for the evaluation of convergent and discriminant validity. In this chapter, we highlight the centrality of construct validation to theory testing in clinical psychology. In doing so, we first provide a brief history of modern validation efforts and describe the foundational role construct validity theory has for modern, scientific clinical psychology. We then highlight four recent developments in construct validity theory and advances in statistical methodology that, we believe, should play an important role in shaping construct and theory validation efforts. We begin with a brief history. An Historical Overview of Validation Efforts in Clinical PsychologyAt the modern beginning of scientific clinical psychology in the beginning of the 20 th century, researchers faced the challenge of developing valid measures without an existing knowledge base on which to rely. The absence of a foundation of knowledge was an enormous problem for test validation efforts. The goal of validating measures of psychological constructs necessarily requires criteria that are themselves valid. One cannot show that a predictor of some form of psychopathology is valid, unless one can show that the predictor relates to an indicator of that form of psychopathology that is, itself, valid. One cannot show that a certain deficit in cognitive processing characterizes individuals with a certain disorder unless one has defined and validly measured the disorder. Inevitably, to validate scores on measures one needs a structure of existing knowledge to which one can relate those scores. To go further, to validate one's claim that scores on a measure play a certain role in a network of psychological processes, one needs valid measures of the different components of the specified processes. As researchers developed measures, and confirmed or disconfirmed early, relatively crude predictive hypotheses, a knowledge base began to develop. The development of a knowledge base made possible the specification of procedures for measure validation. The specification of such procedures, in turn, facilitated further knowledge acquisition. And as knowledge continued to develop, the need for more theoretically sophisticated means of measure and theory validation emerged. We believe the recent history of validation efforts reflects this kind of reciprocal influence between existing knowledge and validation standards. We next briefly describe this process in greater detail. Early Measure Development and ValidityAn often-discussed early measure in the history of validation efforts is the Woodworth Personal Data Sheet (WPDS), a measure developed in 1919 to help the U.S. Army screen out individuals who might be vulnerable to “war neurosis” or “shell shock.” It was subsequently described as measuring emotional stability ( Garrett & Schneck 1928 ; Morey 2002 ). Both during construction and use of the test, researchers showed clear concern with its validity. Unfortunately, their efforts to both develop and validate the test reflected the weak knowledge structure of clinical research at the time. Woodworth constructed the 116 item test by relying on existing clinical psychological knowledge and by using empirical methods. Specifically, he drew his item content from case histories of individuals identified as neurotic. He then administered the items to a normal test group and deleted items scored in the presumably dysfunctional direction by 50% or more of that group ( Garrett & Schneck 1928 ). Clearly, he sought to construct a valid measure of dysfunction. And although not all researchers who used the WPDS concerned themselves with its validity, some did. Flemming & Flemming (1929) chided researchers for neglecting to validate the test, and then conducted their own empirical test of the measure. Items on the WPDS are quite diverse. They include, “Have you ever lost your memory for a time?”, “Can you sit still without fidgeting?”, “Does it make you uneasy to have to cross a wide street or an open square?”, and “Does some particular useless thought keep coming into your mind to bother you?” From the standpoint of today's knowledge base in clinical psychology, each of these four sample items seems to refer to a different construct. It is thus not surprising that the measure did not perform well. It did not differentiate college students from “avowed psychoneurotics” ( Garrett & Schneck 1928 ), nor did it correlate with teacher ratings of students’ emotional stability ( Flemming & Flemming 1929 ). One can see two core limitations underlying the effort to develop this test and validate it. First, in developing the WPDS item pool, Woodworth had to rely on a far too incomplete understanding of psychopathology and its contributors. Second, the validity of the criterion measures was not established independently and was based either on broad diagnostic classification or subjective teacher ratings; surely the validity of these criteria was limited. Researchers at the time expressed concerns related to these limitations. For example, Garrett & Schneck (1928) noted the heterogeneous items and the mixture of complaints represented in the item pool and drew a conclusion that anticipated recent advances in validation theory to be discussed later in this chapter: “It is this [heterogeneity], among other [considerations], which is causing the present-day trend away from the concept of mental disease as an entity. Instead of saying that a patient has this or that disease, the modern psychiatrist prefers to say that the patient exhibits such and such symptoms.” (p. 465). Based on this thinking, Garrett & Schneck (1928) investigated relations among individual items and specific diagnoses (rather than membership in the general category of “mentally disturbed”). In doing so, they recognized the need to avoid combining items of different content as well as the need to avoid combining individuals with different symptom pictures. Their use of an empirical item – person classification produced very different results from prior rational classifications ( Laird 1925 ), thus (a) implicating the importance of empirical validation and (b) anticipating criterion-keying methods of test construction. In addition, they anticipated the current appreciation for construct homogeneity, with its emphasis on unidimensional traits and unidimensional symptoms as the preferred objects of theoretical study and measure validation ( Edwards 2001 ; McGrath 2005 ; Smith et al. 2003 ; Smith & Combs, 2008 ). The Validation of Measures as their Ability to Predict CriteriaDuring the early and middle parts of the 20 th century, test validity came to be understood in terms of a test's ability to predict a practical criterion ( Cureton 1950 ; Kane 2001 ). This focus on criterion prediction may have been a function of three forces: advances in substantive knowledge and precision of thought in the field, the obvious limitations in the tests constructed on purely rational grounds, and a philosophy-based suspicion of theories describing unobservable entities ( Blumberg & Feigl 1931 ). Indeed, many validation theorists explicitly rejected the idea that scores on a test mean anything beyond their ability to predict an outcome. As Anastasi (1950) put it, “It is only as a measure of a specifically defined criterion that a test can be objectively validated at all . . . . To claim that a test measures anything over and above its criterion is pure speculation.” (p. 67) At the time, this approach to measure validation proved quite useful: it led to the generation of new methods of test construction as well as to important substantive advances in knowledge. Concerning test construction, it led to the criterion-keying approach, in which one selects items entirely on the basis of whether the items predict the criterion. This method represented an important advance: to some degree, validity as successful criterion prediction was built into the test. The method worked well. Two of the most prominent measures of personality and psychopathology, the MMPI ( Butcher 1995 ) and the CPI ( Megargee, 2008 ), were developed using criterion-keying. Each of those measures has generated a wealth of knowledge concerning personality, psychopathology, and adjustment: there are thousands of studies attesting to the measures’ clinical value. For example, the MMPI-2 distinguishes between psychiatric inpatients and outpatients and facilitates treatment planning ( Butcher 1990 ; Greene 2006 ; Nichols & Crowhurst 2006 ; Perry et al. 2006 ). It has also been applied usefully to normal populations (such as in personnel assessment: Butcher 2002 ; Derksen et al. 2003 ), to head-injured populations ( Gass 2002 ), and in correctional facilities ( Megargee 2006 ). The CPI validly predicts a wide range of criteria as well ( Gough 1996 ). As Kane (2001) noted, the criterion-related validity perspective also led to more sophisticated treatments of the relationship between test scores and criteria, as well as to the development of utility-based decision rules (see Cronbach & Gleser 1965 ). Perhaps it is also true that the focus on prediction of criteria as the defining feature of validity contributed to the finding that statistical combinations of test data are superior to clinical combinations, and that this is true across domains of inquiry ( Grove et al. 2000 ; Swets et al. 2000 ). As prediction improved and knowledge advanced using this criterion validity perspective, the ultimate limitations of the method became clear. One core limitation reflects a difficulty in prediction that was present from the beginning: tests of criterion-related validity are only as good as the criteria used in the prediction task. As Bechtoldt (1951) put it, reliance on criterion-related validity “involves the acceptance of a set of operations as an adequate definition of whatever is to be measured [or predicted].” (p. 1245). Typically, the validity of the criterion was presumed, not evaluated independently. In hindsight, there was good reason to question the validity of many criteria: they were often based on some form of judgment (crude diagnostic classification, teacher rating), and those judgments had to be made with an insufficiently developed knowledge base. Limitations in the validity of criteria impose limitations in one's capacity to validate a measure. The second limitation is one that led to the development of construct validity theory and that could only have become apparent once the core knowledge base in clinical psychology had developed sufficiently: the criterion-related validity approach does not facilitate the development of basic theory. When tests are developed for the specific intent of predicting a circumscribed criterion, as is the case with criterion-keying test construction, and when they are only validated with respect to that predictive task, as is the case with criterion-related validity, the validation process is likely to contribute little to theory development. As a result, criterion-related validity findings tend not to provide a strong foundation for deducing likely relationships among variables, and hence for the development of generative theory. The Emergence of Construct ValidityIn the early 1950's there was an emerging concern with theory development that led to Meehl and Challman's introduction of the concept of construct validity in the 1954 Technical Recommendations ( American Psychological Association 1954 ). Their work was part of the work of the American Psychological Association's Committee on Psychological Tests. In our view, the developing focus on theory was made possible, in part, by the substantive advances in clinical knowledge facilitated by the criterion-related validity approach. Perhaps ironically, the success of the criterion-related validity method led to its ultimate replacement with construct validity theory. The criterion approach led to significant advances in knowledge, which helped facilitate the development of integrative theories concerning cognition, personality, behavior, and psychopathology. But such theories could not be validated using the criterion approach; there was thus a need for advances in validation theory to make possible the emerging theoretical advances. This need was addressed by several construct validity authors in the middle of the 20 th century ( Campbell & Fiske 1959 ; Cronbach & Meehl 1955 ; Loevinger 1957 ). Indeed, theoretical progress in clinical psychology has substantially depended on four seminal papers all published within a decade. The first ( MacCorquodale & Meehl 1948 ) promoted the philosophical legitimacy of hypothetical constructs, concepts that have a “cognitive factual reference” (p. 107) that goes beyond the data used to support them. That is, hypothetical constructs are hypotheses about the existence of entities, processes, or events that are not directly observed. That seminal paper advanced the legitimacy of psychological theories that describe entities that underlie, but are not equivalent to, what is observed in the laboratory or other research setting. The second ( Cronbach & Meehl 1955 ) described the methods and rules of inference by which one develops evidence for the validity of measures of such hypothetical constructs. Construct validation tests are also tests of the validity of the theory that specifies a measure's presumed meaning. We use the word developed rather than established to emphasize that construct validation is an ongoing process, the process of theory testing. Central to Cronbach and Meehl's conceptualization of construct validity was the need to articulate specific theories describing relations among psychological processes, in order to then evaluate the performance of measures thought to represent one such process (see also Garner et al. 1956 ). Cronbach & Meehl (1955) emphasized deductive processes in construct validity. The third ( Loevinger 1957 ) identified the construct validation process as the general framework for the development and testing of psychological theories and the measures used to represent theoretical constructs. In Loevinger's view, construct validity subsumed both content validity and predictive/concurrent, or empirical, validity. In short, construct validity is validity (see also, Landy 1986 , Messick 1995 ). The fourth paper ( Campbell & Fiske 1959 ) considered issues in the validation of purported indicators of a construct. The title of their article, “Convergent and Discriminant Validation by the Multitrait-Multimethod Matrix (MTMM),” refers to two of the three core ideas in their article that remain crucial in the process of validation of a measure as a construct indicator. First, all measures are trait (construct)-method units. That is, variance in all psychological measures consists of the substantive construct variance, variance due to the method of measurement that is independent of the construct and, of course, errors of measurement. Second, two types of evidence are required to validate a test or other measurement in psychology. The first, convergent validity, is demonstrated by associations among ” independent measurement procedures” designed to reflect the same or similar constructs ( Campbell & Fiske 1959 , p. 81, emphasis added). The second aspect of measurement validity, discriminant validity iii requires that a new measure of a construct be substantially less correlated with measures of conceptually unrelated constructs than with other indicators of that construct. Discriminant validity requires the contrast of relationships of measures of constructs in the same conceptual domain, e.g. personality or symptom dimension constructs. Although Campbell & Fiske (1959) gave even weight to convergent and discriminant validity, in later work, the initial primacy of convergent validity is acknowledged ( Cook & Campbell 1979 ; see Ozer 1989 ). Third, because of the ever-present, often substantial method variance in all psychological measures, validation studies require the simultaneous consideration of 2 or more traits measured by at least 2 different methods. Campbell & Fiske (1959) referred to this approach as multitrait - multimethod matrix methodology; we return to this specific methodology at the end of this article. Although these papers are over 50 years old, each remains an invaluable place to begin one's mastery of the concept of construct validity. From the first three of these foundational papers, we understand that each study using a measure is simultaneously a test of the validity of a measure and a test of the theory defining the construct. Each new test provides additional information supporting or undermining one's theory or validation claims; with each new test, the validity evidence develops further. Thus, validation is a process not an outcome. Often, the construct validity of a measure is described as “demonstrated,” which is incorrect ( Cronbach & Meehl 1955 ). Although the process is ongoing, it is not necessarily infinite. For example, if a well validated measure such as the Wechsler Adult Intelligence Scale -III ( Wechsler 1997 ) or the Positive and Negative Affect Scale ( Watson et al. 1988b ) does not behave as “expected” in a study, the measure would not be abandoned. One would likely retain one's confidence in the measure and consider other possible explanations for the outcome, such as deficient research design. Since the time of these articles, it has also become clear that researchers should concern themselves with construct validity from the beginning of the test construction process. To develop a measure that validly represents a psychological entity, researchers should carefully define the construct and select items representing the definition ( Clark & Watson 1995 ). This reasoning extends to the selection of parameters for manipulation in experimental psychopathology (see Knight & Silverstein 2001 ). As Bryant (2000) effectively put it for the assessment of a trait, Imagine, for example, that you created an instrument to measure the extent to which an individual is a “nerd.” To demonstrate construct validity, you would need a clear initial definition of what a nerd is to show that the instrument in fact measures “nerdiness.” Furthermore, without a precise definition of nerd, you would have no way of distinguishing your measure of the nerdiness construct from measures of shyness, introversion or nonconformity. (p. 112). There have been four recent developments in perspectives on construct validity theory of importance for clinical psychological measurement. First, the philosophical understanding of scientific inquiry has evolved in ways that underscore both the complexity and the indeterminate nature of the validation process ( Bartley 1987 ; Weimer 1979 ). Second, it has become apparent that the relative absence of strong, precise theories in clinical psychology sometimes leads to weak, non-informative validation tests ( Cronbach 1988 ; Kane 2001 ). Appreciation of this has led theorists to re-emphasize the centrality of theory-testing in construct validation ( Borsboom et al. 2004 ; Kane 2001 ). Third, researchers have accentuated the need to consider as an aspect of construct validity, evaluation of theories describing the psychological processes that lead to responses in psychological experiments such as are used in experimental psychopathology research. Tests of such theories are evaluations of construct representation ( Whitely [now Embretson] 1983 ; Embretson 1998 ; see, Knight & Silverstein 2001 ). Fourth, researchers have stressed the importance of specifying and measuring homogeneous constructs, so the meaning of validation tests is unambiguous ( Edwards 2001 ; Hough & Schneider 1995 ; Schneider et al. 1996 ; McGrath 2005 ; Smith et al. 2003 ; Smith & McCarthy 1995 ; G.T. Smith, D.M. McCarthy, T.B. Zapolski, submitted manuscript). We consider each of these in turn. But first, what is the current view of construct validity in assessment? Current Views on Construct Validity in Psychological MeasurementConstruct validity is now generally viewed as a unifying form of validity for psychological measurements, subsuming both content and criterion validity, which traditionally had been treated as distinct forms of validity ( Landy 1986 ). Messick (1989 , as discussed in Messick 1995) has argued that even this notion of validity is also too narrow. In his view “[v]alidity is an overall evaluative judgment of the degree to which [multiple forms of] evidence and theoretical rationales support the adequacy and appropriateness of interpretations and actions on the basis of test scores...( Messick 1995 , p. 741),” That is, construct validity is comprehensive, encompassing all sources of evidence supporting specific interpretations of a score from a measure as well as actions based on such interpretations. Messick, writing mainly with reference to educational assessment, identified six contributors to construct validity ( Messick 1995 , see Figure 1, p. 748): (1) content relevance and technical quality; (2) theoretical understanding of scores and associated empirical evidence, including process analyses; (3) structural data; (4) generalizability; (5) external correlates; and (6) consequences of score interpretation We focus here on aspects (2), (3) and (5) , considering points (1) and (4) to be relatively well-established and not controversial, and the practical consequence of test use (point 6) to be beyond the scope of this chapter (but see Youngstrom 2008 ) Advances in Philosophy of ScienceIn the first half of the 20 th century, many philosophers of science held the view that theories could be fully justified or fully disproved based on empirical evidence. The classic idea of the critical experiment that could falsify a theory is part of this perspective, which has been called justificationism ( Bartley 1962 ; Duhem 1914 /1991; Lakatos 1968 ). Logical positivism ( Blumberg & Feigl 1931 ), with its belief that theories are straightforward derivations from observed facts, is one example of justificationist philosophy of science. From this perspective, one could imagine the validity of a theory and its accompanying measures being fully and unequivocally established as a result of a series of critical experiments. However, advances in the philosophy of science have led to a convergence on a different perspective, referred to as nonjustificationism ( Bartley 1987 ; Campbell 1987 , 1990 ; Feyerabend 1970 ; Kuhn 1970 ; Lakatos 1968 ; Weimer 1979 ). The nonjustificationist perspective is that no theory is ever fully proved or disproved. Instead, in the ongoing process of theory development and evaluation, at a given time certain theories are viewed as closer approximations to truth than are other theories. From this perspective (which dominates current philosophy of science, despite disagreement both within and outside this framework: Hacking 1999 ; Kusch 2002 ; Latour 1999 ), science is understood to be characterized by a lack of certainty. The reason for the uncertainty is as follows. When one tests any theory, such as “individual differences in personality cause individuals to react differently to the same stimulus” (a theory of considerable importance for understanding the process of risk for psychopathology: Caspi 1993 ), one is presupposing the validity of multiple theories in order to conduct the test ( Lakatos 1999 ; Meehl 1978 , 1990 ). In this example, one must accept that (1) there are reliable individual differences in personality that are not fully a function of context; (2) one has measured the appropriate domains of individual differences in personality; (3) one's measure of personality is valid, in that variation on dimensions of personality underlie variation in responses to the measure; (4) one's measure of personality does not represent other, non-personality processes to any substantial degree; (5) one's measure of each specific dimension of personality is coherent and unidimensional, i.e., does not represent variation on multiple dimensions simultaneously; (6) one can validly expose different individuals to precisely the same stimulus; (7) one can validly measure reactions to that stimulus; and so on. It is easy to see that a failed test of the initial, core hypothesis could actually be due not just to a failure of the theory, but instead to failures in any number of “auxiliary” theories invoked to test the hypothesis. Researchers typically consider a number of different possibilities when faced with a non-supportive finding. Often, when one faces a negative finding for a theory one believes has strong support otherwise, one questions any number of auxiliary issues: measurement, sample, context, etc. Doing so is quite appropriate ( Cronbach & Meehl 1955 ). Science is characterized by ongoing debates between proponents and opponents of a theoretical perspective. Through the ongoing process of theoretical criticism and new empirical findings, the debate comes to favor one side over the other. In considering this process, Weimer (1979) concluded that what characterizes science is “comprehensively critical rationalism” (p. 40), by which he meant that every aspect of the research enterprise must be open to criticism and potential revision. Researchers must make judgments as to whether one should question a core theory, an auxiliary theory, or both; they must then investigate the validity of those judgments empirically. Thus, validation efforts can be understood as arguments concerning the overall evaluation of the claimed interpretation of test scores ( Messick 1995 ), or of claims concerning the underlying theory ( Kane 2001 ). The validation enterprise can thus be understood to include a coherent analysis of the evidence for and against theory claims. Researchers can design theory validation tests based on their analysis of the sum total of evidence relevant to the target theory. Interestingly, this perspective, particularly as argued by psychological scientists, has begun to influence inquiry in historically non-empirical fields as well. For example, legal scholars, drawing on construct validation theory, have begun to argue that empirical investigation of legal arguments is a necessary part of the validation of those theories ( Goldman 2007 ). Their contention is that sound arguments for the validity of legal theories require both theoretical coherence and supportive empirical evidence. There is no obvious answer to the question of how one decides which theoretical arguments, embodied by programs of research, are convincing and which are not. Lakatos (1999) referred to progressing versus degenerating research programs. Progressing research programs predict facts that are subsequently confirmed by research; degenerating research programs may offer explanations for existing findings, but they do not make future predictions successfully, and they often require post hoc theoretical shifts to incorporate unanticipated findings ( Lakatos 1999 ). Clearly, this perspective requires judgment on the part of researchers. It is important to appreciate that the concept of the nonjustificationist nature of scientific inquiry did not spring from studies of psychology as a science. Most authors espousing these views have focused primarily on hard sciences, particularly physics. It is a reality of scientific inquiry that findings are always open to challenge and critical evaluation. Indeed, what separates science from other forms of inquiry is that it embraces critical evaluation, both by theory and by empirical investigation ( Weimer 1979 ). A second point is equally important to appreciate: the reality that no theories are ever fully proved or disproved is no excuse to proceed without theory or without clearly articulated theoretical predictions. Strong, Weak, and Informative Programs of Construct ValidationAs discussed recently by Kane (2001) , there have been drawbacks in the use of construct validity theory to organize measure and theory validation. The core idea that one can define constructs by their place in a lawful network of relationships (the network is deduced from the theory) assumes a theoretical precision that tends not to be present in the social sciences. Typically, clinical psychology researchers are faced with the task of validating their measures and theories despite the absence of a set of precisely definable, expected lawful relations among construct measures. Under this circumstance, the meaning of construct validity, and what counts as construct validation, is ambiguous. Cronbach (1988) addressed this issue by contrasting strong and weak programs of construct validity. Strong programs depend on precise theory, and are perhaps accurately understood to represent an ideal. Weak programs, on the other hand, stem from weak, or less fully articulated, theories and construct definitions. With weak validation programs, there is less guidance as to what counts as validity evidence ( Kane 2001 ). One result can be approaches in which almost any correlation can be described as validation evidence ( Cronbach 1988 ). In the absence of a commitment to precise construct definitions and specific theories, validation research can have an ad hoc, opportunistic quality ( Kane 2001 ), the results of which tend not to be very informative. Informative, Rather than Strong or Weak, Theory TestsIn our view, clinical researchers are not wedged between a yet unattainable ideal of strong theory and ill-conceived, weak theory testing. Rather, there is an iterative process in which tests of partially developed theories provide information that leads to theory refinement and elaboration, which in turn provides a sounder basis for subsequent construct and theory validation research. Cronbach & Meehl (1955) referred to this bootstrapping process and to the inductive quality of construct definition and theory articulation; advances in testing partially formed theories lead to the development of more elaborate, complete theories. This process has proven effective; striking advances in clinical research have provided clear benefits to the consumers of clinical services. One example of this process has been the development of an effective psychological treatment for many of the behaviors characteristic of a previously untreatable disorder: borderline personality disorder. Dialectical behavior therapy (DBT) provides improved treatment of parasuicidal behavior and excessive anger ( Linehan 1993 ; Linehan et al. 1993 ). The emergence of this treatment depended on incremental advances in numerous domains of clinical inquiry. First, advances in temperament theory and personality theory led to awareness of the stability of human temperament and personality, even across decades ( Caspi & Roberts 2001 ; Roberts & DelVecchio 2000 ). That finding carried the obvious implication that treatment aimed at altering personality may not prove effective. The second advance was the recognition of disorders of personality, i.e., chronic dysfunction in characteristic modes of thinking, perceiving, and behaving, as distinct from other sources of dysfunction ( Millon et al. 1996 ). That recognition facilitated the emergence of treatments targeted toward one's ongoing, typical mode of reacting and behaving. The third advance was the finding that behavioral interventions were effective for disorders of mood: when depressed individuals began participating in numerous, previously rewarding activities, their mood altered ( Dimidjian et al. 2006 ). DBT can be understood to represent the fruitful integration of each of these three theoretical advances. DBT was designed to treat individuals with borderline personality disorder. One central aspect of DBT is that therapists do not attempt to change borderline clients’ characteristic, intense affective response style: attempts to do so are unlikely to be successful, given the stability of personality. Instead, therapists seek to provide behavioral skills for clients to employ to manage their intense affective reactivity. The therapeutic focus has become managing one's mood effectively, and it has proven effective ( Linehan 1993 ). To facilitate the process of theory development, researchers should consider whether their theoretical statements and tests are informative, given the current state of knowledge ( Smith 2005b ). Is a theory consistent with what else is known in the field ( MacCorquodale & Meehl 1948 )? Can it be integrated with existing knowledge? To what degree does a hypothesis test shed light on the likely validity of a theory, or the likely validity of a measure? Does a hypothesis involve a direct comparison between two, alternative theoretical explanations? Does a hypothesis involve a claim that, if supported, undermines criticism of a theory? Does a hypothesis involve direct criticism of a theory, or a direct challenge to the validity of a measure? Theory tests of this kind will tend to advance knowledge, because they facilitate the central component of the scientific process: critical evaluation and cumulative knowledge. Recent Arguments for a Reconceptualization of the Role of Theory in Clinical ResearchIn recent years, validity theorists have argued for an increased emphasis on theory in several aspects of psychological inquiry ( Barrett 2005 ; Borsboom 2006 ; Borsboom et al. 2003 , 2004 ; Maraun & Peters 2005 ; Michell 2000 , 2001 ). We next review three basic arguments offered in this recent writing; we believe two of these apply, straightforwardly, to clinical science and the third does not. The first, which we consider both relevant to clinical research and uncontroversial, concerns latent variable theory. Latent variable theory reflects the idea that variation in responses to test items indicates variation in levels of an underlying trait. As Borsboom et al. (2003) most recently noted, latent variable theory involves a specific view of causality: variation in a construct causes variation in test scores. When clinical psychology researchers describe a scale as a valid measure of a construct, such as anxiety, they are saying that variation in anxiety among individuals causes variation in those individuals’ test responses. From this point of view, each item on a test is an indicator of the construct of interest. Borsboom et al. (2003) develop the implications of this theory for psychological assessment. The second concerns the basic distinction between theory and empirical data: theories exist independently of data ( Borsboom 2006 ). It is certainly appropriate for researchers to develop, adopt, and promote explicit theories of psychological processes. Of course, ideally, researchers avoid inferring that findings provide stronger support for theories than they do, but that appropriate caution should not dissuade researchers from taking clear theoretical stands. More explicit statements of theory would (a) clarify the degree to which a given empirical test truly pertains to the theory and (b) drive the development of more direct tests of theoretical mechanisms ( Borsboom 2006 ; Borsboom et al. 2004 ). The third recent argument is one that, we believe, does not accurately pertain to the development of clinical science. Several recent authors have emphasized the need for more explicit, well-developed theories in general ( Barrett 2005 ; Borsboom 2006 ; Maraun & Peters 2005 ; Michell 2000 , 2001 ). At least one of these writers ( Borsboom 2006 ) emphasizes the need to begin with precise, fully developed theories; in their view, to do otherwise is to provide a disservice to the field. For example, although psychological theories often refer to causal processes, they are neither detailed nor mathematically formal. From the point of view of these authors, this is regrettable. This point of view has not gone without criticism. Both Clark (2006) and Kane (2006) note that the incomplete knowledge base in psychology requires that any theory be an approximation, to be modified as new knowledge becomes available. Formal mathematical theories of psychological phenomena, especially in clinical psychology, are quite premature. And regardless of how detailed and precise the explication of a theory is, each component of it would necessarily undergo critical evaluation and revision as part of the normal progress of science ( Weimer 1979 ). It seems to us that this process is inevitable and a normal part of scientific inquiry. Construct Representation and Nomothetic SpanConstruct representation. Whitely (1983 ; Embretson 1998) introduced an important distinction in construct validity theory between nomothetic span and construct representation. Nomothetic span refers to the pattern of significant relations among measures of the same or different constructs (i.e., convergent and discriminant validity). Nomothetic span is in the domain of individual differences (correlation). It is particularly relevant to research concerning expected relationships among trait measures or measures of intellectual skills, neuropsychological variables, or measures of personality constructs. For example, IQ has excellent nomothetic span because individual differences in various measures of that construct all show similar meaningful patterns of relationship with other variables as expected ( Whitely 1983 ). The confirmatory factor analysis of a matrix of correlations among measures for which there are specifications of what relationships should be present and which absent is a method for evaluating nomothetic span. Construct representation ( Whitely 1983 ; Embretson 1998 ), on the other hand, refers to the validation of the theory of the response processes that result in a score (such as accuracy or reaction time) in the performance of cognitive tasks. That is, construct representation refers to the psychological processes that lead to a given response on a trial or to the pattern of responses across conditions in an experiment. For many authors, and particularly for cognitive psychologists, construct representation indicates the validity of the dependent variable as an index of a construct ( Borsboom et al. 2004 ; Embretson 1998 ). That is to say, the goal of construct representation is to test a theory of the cognitive processes giving rise to a response. An example may make the notion of construct representation clearer. Carpenter et al. (1990) proposed a theory of matrix reasoning problem solving to account for performance on tests such as Ravens Progressive Matrix test, a widely used measure of intelligence. Their model posited that working memory was a critical determinant of performance and that working memory load is influenced by two parameters: (1) the number of relationships among elements in a matrix, and (2) the level of complexity of the relationships among the elements. Note that these are quantitative variables. So by developing matrix items that systematically varied on these two dimensions, these investigators were able to evaluate the extent to which each parametric variation, separately and conjointly determined performance. The model, in other words, identified the underlying psychological processes that were validated, through accounting for performance on the task as the proposed processes were parametrically manipulated. The validity of the model provides evidence of the construct representation component of the test. The Ravens thus has both evidence of construct representation (model predictions are confirmed) and nomothetic span in that individual difference in performance on the standardized version of the test correlate meaningfully with other variables. Nomothetic span and construct representation aspects of construct validity can complement each other. As an example, the construct representation analysis of Carpenter et al. (1990) is supported by correlational analyses showing that working memory tests but not tests of short term memory are related to measures of fluid intelligence ( Engle et al. 1999 ). On the other hand, measures may have developed evidence of construct validity of one sort but not the other. Most IQ measures have excellent nomothetic span but limited construct representation: scores predict many things, but the specific psychological processes underlying responses (and those underlying processes common across measures), is generally unknown. The converse may also be true. As Whitely (1983) describes, Posner's (1978) verbal encoding task has excellent construct representation: the psychological mechanisms underlying performance are well established. However, the task has poor nomothetic span because individual differences on that task do not correlate well with scores on other measures of verbal encoding ( Whitely 1983 ). Construct representation research in clinical psychologyConstruct representation has been understudied in clinical psychology research, particularly in clinical neuropsychology and experimental psychopathology. Theories of schizophrenia, depression, and other disorders emphasize disruptions in cognitive processes, and the nomothetic span of a number of tests within neuropsychology, cognitive psychology, and clinical cognitive neuroscience paradigms are well established. But the construct representation of such tests is often less well-developed: many are psychologically complex, many are adaptations of paradigms developed for studying normal cognition and, at least in the case of schizophrenia research, many are poorly understood in terms of the underlying processes accounting for task deficits ( Strauss 2001 ; Strauss & Summerfelt 1994 ). How construct representation may be relevant to research with personality or symptom self reports or interviews is unclear and a topic for further conceptual analysis and research. Although construct representation and nomothetic span are distinct, one can influence the other. Performance on cognitive and neuropsychological tasks involves the operation of multiple cognitive processes, each of which may be reliably measured by the task. However, some of the reliable variance may well be construct-irrelevant, ( Messick 1995 ; Silverstein 2008 ). In such instances group differences on a task as well as associations between task performance and conceptually relevant other variables (i.e., apparent nomothetic span) may be due to such reliable but construct-irrelevant variance ( Messick 1995 ; Silverstein 2008 ). Theoretical progress in clinical cognitive neuroscience and experimental psychopathology depend on the conjoint analysis of nomothetic span and construct representation in the evaluation of the construct validity of measures. Conjoint analysis of nomothetic span and construct representation is also important for theory development in the study of personality traits and symptoms, especially as the field becomes more focused on neurobiological processes in personality and psychopathology. For example, there are at least 27 studies of the relation of impulsivity to the Iowa Gambling Task, a proposed measure of neurobiologically based deficits in decision making (PsychInfo Search, July 1, 2008 with terms “Iowa Gambling Task and Impulsivity”). However, none of these studies has evaluated the construct representation of the task, which is necessary to develop links between neurobiology, psychological processes and individual differences in impulsivity. An excellent example of the conjoint evaluation construct representation and nomothetic span is the work of Richard DePue, who has proposed a detailed theory of the biology of extraversion and its link with psychopathology (e.g., Depue & Collins 1999 ). The incorporation of converging operations ( Garner et al. 1956 ) into research designs can facilitate the analysis of construct representation and identify the extent to which correlations between performance and other variables reflect construct-relevant associations. For clinical research, the ability of different tasks or individual difference measures to differentially predict markers of observable, clinically important behaviors speaks to the presence of substantial construct-relevant variance ( Hammond et al. 1986 ). Establishing the construct representation of a measure requires an explicit theoretical analysis of test performance and empirical tests of the theory ( Whitely 1983 ). An example of such a research program is the experimental analysis of the basis of schizophrenia patients’ error patterns on the A-X CPT, a form of vigilance task widely used in schizophrenia research (see Cornblatt & Keilp 1994 ; Nuechterlein 1991 ). In the A-X CPT, subjects must respond to the brief occurrence of an X in a rapidly changing sequence of letters, but only if the X is preceded by an A ( Cohen et al. 1999 ). Experiments evaluating a theory of construct representation in this task suggested deficits in context representation as the most fruitful interpretation of task performance. A number of experiments using converging operations along with manipulations of theoretically proposed constituent processes have converged on this conclusion (see Barch 2005 ; Cohen et al. 1999 ). There is also substantial evidence of nomothetic span validity for the A-X CPT, including the specificity of the deficit to schizophrenia among psychotic disorders, as well as association with specific symptoms, intellectual function, and genetic liability to schizophrenia spectrum disorders ( Barch et al. 2003 ; MacDonald et al. 2005 ). Other research programs suggest that this deficit may be an instance of a more general deficit in contextual coordination at both the behavioral and neural levels ( Phillips & Silverstein 2003 ; Uhlhaas & Silverstein 2005 ). Construct HomogeneityOver the last 10 to 15 years, psychometric theory has evolved in a fundamental way that is crucial for psychopathology researchers to appreciate. In the past, psychometrics writers argued for the importance of including items on scales that tap a broad range of content. Researchers were taught to avoid including items that were highly redundant with each other, because then the breadth of the scale would be diminished and the resulting high reliability would be associated with an attenuation of validity ( Loevinger 1954 ). To take the logic further, researchers were sometimes encouraged to choose items that were largely uncorrelated with each other, so that each new item could add the most possible incremental predictive validity over the other items ( Meehl 1992 ). In recent years, a number of psychometricians have identified a core difficulty with this approach. If items are only moderately inter-correlated, it is likely that they do not represent the same underlying construct. As a result, the meaning of a score on such a test is unclear. Edwards (2001) noted that researchers have long appreciated the need to avoid heterogeneous items: if such an item predicts a criterion, one will not know which aspect of the item accounts for the covariance. The same reasoning extends to tests: if one uses a single score from a test with multiple dimensions, one cannot know which dimensions account for the test's covariance with measures of other constructs. There are two sources of uncertainty built into any validation test that uses a single score to reflect multiple dimensions. The first is that one cannot know the nature of the different dimensions’ contributions to that score, and hence to correlations of the measure with measures of other constructs. The second source of uncertainty is perhaps more severe than the first. The same composite score is likely to reflect different combinations of constructs for different members of the sample. McGrath (2005) clarified this point by drawing a useful distinction between psychological constructs that represent variability on a single dimension, on the one hand, and concepts designed to refer to covariations among unidimensional constructs on the other hand. Consider the NEO-PI-R measure of the five factor model of personality ( Costa & McCrae 1992 ). One of the five factors is neuroticism, which is understood to be composed of six, elemental constructs. Two of those are angry hostility and self-consciousness. Measures of those two traits covary reliably; they consistently fall on a neuroticism factor in exploratory factor analyses conducted in different samples and across cultures ( McCrae et al. 1996 ). However, they are not the same construct. Their correlation was .37 in the standardization sample; they share only 14% of their variance. When concerned with theoretical issues it is appropriate to disattenuate correlations for unreliability. In this instance the common variance between angry hostility and self-consciousness, corrected for unreliability, is estimated to be 19%. Clearly, one person could be high in angry hostility and low in self-consciousness, and another could be low in angry hostility and high in self-consciousness. Those two different patterns could produce exactly the same score on neuroticism as measured by the NEO-PI-R, even though the two traits may have importantly different correlates. For example, the consensus view of psychopathy, based on both expert ratings and measurement, involves being unusually high in angry hostility and unusually low in self-consciousness ( Lynam & Widiger 2007 ). Thus, it makes sense to develop theories relating angry hostility, or self-consciousness, to other constructs, and tests of such theories would be coherent. However, a theory relating overall neuroticism to other constructs must be imprecise and unclear because of the relative independence of the facets of the construct. If neuroticism correlates with another measure, one does not know which traits account for the covariation, or even whether the same traits account for the covariation for each member of the sample. The use of a neuroticism score, obtained as a summation of scores on several, separable traits, is problematic because it introduces theoretical imprecision. That observation is separate from the theoretical claim that there is a unidimensional construct, whether referred to as negative affectivity or emotional instability, which relates to variability on each lower level construct within the broad neuroticism domain. There is, of course, considerable empirical support for that claim ( Costa & McCrae 1992 ; Watson et al. 1988a ), as well as support for the view that each lower level construct shares variance with both general negative affectivity and also has variance specific to the lower level construct ( Krueger et al. 2001 ). We are noting that since the specific variance for each lower level construct can be substantial, summing scores on the lower level constructs to obtain a single overall score introduces theoretical and empirical imprecision as we described above. Hough & Schneider (1995) , McGrath (2005) , Paunonen & Ashton (2001) , Schneider et al. (1996) , and Smith et al. (2003) , among others, have all noted that use of scores of broad measures often obscures predictive relationships. Paunonen (1998) and Paunonen & Ashton (2001) have shown that prediction of theoretically relevant criteria is improved when one uses facets of the big five personality scales, rather than the composite, big five dimensions themselves. Using the NEO-PI-R operationalization of the five factor model of personality, Costa & McCrae (1995) compared different facets of conscientiousness in their prediction of aspects of occupational performance. Dutifulness was related to service orientation (.35) and employee reliability (.38), but achievement striving was not (−.01 and .02, respectively). In contrast, achievement striving was related to sales potential (.22), but dutifulness was not (.06). By definition, correlations of broad conscientiousness (which on the NEO-PI-R sums these two facets with four other facets) will produce correlations in between the high and low values, because the sum effectively averages the different effects of the different facets. Use of the broad score would obscure the different roles of the different facets of conscientiousness. Should one wish to represent the full domain of a higher order dimension, such as conscientiousness or neuroticism, one can include each lower level facet as part of a multivariate analysis (such as multiple regression); doing so preserves the theoretical precision inherent in precise constructs while representing the full variance of the higher order domain ( Goldberg 1993 ; Nunnally & Bernstein 1994 ). Recently, this perspective has been extended to the study of disorders. For example, McGrath (2005) , noting that individuals can obtain the same depression scores with very different symptom patterns, describes depression as a useful social construction but not a coherent psychological entity that can be used in validation studies. Indeed, using factor analysis, Jang et al. (2004) identified 14 subfactors in a set of depression measures. Examples included “feeling blue and lonely,” “insomnia,” “positive affect,” “loss of appetite,” and “psychomotor retardation.” They found that the inter-correlations among the factors ranged from .00 to .34; further, the factors were differentially heritable, with heritability coefficients ranging from .00 to .35. Evidence of multidimensionality is accruing for many disorders, including post-traumatic stress disorder ( King et al. 1998 ; Simms et al. 2002 ), psychopathy ( Brinkley et al. 2004 ), schizotypal personality disorder ( Fossati et al. 2005 ), and many others ( Smith & Combs, 2008 ). For scientific clinical psychology to advance, researchers should study cohesive, unidimensional constructs. To use multi-faceted, complex constructs as predictors or criteria in validity or theory studies is difficult to defend. Researchers are encouraged to generate theories that identify putatively homogenous, coherent constructs. It may often be useful to compare the theory that a putative attribute is homogeneous to the theory that it is a combination of separate attributes. The success of such efforts in the recent past bodes well for continued progress in the field as researchers study unidimensional constructs with meaningful test scores ( Jang et al. 2004 ; Smith et al. 2007 ; Whiteside & Lynam 2001 ). This discussion of construct homogeneity raises two important issues. The first is, when is a construct measure elemental enough? There is a risk of continually parsing constructs until one is left with a content domain specific to a single item, thus losing full coverage of a target construct and attenuating predictive power. We believe the guiding consideration should be theoretical clarity. When there is good theoretical or empirical reason to believe that an item set actually consists of two, separately definable constructs with different psychological meaning, and when those two constructs could reasonably have meaningfully different external correlates, measuring the two separately is likely to improve both understanding and empirical prediction. When there is no sound theoretical basis to separate items into multiple constructs, one should perhaps avoid doing so. The second issue is whether a focus on construct homogeneity leads to a clear and unacceptable loss of parsimony. This possibility merits careful consideration. With respect to etiological models, the use of several homogeneous constructs rather than their aggregate can complicate theory testing, but that difficulty must be weighed against the improved precision of theory tests. It is at least possible that an emphasis on construct homogeneity often does not compromise parsimony. For example, it appears to be the case that four broad personality dimensions and their underlying facets effectively describe the many different forms of dysfunction currently represented by the full set of personality disorders ( Widiger & Simonsen 2005 ; Widiger et al. 2005 ). Perhaps it is instead the case that parsimony has been compromised by the current DSM system that names multiple putative syndromes that often appear to reflect slightly different combinations of personality dimensions. It may be that parsimony would be better served by describing personality dysfunction in terms of a set of core, homogeneous personality traits rather than in terms of combinations of disparate, moderately related symptoms ( Widiger & Trull 2007 ). This logic has been extended beyond the personality disorders domain: Serretti & Olgiati (2004) described basic dimensions of psychosis that apply across current diagnostic distinctions, suggesting parsimony in the dimensional description of psychosis. Empirical Evaluation of Construct ValidityCampbell's & Fiske's (1959) multitrait-multimethod matrix methodology presented a logic for evaluating construct validity through simultaneous evaluation of convergent and discriminant validity, and the contribution of method variance to observed relationships. iv Wothke (1995) nicely summarized the central idea of MTMM matrix methodology: The crossed-measurement design in the MTMM matrix derives from a simple rationale: Traits are universal, manifest over a variety of situations, and detectable with a variety of methods. Most importantly, the magnitude of a trait should not change just because different assessment methods are used (p. 125) Traits are latent variables, inferred constructs. The term trait , as used here, is not limited to enduring characteristics; it applies as well to more transitory phenomena such as moods, emotions, as well as to all other individual differences constructs, e.g., attitudes and psychophysical measurements. Methods for Campbell and Fiske are the procedures through which responses are obtained, the operationalization of the assessment procedures that produce the responses, the quantitative summary of which is the measure itself ( Wothke 1995 ). As Campbell & Fiske (1959) emphasized, measurement methods (method variance) are sources of irrelevant, though reliable, variance. When the same method is used across measures, the presence of reliable method variance can lead to an overestimation of the magnitude of relations among constructs. This can lead to overestimating convergent validity and underestimating discriminant validity. This is why multiple assessment methods are critical in the development of construct validity. Their distinction of validity (the correlation between dissimilar measures of a characteristic) from reliability (the correlation between similar measures of a chartacteristic) hinged on the differences between construct assessment methods. Campbell & Fiske's (1959) observation remains important today: much clinical psychology research relies on the same method for both predictor and criterion measurement, typically self-report questionnaire or interview. Their call for attention to method variance is as relevant today as it was 50 years ago; examination of constructs with different methods is a crucial part of the construct validation process. Of course, the degree to which two methods are independent is not always clear. For example, how different are the methods of interview and questionnaire? Both rely on self-report, so are they independent sources of information? Perhaps not, but they do differ operationally. For example, questionnaire responses are often anonymous, whereas interview responses require disclosure to another. Questionnaire responses are based on the perceptions of the respondent, whereas interview ratings are based, in part, on the perceptions of the interviewer. A conceptually based definition of “method variance” has not been easy to achieve, as Sechrest et al.'s (2000) analysis of this issue demonstrates. Certainly, method differences lie on a continuum where for example, self-report and interview are closer to each other than self-report and informant report or behavioral observation. The guidance provided for evaluating construct validity in 1959 was qualitative; it involved the rule-based examination of patterns of correlations against the expectations of convergent and discriminant validity ( Campbell & Fiske 1959 ). Developments in psychometric theory, multivariate statistics and analysis of latent traits in the decades since the Campbell & Fiske (1959) paper have made available a number of quantitative methods for modeling convergent and discriminant validity across different assessment methods. Bryant (2000) provides a particularly accessible description of using ANOVA (and a nonparametric variant) and confirmatory factor analysis (CFA) in the analysis of MTMM matrices. A major advantage of CFA in construct validity research is the possibility of directly comparing alternative models of relationships among constructs, a critical component of theory testing (see Whitely 1983 ). Covariance component analysis of the MTMM matrix has also been developed ( Wothke 1995 ). Both covariance component analysis and CFA are variants of structural equation models (SEM). With these advances eye-ball examinations of MTMM matrices are no longer sufficient for the evaluation of the trait validity of a measure in modern assessment research. Perhaps the first CFA approach was one that followed very straightforwardly from Campbell & Fiske (1959) : it involved specifying a CFA model in which responses to any item can be understood as reflecting additive effects of trait variance, method variance, and measurement error ( Marsh & Grayson 1995 ; Reichardt & Coleman 1995 ; Widaman 1985 ). So if traits A, B, and C are each measured with methods X, Y, and Z, there are six latent variables: three for the traits and three for the methods. Thus, if indicator i reflects method X for evaluating trait A, that part of the variance of i that is shared with other indicators of trait A is assigned to the trait A factor, that part of the variance of i that is shared with indicators of other constructs measured by method X is assigned to the method X factor, and the remainder is assigned to an error term ( Eid et al. 2003 ; Kenny & Kashy 1992 ). The association of each type of factor with other measures can be examined, so, for example, one can test explicitly the role of a certain trait or a certain type of method variance on responses to a criterion measure. This approach can be expanded to include interactions between traits and methods ( Campbell & O'Connell 1967 , 1982 ), and therefore test multiplicative models ( Browne 1984 ; Cudeck 1988 ). Although the potential advantages of this approach are obvious, it has generally not proven feasible. As noted by Kenny & Kashy (1992) , this approach often results in modeling more factors than there is information to identify them; the result, often, is a statistical failure to converge on a factor solution. That reality has led some researchers to turn away from multivariate statistical methods to evaluate MTMM results. In recent years, however, two alternative CFA modeling approaches have been developed that appear to work well. The first is referred to as the “correlated uniquenesses” approach ( Marsh & Grayson 1995 ). In this approach, one does not model method factors as in the approach previously described. Instead, one identifies the presence of method variance by allowing the residual variances of trait indicators that share the same method to correlate, after accounting for trait variation and covariation. To the degree there are substantial correlations between these residual terms, method variance is considered present and is accounted for statistically (although other forms of reliable specificity may be represented in those correlations as well). As a result, the latent variables reflecting trait variation do not include that method variance: one can test the relation between method-free trait scores and other variables of interest. And, since this approach models only trait factors, it avoids the over-factoring problem of the earlier approach. There is, however, an important limitation to the correlated uniquenesses approach. Without a representation of method variance as a factor, one cannot examine the association of method variance with other constructs, which may be important to do ( Cronbach 1995 ). The second alternative approach provides a way to model some method variance while avoiding the over-factoring problem ( Eid et al. 2003 ). One constructs latent variables to represent all trait factors and all but one method factor. Since there are fewer factors than in the original approach, the resulting solution is mathematically identified: one has not over-factored. The idea is that one method is chosen as the baseline method and is not represented by a latent variable. One evaluates other methods for how they influence results compared to the baseline method. Suppose, for example, that one had interview and collateral report data for a series of traits. One might specify the interview method as the baseline method, so an interview method factor is not modeled as separate from trait variance, and trait scores are really trait-as-measured-by-interview scores. One then models a method factor for collateral report. If the collateral report method leads to higher estimates of trait presence than does the interview, one would find that the collateral report method factor correlated positively with the trait-as-measured-by-interview. That would imply that collaterals report higher levels of the trait than do individuals during interviews. Interestingly, one can assess whether this process works differently for different traits. Perhaps collaterals perceive higher levels of some traits than are reported by interview (unflattering traits?) and lower levels of other traits as reported by interview (flattering traits?). This possibility can be examined empirically using this method. In this way, the Eid et al. (2003) approach makes it possible to identify the contribution of method to measure scores. The limitation of this method, of course, is that the choice of “baseline method” influences the results and may be arbitrary ( Eid et al. 2003 ). Most recently, Courvoisier et al. (2008) have combined this approach with latent state-trait analysis; the latter method allows one to estimate variance due to stable traits, occasion-specific states, and error ( Steyer et al. 1999 ). The result is a single analytic method to estimate variance due to trait, method, state, and error. Among the possibilities offered by this approach is that one can investigate the degree to which method effects are stable or variable over time. We wish to emphasize three points concerning these advances in methods for the empirical evaluation of construct validity. First, the concern that MTMM data could not successfully be analyzed using CFA/SEM approaches is no longer correct. There are now analytic tools that have proven successful ( Eid et al. 2003 ). Second, statistical tools are available that enable one to quantitatively estimate multiple sources of variance that are important to the construct validation enterprise ( Eid et al. 2003 ; Marsh & Grayson 1995 ). One need not guess at the degree to which method variance is present, or the degree to which it is common across traits, or the degree to which it is stable: one can investigate these sources of variance directly. Third, these analytic techniques are increasingly accessible to researchers (see Kline 2005 , for a useful introduction to SEM). Clinical researchers have a validity concern beyond successful demonstration of convergent and discriminant validity. Success at the level of MTMM validity does not assure the measured traits have utility. Typically, one also needs to investigate whether the traits enhance prediction of some criterion of clinical importance. To this end, clinical researchers can rely on a classic contribution by Hammond et al. (1986) . They offered a creative, integrative analytic approach for combining the results of MTMM designs with the evaluation of differential prediction of external criteria. In the best tradition of applying basic science advances to practical prediction, their design integrated the convergent/discriminant validity perspective of Campbell & Fiske (1959) with Brunswik's (1952 , 1956) emphasis on representative design in research, which in part concerned the need to conduct investigations that yield findings one can apply to practical problems. They presented the concept of a performance validity matrix, which adds criterion variables for each trait to the MTMM design. By adding clinical outcome variables to one's MTMM design, one can provide evidence of convergent validity, discriminant validity, and differential clinical prediction in a single study. Such analyses are critical clinically, because this sophisticated treatment of validity is likely to improve the usefulness of measures for clinicians. For many measures, validation research that considers practical prediction improves measures’ “three Ps”: predicting important criteria; prescribing treatments, and understanding the processes underlying personality and psychopathology ( Youngstrom 2008 ), thereby improving clinical assessment. Such practical efforts in assessment must rely on observed scores, confounded as they may be with method variance. Construct validity research provides the clinician with an appreciation of the many factors entering into an observed score and, thus, appreciation of the mix of construct-relevant, reliable construct-irrelevant variance and method variance in any score. (see Richters 1992 ). The term “construct validation” refers to the process of simultaneously validating measures of psychological constructs and the theories of which the constructs are a part. The study of the construct validation process is ongoing. It rests on core principles identified 50 years ago ( Campbell & Fiske 1959 ; Cronbach & Meehl 1955 ; Loevinger 1957 ; MacCorquodale & Meehl 1948 ); those principles remain central to theory testing today. It is also true that our understanding of construct validation has evolved over these 50 years. In this chapter, we emphasized five ways in which this is true. First, advances in philosophy of science have helped clarify the ongoing, indeterminate nature of the construct validation process. This quality of theory testing represents a strength to the scientific method, because it reflects the continuing process of critical evaluation of all aspects of theory and measurement. Second, theoreticians now emphasize the pursuit of informative theory tests, in order to avoid weak, ad hoc theory tests in the absence of fully specified theories. Third, the need to validate clinical, laboratory tasks, by investigating the degree to which responses on a task do reflect the influence of the target construct of interest, is becoming increasingly appreciated. Fourth, the lack of clarity that follows the use of a single score to represent multidimensional constructs has been described; researchers are increasingly relying on unidimensional measures to improve the validity of their theory tests. And fifth, important advances in the means to evaluate validity evidence empirically have been described; researchers have important new statistical tools at their disposal. In sum, there are exciting new developments in the study of how to validate theories and their accompanying measures. These advances promise important improvements in measure and theory validation. As researchers fully incorporate sound construct validation theory in their methods, the rate of progress in clinical psychology research will continue to increase. AcknowledgmentsWe thank Lee Anna Clark and Eric Youngstrom for their most helpful comments and suggestions, and Jill White for her excellent work in preparing the ms. Portions of this work were supported by NIAAA grant 1 RO 1 AA 016166 to Gregory T. Smith. Acronyms Used
Summary of Central Points: 1. The core perspective on construct validation can be understood by considering four classic papers published 50 or more years ago: Campbell & Fiske (1959) , Cronbach & Meehl (1955) , Loevinger (1957) , and MacCorquodale & Meehl (1948) . 2. Measures of psychological constructs are validated by testing whether they relate to measures of other constructs as specified by theory. Each test of relations between measures reflects on the validity of both the measures and the theory driving the test. Construct validation concerns the simultaneous process of measure and theory validation. 3. Current nonjustificationist philosophy of science indicates that no single experiment fully proves or disproves a theory. Instead, evidence for the validity of a measure and of a theory accrues over time, and reflects an analysis of the evidence for and against theory claims. 4. Validation theorists are promoting an increased reliance on theory in clinical research, for, it is argued, advances in knowledge are facilitated by theory-driven research 5. Experimental psychopathology researchers using laboratory tasks should seek evidence that variation in performance on a task reflects variation in the psychological processes of interest more than reliably measured but theoretically irrelevant constructs. 6. Validation efforts benefit when single scores reflect variation on a single dimension of psychological functioning. If a single score reflects multiple dimensions, variation among individuals on that score lacks unambiguous meaning. 7. New statistical tools are available for the quantitative investigation of many aspects of construct validity, including the assessment of convergent and discriminant validity. These tools are increasingly accessible to researchers. Brief Annotations: Borsboom D, Mellenbergh GJ, van Heerden J. 2004. The concept of validity. Psychol. Rev. 111:1061−71. A thoughtful overview of philosophical, methodological and substantive issues in validity theory. Campbell DT, Fiske DW. 1959. Convergent and discriminant validation by the multitraitmultimethod matrix. Psychol. Bull. 56(2):81−105. The classic introduction of multi-trait, multi-method methodology. Cronbach LJ. 1988. Five perspectives on validation argument. In Test Validity, ed. H Wainer, H Braun, pp. 3−17. Hillsdale, NJ: Erlbaum. An insightful review of the extent of progress in implementing sound validation procedures. Cronbach LJ, Meehl PE. 1955. Construct validity in psychological tests. Psychol. Bull. 52:281−302. One of the defining articles on validation theory in psychology, it played a central role in introducing the concept of construct validity to psychology research. Whitely (now Embretson) SE. 1983. Construct validity: construct representation versus nomothetic span. Psychol. Bull. 93:179−97. The seminal analysis of the distinction between nomothetic span and construct representation. Garner WR, Hake HW, Eriksen CW. 1956. Operationism and the concept of perception. Psychol. Rev. 63(3):149−59. A seminal analysis of the critical role of multiple methods for the definition of a construct. Loevinger J. 1957. Objective tests as instruments of psychological theory. Psychol. Rep. Monogr. Supp. 3:635−94. An important, integrative presentation of construct validity as subsuming specific forms of validation tests. MacCorquodale K, Meehl PE. 1948. On a distinction between hypothetical constructs and intervening variables. Psychol. Rev. 55(2):95−107. This paper promoted the philosophical legitimacy of hypothetical constructs. Messick S. 1995. Validity of psychological assessment: validation of inferences from persons’ responses and performances as scientific inquiry into score meaning. Am. Psychol. 50(9):741−49. An important paper emphasizing that validity refers to interpretations of test scores. Smith GT. 2005a. On construct validity: issues of method and measurement. Psychol. Assessment 17:396−408. This paper reviewed recent advances in construct validation theory, including increased precision in differentiating among clinical constructs, and ongoing efforts to improve the construct validation process. 1. Construct: A psychological process or characteristic believed to account for individual or group differences in behavior. 2. Construct validity: evaluation of the extent to which a measure assesses the construct it is deemed to measure. 3. Convergent validity: The relationship among different measures of the same construct. 4. Discriminant validity: Demonstrations that a measure of a construct is unrelated to indicators of theoretically irrelevant constructs in the same domain. 5. Method variance: The association among variables due to the similarity of operations in the measurement of these variables. 6. Multitrait-Multimethod Matrix: Method for evaluating the relative contribution of trait-related and method variance to the correlations among measures of multiple constructs. 7. Nomothetic Span: The meaning of a construct as established though its network of relationships with other constructs. 8. Construct representation: The analysis of psychological processes accounting for responses on a task. 9. Nonjustificationist: The philosophy of science that proposes that no theory is ever fully proven or disproven; rather, theories are selected on the basis of which one of several the bulk of evidence favors. 10. Construct homogeneity: The view that a single score should reflect variation on only a single construct. iii Discriminant validity is sometimes erroneously referred to as divergent validity. iv Campbell and Fiske were concerned with correlation designs relevant to research on traits and other individual differences constructs. Similar issues apply to experimental psychology paradigms as Garner et al. (1956) described in their discussion of convergent operations. This article is particularly relevant to experimental psychopathology where multiple paradigms for the study of cognitive impairments in patients is particularly important for the identification of disordered cognitive mechanisms, structures, or processes (e.g., see Knight & Silverstein 2001 ) LITERATURE CITED
![]() |
IMAGES
VIDEO
COMMENTS
The 4 Types of Validity in Research | Definitions & Examples. Published on September 6, 2019 by Fiona Middleton.Revised on June 22, 2023. Validity tells you how accurately a method measures something. If a method measures what it claims to measure, and the results closely correspond to real-world values, then it can be considered valid.
Reliability vs. Validity in Research | Difference, Types and ...
Examples of Validity. Internal Validity: A randomized controlled trial (RCT) where the random assignment of participants helps eliminate biases. External Validity: A study on educational interventions that can be applied to different schools across various regions. Construct Validity: A psychological test that accurately measures depression levels.
Fundamental concepts of validity, reliability, and generalizability as applicable to qualitative research are then addressed with an update on the current views and controversies. Keywords: Controversies, generalizability, primary care research, qualitative research, reliability, validity. Source of Support: Nil.
Validity in research is the ability to conduct an accurate study with the right tools and conditions to yield acceptable and reliable data that can be reproduced. Researchers rely on carefully calibrated tools for precise measurements. However, collecting accurate information can be more of a challenge. Studies must be conducted in environments ...
Reliability vs Validity in Research | Differences, Types ...
The validity of a research study includes two domains: internal and external validity. Internal validity is defined as the extent to which the observed results represent the truth in the population we are studying and, thus, are not due to methodological errors. In our example, if the authors can support that the study has internal validity ...
As with validity, reliability is an attribute of a measurement instrument - for example, a survey, a weight scale or even a blood pressure monitor. But while validity is concerned with whether the instrument is measuring the "thing" it's supposed to be measuring, reliability is concerned with consistency and stability.
Construct Validity | Definition, Types, & Examples. Published on February 17, 2022 by Pritha Bhandari.Revised on June 22, 2023. Construct validity is about how well a test measures the concept it was designed to evaluate. It's crucial to establishing the overall validity of a method.. Assessing construct validity is especially important when you're researching something that can't be ...
This paper claims that R.J. Herrnstein and C. Murray's The Bell Curve: ... Review of validity research on the stanford-binet intelligence scale: 4th Ed. Psychological Assessment. 102-112. This paper looks at the results of construct and criterion- related validity studies to determine if the SB:FE is a valid measure of intelligence.
Reliability refers to the consistency of the measurement. Reliability shows how trustworthy is the score of the test. If the collected data shows the same results after being tested using various methods and sample groups, the information is reliable. If your method has reliability, the results will be valid. Example: If you weigh yourself on a ...
Peer debriefing is a form of external evaluation of the qualitative research process. Lincoln and Guba (1985, p. 308) describe the role of the peer reviewer as the "devil's advocate.". It is a person who asks difficult questions about the procedures, meanings, interpretations, and conclusions of the investigation.
Research validity in surveys relates to the extent at which the survey measures right elements that need to be measured. In simple terms, validity refers to how well an instrument as measures what it is intended to measure. Reliability alone is not enough, measures need to be reliable, as well as, valid. For example, if a weight measuring scale ...
Reliability and validity: Importance in Medical Research
Internal validity examines whether the study design, conduct, and analysis answer the research questions without bias. External validity examines whether the study findings can be generalized to other contexts. Ecological validity examines, specifically, whether the study findings can be generalized to real-life settings; thus ecological ...
Abstract. This article examines reliability and validity as ways to demonstrate the rigour and trustworthiness of quantitative and qualitative research. The authors discuss the basic principles of ...
Internal validity makes the conclusions of a causal relationship credible and trustworthy. Without high internal validity, an experiment cannot demonstrate a causal link between two variables. Research example. You want to test the hypothesis that drinking a cup of coffee improves memory. You schedule an equal number of college-aged ...
(PDF) Validity and Reliability of the Research Instrument
Without rigor, research is worthless, becomes fiction, and loses its utility. Hence, a great deal of attention is applied to reliability and validity in all research methods. Challenges to rigor in qualitative inquiry interestingly paralleled the blossoming of statistical packages and the development of computing systems in quantitative research.
In this paper, we review factors that introduce biases in RCTs and we propose quantitative and qualitative strategies for colleting relevant data to strengthen internal validity. The factors are related to participants' reactions to randomization, attrition, treatment perceptions, and implementation of the intervention.
Threats to external validity and how to counter them. Threats to external validity are important to recognize and counter in a research design for a robust study. Research example A researcher wants to test the hypothesis that people with clinical diagnoses of mental disorders can benefit from practicing mindfulness daily in just two months ...
The validity and reliability of the scales used in research are important factors that enable the research to yield healthy results. For this reason, it is useful to understand how the reliability ...
Construct validity research provides the clinician with an appreciation of the many factors entering into an observed score and, thus, appreciation of the mix of construct-relevant, reliable construct-irrelevant variance and method variance in any score. ... An important paper emphasizing that validity refers to interpretations of test scores ...