
An official website of the United States government
The .gov means it’s official. Federal government websites often end in .gov or .mil. Before sharing sensitive information, make sure you’re on a federal government site.
The site is secure. The https:// ensures that you are connecting to the official website and that any information you provide is encrypted and transmitted securely.
- Publications
- Account settings
- My Bibliography
- Collections
- Citation manager
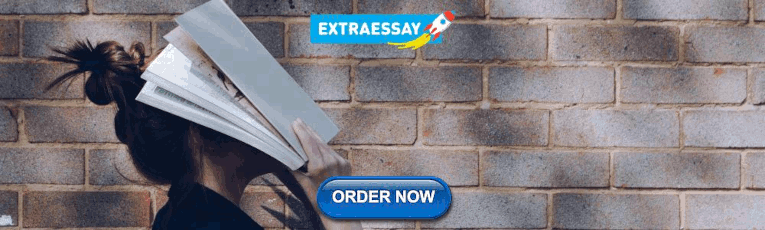
Save citation to file
Email citation, add to collections.
- Create a new collection
- Add to an existing collection
Add to My Bibliography
Your saved search, create a file for external citation management software, your rss feed.
- Search in PubMed
- Search in NLM Catalog
- Add to Search
A review on genetic algorithm: past, present, and future
Affiliation.
- 1 Computer Science and Engineering Department, National Institute of Technology, Hamirpur, India.
- PMID: 33162782
- PMCID: PMC7599983
- DOI: 10.1007/s11042-020-10139-6
In this paper, the analysis of recent advances in genetic algorithms is discussed. The genetic algorithms of great interest in research community are selected for analysis. This review will help the new and demanding researchers to provide the wider vision of genetic algorithms. The well-known algorithms and their implementation are presented with their pros and cons. The genetic operators and their usages are discussed with the aim of facilitating new researchers. The different research domains involved in genetic algorithms are covered. The future research directions in the area of genetic operators, fitness function and hybrid algorithms are discussed. This structured review will be helpful for research and graduate teaching.
Keywords: Crossover; Evolution; Genetic algorithm; Metaheuristic; Mutation; Optimization; Selection.
© Springer Science+Business Media, LLC, part of Springer Nature 2020.
PubMed Disclaimer
Classification of metaheuristic Algorithms
Operators used in GA
Swapping genetic information after a…
Swapping genetic information after a crossover point
Swapping genetic information between crossover…
Swapping genetic information between crossover points
Swapping individual genes
Partially matched crossover (PMX) [117]
Cycle Crossover (CX) [140]
Local and global optima [149]
- Abbasi M, Rafiee M, Khosravi MR, Jolfaei A, Menon VG, Koushyar JM (2020) An efficient parallel genetic algorithm solution for vehicle routing problem in cloud implementation of the intelligent transportation systems. Journal of cloud Computing 9(6)
- Abdelghany A, Abdelghany K, Azadian F. Airline flight schedule planning under competition. Comput Oper Res. 2017;87:20–39.
- Abdulal W, Ramachandram S. Reliability-aware genetic scheduling algorithm in grid environment. Katra, Jammu: International Conference on Communication Systems and Network Technologies; 2011. pp. 673–677.
- Abdullah J. Multiobjectives ga-based QoS routing protocol for mobile ad hoc network. Int J Grid Distrib Comput. 2010;3(4):57–68.
- Abo-Elnaga Y, Nasr S. Modified evolutionary algorithm and chaotic search for Bilevel programming problems. Symmetry. 2020;12:767.
LinkOut - more resources
Full text sources.
- Europe PubMed Central
- PubMed Central
Other Literature Sources
- The Lens - Patent Citations
Miscellaneous
- NCI CPTAC Assay Portal
- Citation Manager
NCBI Literature Resources
MeSH PMC Bookshelf Disclaimer
The PubMed wordmark and PubMed logo are registered trademarks of the U.S. Department of Health and Human Services (HHS). Unauthorized use of these marks is strictly prohibited.
Click through the PLOS taxonomy to find articles in your field.
For more information about PLOS Subject Areas, click here .
Loading metrics
Open Access
Peer-reviewed
Research Article
An improved genetic algorithm and its application in neural network adversarial attack
Contributed equally to this work with: Dingming Yang, Zeyu Yu, Hongqiang Yuan
Roles Conceptualization, Data curation, Formal analysis, Methodology, Software, Validation, Writing – original draft, Writing – review & editing
Affiliation School of Computer Science, Yangtze University, Jingzhou, China

Roles Funding acquisition, Supervision, Validation, Writing – review & editing
Affiliation School of Electronic & Information, Yangtze University, Jingzhou, China
Roles Funding acquisition, Resources, Writing – review & editing
Affiliation School of Urban Construction, Yangtze University, Jingzhou, China
Roles Conceptualization, Project administration, Resources, Supervision, Writing – review & editing
* E-mail: [email protected]
- Dingming Yang,
- Zeyu Yu,
- Hongqiang Yuan,
- Yanrong Cui
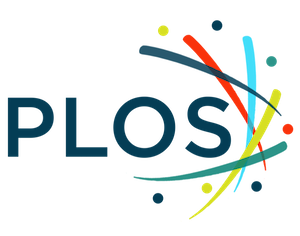
- Published: May 5, 2022
- https://doi.org/10.1371/journal.pone.0267970
- Reader Comments
The choice of crossover and mutation strategies plays a crucial role in the searchability, convergence efficiency and precision of genetic algorithms. In this paper, a novel improved genetic algorithm is proposed by improving the crossover and mutation operation of the simple genetic algorithm, and it is verified by 15 test functions. The qualitative results show that, compared with three other mainstream swarm intelligence optimization algorithms, the algorithm can not only improve the global search ability, convergence efficiency and precision, but also increase the success rate of convergence to the optimal value under the same experimental conditions. The quantitative results show that the algorithm performs superiorly in 13 of the 15 tested functions. The Wilcoxon rank-sum test was used for statistical evaluation, showing the significant advantage of the algorithm at 95% confidence intervals. Finally, the algorithm is applied to neural network adversarial attacks. The applied results show that the method does not need the structure and parameter information inside the neural network model, and it can obtain the adversarial samples with high confidence in a brief time just by the classification and confidence information output from the neural network.
Citation: Yang D, Yu Z, Yuan H, Cui Y (2022) An improved genetic algorithm and its application in neural network adversarial attack. PLoS ONE 17(5): e0267970. https://doi.org/10.1371/journal.pone.0267970
Editor: Mohd Nadhir Ab Wahab, Universiti Sains Malaysia, MALAYSIA
Received: November 24, 2021; Accepted: April 19, 2022; Published: May 5, 2022
Copyright: © 2022 Yang et al. This is an open access article distributed under the terms of the Creative Commons Attribution License , which permits unrestricted use, distribution, and reproduction in any medium, provided the original author and source are credited.
Data Availability: All relevant data are within the paper and its Supporting information files.
Funding: D.Y., Z.Y., H.Y. and Y.C.; This work was supported by the Major Technology Innovation of Hubei Province [2019AAA011]. The funders had no role in study design, data collection and analysis, decision to publish, or preparation of the manuscript.
Competing interests: The authors have declared that no competing interests exist.
1 Introduction
In real life, optimization problems such as shortest path, path planning, task scheduling, parameter tuning, etc. are becoming more and more complex and have complex features such as nonlinear, multi-constrained, high-dimensional, and discontinuous [ 1 ]. Although a series of artificial intelligence algorithms represented by deep learning can solve some optimization problems, they lack mathematical interpretability due to the existence of a large number of nonlinear functions and parameters inside their models, so they are difficult to be widely used in the field of information security. Traditional optimization algorithms and artificial intelligence algorithms can hardly solve complex optimization problems with high dimensionality and nonlinearity in the field of information security.
Therefore, it is necessary to find an effective optimization algorithm to solve such problems. In this background, various swarm intelligence optimization algorithms have been proposed one after another, such as Particle Swarm Optimization(PSO) [ 2 , 3 ], Grey Wolf Optimizer(GWO) [ 4 ], etc. Subsequently, a variety of improved optimization algorithms also have been proposed one after another. For example, the improved genetic algorithm for cloud environment task scheduling [ 5 ], the improved genetic algorithm for flexible job shop scheduling [ 6 ], the improved genetic algorithm for green fresh food logistics [ 7 ], etc.
However, these improved optimization algorithms are improved for domain-specific optimization problems and do not improve the accuracy, convergence efficiency and generalization of the algorithms themselves. In this paper, the crossover operator and mutation operator of the genetic algorithm are improved to improve the convergence efficiency and precision of the algorithm without affecting the effectiveness of the improved genetic algorithm on most optimization problems. The effectiveness of the improved genetic algorithm is also verified through many comparison experiments and applications in the field of neural network adversarial attacks.
- By improving the single-point crossover link of SGA, the fitness function is used as an evaluation index for selecting children after crossover, thus reducing the number of iterations and accelerating the convergence speed.
- By improving the basic bitwise mutation of the SGA, traversing each gene of the offspring and performing selective mutation on them, setting different mutation rates for two parts of a chromosome, thus improving the global search in the stable case of local optimum.
- The improved genetic algorithm is applied to the field of neural network adversarial attack, which increases the speed of adversarial sample generation and improves the robustness of the neural network model.
2 Related works
2.1 genetic algorithm.
Genetic Algorithm is a series of simulation evolutionary algorithms proposed by Holland et al. [ 8 ], and later summarized by DeJong, Goldberg and others. The general flowchart of the Genetic Algorithm is shown in Fig 1 . The Genetic Algorithm first encodes the problem, then calculates the fitness, then selects the parent and the mother by roulette, and finally generates the children with high fitness by crossover and mutation, and finally generates the individuals with high fitness after many iterations, which is the satisfied solution or optimal solution of the problem. Simple Genetic Algorithm (SGA) uses single-point crossover and simple mutation to embody information exchange between individuals and local search, and does not rely on gradient information, so SGA can find the global optimal solution.
- PPT PowerPoint slide
- PNG larger image
- TIFF original image
https://doi.org/10.1371/journal.pone.0267970.g001
2.2 Other meta-heuristic algorithms
The meta-heuristic algorithm is problem-independent, does not exploit the specificity of the problem, and is a general solution. In general, it is not greedy, can explore more search space, and tends to obtain the global optimum. To be more specific, meta-heuristic have one of the most important ideas: a dynamic balance mechanism between diversification and intensification.
The PSO [ 2 , 3 ] algorithm is a swarm intelligence-based global stochastic search algorithm inspired by the results of artificial life research and by simulating the migration and flocking behavior of bird flocks during foraging, and its basic idea is inspired by the results of research on modeling and simulation of birds flock behavior. The GWO algorithm is a swarm intelligence optimization algorithm proposed by Mirjalili et al. [ 4 ]. The algorithm is inspired by the grey wolf prey hunting activity and developed as an optimization search algorithm, which has strong convergence performance, few parameters, and easy implementation. The Marine Predator Algorithm (MPA) [ 9 ] is mainly inspired by foraging strategies widely found in marine predators, namely Lévy and Brownian motion, and optimal encounter rate strategies in biological interactions between predators and prey. The Artificial Gorilla Troops Optimizer (GTO) [ 10 ] was inspired by the gorilla group life behavior. The GTO is characterized by fast search speed and high solution accuracy. The African Vulture Optimization Algorithm(AVOA) [ 11 ] was inspired by the foraging and navigation behavior of African vultures. this algorithm is fast and has high solution accuracy which is widely used in single-objective optimization. The Remora Optimization Algorithm (ROA) [ 12 ] first proposed an intelligent optimization algorithm inspired by the biological habits of the neutrals in nature, which has good solution accuracy and high engineering practical value in both function seeking to solve extreme values and typical engineering optimization problems.
2.3 Neural network adversarial attack
Szegedy et al. [ 13 ] first demonstrated that a highly accurate deep neural network can be misled to make a misclassification by adding a slight perturbation to an image that is imperceptible to the human eye, and also found that the robustness of deep neural networks can be improved by adversarial training. Such phenomena are far-reaching and have attracted many researchers in the area of adversarial attacks and deep learning security. Akhtar and Mian [ 14 ] surveyed 12 attack methods and 15 defense methods for neural networks adversarial attacks. The main attack methods are finding the minimum loss function additive term [ 13 ], increasing the loss function of the classifier [ 15 ], the method of limiting the l_0 norm [ 16 ], changing only one pixel value [ 17 ], etc.
Nguyen et al. [ 18 ] continued to explore the question of “what differences remain between computer and human vision” based on Szegedy et al. [ 13 ]. They used the Evolutionary Algorithm to generate high-confidence adversarial images by iterating over direct-encoded images and CPPN (Compositional Pattern-Producing Network) encoded images, respectively. They obtained high-confidence adversarial samples (fooling images) using the Evolutionary Algorithm on a LeNet model pre-trained on the MNIST dataset [ 19 ] and an AlexNet model pre-trained on the ILSVRC 2012 ImageNet dataset [ 20 , 21 ], respectively.
Neural network adversarial attacks are divided into black-box attacks and white-box attacks. Black-box attacks do not require the internal structure and parameters of the neural network, and the adversarial samples can be generated with optimization algorithms as long as the output classification and confidence information is known. The study of neural network adversarial attacks not only helps to understand the working principle of neural networks but also increases the robustness of neural networks by training with adversarial samples.
3 Approaches
This section improves the single-point crossover and simple mutation of SGA. The fitness function is used as the evaluation index of the crossover link, and the crossover points of the whole chromosome are traversed to improve the efficiency of the search for the best. A selective mutation is performed for each gene of the children’s chromosome, and the mutation rate of the latter half of the chromosome is set to twice that of the first half to improve the global search under the stable situation of local optimum.
3.1 Improved crossover operation
As shown in algorithm 1 is the Python pseudocode for the improved crossover algorithm. The single-point crossover of SGA is to generate a random number within the parental chromosome length range, and then intercept the first half of the father’s chromosome and the second half of the mother’s chromosome to cross-breed the children according to the generated random number. In this paper, the algorithm is improved by trying to cross genes within the parental chromosome length range one by one, calculating the fitness, and picking out the highest fitness children individuals. Experimental data show that such an improvement can reduce the number of iterations and speed up the convergence of fitness.
Algorithm 1 Crossover with fitness as evaluation.
Input : Father’s gene, mother’s gene, fitness function;
Output : Child’s gene;
1: function CROSSOVER( father , mother , fitness )
2: best _ fitness = float . MIN _ VALUE ;
3: best _ child = np . zeros ( father . size );
4: for i = 0 → father . size do
5: current _ child = np . zeros ( father . size );
6: current _ child = np . append ( father [0: i ], mother [ i :]);
7: current _ fitness = fitness ( current _ child );
8: if current _ fitness > best _ fitness then
9: best _ fitness = current _ fitness ;
10: best _ child = current _ child . copy ();
11: end if
12: end for
13: return best _ child
14: end function
3.2 Improved mutation operation
As shown in algorithm 2 is the pseudocode of the improved mutation algorithm. The simple mutation of SGA sets a relatively large mutation rate, and mutates any one gene of the incoming children’s chromosome when the generated random number is smaller than the mutation rate. In this paper, we improve the algorithm by setting a small mutation rate and then selectively mutating each gene of the incoming children’s chromosome. That is, when the generated random number is smaller than the mutation rate, the gene is mutated, and when the traversed gene position is larger than half of the chromosome length, the mutation rate is set to twice the original one (the second half of the gene has relatively less influence on the result). This ensures that the first half of the gene and the second half of the gene have an equal chance of mutation respectively, and can mutate at the same time. When the gene length is 784, the mutation rate of the whole chromosome is 1 − (1 − 0.025) 392 × (1 − 0.05) 392 , which greatly improves the species diversity and at the same time ensures the stability of the species (in the stable situation of the local optimum improves the global search ability), and experimental data show that it can improve the search capability.
Algorithm 2 Mutate child with alter each gene if rand number less than mutate rate.
Input : Child’s gene;
Output : Mutated child’s gene;
1: function MUTATE( child )
2: mutate _ rate = 0.025;
3: for i = 0 → child . size do
4: if i > child . size //2 then
5: mutate _ rate = 0.05;
6: end if
7: if random . random () < mutate _ rate then
8: child [ i ] = ! child [ i ];//child[i] equals 0 or 1
9: end if
10: end for
11: return child
12: end function
4 Numerical experiments and analysis
4.1 test functions.
In order to evaluate the optimization performance of the proposed improved genetic algorithm, 15 representative test functions from AVOA paper of Abdollahzadeh et al. [ 11 ] and Wikipedia [ 22 ] are selected in this paper. Since the proposed improved genetic algorithm is mainly used for the neural network adversarial attack problem, and the neural network has multi-dimensional parameters, the dimensions of the test functions will be tested on 30, 50, and 100, respectively. The details of the formula, dimensions, range, and minimum of the 15 test functions are shown in Tables 1 – 3 , where Table 1 are multi-dimensional test functions with unimodal, Table 2 are multi-dimensional test functions with multi-modal, and Table 3 for fixed-dimensional test functions.
https://doi.org/10.1371/journal.pone.0267970.t001
https://doi.org/10.1371/journal.pone.0267970.t002
https://doi.org/10.1371/journal.pone.0267970.t003
4.2 Experimental environment
The hardware environment of the experiment includes 8G of RAM, i7–4700MQ CPU; the software environment includes Windows 10 system, and the version of Python is 3.8.8. In order to compare the optimization performance of IGA, SGA (Simple Genetic Algorithm), PSO (Particle Swarm Optimization) and GWO (Grey Wolf Optimizer) are selected as the experimental objects for comparison experiments in this paper.
(a) Mutation rate. (b) Population size. (c) Max iteration.
https://doi.org/10.1371/journal.pone.0267970.g002
https://doi.org/10.1371/journal.pone.0267970.t004
4.3 Experimental results and analysis
4.3.1 qualitative result analysis..
(a) Parameter space. (b) Population distribution. (c) Best record. (d) Convergence curve.
https://doi.org/10.1371/journal.pone.0267970.g003
https://doi.org/10.1371/journal.pone.0267970.g004
https://doi.org/10.1371/journal.pone.0267970.g005
https://doi.org/10.1371/journal.pone.0267970.g006
4.3.2 Quantitative result analysis.
In order to make a quantitative comparison with the other three mainstream optimization algorithms, the four optimization algorithms are performed independently for 10 experiments on F1-F11 test functions in dimensions 30, 50, and 100, respectively. The purpose of performing the high-dimensional function test is to test the convergence superiority of IGA on the high-dimensional space for application in the field of neural network adversarial attack. Tables 5 – 7 are the test results of the test functions F1-F11 in 30, 50, and 100 dimensions, respectively. Table 8 shows the results of the four optimization algorithms tested on the test functions F12-F15. The best result, worst result, mean, median, standard deviation, and P-value are compared for 10 experiments. Where P-value is the result of the Wilcoxon rank-sum statistical test and P-value below 5% is significant.
https://doi.org/10.1371/journal.pone.0267970.t005
https://doi.org/10.1371/journal.pone.0267970.t006
https://doi.org/10.1371/journal.pone.0267970.t007
https://doi.org/10.1371/journal.pone.0267970.t008
In Table 5 , IGA achieves significantly superior performance in 9 test functions, PSO is better in F3, and SGA is slightly better in F8. In Tables 6 and 7 , IGA achieves significantly superior performance in 10 test functions, PSO performs better in F3. It can be seen that the performance loss of IGA with increasing dimensionality is not as large as the other three optimization algorithms. In Table 8 , IGA achieves significantly superior performance in 3 test functions, and PSO performs slightly better in F14.
In general, IGA has better iteration efficiency, global search capability, and convergence success rate than the other three optimization algorithms.
5 Application in neural network adversarial attack
5.1 mnst dataset.
The MNST dataset (Mixed National Institute of Standards and Technology database) [ 19 ] is one of the most well-known datasets in the field of machine learning and is used in applications from simple experiments to published paper research. It consists of handwritten digital images from 0–9. The MNIST image data is a single-channel grayscale map of 28 × 28 pixels, with each pixel taking values between 0 and 255, with 60,000 samples in the training set and 10,000 samples in the test set. The general usage of the MNIST dataset is to learn with the training set first and then use the learned model to measure how well the test set can be correctly classified [ 23 ].
5.2 Implementation
As shown in Fig 7(a) , the Deep Convolutional Neural Network (DCNN) pre-trained on the MNST dataset [ 19 ] is used as the experimental object in this paper, and the accuracy of the model is 99.35% with a Loss value of 0.9632. As shown in Fig 7(b) , the model of network adversarial attack is shown. The number of populations of a specific size (set to 100 in this paper) is first generated and then input to the neural network to obtain the confidence of the specified labels. To reduce the computational expense, the input is reduced to a binary image of 28 × 28 and the randomly generated binary image is iterated using the IGA proposed in this paper. Among the 100 individuals, the fathers and mothers with relatively high confidence are selected by roulette selection, and then the children are generated by using the improved crossover link in this paper, and the children from a new population by improving the mutation link until the specified number of iterations. Finally, the individual with the highest confidence is picked from the 100 individuals, which is the binary image with the highest confidence after passing through the neural network.
(a) The structure of DCNN for experiment. (b) The model of network adversarial attack.
https://doi.org/10.1371/journal.pone.0267970.g007
As shown in Fig 8 , the confidence after 99 iterations of DCNN is 99.98% for sample “2”. Sample “6” and sample “4” have the slowest convergence speed, and the confidence of sample “6” is 78.84% after 99 iterations, and the confidence of sample “4” is 78.84% after 99 iterations.
https://doi.org/10.1371/journal.pone.0267970.g008
The statistics of the experimental results are shown in Fig 9 . The binary image of sample “1” generated after 999 iterations has confidence of 99.94% after passing DCNN, which is much higher than the confidence of sample “1” in the MNIST test set in the DCNN control group. In the statistics of the results after initializing the population with the MNIST test set, because the overall confidence of the population initialized with the test set is higher, the increase in confidence during iteration is smaller. The confidence of the sample selected from the MNIST test set is 99.56%, and after 10 iterations the confidence of the sample is 99.80%, and the number “1” becomes vertical; after 89 iterations the confidence is 99.98%, and the number “1” has a tendency to “decompose” gradually.
https://doi.org/10.1371/journal.pone.0267970.g009
As shown in Fig 10 , the reason for this situation is probably that the confidence as a function of the image input is a multi-peak function, and the interval in which the test set images are distributed is not the highest peak of the confidence function. This causes the initial population of the test set to “stray” from some pixels in the images generated by the IGA.
https://doi.org/10.1371/journal.pone.0267970.g010
6 Conclusion
The comparison and simulation experiments show that the improved method proposed in this paper is effective and greatly improves the convergence efficiency, global search capability and convergence success rate. Applying IGA to the field of neural network adversarial attacks can also quickly obtain adversarial samples with high confidence, which is meaningful for the improvement of the robustness and security of neural network models.
In this paper, although the genetic algorithm has been improved to enhance the performance of the genetic algorithm, it is based on the genetic algorithm, so it cannot be completely separated from the general framework of the genetic algorithm, and the problem that the genetic algorithm is relatively slow in a single iteration cannot be solved. We hope to explore a new nature-inspired optimization algorithm in our future work. In addition, the reason why the neural network model has so many adversarial samples, we believe that it is a design flaw in the architecture of the neural network model. In future work, we will also try to explore a completely new way of the infrastructure of neural networks so as to compress the space of adversarial samples.
With the wide application of artificial intelligence and deep learning in the field of computer vision, face recognition has outstanding performance in access control systems and payment systems, which require a fast response to the input face image, but this has instead become a drawback to be hacked. For face recognition systems without in vivo detection, using the method in this paper only requires output labels and confidence information can obtain high confidence images quickly. In summary, neural networks have many pitfalls due to their uninterpretability and still need to be considered carefully for use in important areas.
Supporting information
https://doi.org/10.1371/journal.pone.0267970.s001
- View Article
- Google Scholar
- 2. Eberhart R. and Kennedy J. (1995). A new optimizer using particle swarm theory. In MHS’95. Proceedings of the Sixth International Symposium on Micro Machine and Human Science , pages 39–43. Ieee.
- 3. Kennedy J. and Eberhart R. (1995). Particle swarm optimization. In Proceedings of ICNN’95-international conference on neural networks , volume 4, pages 1942–1948. IEEE.
- 8. Holland J. H. et al. (1975). Adaptation in natural and artificial systems.
- 13. Szegedy C., Zaremba W., Sutskever I., Bruna J., Erhan D., Goodfellow I., et al. (2013). Intriguing properties of neural networks. arXiv preprint arXiv:1312.6199 .
- 15. Kurakin A., Goodfellow I., Bengio S., et al. (2016). Adversarial examples in the physical world.
- 16. Papernot N., McDaniel P., Jha S., Fredrikson M., Celik Z. B., and Swami A. (2016). The limitations of deep learning in adversarial settings. In 2016 IEEE European symposium on security and privacy (EuroS&P) , pages 372–387. IEEE.
- 18. Nguyen A., Yosinski J., and Clune J. (2015). Deep neural networks are easily fooled: High confidence predictions for unrecognizable images. In Proceedings of the IEEE conference on computer vision and pattern recognition , pages 427–436.
- 19. LeCun Y. (1998). The mnist database of handwritten digits. http://yann.lecun.com/exdb/mnist/ .
- 20. Deng J., Dong W., Socher R., Li L.-J., Li K., and Fei-Fei L. (2009). Imagenet: A large-scale hierarchical image database. In 2009 IEEE conference on computer vision and pattern recognition , pages 248–255. Ieee.
- 22. Wikipedia (2021). Test functions for optimization. Website. https://en.wikipedia.org/wiki/Test_functions_for_optimization .
- 23. Yasue S. (2018). Deep Learning from Scratch . “Beijing: Posts and Telecom Press”.
IEEE Account
- Change Username/Password
- Update Address
Purchase Details
- Payment Options
- Order History
- View Purchased Documents
Profile Information
- Communications Preferences
- Profession and Education
- Technical Interests
- US & Canada: +1 800 678 4333
- Worldwide: +1 732 981 0060
- Contact & Support
- About IEEE Xplore
- Accessibility
- Terms of Use
- Nondiscrimination Policy
- Privacy & Opting Out of Cookies
A not-for-profit organization, IEEE is the world's largest technical professional organization dedicated to advancing technology for the benefit of humanity. © Copyright 2024 IEEE - All rights reserved. Use of this web site signifies your agreement to the terms and conditions.
This week: the arXiv Accessibility Forum
Help | Advanced Search
Computer Science > Other Computer Science
Title: genetic algorithm: reviews, implementations, and applications.
Abstract: Nowadays genetic algorithm (GA) is greatly used in engineering pedagogy as an adaptive technique to learn and solve complex problems and issues. It is a meta-heuristic approach that is used to solve hybrid computation challenges. GA utilizes selection, crossover, and mutation operators to effectively manage the searching system strategy. This algorithm is derived from natural selection and genetics concepts. GA is an intelligent use of random search supported with historical data to contribute the search in an area of the improved outcome within a coverage framework. Such algorithms are widely used for maintaining high-quality reactions to optimize issues and problems investigation. These techniques are recognized to be somewhat of a statistical investigation process to search for a suitable solution or prevent an accurate strategy for challenges in optimization or searches. These techniques have been produced from natural selection or genetics principles. For random testing, historical information is provided with intelligent enslavement to continue moving the search out from the area of improved features for processing of the outcomes. It is a category of heuristics of evolutionary history using behavioral science-influenced methods like an annuity, gene, preference, or combination (sometimes refers to as hybridization). This method seemed to be a valuable tool to find solutions for problems optimization. In this paper, the author has explored the GAs, its role in engineering pedagogies, and the emerging areas where it is using, and its implementation.
Subjects: | Other Computer Science (cs.OH) |
Cite as: | [cs.OH] |
(or [cs.OH] for this version) | |
Focus to learn more arXiv-issued DOI via DataCite | |
Journal reference: | International Journal of Engineering Pedagogy (iJEP), 2020 |
Submission history
Access paper:.
- Other Formats

References & Citations
- Google Scholar
- Semantic Scholar
DBLP - CS Bibliography
Bibtex formatted citation.

Bibliographic and Citation Tools
Code, data and media associated with this article, recommenders and search tools.
- Institution
arXivLabs: experimental projects with community collaborators
arXivLabs is a framework that allows collaborators to develop and share new arXiv features directly on our website.
Both individuals and organizations that work with arXivLabs have embraced and accepted our values of openness, community, excellence, and user data privacy. arXiv is committed to these values and only works with partners that adhere to them.
Have an idea for a project that will add value for arXiv's community? Learn more about arXivLabs .

An official website of the United States government
The .gov means it’s official. Federal government websites often end in .gov or .mil. Before sharing sensitive information, make sure you’re on a federal government site.
The site is secure. The https:// ensures that you are connecting to the official website and that any information you provide is encrypted and transmitted securely.
- Publications
- Account settings
Preview improvements coming to the PMC website in October 2024. Learn More or Try it out now .
- Advanced Search
- Journal List
- Springer Nature - PMC COVID-19 Collection

A review on genetic algorithm: past, present, and future
Sourabh katoch.
Computer Science and Engineering Department, National Institute of Technology, Hamirpur, India
Sumit Singh Chauhan
Vijay kumar.
In this paper, the analysis of recent advances in genetic algorithms is discussed. The genetic algorithms of great interest in research community are selected for analysis. This review will help the new and demanding researchers to provide the wider vision of genetic algorithms. The well-known algorithms and their implementation are presented with their pros and cons. The genetic operators and their usages are discussed with the aim of facilitating new researchers. The different research domains involved in genetic algorithms are covered. The future research directions in the area of genetic operators, fitness function and hybrid algorithms are discussed. This structured review will be helpful for research and graduate teaching.
Introduction
In the recent years, metaheuristic algorithms are used to solve real-life complex problems arising from different fields such as economics, engineering, politics, management, and engineering [ 113 ]. Intensification and diversification are the key elements of metaheuristic algorithm. The proper balance between these elements are required to solve the real-life problem in an effective manner. Most of metaheuristic algorithms are inspired from biological evolution process, swarm behavior, and physics’ law [ 17 ]. These algorithms are broadly classified into two categories namely single solution and population based metaheuristic algorithm (Fig. 1 ). Single-solution based metaheuristic algorithms utilize single candidate solution and improve this solution by using local search. However, the solution obtained from single-solution based metaheuristics may stuck in local optima [ 112 ]. The well-known single-solution based metaheuristics are simulated annealing, tabu search (TS), microcanonical annealing (MA), and guided local search (GLS). Population-based metaheuristics utilizes multiple candidate solutions during the search process. These metaheuristics maintain the diversity in population and avoid the solutions are being stuck in local optima. Some of well-known population-based metaheuristic algorithms are genetic algorithm (GA) [ 135 ], particle swarm optimization (PSO) [ 101 ], ant colony optimization (ACO) [ 47 ], spotted hyena optimizer (SHO) [ 41 ], emperor penguin optimizer (EPO) [ 42 ], and seagull optimization (SOA) [ 43 ].

Classification of metaheuristic Algorithms
Among the metaheuristic algorithms, Genetic algorithm (GA) is a well-known algorithm, which is inspired from biological evolution process [ 136 ]. GA mimics the Darwinian theory of survival of fittest in nature. GA was proposed by J.H. Holland in 1992. The basic elements of GA are chromosome representation, fitness selection, and biological-inspired operators. Holland also introduced a novel element namely, Inversion that is generally used in implementations of GA [ 77 ]. Typically, the chromosomes take the binary string format. In chromosomes, each locus (specific position on chromosome) has two possible alleles (variant forms of genes) - 0 and 1. Chromosomes are considered as points in the solution space. These are processed using genetic operators by iteratively replacing its population. The fitness function is used to assign a value for all the chromosomes in the population [ 136 ]. The biological-inspired operators are selection, mutation, and crossover. In selection, the chromosomes are selected on the basis of its fitness value for further processing. In crossover operator, a random locus is chosen and it changes the subsequences between chromosomes to create off-springs. In mutation, some bits of the chromosomes will be randomly flipped on the basis of probability [ 77 , 135 , 136 ]. The further development of GA based on operators, representation, and fitness has diminished. Therefore, these elements of GA are focused in this paper.
The main contribution of this paper are as follows:
- The general framework of GA and hybrid GA are elaborated with mathematical formulation.
- The various types of genetic operators are discussed with their pros and cons.
- The variants of GA with their pros and cons are discussed.
- The applicability of GA in multimedia fields is discussed.
The main aim of this paper is two folds. First, it presents the variants of GA and their applicability in various fields. Second, it broadens the area of possible users in various fields. The various types of crossover, mutation, selection, and encoding techniques are discussed. The single-objective, multi-objective, parallel, and hybrid GAs are deliberated with their advantages and disadvantages. The multimedia applications of GAs are elaborated.
The remainder of this paper is organized as follows: Section 2 presents the methodology used to carry out the research. The classical genetic algorithm and genetic operators are discussed in Section 3 . The variants of genetic algorithm with pros and cons are presented in Section 4 . Section 5 describes the applications of genetic algorithm. Section 6 presents the challenges and future research directions. The concluding remarks are drawn in Section 7 .
Research methodology
PRISMA’s guidelines were used to conduct the review of GA [ 138 ]. A detailed search has been done on Google scholar and PubMed for identification of research papers related to GA. The important research works found during the manual search were also added in this paper. During search, some keywords such as “Genetic Algorithm” or “Application of GA” or “operators of GA” or “representation of GA” or “variants of GA” were used. The selection and rejection of explored research papers are based on the principles, which is mentioned in Table Table1 1 .
Selection criterion for shortlisted research papers
Sr. No. | Parameters | Selection criteria | Elimination criteria |
---|---|---|---|
1 | Duration | Research papers published from 2007 to 2020 | Research papers published before 2007 |
2 | Analysis | Research includes various operators and modification in GA | Research includes operators of other metaheuristics |
3 | Comparison | Research focuses on variants of GA | Research focuses on variants of other metaheuristics. GA included in some part of research |
4 | Applications | Research involves on multimedia, operation management and wireless networks | Research involves on engineering design, data mining, software applications, and astronomy applications |
5 | Study | Research includes mathematical foundation and experimental results | Research includes patent, case study, papers having language other than English |
Total 27,64,792 research papers were explored on Google Scholar, PubMed and manual search. The research work related to genetic algorithm for multimedia applications were also included. During the screening of research papers, all the duplicate papers and papers published before 2007 were discarded. 4340 research papers were selected based on 2007 and duplicate entries. Thereafter, 4050 research papers were eliminated based on titles. 220 research papers were eliminated after reading of abstract. 70 research papers were left after third round of screening. 40 more research papers were discarded after full paper reading and facts found in the papers. After the fourth round of screening, final 30 research papers are selected for review.
Based on the relevance and quality of research, 30 papers were selected for evaluation. The relevance of research is decided through some criteria, which is mentioned in Table Table1. 1 . The selected research papers comprise of genetic algorithm for multimedia applications, advancement of their genetic operators, and hybridization of genetic algorithm with other well-established metaheuristic algorithms. The pros and cons of genetic operators are shown in preceding section.
In this section, the basic structure of GA and its genetic operators are discussed with pros and cons.
Classical GA
Genetic algorithm (GA) is an optimization algorithm that is inspired from the natural selection. It is a population based search algorithm, which utilizes the concept of survival of fittest [ 135 ]. The new populations are produced by iterative use of genetic operators on individuals present in the population. The chromosome representation, selection, crossover, mutation, and fitness function computation are the key elements of GA. The procedure of GA is as follows. A population ( Y ) of n chromosomes are initialized randomly. The fitness of each chromosome in Y is computed. Two chromosomes say C1 and C2 are selected from the population Y according to the fitness value. The single-point crossover operator with crossover probability (C p ) is applied on C1 and C2 to produce an offspring say O . Thereafter, uniform mutation operator is applied on produced offspring ( O ) with mutation probability (M p ) to generate O′ . The new offspring O′ is placed in new population. The selection, crossover, and mutation operations will be repeated on current population until the new population is complete. The mathematical analysis of GA is as follows [ 126 ]:
GA dynamically change the search process through the probabilities of crossover and mutation and reached to optimal solution. GA can modify the encoded genes. GA can evaluate multiple individuals and produce multiple optimal solutions. Hence, GA has better global search capability. The offspring produced from crossover of parent chromosomes is probable to abolish the admirable genetic schemas parent chromosomes and crossover formula is defined as [ 126 ]:
where g is the number of generations, and G is the total number of evolutionary generation set by population. It is observed from Eq.( 1 ) that R is dynamically changed and increase with increase in number of evolutionary generation. In initial stage of GA, the similarity between individuals is very low. The value of R should be low to ensure that the new population will not destroy the excellent genetic schema of individuals. At the end of evolution, the similarity between individuals is very high as well as the value of R should be high.
According to Schema theorem, the original schema has to be replaced with modified schema. To maintain the diversity in population, the new schema keep the initial population during the early stage of evolution. At the end of evolution, the appropriate schema will be produced to prevent any distortion of excellent genetic schema [ 65 , 75 ]. Algorithm 1 shows the pseudocode of classical genetic algorithm.
Algorithm 1: Classical Genetic Algorithm (GA)

Genetic operators
GAs used a variety of operators during the search process. These operators are encoding schemes, crossover, mutation, and selection. Figure Figure2 2 depicts the operators used in GAs.

Operators used in GA
Encoding schemes
For most of the computational problems, the encoding scheme (i.e., to convert in particular form) plays an important role. The given information has to be encoded in a particular bit string [ 121 , 183 ]. The encoding schemes are differentiated according to the problem domain. The well-known encoding schemes are binary, octal, hexadecimal, permutation, value-based, and tree.
Binary encoding is the commonly used encoding scheme. Each gene or chromosome is represented as a string of 1 or 0 [ 187 ]. In binary encoding, each bit represents the characteristics of the solution. It provides faster implementation of crossover and mutation operators. However, it requires extra effort to convert into binary form and accuracy of algorithm depends upon the binary conversion. The bit stream is changed according the problem. Binary encoding scheme is not appropriate for some engineering design problems due to epistasis and natural representation.
In octal encoding scheme, the gene or chromosome is represented in the form of octal numbers (0–7). In hexadecimal encoding scheme, the gene or chromosome is represented in the form of hexadecimal numbers (0–9, A-F) [ 111 , 125 , 187 ]. The permutation encoding scheme is generally used in ordering problems. In this encoding scheme, the gene or chromosome is represented by the string of numbers that represents the position in a sequence. In value encoding scheme, the gene or chromosome is represented using string of some values. These values can be real, integer number, or character [ 57 ]. This encoding scheme can be helpful in solving the problems in which more complicated values are used. As binary encoding may fail in such problems. It is mainly used in neural networks for finding the optimal weights.
In tree encoding, the gene or chromosome is represented by a tree of functions or commands. These functions and commands can be related to any programming language. This is very much similar to the representation of repression in tree format [ 88 ]. This type of encoding is generally used in evolving programs or expressions. Table Table2 2 shows the comparison of different encoding schemes of GA.
Comparison of different encoding schemes
Encoding Scheme | Pros | Cons | Application |
---|---|---|---|
Binary | Easy to implement Faster Execution | No support for inversion operator | Problems that support binary encoding |
Octal | Easy to implement | No support for inversion operator | Limited use |
Hexadecimal | Easy to implement | No support for inversion operator | Limited use |
Permutation | Support inversion operator | No support for binary operators | Task ordering Problem |
Value | No need of value conversion | Requires specific crossover and mutation | Neural Network Problems |
Tree | Operator can easily applied | Difficult to design tree for some problems | Evolving Programs |
Selection techniques
Selection is an important step in genetic algorithms that determines whether the particular string will participate in the reproduction process or not. The selection step is sometimes also known as the reproduction operator [ 57 , 88 ]. The convergence rate of GA depends upon the selection pressure. The well-known selection techniques are roulette wheel, rank, tournament, boltzmann, and stochastic universal sampling.
Roulette wheel selection maps all the possible strings onto a wheel with a portion of the wheel allocated to them according to their fitness value. This wheel is then rotated randomly to select specific solutions that will participate in formation of the next generation [ 88 ]. However, it suffers from many problems such as errors introduced by its stochastic nature. De Jong and Brindle modified the roulette wheel selection method to remove errors by introducing the concept of determinism in selection procedure. Rank selection is the modified form of Roulette wheel selection. It utilizes the ranks instead of fitness value. Ranks are given to them according to their fitness value so that each individual gets a chance of getting selected according to their ranks. Rank selection method reduces the chances of prematurely converging the solution to a local minima [ 88 ].
Tournament selection technique was first proposed by Brindle in 1983. The individuals are selected according to their fitness values from a stochastic roulette wheel in pairs. After selection, the individuals with higher fitness value are added to the pool of next generation [ 88 ]. In this method of selection, each individual is compared with all n-1 other individuals if it reaches the final population of solutions [ 88 ]. Stochastic universal sampling (SUS) is an extension to the existing roulette wheel selection method. It uses a random starting point in the list of individuals from a generation and selects the new individual at evenly spaced intervals [ 3 ]. It gives equal chance to all the individuals in getting selected for participating in crossover for the next generation. Although in case of Travelling Salesman Problem, SUS performs well but as the problem size increases, the traditional Roulette wheel selection performs relatively well [ 180 ].
Boltzmann selection is based on entropy and sampling methods, which are used in Monte Carlo Simulation. It helps in solving the problem of premature convergence [ 118 ]. The probability is very high for selecting the best string, while it executes in very less time. However, there is a possibility of information loss. It can be managed through elitism [ 175 ]. Elitism selection was proposed by K. D. Jong (1975) for improving the performance of Roulette wheel selection. It ensures the elitist individual in a generation is always propagated to the next generation. If the individual having the highest fitness value is not present in the next generation after normal selection procedure, then the elitist one is also included in the next generation automatically [ 88 ]. The comparison of above-mentioned selection techniques are depicted in Table Table3 3 .
Comparison of different selection techniques
Selection Techniques | Pros | Cons |
---|---|---|
Roulette wheel | Easy to implement Simple Free from Bias | Risk of Premature convergence Depends upon variance present in the fitness function |
Rank | Preserve diversity Free from Bias | Slow convergence Sorting required Computationally Expensive |
Tournament | Preserve diversity Parallel Implementation No sorting required | Loss of diversity when the tournament size is large |
Boltzmann | Global optimum achieved | Computationally Expensive |
Stochastic Universal Sampling | Fast Method Free from Bias | Premature convergence |
Elitism | Preserve best Individual in population | Best individual can be lost due to crossover and mutation operators |
Crossover operators
Crossover operators are used to generate the offspring by combining the genetic information of two or more parents. The well-known crossover operators are single-point, two-point, k-point, uniform, partially matched, order, precedence preserving crossover, shuffle, reduced surrogate and cycle.
In a single point crossover, a random crossover point is selected. The genetic information of two parents which is beyond that point will be swapped with each other [ 190 ]. Figure Figure3 3 shows the genetic information after swapping. It replaced the tail array bits of both the parents to get the new offspring.

Swapping genetic information after a crossover point
In a two point and k-point crossover, two or more random crossover points are selected and the genetic information of parents will be swapped as per the segments that have been created [ 190 ]. Figure Figure4 4 shows the swapping of genetic information between crossover points. The middle segment of the parents is replaced to generate the new offspring.

Swapping genetic information between crossover points
In a uniform crossover, parent cannot be decomposed into segments. The parent can be treated as each gene separately. We randomly decide whether we need to swap the gene with the same location of another chromosome [ 190 ]. Figure Figure5 5 depicts the swapping of individuals under uniform crossover operation.

Swapping individual genes
Partially matched crossover (PMX) is the most frequently used crossover operator. It is an operator that performs better than most of the other crossover operators. The partially matched (mapped) crossover was proposed by D. Goldberg and R. Lingle [ 66 ]. Two parents are choose for mating. One parent donates some part of genetic material and the corresponding part of other parent participates in the child. Once this process is completed, the left out alleles are copied from the second parent [ 83 ]. Figure Figure6 6 depicts the example of PMX.

Partially matched crossover (PMX) [ 117 ]
Order crossover (OX) was proposed by Davis in 1985. OX copies one (or more) parts of parent to the offspring from the selected cut-points and fills the remaining space with values other than the ones included in the copied section. The variants of OX are proposed by different researchers for different type of problems. OX is useful for ordering problems [ 166 ]. However, it is found that OX is less efficient in case of Travelling Salesman Problem [ 140 ]. Precedence preserving crossover (PPX) preserves the ordering of individual solutions as present in the parent of offspring before the application of crossover. The offspring is initialized to a string of random 1’s and 0’s that decides whether the individuals from both parents are to be selected or not. In [ 169 ], authors proposed a modified version of PPX for multi-objective scheduling problems.
Shuffle crossover was proposed by Eshelman et al. [ 20 ] to reduce the bias introduced by other crossover techniques. It shuffles the values of an individual solution before the crossover and unshuffles them after crossover operation is performed so that the crossover point does not introduce any bias in crossover. However, the utilization of this crossover is very limited in the recent years. Reduced surrogate crossover (RCX) reduces the unnecessary crossovers if the parents have the same gene sequence for solution representations [ 20 , 139 ]. RCX is based on the assumption that GA produces better individuals if the parents are sufficiently diverse in their genetic composition. However, RCX cannot produce better individuals for those parents that have same composition. Cycle crossover was proposed by Oliver [ 140 ]. It attempts to generate an offspring using parents where each element occupies the position by referring to the position of their parents [ 140 ]. In the first cycle, it takes some elements from the first parent. In the second cycle, it takes the remaining elements from the second parent as shown in Fig. 7 .

Cycle Crossover (CX) [ 140 ]
Table Table4 4 shows the comparison of crossover techniques. It is observed from Table Table4 4 that single and k-point crossover techniques are easy to implement. Uniform crossover is suitable for large subsets. Order and cycle crossovers provide better exploration than the other crossover techniques. Partially matched crossover provides better exploration. The performance of partially matched crossover is better than the other crossover techniques. Reduced surrogate and cycle crossovers suffer from premature convergence.
Comparison of different crossover techniques
Technique | Pros | Cons |
---|---|---|
Single point | Easy to implement Simple | Less diverse solutions |
Two and K-point | Easy to implement | Less diverse solutions Applicable on small subsets |
Reduced Surrogate | Better performance over small optimization problems | Premature convergence |
Uniform | Unbiased Exploration Applicable on large subsets Better recombination potential | Less diverse solutions |
Precedence Preservative (PPX) | Better offspring generation | Redundancy Problem |
Order Crossover (OX) | Better Exploration | Loss of information from previous individual |
Cycle Crossover | Unbiased Exploration | Premature convergence |
Partially Mapped (PMX) | Better Convergence rate Superior than the other crossovers | NA |
Mutation operators
Mutation is an operator that maintains the genetic diversity from one population to the next population. The well-known mutation operators are displacement, simple inversion, and scramble mutation. Displacement mutation (DM) operator displaces a substring of a given individual solution within itself. The place is randomly chosen from the given substring for displacement such that the resulting solution is valid as well as a random displacement mutation. There are variants of DM are exchange mutation and insertion mutation. In Exchange mutation and insertion mutation operators, a part of an individual solution is either exchanged with another part or inserted in another location, respectively [ 88 ].
The simple inversion mutation operator (SIM) reverses the substring between any two specified locations in an individual solution. SIM is an inversion operator that reverses the randomly selected string and places it at a random location [ 88 ]. The scramble mutation (SM) operator places the elements in a specified range of the individual solution in a random order and checks whether the fitness value of the recently generated solution is improved or not [ 88 ]. Table Table5 5 shows the comparison of different mutation techniques.
Comparison of different mutation operators
Operator | Pros | Cons |
---|---|---|
Displacement Mutation | Easy to implement Applicable on small problem instances | Risk of Premature convergence |
Simple-Inversion Mutation | Easy to implement | Premature convergence |
Scramble Mutation | Affects large number of genes Applicable on large problem instances | Disturbance in the population Deterioration of solution quality in some problems |
Table Table6 6 shows the best combination of encoding scheme, mutation, and crossover techniques. It is observed from Table Table6 6 that uniform and single-point crossovers can be used with most of encoding and mutation operators. Partially matched crossover is used with inversion mutation and permutation encoding scheme provides the optimal solution.
Best combination of various operators under optimal Environment
Encoding Scheme | Mutation | Crossover |
---|---|---|
Binary Encoding | Inversion | Uniform, Arithmetic, 1-Point, N-Point |
Permutation | Inversion | Partially Matched Crossover, Cycle Crossover, Order Crossover |
Value | Displacement | Uniform, Arithmetic, 1-Point, N-Point |
Tree | Scramble | Uniform, 1-Point |
Variants of GA
Various variants of GA’s have been proposed by researchers. The variants of GA are broadly classified into five main categories namely, real and binary coded, multiobjective, parallel, chaotic, and hybrid GAs. The pros and cons of these algorithms with their application has been discussed in the preceding subsections.
Real and binary coded GAs
Based on the representation of chromosomes, GAs are categorized in two classes, namely binary and real coded GAs.
Binary coded GAs
The binary representation was used to encode GA and known as binary GA. The genetic operators were also modified to carry out the search process. Payne and Glen [ 153 ] developed a binary GA to identify the similarity among molecules. They used binary representation for position of molecule and their conformations. However, this method has high computational complexity. Longyan et al. [ 203 ] investigated three different method for wind farm design using binary GA (BGA). Their method produced better fitness value and farm efficiency. Shukla et al. [ 185 ] utilized BGA for feature subset selection. They used mutual information maximization concept for selecting the significant features. BGAs suffer from Hamming cliffs, uneven schema, and difficulty in achieving precision [ 116 , 199 ].
Real-coded GAs
Real-coded GAs (RGAs) have been widely used in various real-life applications. The representation of chromosomes is closely associated with real-life problems. The main advantages of RGAs are robust, efficient, and accurate. However, RGAs suffer from premature convergence. Researchers are working on RGAs to improve their performance. Most of RGAs are developed by modifying the crossover, mutation and selection operators.
The searching capability of crossover operators are not satisfactory for continuous search space. The developments in crossover operators have been done to enhance their performance in real environment. Wright [ 210 ] presented a heuristics crossover that was applied on parents to produce off-spring. Michalewicz [ 135 ] proposed arithmetical crossover operators for RGAs. Deb and Agrawal [ 34 ] developed a real-coded crossover operator, which is based on characteristics of single-point crossover in BGA. The developed crossover operator named as simulated binary crossover (SBX). SBX is able to overcome the Hamming cliff, precision, and fixed mapping problem. The performance of SBX is not satisfactory in two-variable blocked function. Eshelman et al. [ 53 ] utilized the schemata concept to design the blend crossover for RGAs. The unimodal normal distribution crossover operator (UNDX) was developed by Ono et al. [ 144 ]. They used ellipsoidal probability distribution to generate the offspring. Kita et al. [ 106 ] presented a multi-parent UNDX (MP-UNDX), which is the extension of [ 144 ]. However, the performance of RGA with MP-UNDX is much similar to UNDX. Deep and Thakur [ 39 ] presented a Laplace crossover for RGAs, which is based on Laplacian distribution. Chuang et al. [ 27 ] developed a direction based crossover to further explore the all possible search directions. However, the search directions are limited. The heuristic normal distribution crossover operator was developed by Wang et al. [ 207 ]. It generates the cross-generated offspring for better search operation. However, the better individuals are not considered in this approach. Subbaraj et al. [ 192 ] proposed Taguchi self-adaptive RCGA. They used Taguchi method and simulated binary crossover to exploit the capable offspring.
Mutation operators generate diversity in the population. The two main challenges have to tackle during the application of mutation. First, the probability of mutation operator that was applied on population. Second, the outlier produced in chromosome after mutation process. Michalewicz [ 135 ] presented uniform and non-uniform mutation operators for RGAs. Michalewicz and Schoenauer [ 136 ] developed a special case of uniform mutation. They developed boundary mutation. Deep and Thakur [ 38 ] presented a novel mutation operator based on power law and named as power mutation. Das and Pratihar [ 30 ] presented direction-based exponential mutation operator. They used direction information of variables. Tang and Tseng [ 196 ] presented a novel mutation operator for enhancing the performance of RCGA. Their approach was fast and reliable. However, it stuck in local optima for some applications. Deb et al. [ 35 ] developed polynomial mutation that was used in RCGA. It provides better exploration. However, the convergence speed is slow and stuck in local optima. Lucasius et al. [ 129 ] proposed a real-coded genetic algorithm (RCGA). It is simple and easy to implement. However, it suffers from local optima problem. Wang et al. [ 205 ] developed multi-offspring GA and investigated their performance over single point crossover. Wang et al. [ 206 ] stated the theoretical basis of multi-offspring GA. The performance of this method is better than non-multi-offspring GA. Pattanaik et al. [ 152 ] presented an improvement in the RCGA. Their method has better convergence speed and quality of solution. Wang et al. [ 208 ] proposed multi-offspring RCGA with direction based crossover for solving constrained problems.
Table Table7 7 shows the mathematical formulation of genetic operators in RGAs.
Mathematical formulation of genetic operators in RGAs
Ref. | Operator | Mathematical Formulation |
---|---|---|
[ ] | Simulated Binary crossover |
Here, two off-springs ( and ) are generated. and are individuals. is a variable whose value lies in the interval of [0, ∞) |
[ ] | Blend crossover | Offspring is generated from parents and from interval [ − (( − ) ), + (( − ) )]where = min( , ) and = max( , ). is a variable whose value lies in the interval of [0, 1] |
[ ] | Arithmetic crossover Geometric crossover | Arithmetic crossover
Geometric crossover
|
[ ] | Unimodal normal distribution crossover operator |
where , = 1, …, − 1 are orthogonal bases that perpendicular to . is the midpoint and is difference vector. is a random vale taken from normal distribution and are 1 random values follows a normal distribution. is the length from parent 3 to perpendicular line. |
[ ] | Laplace crossover |
Here,
Where and are variables. The default values of and are 0 and 1, respectively. is random variable. |
Multiobjective GAs
Multiobjective GA (MOGA) is the modified version of simple GA. MOGA differ from GA in terms of fitness function assignment. The remaining steps are similar to GA. The main motive of multiobjective GA is to generate the optimal Pareto Front in the objective space in such a way that no further enhancement in any fitness function without disturbing the other fitness functions [ 123 ]. Convergence, diversity, and coverage are main goal of multiobjective GAs. The multiobjective GAs are broadly categorized into two categories namely, Pareto-based, and decomposition-based multiobjective GAs [ 52 ]. These techniques are discussed in the preceding subsections.
Pareto-based multi-objective GA
The concept of Pareto dominance was introduced in multiobjective GAs. Fonseca and Fleming [ 56 ] developed first multiobjective GA (MOGA). The niche and decision maker concepts were proposed to tackle the multimodal problems. However, MOGA suffers from parameter tuning problem and degree of selection pressure. Horn et al. [ 80 ] proposed a niched Pareto genetic algorithm (NPGA) that utilized the concept of tournament selection and Pareto dominance. Srinivas and Deb [ 191 ] developed a non-dominated sorting genetic algorithm (NSGA). However, it suffers from lack of elitism, need of sharing parameter, and high computation complexity. To alleviate these problems, Deb et al. [ 36 ] developed a fast elitist non-dominated sorting genetic algorithm (NSGA-II). The performance of NSGA-II may be deteriorated for many objective problems. NSGA-II was unable to maintain the diversity in Pareto-front. To alleviate this problem, Luo et al. [ 130 ] introduced a dynamic crowding distance in NSGA-II. Coello and Pulido [ 28 ] developed a multiobjective micro GA. They used an archive for storing the non-dominated solutions. The performance of Pareto-based approaches may be deteriorated in many objective problems [ 52 ].
Decomposition-based multiobjective GA
Decomposition-based MOGAs decompose the given problem into multiple subproblems. These subproblems are solved simultaneously and exchange the solutions among neighboring subproblems [ 52 ]. Ishibuchi and Murata [ 84 ] developed a multiobjective genetic local search (MOGLS). In MOGLS, the random weights were used to select the parents and local search for their offspring. They used generation replacement and roulette wheel selection method. Jaszkiewicz [ 86 ] modified the MOGLS by utilizing different selection mechanisms for parents. Murata and Gen [ 141 ] proposed a cellular genetic algorithm for multiobjective optimization (C-MOGA) that was an extension of MOGA. They added cellular structure in MOGA. In C-MOGA, the selection operator was performed on the neighboring of each cell. C-MOGA was further extended by introducing an immigration procedure and known as CI-MOGA. Alves and Almeida [ 11 ] developed a multiobjective Tchebycheffs-based genetic algorithm (MOTGA) that ensures convergence and diversity. Tchebycheff scalar function was used to generate non-dominated solution set. Patel et al. [ 151 ] proposed a decomposition based MOGA (D-MOGA). They integrated opposition based learning in D-MOGA for weight vector generation. D-MOGA is able to maintain the balance between diversity of solutions and exploration of search space.
Parallel GAs
The motivation behind the parallel GAs is to improve the computational time and quality of solutions through distributed individuals. Parallel GAs are categorized into three broad categories such as master-slave parallel GAs, fine grained parallel GAs, and multi-population coarse grained parallel Gas [ 70 ]. In master-slave parallel GA, the computation of fitness functions is distributed over the several processors. In fine grained GA, parallel computers are used to solve the real-life problems. The genetic operators are bounded to their neighborhood. However, the interaction is allowed among the individuals. In coarse grained GA, the exchange of individuals among sub-populations is performed. The control parameters are also transferred during migration. The main challenges in parallel GAs are to maximize memory bandwidth and arrange threads for utilizing the power of GPUs [ 23 ]. Table Table8 8 shows the comparative analysis of parallel GAs in terms of hardware and software. The well-known parallel GAs are studied in the preceding subsections.
Analysis of parallel GAs in terms of hardware and software
Ref. | Hardware | No. of processors | Language used | API | Application |
---|---|---|---|---|---|
[ ] | Cluster | 130 | JAVA | – | Data Mining |
[ ] | Multicore CPU | 8 | JAVA | Path Finding | |
[ ] | Cluster | 30 | Fortran | MPI | Road Traffic |
[ ] | Cluster | 48 | JavaScript | Node.JS | Building Structure |
[ ] | Multicore CPU | 8 | JAVA | java.util.component | Land Planning |
[ ] | Multicore CPU | 3 | – | – | Job Scheduling |
[ ] | Cloud | 300 | – | MPI | Internet of Things |
[ ] | Cluster | 100 | – | MPI | Wireless Network |
[ ] | GPU | 448 | – | CUDA | Scheduling |
[ ] | – | 240 | – | – | Nanoscience |
[ ] | GPU | 512 | – | CUDA | Electronics |
Master slave parallel GA
The large number of processors are utilized in master-slave parallel GA (MS-PGA) as compared to other approaches. The computation of fitness functions may be increased by increasing the number of processors. Hong et al. [ 79 ] used MS-PGA for solving data mining problems. Fuzzy rules are used with parallel GA. The evaluation of fitness function was performed on slave machines. However, it suffers from high computational time. Sahingzo [ 174 ] implemented MS-PGA for UAV path finding problem. The genetic operators were executed on processors. They used multicore CPU with four cores. Selection and fitness evaluation was done on slave machines. MS-PGA was applied on traffic assignment problem in [ 127 ]. They used thirty processors to solve this problem at National University of Singapore. Yang et al. [ 213 ] developed a web-based parallel GA. They implemented the master slave version of NSGA-II in distributed environment. However, the system is complex in nature.
Fine grained parallel GA
In last few decades, researchers are working on migration policies of fine grained parallel GA (FG-PGA). Porta et al. [ 161 ] utilized clock-time for migration frequency, which is independent of generations. They used non-uniform structure and static configuration. The best solution was selected for migration and worst solution was replaced with migrant solution. Kurdi [ 115 ] used adaptive migration frequency. The migration procedure starts until there is no change in the obtained solutions after ten successive generations. The non-uniform and dynamic structure was used. In [ 209 ], local best solutions were synchronized and formed a global best solutions. The global best solutions were transferred to all processors for father execution. The migration frequency depends upon the number of generation. They used uniform structure with fixed configuration. Zhang et al. [ 220 ] used parallel GA to solve the set cover problem of wireless networks. They used divide-and-conquer strategy to decompose the population into sub-populations. Thereafter, the genetic operators were applied on local solutions and Kuhn-Munkres was used to merge the local solutions.
Coarse grained parallel GA
Pinel et al. [ 158 ] proposed a GraphCell. The population was initialized with random values and one solution was initialized with Min-min heuristic technique. 448 processors were used to implement the proposed approach. However, coarse grained parallel GAs are less used due to complex in nature. The hybrid parallel GAs are widely used in various applications. Shayeghi et al. [ 182 ] proposed a pool-based Birmingham cluster GA. Master node was responsible for managing global population. Slave node selected the solutions from global population and executed it. 240 processors are used for computation. Roberge et al. [ 170 ] used hybrid approach to optimize switching angle of inverters. They used four different strategies for fitness function computation. Nowadays, GPU, cloud, and grid are most popular hardware for parallel GAs [ 198 ].
Chaotic GAs
The main drawback of GAs is premature convergence. The chaotic systems are incorporated into GAs to alleviate this problem. The diversity of chaos genetic algorithm removes premature convergence. Crossover and mutation operators can be replaced with chaotic maps. Tiong et al. [ 197 ] integrated the chaotic maps into GA for further improvement in accuracy. They used six different chaotic maps. The performance of Logistic, Henon and Ikeda chaotic GA performed better than the classical GA. However, these techniques suffer from high computational complexity. Ebrahimzadeh and Jampour [ 48 ] used Lorenz chaotic for genetic operators of GA to eliminate the local optima problem. However, the proposed approach was unable to find relationship between entropy and chaotic map. Javidi and Hosseinpourfard [ 87 ] utilized two chaotic maps namely logistic map and tent map for generating chaotic values instead of random selection of initial population. The proposed chaotic GA performs better than the GA. However, this method suffers from high computational complexity. Fuertes et al. [ 60 ] integrated the entropy into chaotic GA. The control parameters are modified through chaotic maps. They investigated the relationship between entropy and performance optimization.
Chaotic systems have also used in multiobjective and hybrid GAs. Abo-Elnaga and Nasr [ 5 ] integrated chaotic system into modified GA for solving Bi-level programming problems. Chaotic helps the proposed algorithm to alleviate local optima and enhance the convergence. Tahir et al. [ 193 ] presented a binary chaotic GA for feature selection in healthcare. The chaotic maps were used to initialize the population and modified reproduction operators were applied on population. Xu et al. [ 115 ] proposed a chaotic hybrid immune GA for spectrum allocation. The proposed approach utilizes the advantages of both chaotic and immune operator. However, this method suffers from parameter initialization problem.
Genetic Algorithms can be easily hybridized with other optimization methods for improving their performance such as image denoising methods, chemical reaction optimization, and many more. The main advantages of hybridized GA with other methods are better solution quality, better efficiency, guarantee of feasible solutions, and optimized control parameters [ 51 ]. It is observed from literature that the sampling capability of GAs is greatly affected from population size. To resolve this problem, local search algorithms such as memetic algorithm, Baldwinian, Lamarckian, and local search have been integrated with GAs. This integration provides proper balance between intensification and diversification. Another problem in GA is parameter setting. Finding appropriate control parameters is a tedious task. The other metaheuristic techniques can be used with GA to resolve this problem. Hybrid GAs have been used to solve the issues mentioned in the preceding subsections [ 29 , 137 , 186 ].
Enhance search capability
GAs have been integrated with local search algorithms to reduce the genetic drift. The explicit refinement operator was introduced in local search for producing better solutions. El-Mihoub et al. [ 54 ] established the effect of probability of local search on the population size of GA. Espinoza et al. [ 50 ] investigated the effect of local search for reducing the population size of GA. Different search algorithms have been integrated with GAs for solving real-life applications.
Generate feasible solutions
In complex and high-dimensional problems, the genetic operators of GA generate infeasible solutions. PMX crossover generates the infeasible solutions for order-based problems. The distance preserving crossover operator was developed to generate feasible solutions for travelling salesman problem [ 58 ]. The gene pooling operator instead of crossover was used to generate feasible solution for data clustering [ 19 ]. Konak and Smith [ 108 ] integrated a cut-saturation algorithm with GA for designing the communication networks. They used uniform crossover to produce feasible solutions.
Replacement of genetic operators
There is a possibility to replace the genetic operators which are mentioned in Section 3.2 with other search techniques. Leng [ 122 ] developed a guided GA that utilizes the penalties from guided local search. These penalties were used in fitness function to improve the performance of GA. Headar and Fukushima [ 74 ] used simplex crossover instead of standard crossover. The standard mutation operator was replaced with simulated annealing in [ 195 ]. The basic concepts of quantum computing are used to improve the performance of GAs. The heuristic crossover and hill-climbing operators can be integrated into GA for solving three-matching problem.
Optimize control parameters
The control parameters of GA play a crucial role in maintaining the balance between intensification and diversification. Fuzzy logic has an ability to estimate the appropriate control parameters of GA [ 167 ]. Beside this, GA can be used to optimize the control parameters of other techniques. GAs have been used to optimize the learning rate, weights, and topology of neutral networks [ 21 ]. GAs can be used to estimate the optimal value of fuzzy membership in controller. It was also used to optimize the control parameters of ACO, PSO, and other metaheuristic techniques [ 156 ]. The comparative analysis of well-known GAs are mentioned in Table Table9 9 .
Comparative study of GA’s variants in terms of pros and cons
Reference | Year | Pros | Cons | Application |
---|---|---|---|---|
Real-Coded GAs | ||||
[ ] | 1989 | No encoding required Simple Fast Convergence | Trapped in Local optima | Chemo-metrics |
[ ] | 1997 | Better Performance | Trapped in Local optima | Optimization Problems |
[ ] | 2007 | Fast convergence | Less success rate | Optimization Problems |
[ ] | 2011 | Better convergence speed Less computational cost | Premature convergence | Economic dispatch |
[ ] | 2013 | Better search capability Fast convergence | Premature convergence for some applications | Optimization Problems |
[ ] | 2014 | Better Exploration | Stuck in Local optima Slow convergence speed | Optimization Problems |
[ ] | 2016 | Better offspring generation | Limited search directions | Optimization Problems |
[ ] | 2016 | Fast convergence Better population diversity | Expensive computational cost | Traveling Salesman Problem |
[ ] | 2018 | Guarantee cross-generated offspring | Better individuals not considered | Optimization Problems |
[ ] | 2018 | Fast convergence Better solution quality | Premature convergence | Economic dispatch |
[ ] | 2019 | Better convergence Not stuck in local optima | Computationally expensive | Optimization Problems |
[ ] | 2020 | Better performance Suitable for constrained search space | Tuning of crossover operator required | Optimization Problems |
[ ] | 2012 | Superior performance over standard GA | More computational time required | Optimization Problems |
[ ] | 2013 | Improved performance due to chaotic process Better convergence | Unable to classify the chaotic map and its relationship with entropy | Optimization Problems |
[ ] | 2015 | Enhance diversity of population Avoid local optima | More computational time required | Optimization Problems |
[ ] | 2019 | Able to establish relationship between chaotic map and entropy Better solution quality | Influence of multifractals in initial population for some applications | Optimization Problems |
[ ] | 2020 | Fast convergence Better performance | More computational time required | Bi-level Programming |
[ ] | 2020 | Better classification accuracy | – | Healthcare |
[ ] | 2020 | Better performance | Parameter initialization | Spectrum Allocation |
Parallel GAs | ||||
[ ] | 2018 | Fast Execution Better convergence | Unable to utilize the full power of machine | Text Feature Clustering |
[ ] | 2018 | Better optimization accuracy Low computational time | Require optimize instruction execution | Optimization Problems |
[ ] | 2019 | Fast convergence | Inferior solution quality | Community Detection |
[ ] | 2020 | Easy to implement Faster execution | More improvement in GPU utilization required | Logistics Management |
[ ] | 2020 | Optimize memory access Optimize instruction execution | Need of GPU accelerated libraries | Railway Scheduling |
[ ] | 2020 | Better performance Highest Speedup | Low utilization of GPU cluster | Transportation System |
Binary Coded GAs | ||||
[ ] | 1993 | Fast convergence | More computational time required | Molecular Recognition |
[ ] | 2014 | Superior Performance Flexible | Tuning of control parameters | Wind Farm Design |
[ ] | 2019 | Fast Efficient searching capability | Influence from setting of control parameters | Feature Selection |
Hybrid GAs | ||||
[ ] | 2020 | Faster convergence rate Better distribution | Parameter tuning is required for better result | Routing |
[ ] | 2020 | Better Line of Code decline rate Improve performance of GA | Slow convergence | Program Analysis |
[ ] | 2020 | Better accuracy score | Stuck in local optima | Accidental Death Record |
[ ] | 2020 | Robust Efficient Accurate | Premature convergence | Stock Market Prediction |
[ ] | 2020 | Improve local search capability | Premature convergence | Job Scheduling |
[ ] | 2020 | Improve local search capability Better solution quality | Slow convergence | Travelling Salesman Problem |
[ ] | 2020 | Better solution quality | Premature convergence | Feature Selection |
[ ] | 2018 | Better search space | Unable to capture quantitative information | Agriculture |
[ ] | 2018 | Better classification accuracy | Slow convergence | Agriculture |
[ ] | 2018 | Superior performance | High computational time | Function Approximation |
Applications
Genetic Algorithms have been applied in various NP-hard problems with high accuracy rates. There are a few application areas in which GAs have been successfully applied.
Operation management
GA is an efficient metaheuristic for solving operation management (OM) problems such as facility layout problem (FLP), supply network design, scheduling, forecasting, and inventory control.
Facility layout
Datta et al. [ 32 ] utilized GA for solving single row facility layout problem (SRFLP). For SRFLP, the modified crossover and mutation operators of GA produce valid solutions. They applied GA to large sized problems that consists of 60–80 instances. However, it suffers from parameter dependency problem. Sadrzadeh [ 173 ] proposed GA for multi-line FLP have multi products. The facilities were clustered using mutation and heuristic operators. The total cost obtained from the proposed GA was decreased by 7.2% as compared to the other algorithms. Wu et al. [ 211 ] implemented hierarchical GA to find out the layout of cellular manufacturing system. However, the performance of GA is greatly affected from the genetic operators. Aiello et al. [ 7 ] proposed MOGA for FLP. They used MOGA on the layout of twenty different departments. Palomo-Romero et al. [ 148 ] proposed an island model GA to solve the FLP. The proposed technique maintains the population diversity and generates better solutions than the existing techniques. However, this technique suffers from improper migration strategy that can be utilized for improving the population. GA and its variants has been successfully applied on FLP [ 103 , 119 , 133 , 201 ].
GA shows the superior performance for solving the scheduling problems such as job-shop scheduling (JSS), integrated process planning and scheduling (IPPS), etc. [ 119 ]. To improve the performance in the above-mentioned areas of scheduling, researchers developed various genetic representation [ 12 , 159 , 215 ], genetic operators, and hybridized GA with other methods [ 2 , 67 , 147 , 219 ].
Inventory control
Besides the scheduling, inventory control plays an important role in OM. Backordering and lost sales are two main approaches for inventory control [ 119 ]. Hiassat et al. [ 76 ] utilized the location-inventory model to find out the number and location of warehouses. Various design constraints have been added in the objective functions of GA and its variants for solving inventory control problem [].
Forecasting and network design
Forecasting is an important component for OM. Researchers are working on forecasting of financial trading, logistics demand, and tourist arrivals. GA has been hybridized with support vector regression, fuzzy set, and neural network (NN) to improve their forecasting capability [ 22 , 78 , 89 , 178 , 214 ]. Supply network design greatly affect the operations planning and scheduling. Most of the research articles are focused on capacity constraints of facilities [ 45 , 184 ]. Multi-product multi-period problems increases the complexity of supply networks. To resolve the above-mentioned problem, GA has been hybridized with other techniques [ 6 , 45 , 55 , 188 , 189 ]. Multi-objective GAs are also used to optimize the cost, profit, carbon emissions, etc. [ 184 , 189 ].
GAs have been applied in various fields of multimedia. Some of well-known multimedia fields are encryption, image processing, video processing, medical imaging, and gaming.
Information security
Due to development in multimedia applications, images, videos and audios are transferred from one place to another over Internet. It has been found in literature that the images are more error prone during the transmission. Therefore, image protection techniques such as encryption, watermarking and cryptography are required. The classical image encryption techniques require the input parameters for encryption. The wrong selection of input parameters will generate inadequate encryption results. GA and its variants have been used to select the appropriate control parameters. Kaur and Kumar [ 96 ] developed a multi-objective genetic algorithm to optimize the control parameters of chaotic map. The secret key was generated using beta chaotic map. The generated key was use to encrypt the image. Parallel GAs were also used to encrypt the image [ 97 ].
Image processing
The main image processing tasks are preprocessing, segmentation, object detection, denoising, and recognition. Image segmentation is an important step to solve the image processing problems. Decomposing/partitioning an image requires high computational time. To resolve this problem, GA is used due to their better search capability [ 26 , 102 ]. Enhancement is a technique to improve the quality and contrast of an image. The better image quality is required to analyze the given image. GAs have been used to enhance natural contrast and magnify image [ 40 , 64 , 99 ]. Some researchers are working on hybridization of rough set with adaptive genetic algorithm to merge the noise and color attributes. GAs have been used to remove the noise from the given image. GA can be hybridized with fuzzy logic to denoise the noisy image. GA based restoration technique can be used to remove haze, fog and smog from the given image [ 8 , 110 , 146 , 200 ]. Object detection and recognition is a challenging issue in real-world problem. Gaussian mixture model provides better performance during detection and recognition process. The control parameters are optimized through GA [ 93 ].
Video processing
Video segmentation has been widely used in pattern recognition, and computer vision. There are some critical issues that are associated with video segmentation. These are distinguishing object from the background and determine accurate boundaries. GA can be used to resolve these issues [ 9 , 105 ]. GAs have been implemented for gesture recognition successfully by Chao el al. [ 81 ] used GA for gesture recognition. They applied GAs and found an accuracy of 95% in robot vision. Kaluri and Reddy [ 91 ] proposed an adaptive genetic algorithm based method along with fuzzy classifiers for sign gesture recognition. They reported an improved recognition rate of 85% as compared to the existing method that provides 79% accuracy. Beside the gesture recognition, face recognition play an important role in criminal identification, unmanned vehicles, surveillance, and robots. GA is able to tackle the occlusion, orientations, expressions, pose, and lighting condition [ 69 , 95 , 109 ].
Medical imaging
Genetic algorithms have been applied in medical imaging such as edge detection in MRI and pulmonary nodules detection in CT scan images [ 100 , 179 ]. In [ 120 ], authors used a template matching technique with GA for detecting nodules in CT images. Kavitha and Chellamuthu [ 179 ] used GA based region growing method for detecting the brain tumor. GAs have been applied on medical prediction problems captured from pathological subjects. Sari and Tuna [ 176 ] used GA used to solve issues arises in biomechanics. It is used to predict pathologies during examination. Ghosh and Bhattachrya [ 62 ] implemented sequential GA with cellular automata for modelling the coronavirus disease 19 (COVID-19) data. GAs can be applied in parallel mode to find rules in biological datasets [ 31 ]. The authors proposed a parallel GA that runs by dividing the process into small sub-generations and evaluating the fitness of each individual solution in parallel. Genetic algorithms are used in medicine and other related fields. Koh et al. [ 61 ] proposed a genetic algorithm based method for evaluation of adverse effects of a given drug.
Precision agriculture
GAs have been applied on various problems that are related to precision agriculture. The main issues are crop yield, weed detection, and improvement in farming equipment. Pachepsky and Acock [ 145 ] implemented GA to analyze the water capacity in soil using remote sensing images. The crop yield can be predicted through the capacity of water present in soil. The weed identification was done through GA in [ 142 ]. They used aerial image for classification of plants. In [ 124 ], color image segmentation was used to discriminate the weed and plant. Peerlink et al. [ 154 ] determined the appropriate rate of fertilizer for various portions of agriculture field. They GA for determining the nitrogen in wheat field. The energy requirements in water irrigation systems can be optimized by viewing it as a multi-objective optimization problem. The amount of irrigation required and thus power requirements change continuously in a SMART farm. Therefore, GA can be applied in irrigation systems to reduce the power requirements [ 33 ].
GAs have been successfully used in games such as gomoku. In [ 202 ], the authors shown that the GA based approach finds the solution having the highest fitness than the normal tree based methods. However, in real-time strategy based games, GA based solutions become less practical to implement [ 82 ]. GAs have been implemented for path planning problems considering the environment constraints as well as avoiding the obstacles to reach the given destination. Burchardt and Salomon [ 18 ] described an implementation for path planning for soccer games. GA can encode the path planning problems via the coordinate points of a two-dimensional playing field, hence resulting in a variable length solution. The fitness function in path planning considers length of path as well as the collision avoiding terms for soccer players.
Wireless networking
Due to adaptive, scalable, and easy implementation of GA, it has been used to solve the various issues of wireless networking. The main issues of wireless networking are routing, quality of service, load balancing, localization, bandwidth allocation and channel assignment [ 128 , 134 ]. GA has been hybridized with other metaheuristics for solving the routing problems. Hybrid GA not only producing the efficient routes among pair of nodes, but also used for load balancing [ 24 , 212 ].
Load balancing
Nowadays, multimedia applications require Quality-of-Service (QoS) demand for delay and bandwidth. Various researchers are working on GAs for QoS based solutions.GA produces optimal solutions for complex networks [ 49 ]. Roy et al. [ 172 ] proposed a multi-objective GA for multicast QoS routing problem. GA was used with ACO and other search algorithms for finding optimal routes with desired QoS metrics. Load balancing is another issue in wireless networks. Scully and Brown [ 177 ] used MicroGAs and MacroGAs to distribute the load among various components of networks. He et al. [ 73 ] implemented GA to determine the balance load in wireless sensor networks. Cheng et al. [ 25 ] utilized distributed GA with multi-population scheme for load balancing. They used load balancing metric as a fitness function in GA.
Localization
The process of determining the location of wireless nodes is called as localization. It plays an important role in disaster management and military services. Yun et al. [ 216 ] used GA with fuzzy logic to find out the weights, which are assigned according to the signal strength. Zhang et al. [ 218 ] hybridized GA with simulated annealing (SA) to determine the position of wireless nodes. SA is used as local search to eliminate the premature convergence.
Bandwidth and channel allocation
The appropriate bandwidth allocation is a complex task. GAs and its variants have been developed to solve the bandwidth allocation problem [ 92 , 94 , 107 ]. GAs were used to investigate the allocation of bandwidth with QoS constraints. The fitness function of GAs may consists of resource utilization, bandwidth distribution, and computation time [ 168 ]. The channel allocation is an important issue in wireless networks. The main objective of channel allocation is to simultaneously optimize the number of channels and reuse of allocated frequency. Friend et al. [ 59 ] used distributed island GA to resolve the channel allocation problem in cognitive radio networks. Zhenhua et al. [ 221 ] implemented a modified immune GA for channel assignment. They used different encoding scheme and immune operators. Pinagapany and Kulkarni [ 157 ] developed a parallel GA to solve both static and dynamic channel allocation problem. They used decimal encoding scheme. Table Table10 10 summarizes the applications of GA and its variants.
Applications of GA
Broad Area | Sub-domain | Target Problems | Variants of GA | Ref. |
---|---|---|---|---|
Operation Management | Facility layout Design | Static facility layout problem Dynamic facility layout problem Flexible bay structure | GA, MOGA, Parallel GA, Hierarchical GA | [ , , , , , , , , ] |
Supply network design | Multi-product, multi-period Multi-product, single-period Single-product, single-period | GA, NSGA-II, GA + PSO, MOGA, GA + Fuzzy | [ , , , , , ] | |
Scheduling | Vehicle routing Resource sharing and scheduling Machine scheduling Airline flight scheduling | GA, GA + BB, GA + ABC, GA + Local search, MOGA, NSGA-II, Hierarchical GA | [A132–138] | |
Forecasting | Financial trading Tourism demand Healthcare demand | GA, Chaotic GA, Adaptive GA, GA + NN | [ , , , , ] | |
Inventory control | Inventory-routing Lot sizing Location-inventory routing | GA, NSGA-II | [ , , ] | |
Multimedia | Information Security | Encryption Watermarking | GA, Parallel GA, NSGA-II, NSGA | [ – , ] |
Image Processing | Segmentation Enhancement Object detection De-noising Recognition | GA, NSGA-II, Parallel GA, Hybrid GA, Adaptive GA, Chaotic GA | [ , , , , , , , , , , ] | |
Video Processing | Video segmentation Gesture recognition Face recognition | GA, NSGA, Adaptive GA, Hybrid GA | [ , , , , , , , , ] | |
Medical Imaging | Tumor diagnosis COVID-19 diagnosis Bioinformatics | GA, Hybrid GA, Parallel GA, Sequential GA | [ , , , , , ] | |
Precision Agriculture | Weed detection Crop management Water irrigation | GA, Hybrid GA, NSGA | [ , , , , , ] | |
Gaming | Google Chrome dinosaur Chess Strategic games | GA, Coevolutionary GA, NSGA | [ , , ] | |
Wireless Networking | Wireless mesh networks Mobile Ad-hoc networks Wireless sensor networks | Routing | GA, Sequential GA, MOGA | [ , , ] |
Quality of Service | MOGA, GA + Fuzzy Logic, NSGA, GA + ACO,NSGA-II | [ , , , , ] | ||
Load balancing | MicroGA, MacroGA, Distributed GA | [ , , ] | ||
Localization | MicroGA, GA + SA, GA + Fuzzy Logic | [ , , ] | ||
Bandwidth allocation | GA, Distributed GA, GA + Local search, GA + Greedy Algorithms, MOGA | [ , , , ] | ||
Channel assignment | MOGA, Parallel GA, Distributed Island GA | [ , , ] |
Challenges and future possibilities
In this section, the main challenges faced during the implementation of GAs are discussed followed by the possible research directions.
Despite the several advantages, there are some challenges that need to be resolved for future advancements and further evolution of genetic algorithms. Some major challenges are given below:
Selection of initial population
Initial population is always considered as an important factor for the performance of genetic algorithms. The size of population also affects the quality of solution [ 160 ]. The researchers argue that if a large population is considered, then the algorithm takes more computation time. However, the small population may lead to poor solution [ 155 ]. Therefore, finding the appropriate population size is always a challenging issue. Harik and Lobo [ 71 ] investigated the population using self-adaption method. They used two approaches such as (1) use of self-adaption prior to execution of algorithm, in which the size of population remains the same and (2) in which the self-adaption used during the algorithm execution where the population size is affected by fitness function.
Premature convergence
Premature convergence is a common issue for GA. It can lead to the loss of alleles that makes it difficult to identify a gene [ 15 ]. Premature convergence states that the result will be suboptimal if the optimization problem coincides too early. To avoid this issue, some researchers suggested that the diversity should be used. The selection pressure should be used to increase the diversity. Selection pressure is a degree which favors the better individuals in the initial population of GA’s. If selection pressure (SP1) is greater than some selection pressure (SP2), then population using SP1 should be larger than the population using SP2. The higher selection pressure can decrease the population diversity that may lead to premature convergence [ 71 ].
Convergence property has to be handled properly so that the algorithm finds global optimal solution instead of local optimal solution (see Fig. Fig.8). 8 ). If the optimal solution lies in the vicinity of an infeasible solution, then the global nature of GA can be combined with local nature of other algorithms such as Tabu search and local search. The global nature of genetic algorithms and local nature of Tabu search provide the proper balance between intensification and diversification.

Local and global optima [ 149 ]
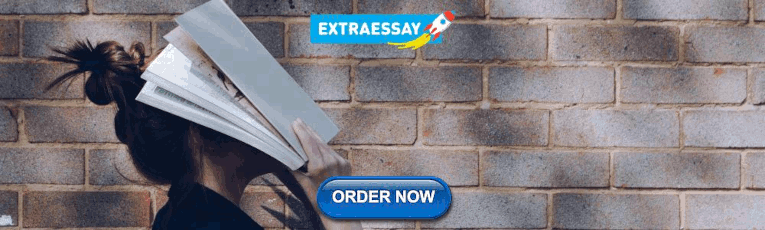
Selection of efficient fitness functions
Fitness function is the driving force, which plays an important role in selecting the fittest individual in every iteration of an algorithm. If the number of iterations are small, then a costly fitness function can be adjusted. The number of iterations increases may increase the computational cost. The selection of fitness function depends upon the computational cost as well as their suitability. In [ 46 ], the authors used Davies-Bouldin index for classification of documents.
Degree of mutation and crossover
Crossover and mutation operators are the integral part of GAs. If the mutation is not considered during evolution, then there will be no new information available for evolution. If crossover is not considered during evolution, then the algorithm can result in local optima. The degree of these operators greatly affect the performance of GAs [ 72 ]. The proper balance between these operators are required to ensure the global optima. The probabilistic nature cannot determine the exact degree for an effective and optimal solution.
Selection of encoding schemes
GAs require a particular encoding scheme for a specific problem. There is no general methodology for deciding whether the particular encoding scheme is suitable for any type of real-life problem. If there are two different problems, then two different encoding schemes are required. Ronald [ 171 ] suggested that the encoding schemes should be designed to overwhelm the redundant forms. The genetic operators should be implemented in a manner that they are not biased towards the redundant forms.
Future research directions
GAs have been applied in different fields by modifying the basic structure of GA. The optimality of a solution obtained from GA can be made better by overcoming the current challenges. Some future possibilities for GA are as follows:
- There should be some way to choose the appropriate degree of crossover and mutation operators. For example Self-Organizing GA adapt the crossover and mutation operators according to the given problem. It can save computation time that make it faster.
- Future work can also be considered for reducing premature convergence problem. Some researchers are working in this direction. However, it is suggested that new methods of crossover and mutation techniques are required to tackle the premature convergence problem.
- Genetic algorithms mimic the natural evolution process. There can be a possible scope for simulating the natural evolution process such as the responses of human immune system and the mutations in viruses.
- In real-life problems, the mapping from genotype to phenotype is complex. In this situation, the problem has no obvious building blocks or building blocks are not adjacent groups of genes. Hence, there is a possibility to develop novel encoding schemes to different problems that does not exhibit same degree of difficulty.
Conclusions
This paper presents the structured and explained view of genetic algorithms. GA and its variants have been discussed with application. Application specific genetic operators are discussed. Some genetic operators are designed for representation. However, they are not applicable to research domains. The role of genetic operators such as crossover, mutation, and selection in alleviating the premature convergence is studied extensively. The applicability of GA and its variants in various research domain has been discussed. Multimedia and wireless network applications were the main attention of this paper. The challenges and issues mentioned in this paper will help the practitioners to carry out their research. There are many advantages of using GAs in other research domains and metaheuristic algorithms.
The intention of this paper is not only provide the source of recent research in GAs, but also provide the information about each component of GA. It will encourage the researchers to understand the fundamentals of GA and use the knowledge in their research problems.
Publisher’s note
Springer Nature remains neutral with regard to jurisdictional claims in published maps and institutional affiliations.
Genetic Algorithms and Artificial Life
Stephanie forrest, melanie mitchell.
Paper #: 93-11-072
Genetic algorithms are computational models of evolution that play a central role in many artificial life models. We review the history and current scope of research on genetic algorithms in artificial life, using illustrative examples in which the genetic algorithms is used to study how learning and evolution interact, and to model ecosystems, immune system, cognitive systems, and social systems. We also outline a number of open questions and future directions for genetic algorithms in artificial life research.
- Sign Up For SFI News

Maintenance work is planned from 22:00 BST on Monday 16th September 2024 to 22:00 BST on Tuesday 17th September 2024.
During this time the performance of our website may be affected - searches may run slowly, some pages may be temporarily unavailable, and you may be unable to access content. If this happens, please try refreshing your web browser or try waiting two to three minutes before trying again.
We apologise for any inconvenience this might cause and thank you for your patience.
Chemical Science
Augmenting genetic algorithms with machine learning for inverse molecular design.
Evolutionary and machine learning methods have been successfully applied to the generation of molecules and materials exhibiting desired properties. The combination of these two paradigms in inverse design tasks can yield powerful methods that explore massive chemical spaces more efficiently, improving the quality of the generated compounds. However, such synergistic approaches are still an incipient area of research and appear underexplored in the literature. This perspective covers different ways of incorporating machine learning approaches into evolutionary learning frameworks, with the overall goal of increasing the optimization efficiency of genetic algorithms. In particular, machine learning surrogate models for faster fitness function evaluation, discriminator models to control population diversity on-the-fly, machine learning based crossover operations, and evolution in latent space are discussed. The further potential of these synergistic approaches in generative tasks is also assessed, outlining promising directions for future developments.
Transparent peer review
To support increased transparency, we offer authors the option to publish the peer review history alongside their article.
View this article’s peer review history
Article information

Download Citation
Permissions.

H. Kneiding and D. Balcells, Chem. Sci. , 2024, Accepted Manuscript , DOI: 10.1039/D4SC02934H
This article is licensed under a Creative Commons Attribution 3.0 Unported Licence . You can use material from this article in other publications without requesting further permissions from the RSC, provided that the correct acknowledgement is given.
Read more about how to correctly acknowledge RSC content .
Social activity
Search articles by author.
This article has not yet been cited.
Advertisements
Thank you for visiting nature.com. You are using a browser version with limited support for CSS. To obtain the best experience, we recommend you use a more up to date browser (or turn off compatibility mode in Internet Explorer). In the meantime, to ensure continued support, we are displaying the site without styles and JavaScript.
- View all journals
- Explore content
- About the journal
- Publish with us
- Sign up for alerts
- Open access
- Published: 11 September 2024
Parameter identification of Johnson–Cook constitutive model based on genetic algorithm and simulation analysis for 304 stainless steel
- Xinyang Jiang 1 ,
- Jinfu Ding 1 ,
- Chengwu Wang 1 , 2 ,
- E Shiju 1 ,
- Ling Hong 3 ,
- Weifeng Yao 4 ,
- Huadong Wang 1 ,
- Chongqiu Zhou 1 &
Scientific Reports volume 14 , Article number: 21221 ( 2024 ) Cite this article
Metrics details
- Engineering
- Materials science
Addressing the significant discrepancy between actual experimental cutting force and its predicted values derived from traditional constitutive model parameter identification methods, a reverse identification research of the Johnson–Cook (J-C) constitutive model for 304 stainless steel was conducted via genetic algorithm. Considering actual cutting zone and the influence of feed motion on the rake (flank) angle, an unequal division shear zone model was established to implement the theoretical calculation for shear zone stress. Through cutting experiments, the spindle speed was negatively correlated with the cutting force at first, and then became positively correlated; The empirical formula (EXP model) for turning force was corrected, revealing that the EXP model was unable to provide optimal predicted values for cutting force. The influence of the J-C constitutive parameter C on the cutting morphology was firstly investigated through simulation analysis, and determined an appropriate value for C , then obtained the precise values for the other four constitutive parameters by genetic algorithm. Moreover, the simulated values of cutting force in JC1 model (obtained from the Split Hopkinson Pressure Bar test) and JCM model (the improved model using genetic algorithm) were obtained by three-dimensional (3-D) simulation via FEM software. The results indicated that, the maximum error between actual experimental cutting force and its simulated values (by JCM model) was 14.8%, with an average error of 6.38%. These results outperformed the JC1 and EXP models, suggesting that the JCM model identified via genetic algorithm was more reliable.
Introduction
Owing to excellent performance, such as high thermal stability, corrosion resistance, and high-temperature resistance, 304 stainless steel (0Cr19Ni9) is widely applied in industries like chemical engineering, aerospace, medical equipment, and food processing 1 . The hardness and strength of 304 stainless steel are relatively low, while its plasticity and toughness are relatively high. Moreover, its thermal conductivity is inferior, leading to high cutting temperature, severe work hardening and more machining force, which gave rise to the production of continuous and non-breakable chips 2 . These can easily damage the surface of the workpiece 3 , and seriously affect its surface accuracy and decrease the durability of the cutting tool, ultimately reducing processing efficiency and quality 4 , 5 . Therefore, the cutting force continues to be one of the essential elements in the investigation of the machinability of 304 stainless steel.
To accurately depict the mechanism of cutting force formation, it is necessary to analyze the shear zone at the interaction between tool tip and workpiece. Oxley 6 was the first to propose the parallel shear zone model, suggesting that the shear zones were parallel and bisected by primary shear plane. However, Astakhov 7 and Tounsi et al. 8 found after experimental researches that the shear zones were not bisected, although they were parallel. Based on the unequal division shear zone model, Zhou et al. 9 established a temperature distribution model. After comparative analysis, their predicted results indicated that the present model was in good agreement with the actual experimental results. Therefore, to delve into the stress–strain relationship of materials, researchers have established various constitutive models, such as the Bonder-Partom model 10 , Johnson–Cook (J-C) model 11 and Zerillo-Armstrong model 12 . Among them, the J–C model is most widely applied. Its main parameters are: the initial yield stress A , the material strain strengthening coefficient B , the strain rate sensitivity C , the thermal softening index m , and the hardening index n .
Typically, the applicable strain rate range in Split Hopkinson Pressure Bar (SHPB) experiments lies between 10 3 s −1 and 10 4 s -1 , while in quasi-static tests, it spans from 10 –4 s -1 to 10 –1 s -1 . However, during machining processes, the strain rate of the material can reach up to 10 5 s -1 13 . This indicates that the constitutive parameters derived from SHPB or quasi-static tests do not accurately represent the stress–strain relationship of materials under cutting conditions. Therefore, many scholars have conducted a series of studies on constitutive models and their parameters. Hou et al. 14 studied the plastic response of hot-extruded Mg-10Gd-2Y-0.5Zr alloy under both quasi-static and wide range temperature, finally obtained an improved J–C model. Meng et al. 15 obtained two J-C constitutive models for ADC12 alloy using split Hopkinson pressure bar (SHPB) experiments. The comparison between simulated flow stress and experimental data suggested that the refined J-C constitutive model offered a more precise prediction of flow stress under conditions of high strain rates and elevated temperatures. Chen et al. 16 identified J-C constitutive parameters ( C and m ) by conducting two-dimensional (2-D) cutting force simulations via orthogonal cutting experiments for Al6063 aluminum material, and subsequently obtained other constitutive parameters ( A , B , and n ) by compression tests. It was found that this method was much more economical and could obtain more accurate simulated cutting forces, by comparison with the traditional SHPB experiment. Shen et al. 17 acquired the J-C constitutive model of TC17 titanium alloy through SHPB experiment, and also obtained another J-C constitutive model via parameter identification. By contrast, the model parameters obtained by parameter identification were more suitable for the prediction of cutting forces.
Additionally, due to the inability of constitutive parameters to accurately characterize the stress–strain relationship under specific operational conditions, many scholars have conducted studies on the numerical optimization of constitutive parameters Nguyen and Hosseini 18 combined J-C flow stress model with Oxley machining model to calculate the cutting forces during milling, finally obtained the J-C constitutive parameters ( C , m ) and thermal coefficients ( η , ψ ) of AISI 4340 hardened steel. They found that the results of predicted cutting forces revealed great consistency with its experimental value. Zhou et al. 19 realized the least squares identification of J-C constitutive parameters and improved the prediction accuracy of cutting force by modeling and simulation for H13 steel and Ti-6Al-4 V titanium alloy. Zou et al. 13 implemented the reverse identification of J-C parameters of 304 stainless steel by adopting the minimum error between experimental value and simulated value of cutting force as the objective function of genetic algorithm. They also established the three-dimensional (3-D) model as the initial model for finite element iteration, compared the experimental cutting force, chip morphology, and residual stress. It was found that the constitutive parameters A and B had a much greater impact on cutting force than parameters C , m , and n during simulation. According to hot tensile test, neural networks and genetic algorithm, Yao et al. 20 obtained the constitutive parameters of AA6061 aluminum alloy via reverse identification. It was reported that the accuracy of hybrid identification method was better than single optimized algorithm, since the initial population of genetic algorithm was optimized by neural network.
The objective of this study is to efficiently obtain more accurate constitutive parameters via genetic algorithm. Firstly, considering the influence of tool parameters on cutting zone and the impact of feed motion on the rake (flank) angle, an unequal division shear zone model was established. Then, the effect of the constitutive parameter C on chip morphology was figured out via finite element simulation. Furthermore, turning experiments were conducted to analyze the effects of experimental parameters (spindle speed n , feed rate f , and cutting depth a p ) on cutting force, and J-C constitutive model parameters were identified via genetic algorithm. Finally, the feasibility of the reverse identification method and the reliability as well as accuracy of constitutive model were verified by comparing the predicted results of empirical formula with simulation results.
Cutting zone modeling
Figure 1 exhibits the scheme of cutting zone during feed motion. During one complete revolution of the spindle, the tool progresses from position I to position II. The cutting thickness a is the dimension of the cutting layer measured perpendicularly to the main cutting edge within the base, representing the load on the cutting edge per unit length; The cutting width b refers to the projection of working length of the main cutting edge on base plane. The relationships between cutting thickness a , cutting width b , feed rate f (The distance S , which the tool travels as the workpiece makes one full rotation, is numerically equivalent to the feed rate f ), and cutting depth a p are as follows:
where, \({K}_{r}\) refers to the tool cutting edge angle.
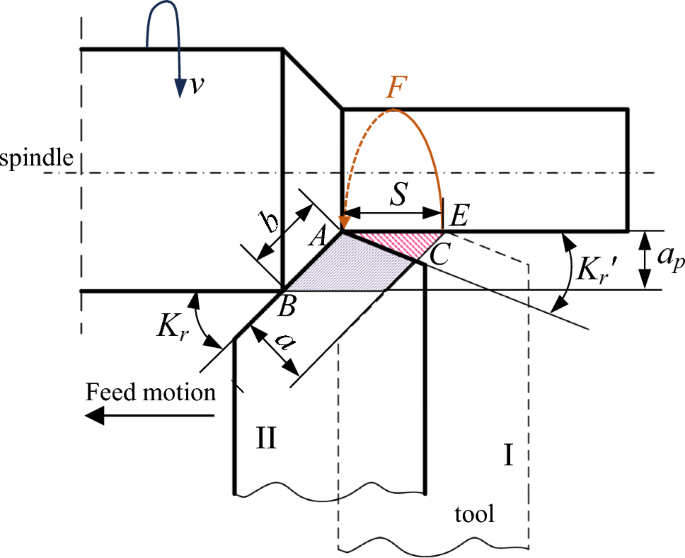
Cutting thickness and cutting width.
Theoretical cutting zone S A was calculated through cutting thickness a and cutting width b :
Due to the presence of end cutting edge angle \({K}_{r}{\prime}\) on turning tool, and the trajectory of tool tip following an arc EFA along the outer circumference of the workpiece, a small residual zone (see S △ ACE shown in Fig. 1 ) is left on the machined workpiece surface. Therefore, the actual cutting zone could be obtained via subtracting the theoretical cutting zone \({S}_{\text{A}}\) by the residual zone S∆ ACE :
As shown in Fig. 2 , due to the feed motion of lathe machining, the actual cutting surface is a helical surface with a helix angle \(\mu\) , which will affect the rake angle \({\gamma }_{0}\) and flank angle \({\alpha }_{0}\) :
where, d is workpiece diameter (mm). Then, the actual rake angle \({\gamma }_{0}{\prime}\) and flank angle \({\alpha }_{0}{\prime}\) are:
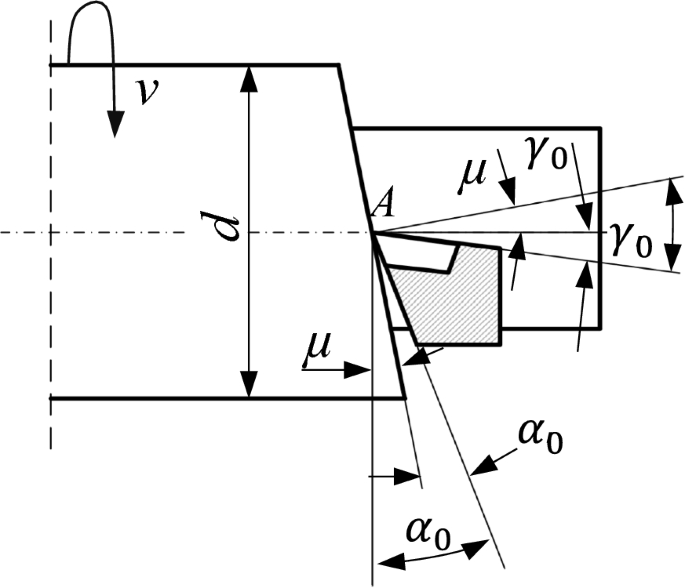
The influence of feed motion on rake and flank angles.
Establishment of unequal division shear zone model and constitutive equation
As shown in Fig. 3, a shear zone model of the workpiece during the turning process has been constructed. Longitudinal turning is considered as oblique cutting (see Fig. 3 a) due to the existence of the inclination angle \({\lambda }_{s}\) . The shear zone in the equivalent plane P n of orthogonal cutting is shown in Fig. 3 b) after equivalently converting oblique cutting to orthogonal cutting 21 , 22 .
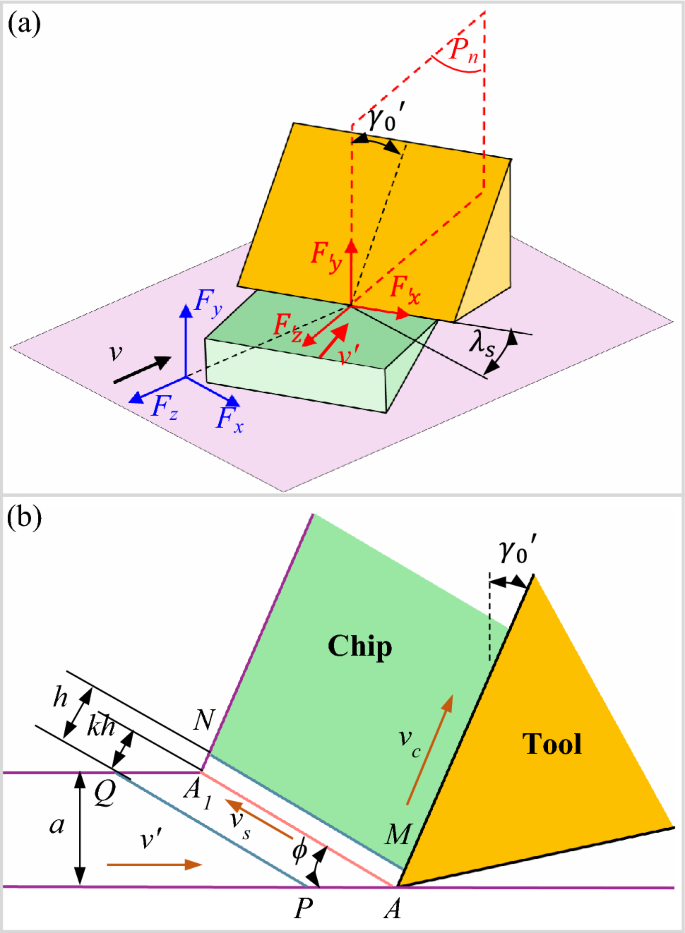
( a ) Oblique cutting model; ( b )The unequal division shear zone model in the equivalent plane P n 21 .
The projections of cutting force F y , main cutting force F z , and cutting speed v in the equivalent plane P n are denoted as F y ', F z ', and v ', respectively. Their relationships are as follows:
It is reported in literature 23 that the shear zone of cutting deformation was located at the contacting point of tool tip and workpiece, and the primary shear zone was very narrow with high strain rate. Oxley 6 thought that the primary shear plane bisected the primary shear zone and was parallel to the initial shear plane and final shear plane. Astakhov 7 believed that the speed of machining chips within the shear zone varied, and divided it into narrow and wide zones. Tounsi 8 continued Astakhov's theory and defined the thickness of the wide zone as k times the overall thickness of the primary shear band. Li et al. 24 employed an unequal division shear zone model to predict the cutting forces during orthogonal cutting, and their predicted results were in good agreement with the experimental outcomes. Therefore, this study established an unequal division shear zone model (see Fig. 3 b).
As shown in Fig. 3 b), the shear velocity v s is parallel to the primary shear plane AA 1 , while the chip velocity v c is parallel to the rake face of tool. The primary shear plane AA 1 is parallel with the initial shear plane PQ and the final shear plane MN , dividing the shear zone into two unequal parts. The distance from PQ to MN is h , and the distance from AA 1 to PQ is k times h . The relationship between the shear zone unequal division coefficient k , the shear angle ϕ and the actual rake angle \({\gamma }_{0}{\prime}\) is obtained from literature 8 :
The approximation formula of shear angle \(\phi\) by Lee and Shaffer gives:
The friction angle \(\beta\) can be obtained from the following formula:
The strain \(\varepsilon_{AB}\) of the primary shear plane:
The strain rate \(\dot{\varepsilon }_{AB}\) of the primary shear plane:
The shear stress \(\tau_{AB}\) of the primary shear plane:
The temperature \(T_{AB}\) of the primary shear plane:
where, \(\rho\) refers to the material density, \({C}_{p}\) represents the specific heat capacity. Through the relationship between \({\tau }_{AB}\) and principal stress σ AB , then \({\sigma }_{AB}=\sqrt{3}{\tau }_{AB}\) . \({\tau }_{0}=\frac{{\sigma }_{e}}{\sqrt{3}}\) , and \({\sigma }_{e}\) refers to the elastic limit stress.
The constitutive model 25 is a general model that describes all the fundamental information during material deformation process. It can depict the relationships among various thermomechanical parameters induced by mechanical-thermal coupling in deformation, such as stress and strain, strain rate and temperature.
In this study, the Johnson–Cook constitutive model is used to describe the relationship of stress and strain. Its expression is as follow:
where, A represents the initial yield stress, MPa; B is the material strain strengthening coefficient, MPa; C represents the strain rate sensitivity; m expresses the thermal softening index; n is the hardening index exponent; \(\sigma\) is the flow stress, MPa; \(\varepsilon\) refers to the equivalent plastic strain; \(\dot{\varepsilon }\) ̇ denotes the equivalent strain rate, \({s}^{-1}\) ; \(\dot{{\varepsilon }_{0}}\) is the reference strain rate of 304 stainless steel material, \({s}^{-1}\) ; \(T\) expresses the material deformation temperature, ℃; \({T}_{m}\) is the melting temperature, ℃; \({T}_{r}\) is the room temperature, ℃.
According to the least squares principle, the fitness function of genetic algorithm is obtained as 8 :
N represents the number of experimental groups, i expresses the experimental sequence number, and k 1 refers to a proportionality constant value -1.
Turning experiments and result analysis
The cutting system used in this study is shown in Fig. 4 . The material employed in cutting experiment was 304 stainless steel rod, with a diameter of 40 mm and a length of 400 mm. The cutting equipment used was ordinary lathe (CA6140 400 × 1000), assembled with a turning tool holder (MWLNR2020K08), made of 40CR-42CRMO, with tool cutting edge angle \({K}_{r}\) of 95°, end cutting edge angle \({K}_{r}{\prime}\) of 5°and a dimension of 20 × 125 × 20 mm. The model of turning insert was WNMG080408-SM, made of WM3125, with corner radius of 0.8 mm, rake angle \({\gamma }_{0}\) of 6° and inclination angle \({\lambda }_{s}\) of 6°.
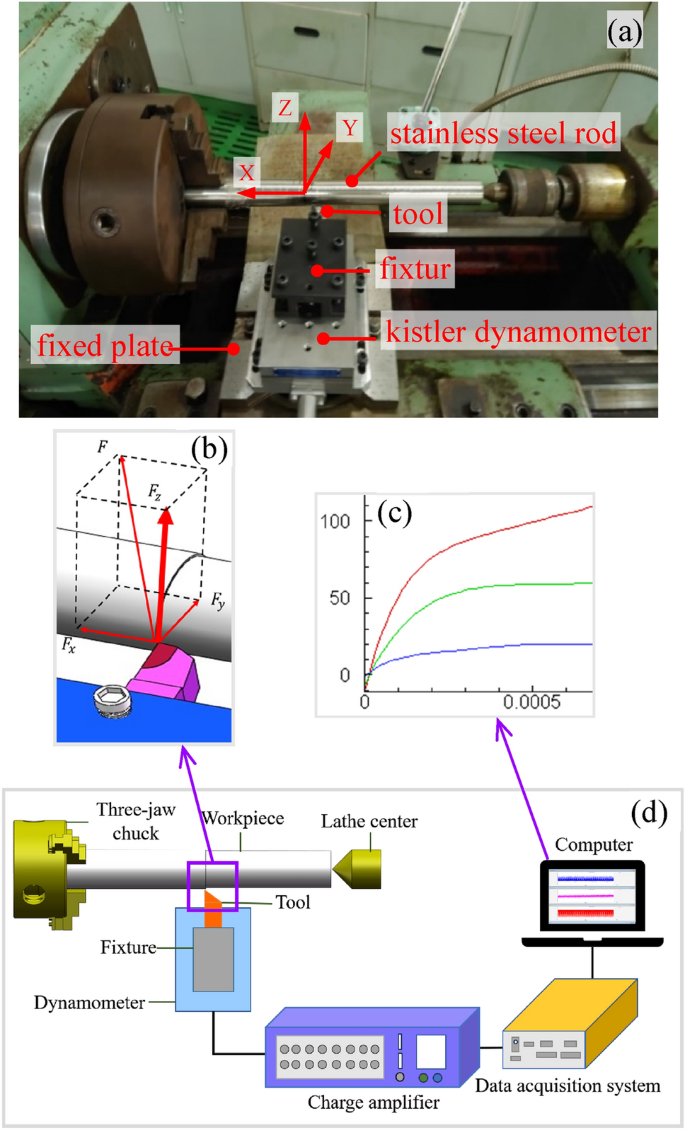
Turning test devices.
In this experiment, the cutting forces were measured with a force measuring system, which include a Kistler dynamometer type 9257B, charge amplifier type 5080A, 5697A data acquisition system, and computer with the DynoWare software, as shown in Fig. 4 d. In actual turning process on ordinary lathe, the lathe spindle speed ( n ) remained constant, whereas without the help of computer numerical control, the cutting speed ( v ) would vary with the change of rod diameter. Therefore, the spindle speed rather than cutting speed was focused through experimental discussing and analyzing. As shown in Table 1 , the spindle speed ( n ), cutting depth ( a p ), and feed rate ( f ) were chosen as experimental parameters. As shown in Table 2 , a three-factor three-level cutting experiment was designed with a total of 27 groups experimental data, additionally, the cutting speed is also provided. Table 3 and Fig. 5 were obtained by range analysis of F z , Delta represents the extent to which the parameter affects the cutting force F z . The larger the Delta value, the greater its impact, resulting in a smaller assigned Rank.
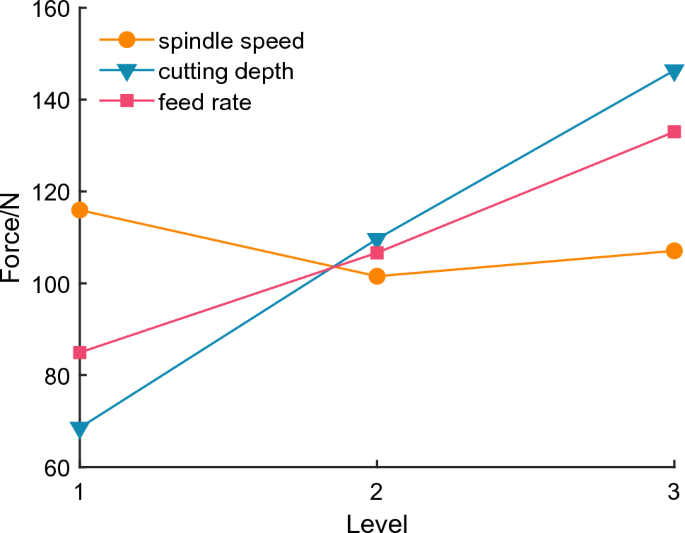
The influence of experimental parameters on cutting force.
According to the data presented in Table 3 , it is evident that the cutting depth (Delta = 77.82, Rank = 1) exerts the most significant influence on F z . Subsequently, the feed rate (Delta = 48.05, Rank = 2) follows in terms of impact, and lastly, the spindle speed (Delta = 14.4, Rank = 3). Based on the findings presented in Fig. 5 , the cutting depth and feed rate have a positive correlation with the cutting force F z . Equation ( 1 )–( 4 ) demonstrate that augmenting the cutting depth and feed rate will have an impact on the cutting width and thickness, thereby resulting in an expansion of the cutting zone. During the initial stages of cutting experiments, cutting force measurements were conducted at spindle speeds of 560 and 710 r/min. The impact of the workpiece diameter on the cutting speed proved to be minimal. In the range of 560 to 710 r/min, as the spindle speed increases, the cutting speed also increases, leading to a greater plastic deformation of the workpiece within a given time frame. Consequently, the spindle speed has a positive correlation with the cutting force F z . While, at a spindle speed of 400 r/min, significant workpiece wear was observed. Results in a decrease in cutting speed and an increase in the rake angle during the feed motion, led to a reduction in both shear surface strain and strain rate. Consequently, the spindle speed between 400 and 560 r/min was inversely correlated with the cutting force F z .
Genetic algorithm identification of J–C model constitutive parameters
Genetic algorithm (GA) is a method for searching the optimal solution by simulating the natural evolutionary process. Renowned for its robust global search capability, GA has been applied in parameter identification. Zhou et al. utilized the genetic algorithm to identify and improve J-C constitutive model, achieving higher precision in predicting cutting forces 13 , 19 . Such findings indicated that genetic algorithm could effectively search for optimal solution in multifactorial cutting experiments to find the best approaches. In this paper, GA was employed to determine the parameters of J-C constitutive model, as depicted in Fig. 6 . Multiple sets of initial values for J-C constitutive parameters was firstly generated at random, then convert these values into binary encoding and input them into the fitness function derived from the least squares principle (as shown in Eq. ( 16 )) to calculate the fitness values. Furthermore, binary encodings were chosen through a random uniform distribution, followed by crossover and mutation operations on the data to produce offspring data. This procedure was reiterated in a secondary loop until the fitness values satisfied the set criteria or the iteration count reached its maximum, culminating in the release of optimal solution.
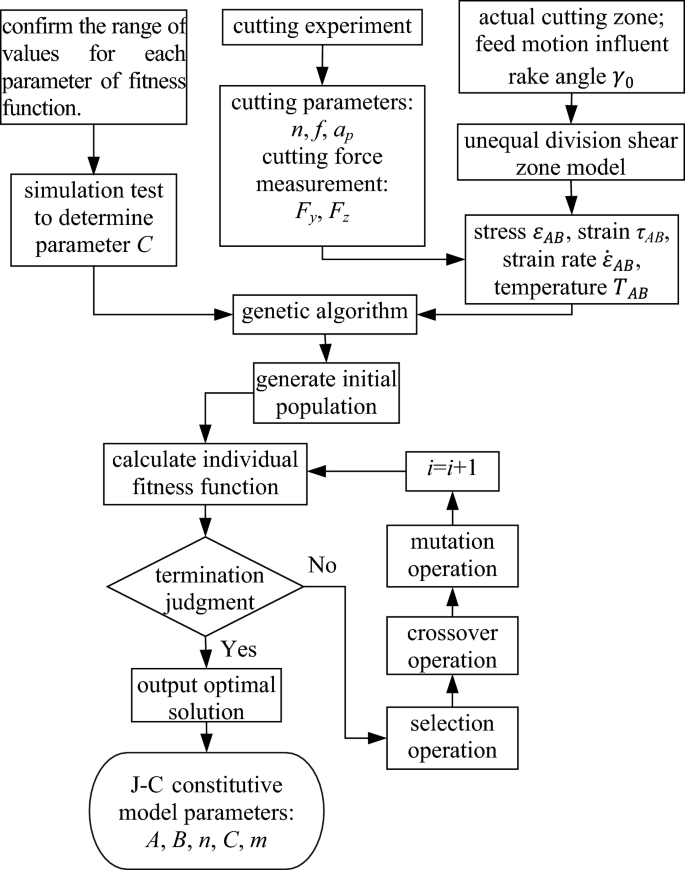
Inverse identification process of constitutive parameters.
To enhance the search velocity and precision of parameter identification using genetic algorithm, it was imperative to define logical bounds for the J-C constitutive parameters. This is crucial because different identification techniques can produce varying parameter values. In pursuit of the optimal solution for the fitness function mentioned in Fig. 6 , this study collated data from extensive literature reviews, presenting constitutive parameters values, as detailed in Table 4 .
From Table 4 , the range of constitutive parameters can be determined as follows:
Initially, the genetic algorithm was used to identify the five parameters A , B , n , C , and m . However, only the value of C consistently remained at 0.14 according to the identification results, falling into a local minimum. The values of the other four parameters ( A , B , n , m ) would vary within a certain range, and when the five parameters obtained via the genetic algorithm were used for simulation, the simulation could not proceed normally, and the force curve could not stabilize.
Research revealed that among the five constitutive parameters A , B , n , C , and m , A and B have a significant impact on simulated cutting force, while the impact of C is minor 13 . However, after simulation tests, within the range of 0.004 ≤ C ≤ 0.14, larger value of parameter C resulted in unstable simulation result and irregular chip morphology. Parameter C has the largest range of variation comparing to other four parameters ( A , B , n , m ) in Table 4 , then it is prone to falling into local minimum in the genetic algorithm, resulting in premature termination of the simulation. Therefore, it is necessary to discuss and analyze the value of C to ensure the generation of regular chips. Since the C value of 0.0067 in the JC1 model from Table 4 is the smallest, research based on the constitutive parameters of the JC1 model was conducted. Taking the parameter C = 0.0067 (0%) from the JC1 model as a baseline, the values of C are set to 0.004 (-40%), 0.0054 (-20%), 0.008 (20%), 0.0094 (40%), and the maximum value of C from Table 4 , which is 0.136, with a total of six levels for simulation analysis using the first set of cutting parameters (spindle speed n with 400 r/min, cutting speed v with 39.94 m/min, feed rate f with 0.08 mm/r, cutting depth with 0.1 mm) in Table 2 . The resulting chip morphologies are shown in Fig. 7 . Additionally, different values of the constitutive parameter C resulted in different distributions of shear zone and strain rate. As shown in Fig. 8 , the shear zone and strain rate distribution results were obtained through software analysis; Along the radial center of strain rate cloud diagram in the shear zone, the strain rate and stress were extracted, and their maximum values are recorded in Table 5 . Combining Fig. 7 a and 8 a, when C was 0.136, the maximum strain rate and stress were 58,201.29 \({s}^{-1}\) and 2211.28 MPa (as shown in Table 5 ), and no chip was produced. The reason is that the increase of C value leading to higher stress in the shear zone and elevated cutting temperatures, which surpassed the set melting point, finally preventing chip formation. As shown in Fig. 7 e,f, when C were 0.008 and 0.0094, the simulation temperature remains high, hence, no complete chips were formed; by comparing the chip morphologies in Fig. 7 b–d, it is observed that the most regular chips are produced when C was 0.0054, obtaining the most stable simulation. And as shown in Table 5 , when C is 0.0054, the simulation force F s (65.31 N) is closest to the experimental force F z (62.46 N) corresponding to Table 2 . Consequently, C was determined to be 0.0054, then reverse identification was utilized to obtain four remaining parameters ( A , B , n , m ).
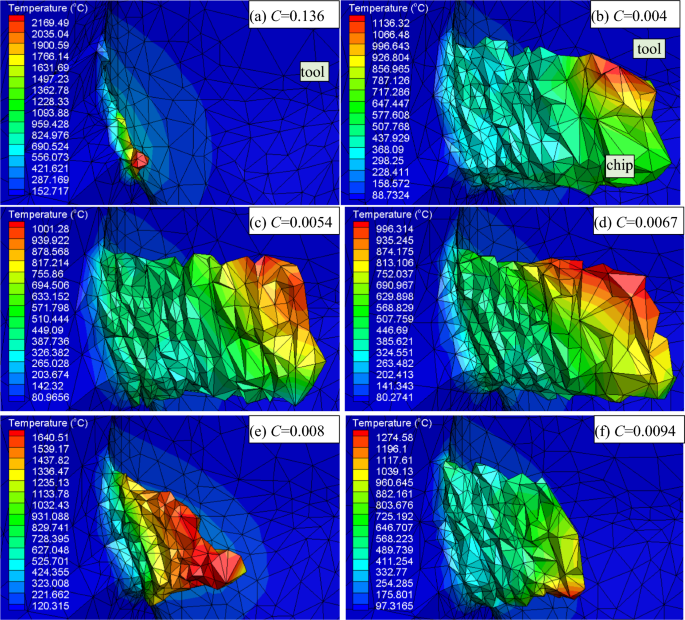
Simulation results of chip morphology with different constitutive parameter C values.
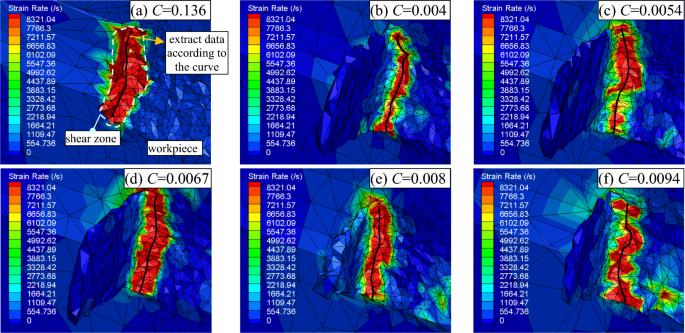
Distributions of shear zone and strain rates for different values of the constitutive parameter C.
( A = 452.0, B = 694.0, n = 0.311, m = 0.996).
The fitness function and the constraint matrix of constitutive parameters were coded. Genetic algorithm was employed for computation via MATLAB with the following parameters: population size was 20, evolution times was 500, and algorithm tolerance is 10 –6 . The fitness evolution trend was depicted in Fig. 9 . By the time the population reached the 190th generation, it exhibited signs of stabilization and convergence. At this juncture, genetic algorithm terminated its operation. The output values for parameters A , B , n , and m were determined as the optimal solutions. Hence, the constitutive parameters for 304 stainless steel, derived from genetic algorithm, are presented in Table 6 and are designated as the JCM model.
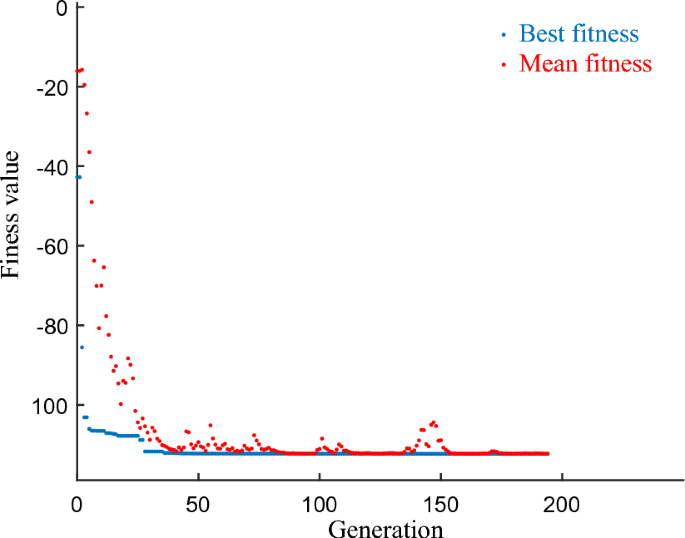
Fitness trend.
FEM simulation and empirical model prediction of cutting force
Fem simulation.
In this study, a three-dimensional turning simulation was conducted via FEM software. Given that the workpiece diameter exceeded 20 mm, its circumferential radius was sufficiently large, thus the circular motion at the tool-workpiece contact point in Fig. 10 a could be approximated as the linear motion shown in Fig. 10 b. The designated cutting zone had dimensions of length ( L ) 5 mm, height ( H ) 2 mm, width ( W ) 1 mm and the tool tip model was imported. As the cutting speed cannot be fixed on an ordinary lathe, the spindle speed was selected as experimental parameter. Combined with the diameter of 304 stainless steel rod, the spindle speed was translated into cutting speed. The model mesh employed an adaptive grid, where software automatically generated a denser mesh in the cutting area and subdivided the mesh when the stress was higher. The study carried out two different simulations: Simulations based on JC1 model (as detailed in Table 4 ) and corresponding material parameters in literature 26 Simulations based on the JCM model (from genetic algorithm, as detailed in Table 6 ), and the material parameters presented in Table 7 .
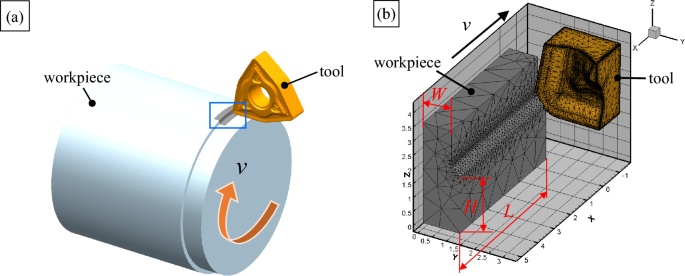
The 3-D simulation model.
Empirical model prediction
Cutting force prediction could be implemented not only through simulation but also using empirical formulas, such as the Eq. ( 19 ) 30 . In this study, the data of Table 2 was substituted into the Eq. ( 19 ) to conform the undetermined constants.
In the equation, C 1 , a 1 to a 3 are all undetermined constants.
To linearize the nonlinear function, logarithm calculation was simultaneously executed for the both sides of Eq. ( 19 ), as shown in Eq. ( 20 ) 30 :
Let \(b_{0} = \lg c_{1} + a_{1} \lg n + a_{2} \lg f + a_{3} \lg a_{p}\) \(x_{2} = \lg f,\,x_{3} = \lg a_{p}\) With these, the linear regression equation was obtained:
After executing multivariate linear regression on Eq. ( 21 ), the resultant empirical prediction model for cutting force was derived as:
Figure 11 shows the regression residuals of prediction model. All residual data contains zero point, signifying the relative reliability of the prediction model. This empirical prediction model was referred to as EXP model. Within this model, the absolute value of corresponding exponent signifies the extent of its influence on predicted force. According to the Eq. ( 22 ), it's evident that cutting depth (0.6921) exerts the most significant influence on cutting force, followed closely by feed rate (0.6899), while spindle speed (-0.2055) exhibits the least impact.
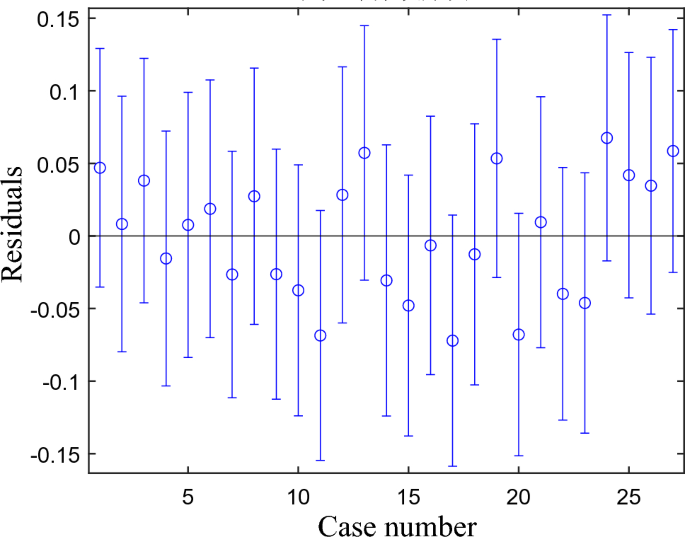
Residual case order plot.
As shown in Table 8 , the cutting force errors between each model value and its experimental value were calculated. Based on Table 8 , a cutting force graph was depicted in Fig. 12 and an error graph was shown in Fig. 13 . In JC1 model, the average error of cutting force was 16.60%, with a minimum value of 2.03% and a maximum value of 55.51%. The JC1 model exhibits a large mean error and fluctuation range (50%). This is attributed to the fact that the J-C constitutive parameters obtained from the SHPB test are just applicable within a strain rate range of 10 3 s -1 ~ 10 4 s -1 . However, under turning conditions, the material's strain rate can reach 10 5 s −1 . Therefore, the JC1 model could not accurately reflect actual turning experiment situation. The EXP model reported an average error of 8.54%, with values spanning from 1.56% at the lowest to 18.11% at the highest. Its error fluctuation was relatively small, however, as shown in Table 8 , the predicted force F z reached its maximum value of 185.09 N in the 9th group (n = 400 r/min, f = 0.15 mm/r, a p = 0.3 mm), which is inconsistent with the trend of the experimental values of cutting force. While JCM model, identified through genetic algorithm, showed an average error of 6.38%, with its values fluctuating between 0.51% and 14.80%. The error range, maximum value, and average value of cutting force in JCM model were all smaller than those of EXP model, generally speaking, the error fluctuation of this model was the smallest. In this study, compared to EXP model, JCM model not only more precisely depicted the changes of actual experimental cutting force, but also coincided with actual cutting force better. It should be noted that EXP model was predicated via only three parameters—spindle speed n , feed rate f , and cutting depth a p . While JCM model encompasses more factors of cutting process, such as the variations of cutting zone, feed motion, changes of workpiece diameter, etc.
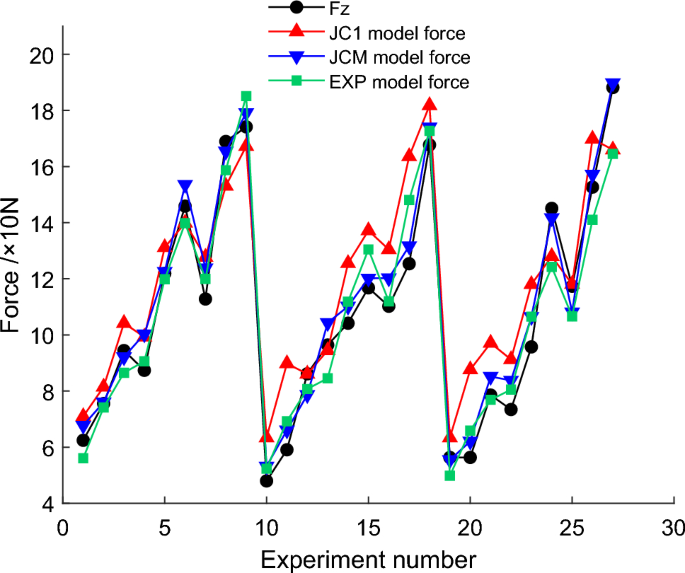
Cutting force graph.
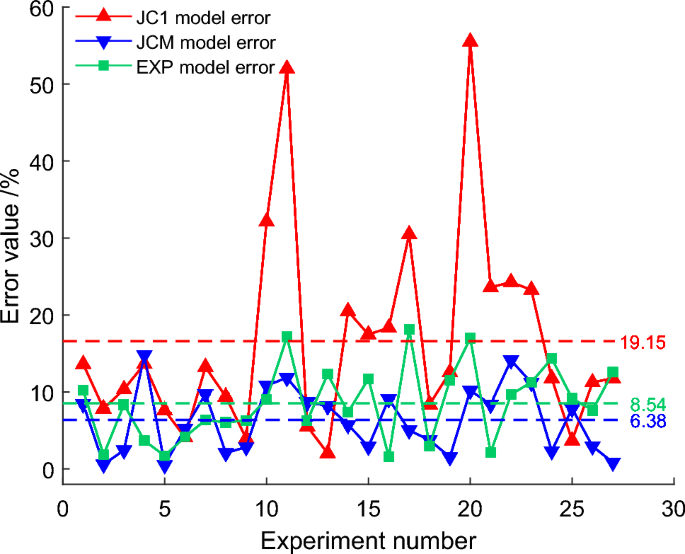
Error graph.
1. Parameter optimization of Johnson–Cook (J-C) constitutive model for 304 stainless steel was realized by utilizing the characteristics of genetic algorithm that could efficiently obtain the optimal solutions. It could accurately reflect the state of turning machining under high strain rate conditions. The simulated values of cutting force aligned closely with its experimental values, exhibiting minimal deviation and maintaining the same trend of value changes.
2. Considering the influence of actual cutting zone and the impact of feed motion on the rake (flank) angle, an unequal division shear zone model was established in the construction process of J-C constitutive model. FEM simulation results implied that, if other parameters were held constant, the increase of C value would lead to greater stress in the shear zone and thicker chip layer that accumulated at the tool tip, causing temperature increase, resulting in the instability of cutting force.
3. Range analysis was implemented for turning experimental data, comparing the Delta and Rnak values, it was discerned that both cutting depth and feed rate had a positive correlation with cutting force. However, within the range of 400 ~ 710 r/min, spindle speed initially exhibited a negative correlation and later indicated a positive correlation with cutting force, due to the diminishing workpiece diameter which caused a decrease in cutting speed and an increase in rake angle.
4. In JC1 model, the maximum error between simulated and experimental cutting forces was 55.51%, with an average error of 16.60%. This large fluctuation in error indicated that the STN model could not accurately reflect actual turning experiment conditions. EXP model was derived through multiple linear regression, its maximum error between predicted and experimental values was 18.11%, with an average error of 6.38%. Moreover, the predicted values of cutting force failed to consistently align with experimental results. The range of cutting forces simulated by JCM model was 53.19 ~ 189.75 N. The maximum error between experimental value and simulated value was 14.8%, with an average error of 6.38%. These results demonstrated that JCM model suited the actual experiments conducted in this study the most.
5. It was evident that the error range and average values of JCM model identified in this paper were superior to those of JC1 and EXP models. It suggested that JCM model could accurately capture the variations of cutting force for 304 stainless steel workpieces with different diameters, especially at low to medium spindle speeds. The feasibility of reverse identification method based on genetic algorithm and the reliability as well as accuracy of J-C constitutive model were also verified.
Data availability
The datasets used and/or analysed during the current study are available from the corresponding author on reasonable request. Correspondence and requests for materials should be addressed to C.W.
Wang, H., Chen, L., Liu, D., Song, G. & Tang, G. Study on electropulsing assisted turning process for AISI 304 stainless steel. Mater. Sci. Technol. 31 , 1564–1571 (2015).
Article ADS CAS Google Scholar
Zahrani, E. G., Azarhoushang, B. & Wilde, J. Evaluation of chip breaking in combined laser-turning process. J. Manufact. Process. 77 , 722–729 (2022).
Article Google Scholar
Ahmed, Y. S., Paiva, J. M. & Veldhuis, S. C. Characterization and prediction of chip formation dynamics in machining austenitic stainless steel through supply of a high-pressure coolant. Int. J. Adv. Manuf. Technol. 102 , 1671–1688 (2019).
Du, H., Karasev, A., Bjork, T., Loyquist, S. & Jonsson, P. G. Assessment of Chip Breakability during Turning of Stainless Steels Based on Weight Distributions of Chips. Metals 10 , 675 (2020).
Xu, Y., Wan, Z., Zou, P. & Zhang, Q. Experimental study on chip shape in ultrasonic vibration-assisted turning of 304 austenitic stainless steel. Adv. Mech. Eng. 11 , 1–17 (2019).
Oxley, P. A mechanics of machining approach to assessing machinability. In Proceedings twenty-second international machine tool design and research conference (ed. Oxley, P.) (Springer, 1982).
Google Scholar
Astakhov, V. P., Osman, M. O. M. & Hayajneh, M. T. Re-evaluation of the basic mechanics of orthogonal metal cutting: velocity diagram, virtual work equation and upper-bound theorem. Int. J. Mach. Tools Manufact. 41 , 393–418 (2001).
Tounsi, N., Vincenti, J., Otho, A. & Elbestawi, M. A. From the basic mechanics of orthogonal metal cutting toward the identification of the constitutive equation. Int. J. Mach. Tools Manufact. 42 , 1373–1383 (2002).
Zhou, F., Wang, X., Hu, Y. & Ling, L. Modeling temperature of non-equidistant primary shear zone in metal cutting. Int. J. Therm. Sci. 73 , 38–45 (2013).
Bodner, S. R. & Partom, Y. Constitutive equations for elastic-Viscoplastic strain-hardening materials. J. Appl.Mech. 42 , 385–389 (1975).
Article ADS Google Scholar
Johnson, G. R. & Cook, W. H. Fracture characteristics of three metals subjected to various strains, strain rates, temperatures and pressures. Eng. Fract. Mech. 21 , 31–48 (1985).
Zerilli, F. J. & Armstrong, R. W. Dislocation-mechanics-based constitutive relations for material dynamics calculations. J. Appl. Phys. 61 , 1816–1825 (1987).
Zou, Z. et al. Research on inverse identification of johnson-cook constitutive parameters for turning 304 stainless steel based on coupling simulation. J. Mater. Res. Technol.-JMRT 23 , 2244–2262 (2023).
Article CAS Google Scholar
Hou, Q. Y. & Wang, J. T. A modified Johnson-Cook constitutive model for Mg–Gd–Y alloy extended to a wide range of temperatures. Comput. Mater. Sci. 50 , 147–152 (2010).
Meng, X., Lin, Y. & Mi, S. An improved Johnson-cook constitutive model and its experiment validation on cutting force of ADC12 aluminum alloy during high-speed milling. Metals 10 , 1038 (2020).
Chen, X., Wang, X., Xie, L., Wang, T. & Ma, B. Determining Al6063 constitutive model for cutting simulation by inverse identification method. Int. J. Adv. Manuf. Technol. 98 , 47–54 (2018).
Shen, X., Zhang, D., Yao, C., Tan, L. & Li, X. Research on parameter identification of Johnson-Cook constitutive model for TC17 titanium alloy cutting simulation. Mater. Today Commun. 31 , 103772 (2022).
Nguyen, N. & Hosseini, A. Direct calculation of Johnson-Cook constitutive material parameters for oblique cutting operations. J. Manufact. Process. 92 , 226–237 (2023).
Zhou, T. et al. Inverse identification of material constitutive parameters based on co-simulation. J. Mater. Res. Technol. 20 , 221–237 (2022).
Yao, D., Duan, Y., Li, M. & Guan, Y. Hybrid identification method of coupled viscoplastic-damage constitutive parameters based on BP neural network and genetic algorithm. Eng. Fract.Mech. 257 , 108027 (2021).
Li, B. & Zhang, R. Analytical prediction of cutting forces in cylindrical turning of 304 stainless steel using unequal division shear zone theory. Int. J. Adv. Manuf. Technol. 124 , 3201–3215 (2023).
Fu, Z., Yang, W., Wang, X. & Leopold, J. Analytical modelling of milling forces for helical end milling based on a predictive machining theory. Proc. CIRP 31 , 258–263 (2015).
Lalwani, D. I., Mehta, N. K. & Jain, P. K. Extension of Oxley’s predictive machining theory for Johnson and Cook flow stress model. J. Mater. Process. Technol. 209 , 5305–5312 (2009).
Li, B., Wang, X., Hu, Y. & Li, C. Analytical prediction of cutting forces in orthogonal cutting using unequal division shear-zone model. Int. J. Adv. Manuf. Technol. 54 , 431–443 (2011).
Johnson, G. R. & Cook, W. A Constitutive Model And Data For Metals Subjected To Large Strains, High Strain Rates And High Temperatures. (2018).
Zhang, W., Wang, X., Hu, Y. & Wang, S. Predictive modelling of microstructure changes, micro-hardness and residual stress in machining of 304 austenitic stainless steel. Int. J. Mach. Tools Manufact. 130–131 , 36–48 (2018).
Ling, L., Li, X., Wang, X. & Hu, Y. Constitustive model of stainless steel 0Cr18Ni9 and Its influence on cutting force prediction. China Mech. Eng. 23 , 2243–2248 (2012).
CAS Google Scholar
Laakso, S. V. A., Agmell, M. & Ståhl, J.-E. The mystery of missing feed force—The effect of friction models, flank wear and ploughing on feed force in metal cutting simulations. J. Manufact. Process. 33 , 268–277 (2018).
Li, J. C., Chen, X. W. & Huang, F. L. FEM analysis on the deformation and failure of fiber reinforced metallic glass matrix composite. Mater. Sci. Eng. A 652 , 145–166 (2016).
Xu, C. et al. Experimental tests and empirical models of the cutting force and surface roughness when cutting 1Cr13 martensitic stainless steel with a coated carbide tool. Adv. Mech. Eng. 8 , 1687814016673753 (2016).
Download references
Acknowledgements
This work was supported by the National Key R&D Program of China (No. 2018YFE0199100), the Joint Fund of Zhejiang Provincial Natural Science Foundation of China under Grant No. LZY23E050003 and LZY23E050002, and Public Welfare Project of Zhejiang Province (No.LGG22F030013). The authors also sincerely appreciate the technical support from the optical 3D surface profilometer (CHOTEST SuperView W1).
Author information
Authors and affiliations.
College of Engineering, Zhejiang Normal University, Jinhua, 321004, China
Xinyang Jiang, Jinfu Ding, Chengwu Wang, E Shiju, Huadong Wang & Chongqiu Zhou
Key Laboratory of Urban Rail Transit Intelligent Operation and Maintenance Technology & Equipment of Zhejiang Province, Zhejiang Normal University, Jinhua, 321004, China
Chengwu Wang
Zhejiang Shuohe Robot Technology Co., Ltd, Jinhua, 321000, China
College of Mechanical and Electrical Engineering, Shaoxing University, Shaoxing, 321017, China
Weifeng Yao
College of Electrical and Information Engineering, Quzhou University, Quzhou, 324000, China
You can also search for this author in PubMed Google Scholar
Contributions
X.J., J.D. and C.W. proposed the concept of the paper, designed the experiments and wrote the main manuscript text. X.J. prepared Figs. 1 – 13 . S.E., L.H. and W.Y. assisted in the experiment. H.W., C.Z. and W.Y. contributed in data analysis. All authors reviewed the manuscript.
Corresponding authors
Correspondence to Chengwu Wang or Ling Hong .
Ethics declarations
Competing interests.
The authors declare no competing interests.
Additional information
Publisher's note.
Springer Nature remains neutral with regard to jurisdictional claims in published maps and institutional affiliations.
Rights and permissions
Open Access This article is licensed under a Creative Commons Attribution-NonCommercial-NoDerivatives 4.0 International License, which permits any non-commercial use, sharing, distribution and reproduction in any medium or format, as long as you give appropriate credit to the original author(s) and the source, provide a link to the Creative Commons licence, and indicate if you modified the licensed material. You do not have permission under this licence to share adapted material derived from this article or parts of it. The images or other third party material in this article are included in the article’s Creative Commons licence, unless indicated otherwise in a credit line to the material. If material is not included in the article’s Creative Commons licence and your intended use is not permitted by statutory regulation or exceeds the permitted use, you will need to obtain permission directly from the copyright holder. To view a copy of this licence, visit http://creativecommons.org/licenses/by-nc-nd/4.0/ .
Reprints and permissions
About this article
Cite this article.
Jiang, X., Ding, J., Wang, C. et al. Parameter identification of Johnson–Cook constitutive model based on genetic algorithm and simulation analysis for 304 stainless steel. Sci Rep 14 , 21221 (2024). https://doi.org/10.1038/s41598-024-71671-1
Download citation
Received : 20 May 2024
Accepted : 29 August 2024
Published : 11 September 2024
DOI : https://doi.org/10.1038/s41598-024-71671-1
Share this article
Anyone you share the following link with will be able to read this content:
Sorry, a shareable link is not currently available for this article.
Provided by the Springer Nature SharedIt content-sharing initiative
By submitting a comment you agree to abide by our Terms and Community Guidelines . If you find something abusive or that does not comply with our terms or guidelines please flag it as inappropriate.
Quick links
- Explore articles by subject
- Guide to authors
- Editorial policies
Sign up for the Nature Briefing newsletter — what matters in science, free to your inbox daily.

- Analysis of Algorithms
- Backtracking
- Dynamic Programming
- Divide and Conquer
- Geometric Algorithms
- Mathematical Algorithms
- Pattern Searching
- Bitwise Algorithms
- Branch & Bound
- Randomized Algorithms
Genetic Algorithms
Genetic Algorithms(GAs) are adaptive heuristic search algorithms that belong to the larger part of evolutionary algorithms. Genetic algorithms are based on the ideas of natural selection and genetics. These are intelligent exploitation of random searches provided with historical data to direct the search into the region of better performance in solution space. They are commonly used to generate high-quality solutions for optimization problems and search problems.
Genetic algorithms simulate the process of natural selection which means those species that can adapt to changes in their environment can survive and reproduce and go to the next generation. In simple words, they simulate “survival of the fittest” among individuals of consecutive generations to solve a problem. Each generation consists of a population of individuals and each individual represents a point in search space and possible solution. Each individual is represented as a string of character/integer/float/bits. This string is analogous to the Chromosome.
Foundation of Genetic Algorithms
Genetic algorithms are based on an analogy with the genetic structure and behavior of chromosomes of the population. Following is the foundation of GAs based on this analogy –
- Individuals in the population compete for resources and mate
- Those individuals who are successful (fittest) then mate to create more offspring than others
- Genes from the “fittest” parent propagate throughout the generation, that is sometimes parents create offspring which is better than either parent.
- Thus each successive generation is more suited for their environment.
Search space
The population of individuals are maintained within search space. Each individual represents a solution in search space for given problem. Each individual is coded as a finite length vector (analogous to chromosome) of components. These variable components are analogous to Genes. Thus a chromosome (individual) is composed of several genes (variable components).

Fitness Score
A Fitness Score is given to each individual which shows the ability of an individual to “compete” . The individual having optimal fitness score (or near optimal) are sought.
The GAs maintains the population of n individuals (chromosome/solutions) along with their fitness scores.The individuals having better fitness scores are given more chance to reproduce than others. The individuals with better fitness scores are selected who mate and produce better offspring by combining chromosomes of parents. The population size is static so the room has to be created for new arrivals. So, some individuals die and get replaced by new arrivals eventually creating new generation when all the mating opportunity of the old population is exhausted. It is hoped that over successive generations better solutions will arrive while least fit die.
Each new generation has on average more “better genes” than the individual (solution) of previous generations. Thus each new generations have better “partial solutions” than previous generations. Once the offspring produced having no significant difference from offspring produced by previous populations, the population is converged. The algorithm is said to be converged to a set of solutions for the problem.
Operators of Genetic Algorithms
Once the initial generation is created, the algorithm evolves the generation using following operators – 1) Selection Operator: The idea is to give preference to the individuals with good fitness scores and allow them to pass their genes to successive generations. 2) Crossover Operator: This represents mating between individuals. Two individuals are selected using selection operator and crossover sites are chosen randomly. Then the genes at these crossover sites are exchanged thus creating a completely new individual (offspring). For example –
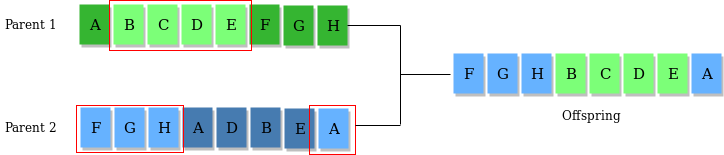
3) Mutation Operator: The key idea is to insert random genes in offspring to maintain the diversity in the population to avoid premature convergence. For example –
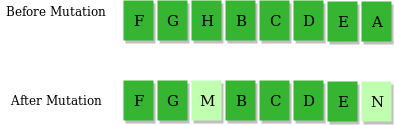
The whole algorithm can be summarized as –
Example problem and solution using Genetic Algorithms
Given a target string, the goal is to produce target string starting from a random string of the same length. In the following implementation, following analogies are made –
- Characters A-Z, a-z, 0-9, and other special symbols are considered as genes
- A string generated by these characters is considered as chromosome/solution/Individual
Fitness score is the number of characters which differ from characters in target string at a particular index. So individual having lower fitness value is given more preference.
Note: Every-time algorithm start with random strings, so output may differ
As we can see from the output, our algorithm sometimes stuck at a local optimum solution, this can be further improved by updating fitness score calculation algorithm or by tweaking mutation and crossover operators.
Why use Genetic Algorithms
- They are Robust
- Provide optimisation over large space state.
- Unlike traditional AI, they do not break on slight change in input or presence of noise
Application of Genetic Algorithms
Genetic algorithms have many applications, some of them are –
- Recurrent Neural Network
- Mutation testing
- Code breaking
- Filtering and signal processing
- Learning fuzzy rule base etc
Please Login to comment...
Similar reads.
- 105 Funny Things to Do to Make Someone Laugh
- Best PS5 SSDs in 2024: Top Picks for Expanding Your Storage
- Best Nintendo Switch Controllers in 2024
- Xbox Game Pass Ultimate: Features, Benefits, and Pricing in 2024
- #geekstreak2024 – 21 Days POTD Challenge Powered By Deutsche Bank
Improve your Coding Skills with Practice
What kind of Experience do you want to share?
Research on Optimization Calculation Model of Distributed Power Reactive Power Based on Genetic Algorithm
New citation alert added.
This alert has been successfully added and will be sent to:
You will be notified whenever a record that you have chosen has been cited.
To manage your alert preferences, click on the button below.
New Citation Alert!
Please log in to your account
Information & Contributors
Bibliometrics & citations, recommendations, research on reactive power and voltage control model under high wind power penetration rate.
Because of the characteristics of the wind resource is random, intermittent,volatility, wind power also has the corresponding features, resulting in wind farm voltage fluctuations. When the wind farm voltage large perturbations, if not enough new ...
Comprehensive learning particle swarm optimization for reactive power dispatch
Reactive power dispatch (RPD) is an optimization problem that reduces grid congestion by minimizing the active power losses for a fixed economic power dispatch. RPD reduces power system losses by adjusting the reactive power control variables such as ...
Power Consumption Calculation of AP-DCD Algorithm Using FPGA Platform
This paper presented the analysis of total power dissipation of AP-DCD algorithm using field programmable gate arrays (FPGAs). The static and dynamic power dissipation investigated using leakage current, supply voltage, effective capacitance and ...
Information
Published in.

Association for Computing Machinery
New York, NY, United States
Publication History
Permissions, check for updates.
- Research-article
- Refereed limited
Contributors
Other metrics, bibliometrics, article metrics.
- 0 Total Citations
- 8 Total Downloads
- Downloads (Last 12 months) 8
- Downloads (Last 6 weeks) 1
View Options
Login options.
Check if you have access through your login credentials or your institution to get full access on this article.
Full Access
View options.
View or Download as a PDF file.
View online with eReader .
HTML Format
View this article in HTML Format.
Share this Publication link
Copying failed.
Share on social media
Affiliations, export citations.
- Please download or close your previous search result export first before starting a new bulk export. Preview is not available. By clicking download, a status dialog will open to start the export process. The process may take a few minutes but once it finishes a file will be downloadable from your browser. You may continue to browse the DL while the export process is in progress. Download
- Download citation
- Copy citation
We are preparing your search results for download ...
We will inform you here when the file is ready.
Your file of search results citations is now ready.
Your search export query has expired. Please try again.
Information
- Author Services
Initiatives
You are accessing a machine-readable page. In order to be human-readable, please install an RSS reader.
All articles published by MDPI are made immediately available worldwide under an open access license. No special permission is required to reuse all or part of the article published by MDPI, including figures and tables. For articles published under an open access Creative Common CC BY license, any part of the article may be reused without permission provided that the original article is clearly cited. For more information, please refer to https://www.mdpi.com/openaccess .
Feature papers represent the most advanced research with significant potential for high impact in the field. A Feature Paper should be a substantial original Article that involves several techniques or approaches, provides an outlook for future research directions and describes possible research applications.
Feature papers are submitted upon individual invitation or recommendation by the scientific editors and must receive positive feedback from the reviewers.
Editor’s Choice articles are based on recommendations by the scientific editors of MDPI journals from around the world. Editors select a small number of articles recently published in the journal that they believe will be particularly interesting to readers, or important in the respective research area. The aim is to provide a snapshot of some of the most exciting work published in the various research areas of the journal.
Original Submission Date Received: .
- Active Journals
- Find a Journal
- Proceedings Series
- For Authors
- For Reviewers
- For Editors
- For Librarians
- For Publishers
- For Societies
- For Conference Organizers
- Open Access Policy
- Institutional Open Access Program
- Special Issues Guidelines
- Editorial Process
- Research and Publication Ethics
- Article Processing Charges
- Testimonials
- Preprints.org
- SciProfiles
- Encyclopedia
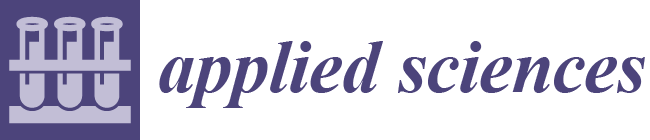
Article Menu

- Subscribe SciFeed
- Recommended Articles
- Google Scholar
- on Google Scholar
- Table of Contents
Find support for a specific problem in the support section of our website.
Please let us know what you think of our products and services.
Visit our dedicated information section to learn more about MDPI.
JSmol Viewer
Research on ride comfort control of air suspension based on genetic algorithm optimized fuzzy pid.
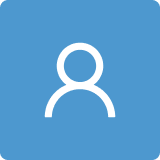
1. Introduction
2. dynamic modeling of air suspension systems, 2.1. vehicle model construction, 2.2. road input model, 2.3. selection of simulation parameters, 3. control algorithm, 3.1. pid controller, 3.2. fuzzy pid controller, 3.2.1. input and output of fuzzy controller, 3.2.2. selection of input and output variable domains, 3.2.3. membership functions of fuzzy variables, 3.2.4. establishment of fuzzy control rules, 3.3. ga f-pid controller, 3.3.1. selection of objective function, 3.3.2. controller ga optimization algorithm, 4. experimental research, 4.1. air suspension system bench test, 4.2. model validation, 5. simulation analysis, 6. conclusions, author contributions, institutional review board statement, informed consent statement, data availability statement, conflicts of interest.
- Ming, M. Intelligent Manufacturing Technology of New Energy Vehicle Lightweight Chassis Suspension System. Mach. Build. Autom. 2021 , 50 , 224–226. [ Google Scholar ]
- Chao, L.Y. Suspension System Optimization and Study on Control of the Electric Vehicle. Master’s Thesis, Qingdao University of Science, Qingdao, China, 2018. [ Google Scholar ]
- Lei, S.S. Research on Control Strategy of Charging and Deflating Characteristics of Electronically Controlled Active Air Suspension. Master’s Thesis, Taiyuan University of Technology, Taiyuan, China, 2019. [ Google Scholar ]
- Rui, B. Nonlinear adaptive sliding-mode control of the electronically controlled air suspension system. Int. J. Adv. Robot. Syst. 2019 , 16 , 1729881419881527. [ Google Scholar ] [ CrossRef ]
- Nazemian, H.; Masih-Tehrani, M. Hybrid Fuzzy-PID Control Development for a Truck Air Suspension System. SAE Int. J. Commer. Veh. 2020 , 13 , 55–69. [ Google Scholar ] [ CrossRef ]
- Li, H.; Li, S.; Sun, W.; Wang, L.; Lv, D. The optimum matching control and dynamic analysis for air suspension of multi-axle vehicles with anti-roll hydraulically interconnected system. Mech. Syst. Signal Process. 2020 , 139 , 106605. [ Google Scholar ] [ CrossRef ]
- Nguyen, V.; Jiao, R.; Zhang, J. Control Performance of Damping and Air Spring of Heavy Truck Air Suspension System with Optimal Fuzzy Control. SAE Int. J. Veh. Dyn. Stab. NVH 2020 , 4 , 179–194. [ Google Scholar ] [ CrossRef ]
- Prassad, S.G.; Malar Mohan, K. A contemporary adaptive air suspension using LQR control for passenger vehicles. ISA Trans. 2019 , 93 , 244–254. [ Google Scholar ]
- Chen, Y.; Zhang, S.; Mao, E.; Du, Y.; Chen, J.; Yang, S. Height stability control of a large sprayer body based on air suspension using the sliding mode approach. Inf. Process. Agric. 2020 , 7 , 20–29. [ Google Scholar ] [ CrossRef ]
- Ma, X.F.; Li, K. The Simulation of Air Suspension System Based on PID Control Strategy. Autmobile Appl. Technol. 2017 , 8 , 142–145. [ Google Scholar ] [ CrossRef ]
- Anh, N.T. Control an active suspension system by using PID and LQR controller. Int. J. Mech. Prod. Eng. Res. Dev. 2020 , 10 , 7003–7012. [ Google Scholar ]
- Ab Talib, M.H.; Mat Darus, I.Z.; Mohd Samin, P.; Mohd Yatim, H.; Ardani, M.I.; Shaharuddin, N.M.; Hadi, M.S. Vibration control of semi-active suspension system using PID controller with advanced firefly algorithm and particle swarm optimization. J. Ambient. Intell. Humaniz. Comput. 2021 , 12 , 1119–1137. [ Google Scholar ] [ CrossRef ]
- Tian, M.; Nguyen, V. Control performance of suspension system of cars with PID control based on 3D dynamic model. Journal of Mechanical Engineering. Autom. Control. Syst. 2020 , 1 , 1–10. [ Google Scholar ]
- Shafiei, B. A Review on PID Control System Simulation of the Active Suspension System of a Quarter Car Model While Hitting Road Bumps. J. Inst. Eng. (India) Ser. C 2022 , 103 , 1001–1011. [ Google Scholar ] [ CrossRef ]
- Han, S.Y.; Dong, J.F.; Zhou, J.; Chen, Y.H. Adaptive fuzzy PID control strategy for vehicle active suspension based on road evaluation. Electronics 2022 , 11 , 921. [ Google Scholar ] [ CrossRef ]
- Bashir, A.O.; Rui, X.; Zhang, J. Ride comfort improvement of a semi-active vehicle suspension based on hybrid fuzzy and fuzzy-PID controller. Stud. Inform. Control 2019 , 28 , 421–430. [ Google Scholar ] [ CrossRef ]
- Zhang, P.; Yue, H.; Shi, Z.; Lin, J.; Chen, Z. A fuzzy pid algorithm-based attitude control method of suspension-type small rail vehicles. J. Vib. Eng. Technol. 2022 , 10 , 111–130. [ Google Scholar ] [ CrossRef ]
- Ilesanmi, D.; Khumbulani, M.; Adefemi, A.; Kazeem, B. The use of adaptive fuzzy-PID for vibration control in the suspension system of a railcar. In Proceedings of the 2020 IEEE 11th International Conference on Mechanical and Intelligent Manufacturing Technologies (ICMIMT), Cape Town, South Africa, 20–22 January 2020; pp. 130–134. [ Google Scholar ]
- Chen, G.; Lv, S.; Dai, J. Study on PID control of vehicle semi-active suspension based on genetic algorithm. Int. J. Innov. Comput. Inf. Control 2019 , 15 , 1093–1114. [ Google Scholar ]
- Wang, Y.; Chen, K.; Dong, M. Research on active suspension system of heavy commercial vehicle controlled by PID controller based on genetic algorithm. In Advances in Precision Instruments and Optical Engineering, Proceedings of the International Conference on Precision Instruments and Optical Engineering, Guangzhou, China, 20–22 August 2021 ; Springer Nature: Singapore, 2022; pp. 555–566. [ Google Scholar ]
- Mudduluru, S.R.; Chizari, M. Quarter and full car models optimisation of passive and active suspension system using genetic algorithm. arXiv 2021 , arXiv:2101.12629. [ Google Scholar ]
- Rajamani, R. Vehicle Dynamics and Control ; Springer: New York, NY, USA, 2011. [ Google Scholar ]
- GB 7031-1987 ; Vehicle Vibration Input—Road Flatness Representation. China Standard Press: Beijing, China, 1987.
Click here to enlarge figure
Name | Notation | Value |
---|---|---|
Sprung mass | m /kg | 500 |
Unsprung mass | m /kg | 50 |
Suspension damping | c/N·s·m | 1700 |
Tire stiffness | k /N·m | 200,000 |
Parameters | Rise Time | Overshoot | Setting Time | Steady-State Error |
---|---|---|---|---|
K | Decrease | Increase | Slight Change | Decrease |
K | Decrease | Increase | Increase | Eliminate |
K | Slight Change | Decrease | Decrease | Slight Change |
e | ec | ||||||
---|---|---|---|---|---|---|---|
NB | NM | NS | ZO | PS | PM | PB | |
NB | PB | PB | PM | PM | PS | ZO | ZO |
NM | PB | PB | PM | PS | PS | ZO | NS |
NS | PM | PM | PM | PS | ZO | NS | NS |
ZO | PM | PM | PS | ZO | NS | NM | NM |
PS | PS | PS | ZO | NS | NS | NM | NM |
PM | PS | ZO | NS | NM | NM | NM | NB |
PB | ZO | ZO | NM | NM | NM | NB | NB |
e | ec | ||||||
---|---|---|---|---|---|---|---|
NB | NM | NS | ZO | PS | PM | PB | |
NB | NB | NB | NM | NM | NS | ZO | ZO |
NM | NB | NB | NM | NS | NS | ZO | ZO |
NS | NB | NM | NS | NS | ZO | PS | PS |
ZO | NM | NM | NS | ZO | PS | PM | PM |
PS | NM | NS | ZO | PS | PS | PM | PB |
PM | ZO | ZO | PS | PS | PM | PB | PB |
PB | ZO | ZO | PS | PM | PM | PB | PB |
e | ec | ||||||
---|---|---|---|---|---|---|---|
NB | NM | NS | ZO | PS | PM | PB | |
NB | PS | NS | NB | NB | NB | NM | PS |
NM | PS | NS | NB | NM | NM | NS | ZO |
NS | ZO | NS | NM | NM | NS | NS | ZO |
ZO | ZO | NS | NS | NS | NS | NS | ZO |
PS | ZO | ZO | ZO | ZO | ZO | ZO | ZO |
PM | PB | PS | PS | PS | PS | PS | PB |
PB | PB | PM | PM | PM | PS | PS | PB |
Parameters | Explanation | Parameters | Explanation |
---|---|---|---|
Encoding Method | Binary Encoding | Mutation Function | Constrained Adaptive Mutation |
Initial Population | Randomly Generate Within Specified Bounds | Crossover Probability | 0.9 |
Population Size | 30 | Mutation Function | 0.1 |
Selection Function | Random Uniform Selection | Maximum Evolution Generations | 100 |
Crossover Function | Diversified Crossover | Stopping Generation | 100 |
Evaluation Criteria | Passive Suspension | Fuzzy PID | GA-Fuzzy PID | Percentage of Fuzzy PID Optimization | Percentage of GA-Fuzzy PID Optimization |
---|---|---|---|---|---|
Vehicle Vertical Acceleration (m/s ) | 1.0473 | 0.8169 | 0.7541 | 22% | 28% |
Suspension Wheel Travel (m) | 0.0156 | 0.0129 | 0.0117 | 17% | 25% |
Dynamic Tire Load (kN) | 4.3454 | 4.1281 | 4.0412 | 5% | 7% |
Evaluation Criteria | Passive Suspension | Fuzzy PID | GA-Fuzzy PID | Percentage of Fuzzy PID Optimization | Percentage of GA-Fuzzy PID Optimization |
---|---|---|---|---|---|
Vehicle Vertical Acceleration (m/s ) | 1.8062 | 1.3908 | 1.2643 | 23% | 30% |
Suspension Wheel Travel (m) | 0.0337 | 0.0276 | 0.0249 | 18% | 26% |
Dynamic Tire Load (kN) | 6.0895 | 5.7241 | 5.5414 | 6% | 9% |
Evaluation Criteria | Passive Suspension | Fuzzy PID | GA-Fuzzy PID | Percentage of Fuzzy PID Optimization | Percentage of GA-Fuzzy PID Optimization |
---|---|---|---|---|---|
Vehicle Vertical Acceleration (m/s ) | 2.7638 | 2.0729 | 1.9070 | 25% | 31% |
Suspension Wheel Travel (m) | 0.0611 | 0.0489 | 0.0446 | 20% | 27% |
Dynamic Tire Load (kN) | 8.8378 | 8.3959 | 8.1308 | 5% | 8% |
The statements, opinions and data contained in all publications are solely those of the individual author(s) and contributor(s) and not of MDPI and/or the editor(s). MDPI and/or the editor(s) disclaim responsibility for any injury to people or property resulting from any ideas, methods, instructions or products referred to in the content. |
Share and Cite
Zhang, S.; Li, M.; Li, J.; Xu, J.; Wang, Z.; Liu, S. Research on Ride Comfort Control of Air Suspension Based on Genetic Algorithm Optimized Fuzzy PID. Appl. Sci. 2024 , 14 , 7787. https://doi.org/10.3390/app14177787
Zhang S, Li M, Li J, Xu J, Wang Z, Liu S. Research on Ride Comfort Control of Air Suspension Based on Genetic Algorithm Optimized Fuzzy PID. Applied Sciences . 2024; 14(17):7787. https://doi.org/10.3390/app14177787
Zhang, Shaobo, Mei Li, Jinsong Li, Jie Xu, Zelong Wang, and Shuaihang Liu. 2024. "Research on Ride Comfort Control of Air Suspension Based on Genetic Algorithm Optimized Fuzzy PID" Applied Sciences 14, no. 17: 7787. https://doi.org/10.3390/app14177787
Article Metrics
Article access statistics, further information, mdpi initiatives, follow mdpi.
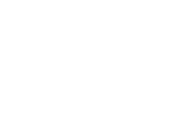
Subscribe to receive issue release notifications and newsletters from MDPI journals
A hybrid genetic algorithm with an adaptive diversity control technique for the homogeneous and heterogeneous dial-a-ride problem
- Original Research
- Published: 09 September 2024
Cite this article
- Somayeh Sohrabi 1 ,
- Koorush Ziarati ORCID: orcid.org/0000-0001-7618-6807 1 &
- Morteza Keshtkaran 1
Dial-a-Ride Problem (DARP) is one of the classic routing problems with pairing and precedence constraints. Due to these types of constraints, it is quite challenging to design an efficient evolutionary algorithm for solving this problem. In this paper, a genetic algorithm in combination with a variable neighborhood descent procedure is suggested to solve the DARP. This algorithm, which is called Hybrid Genetic Algorithm (HGA), is independent of any repairing procedure or user-defined penalty factors. Instead, it uses the constraint dominance principle with respect to the number of unserved requests. Our algorithm employs an adaptive population management technique which takes into account not only the quality of solutions but also their contribution in the diversity level. To do so efficiently, this population management technique utilizes a simple arc-based representation for the DARP solutions. A route-based crossover procedure known as Route Exchange Crossover is used in the HGA. This crossover method is thoroughly compared with five other crossover techniques including a new one called Block Exchange Crossover. The HGA produces competitive solutions in comparison with the state-of-the-art methods for tackling the DARP and Heterogeneous DARP (H-DARP). It obtains the optimal solutions of all the small and medium size standard instances of the DARP and finds new best results for two large ones with unknown optimal solutions. Moreover, for 12 out of 24 new instances of the H-DARP, the best known solutions are improved using the HGA.
This is a preview of subscription content, log in via an institution to check access.
Access this article
Subscribe and save.
- Get 10 units per month
- Download Article/Chapter or eBook
- 1 Unit = 1 Article or 1 Chapter
- Cancel anytime
Price includes VAT (Russian Federation)
Instant access to the full article PDF.
Rent this article via DeepDyve
Institutional subscriptions
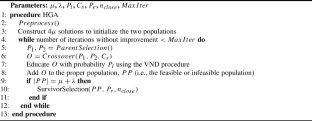
Explore related subjects
- Artificial Intelligence
Atahran, A., Lenté, C., & T’kindt, V. (2014). A multicriteria dial-a-ride problem with an ecological measure and heterogeneous vehicles. Journal of Multi-Criteria Decision Analysis, 21 , 279–298.
Article Google Scholar
Braekers, K., Caris, A., & Janssens, G. K. (2014). Exact and meta-heuristic approach for a general heterogeneous dial-a-ride problem with multiple depots. Transportation Research Part B: Methodological, 67 , 166–186.
Chassaing, M., Duhamel, C., & Lacomme, P. (2016). An ELS-based approach with dynamic probabilities management in local search for the Dial-A-Ride Problem. Engineering Applications of Artificial Intelligence, 2 (48), 119–133.
Chevrier, R., Liefooghe, A., Jourdan, L., & Dhaenens, C. (2012). Solving a dial-a-ride problem with a hybrid evolutionary multi-objective approach: Application to demand responsive transport. Applied Soft Computing Journal, 4 (12), 1247–1258.
Cordeau, J. F. (2006). A branch-and-cut algorithm for the dial-a-ride problem. Operations Research, 5 (54), 573–586.
Cordeau, J. F., & Laporte, G. (2003). The Dial-a-Ride Problem (DARP): Variants, modeling issues and algorithms. Quarterly Journal of the Belgian, French and Italian Operations Research Societies, 1 , 89–101.
Google Scholar
Cordeau, J. F., & Laporte, G. (2003). A tabu search heuristic for the static multi-vehicle dial-a-ride problem. Transportation Research Part B: Methodological, 37 , 579–594.
Cordeau, J. F., & Laporte, G. (2007). The dial-a-ride problem: Models and algorithms. Annals of Operations Research, 9 (153), 29–46.
Deb, K. (2000). An efficient constraint handling method for genetic algorithms. Computers Methods in Applied Mechanics and Engineering, 186 , 311–338.
Garcia-Najera, A., & Bullinaria, J. A. (2011). An improved multi-objective evolutionary algorithm for the vehicle routing problem with time windows (Vol. 38, pp. 287–300). Elsevier Ltd.
Gschwind, T., & Drexl, M. (2019). Adaptive large neighborhood search with a constant-time feasibility test for the dial-a-ride problem. Transportation Science, 3 (53), 480–491.
Ho, S. C., Szeto, W. Y., Kuo, Y. H., Leung, J. M. Y., Petering, M., & Tou, T. W. H. (2018). A survey of dial-a-ride problems: Literature review and recent developments. Transportation Research Part B: Methodological, 5 (111), 395–421.
Hunsaker, B., & Savelsbergh, M. (2002). Operations research letters EEcient feasibility testing for dial-a-ride problems. Operations Research Letters, 30 , 169–173.
Jorgensen, R. M., Larsen, J., & Bergvinsdottir, K. B. (2007). Solving the dial-a-ride problem using genetic algorithms. Journal of the Operational Research Society, 58 , 1321–1331.
Jozefowiez, N., Semet, F., & Talbi, E. G. (2009). An evolutionary algorithm for the vehicle routing problem with route balancing. European Journal of Operational Research, 6 (195), 761–769.
Karakatič, S., & Podgorelec, V. (2015). A survey of genetic algorithms for solving multi depot vehicle routing problem. Applied Soft Computing, 27 , 519–532.
Malheiros, I., Ramalho, R., Passeti, B., Bulhões, T., & Subramanian, A. (2021). A hybrid algorithm for the multi-depot heterogeneous dial-a-ride problem. Computers and Operations Research, 5 , 129.
Masmoudi, M. A., Braekers, K., Masmoudi, M., & Dammak, A. (2017). A hybrid genetic algorithm for the heterogeneous dial-A-ride problem. Computers and Operations Research, 5 (81), 1–13.
Mendoza, J. E., Castanier, B., Guéret, C., Medaglia, A. L., & Velasco, N. (2010). A memetic algorithm for the multi-compartment vehicle routing problem with stochastic demands. Computers and Operations Research, 11 (37), 1886–1898.
Molenbruch, Y., Braekers, K., & Caris, A. (2017). Typology and literature review for dial-a-ride problems. Annals of Operations Research, 12 (259), 295–325.
Mourad, A., Puchinger, J., & Chu, C. (2019). A survey of models and algorithms for optimizing shared mobility. Transportation Research Part B: Methodological, 5 (123), 323–346.
Núñez, A., Cortés, C. E., Sáez, D., Schutter, B. D., & Gendreau, M. (2014). Multiobjective model predictive control for dynamic pickup and delivery problems. Control Engineering Practice, 32 , 73–86.
Ombuki, B., Ross, B. J., & Hanshar, F. (2006). Multi-objective genetic algorithms for vehicle routing problem with time windows. Applied Intelligence, 24 , 17–30.
Parragh, S. N. (2011). Introducing heterogeneous users and vehicles into models and algorithms for the dial-a-ride problem. Transportation Research Part C: Emerging Technologies, 19 , 912–930.
Parragh, S. N., Doerner, K. F., & Hartl, R. F. (2010). Variable neighborhood search for the dial-a-ride problem. Computers and Operations Research, 6 (37), 1129–1138.
Parragh, S. N., & Schmid, V. (2013). Hybrid column generation and large neighborhood search for the dial-a-ride problem. Computers and Operations Research, 1 (40), 490–497.
Pereira, F. B., Tavares, J., Machado, P., & Costa, E. (2002). GVR: A new genetic representation for the vehicle routing problem. In M. O’Neill, R. F. E. Sutcliffe, C. Ryan, M. Eaton, & N. J. L. Griffith (Eds.), Artificial intelligence and cognitive science berlin (pp. 95–102). Springer.
Chapter Google Scholar
Potvin, J. Y., & Bengio, S. (1996). The vehicle routing problem with time windows part II: Genetic search. INFORMS Journal on Computing, 8 , 165–172.
Rahimi, I., Gandomi, A. H., Chen, F., & Mezura-Montes, E. (2023). A review on constraint handling techniques for population-based algorithms: From single-objective to multi-objective optimization. Archives of Computational Methods in Engineering, 4 (30), 2181–2209.
Ropke, S., Cordeau, J. F., & Laporte, G. (2007). Models and branch-and-cut algorithms for pickup and delivery problems with time windows. Networks: An International Journal, 49 , 258–272.
Ropke, S., & Pisinger, D. (2006). An adaptive large neighborhood search heuristic for the pickup and delivery problem with time windows. Transportation Science, 40 , 455–472.
Tan, K. C., Chew, Y. H., & Lee, L. H. (2006). A hybrid multiobjective evolutionary algorithm for solving vehicle routing problem with time windows. Computational Optimization and Applications, 5 (34), 115–151.
Toth, P., & Vigo, D. (2014). Vehicle routing . Society for Industrial and Applied Mathematics.
Book Google Scholar
Vidal, T. (2022). Hybrid genetic search for the CVRP: Open-source implementation and SWAP* neighborhood. Computers and Operations Research, 140 , 105643.
Vidal, T., Crainic, T. G., Gendreau, M., Lahrichi, N., & Rei, W. (2012). A hybrid genetic algorithm for multidepot and periodic vehicle routing problems. Operations Research, 5 (60), 611–624.
Vidal, T., Crainic, T. G., Gendreau, M., & Prins, C. (2013). A hybrid genetic algorithm with adaptive diversity management for a large class of vehicle routing problems with time-windows. Computers and Operations Research, 1 (40), 475–489.
Zhang, Z., Liu, M., & Lim, A. (2015). A memetic algorithm for the patient transportation problem. Omega, 7 (54), 60–71.
Download references
Acknowledgements
The authors would like to sincerely thank Professor Kris Braekers for sharing the code of the DA. Besides, we are extremely grateful to Professor Anand Subramanian and his colleagues for executing the HILS code considering the new H-DARP instances.
Author information
Authors and affiliations.
Department of Computer Science, Engineering and Information Technology, Shiraz University, Shiraz, Iran
Somayeh Sohrabi, Koorush Ziarati & Morteza Keshtkaran
You can also search for this author in PubMed Google Scholar
Corresponding author
Correspondence to Koorush Ziarati .
Additional information
Publisher's note.
Springer Nature remains neutral with regard to jurisdictional claims in published maps and institutional affiliations.
Supplementary Information
Below is the link to the electronic supplementary material.
Supplementary file 1 (pdf 88 KB)
Rights and permissions.
Springer Nature or its licensor (e.g. a society or other partner) holds exclusive rights to this article under a publishing agreement with the author(s) or other rightsholder(s); author self-archiving of the accepted manuscript version of this article is solely governed by the terms of such publishing agreement and applicable law.
Reprints and permissions
About this article
Sohrabi, S., Ziarati, K. & Keshtkaran, M. A hybrid genetic algorithm with an adaptive diversity control technique for the homogeneous and heterogeneous dial-a-ride problem. Ann Oper Res (2024). https://doi.org/10.1007/s10479-024-06194-z
Download citation
Received : 04 November 2023
Accepted : 26 July 2024
Published : 09 September 2024
DOI : https://doi.org/10.1007/s10479-024-06194-z
Share this article
Anyone you share the following link with will be able to read this content:
Sorry, a shareable link is not currently available for this article.
Provided by the Springer Nature SharedIt content-sharing initiative
- On-demand deliveries
- Shared mobility systems
- Pickup and delivery problem (PDP)
- Dial-a-ride problem (DARP)
- Genetic algorithm
- Population management
- Find a journal
- Publish with us
- Track your research
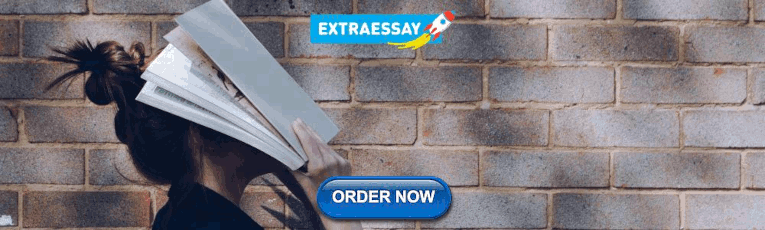
IMAGES
VIDEO
COMMENTS
The research work related to genetic algorithm for multimedia applications were also included. During the screening of research papers, all the duplicate papers and papers published before 2007 were discarded. 4340 research papers were selected based on 2007 and duplicate entries. Thereafter, 4050 research papers were eliminated based on titles ...
A genetic algorithm (GA) is an evolutionary algorithm inspired by the natural selection and biological processes of reproduction of the fittest individual. GA is one of the most popular optimization algorithms that is currently employed in a wide range of real applications. Initially, the GA fills the population with random candidate solutions and develops the optimal solution from one ...
The genetic algorithms of great interest in research community are selected for analysis. This review will help the new and demanding researchers to provide the wider vision of genetic algorithms. The well-known algorithms and their implementation are presented with their pros and cons. The genetic operators and their usages are discussed with ...
Paper— Genetic Algorithm: Reviews, Implementation and Applications Keywords— Genetic Algorithm, Search Techniques, Random Tests, Evolution, Applications. 1 Introduction The GA is a meta-heuristic motivated by the evolution process and belongs to the large class of evolutionary algorithms in informatics and computational mathematics.
The research work related to genetic algorithm for multimedia applications were also included. During the screening of research papers, all the duplicate papers and papers published before 2007 were discarded. 4340 research papers were selected based on 2007 and duplicate entries. Thereafter, 4050 research papers were eliminated based on titles ...
2.1 Genetic algorithm. Genetic Algorithm is a series of simulation evolutionary algorithms proposed by Holland et al. [], and later summarized by DeJong, Goldberg and others.The general flowchart of the Genetic Algorithm is shown in Fig 1.The Genetic Algorithm first encodes the problem, then calculates the fitness, then selects the parent and the mother by roulette, and finally generates the ...
Genetic Algorithm (GA) may be attributed as method for optimizing the search tool for difficult problems based on genetics selection principle. In additions to Optimization it also serves the purpose of machine learning and for Research and development. It is analogous to biology for chromosome generation with variables such as selection, crossover and mutation together constituting genetic ...
An intelligent bionic algorithm with great global optimization potential, the genetic algorithm evolved in a manner analogous to the natural process of genetic evolution in living creatures. This paper first explains the foundation of genetic algorithms, which is based on Darwin's "survival of the fittest" principle, then outlining the algorithm's primary features and briefly discussing ...
The 2006 NASA ST5 spacecraft antenna. This complicated shape was found by an evolutionary computer design program to create the best radiation pattern. It is known as an evolved antenna.. In computer science and operations research, a genetic algorithm (GA) is a metaheuristic inspired by the process of natural selection that belongs to the larger class of evolutionary algorithms (EA).
A genetic algorithm (GA) is an evolutionary algorithm inspired by the natural selection and biological processes of reproduction of the fittest individual. GA is one of the most popular ...
Nowadays genetic algorithm (GA) is greatly used in engineering pedagogy as an adaptive technique to learn and solve complex problems and issues. It is a meta-heuristic approach that is used to solve hybrid computation challenges. GA utilizes selection, crossover, and mutation operators to effectively manage the searching system strategy. This algorithm is derived from natural selection and ...
The genetic algorithms of great interest in research community are selected for analysis. This review will help the new and demanding researchers to provide the wider vision of genetic algorithms.
Genetic algorithms have been used in science and engineering as adaptive algorithms for solving practical problems and as computational models of natural evolutionary systems. This brief, accessible introduction describes some of the most interesting research in the field and also enables readers to implement and experiment with genetic ...
A great wealth of information is hidden amid medical research data that in some cases cannot be easily analyzed, if at all, using classical statistical methods. ... we introduce the genetic algorithm and its applications in medicine. The use of the genetic algorithm has promising implications in various medical specialties including radiology ...
The natural selection theory is the foundation of the genetic algorithms, which are crucial in solving such complex issues. Numerous problems are optimized in the literature using genetic algorithms. ... This review's objective is to outline the current course of human genetic research in light of developments in phenome-wide research, a ...
The research work related to genetic algorithm for multimedia applications were also included. During the screening of research papers, all the duplicate papers and papers published before 2007 were discarded. 4340 research papers were selected based on 2007 and duplicate entries. Thereafter, 4050 research papers were eliminated based on titles ...
The site is a long-established resource for the genetic algorithm and evolutionary computation communities and contains lists of research groups, downloadable software and links to related sites of interest. The Archive also maintains an archive of postings to the EC Digest mailing list (formerly GA-List).
A genetic algorithm is a special type of evolutionary algorithm that uses evolutionary biology techniques such as heredity, mutation biology, and Darwin's principles of choice to find the optimal formula for predicting or matching the pattern. ... Hemangi Oza, in Petroleum Research, 2021. 2.3 Genetic algorithm. Genetic Algorithm (GA) is an ...
Genetic algorithms are extremely popular methods for solving optimization problems. They are a population-based method that combine solutions to produce offspring using operators including crossover and mutation. ... Parallelization is a less frequently research topic now as computing power has increased significantly, but for some problems ...
Genetic algorithms are computational models of evolution that play a central role in many artificial life models. We review the history and current scope of research on genetic algorithms in artificial life, using illustrative examples in which the genetic algorithms is used to study how learning and evolution interact, and to model ecosystems, immune system, cognitive systems, and social systems.
Thus, genetic algorithms have been used to solve myriads of real-world optimization problems and are an essential subject of research in optimization and related fields. They are known for their multidisciplinary usage across disciplines such as natural, social, and earth sciences, finance, or economics.
GA is a powerful population-based search metaheuristic algorithm. It is inspired by evolution and its concepts such as reproduction and survival of the fittest. In this explanation, I covered how GA is applied to continuous optimization problems where the chromosomes are represented (encoded) with 0s and 1s.
However, such synergistic approaches are still an incipient area of research and appear underexplored in the literature. This perspective covers different ways of incorporating machine learning approaches into evolutionary learning frameworks, with the overall goal of increasing the optimization efficiency of genetic algorithms.
Genetic algorithm was employed for computation via MATLAB with the following parameters: population size was 20, evolution times was 500, and algorithm tolerance is 10 -6. The fitness evolution ...
Genetic Algorithms. Genetic Algorithms (GAs) are adaptive heuristic search algorithms that belong to the larger part of evolutionary algorithms. Genetic algorithms are based on the ideas of natural selection and genetics. These are intelligent exploitation of random searches provided with historical data to direct the search into the region of ...
We propose a novel deep learning-based approach called the genetic algorithm with neural cost predictor to tackle the challenge and simplify algorithm developments. For each higher-level decision candidate, we predict the objective function values of the underlying vehicle routing problems using a pretrained graph neural network without ...
Genetic algorithm is introduced to perform reactive power optimization calculation on distribution network to improve the performance and efficiency of the system. Finally, the model optimization is verified, and the maximum loss of the system is reduced by 24.69kW after accessing the model compensation, which is a relative reduction of 51.38% ...
This strategy involves a genetic algorithm-optimized fuzzy PID air suspension control approach specifically targeting the fuzzy PID controller for air suspension. A 1/4 two-degree-of-freedom air suspension fuzzy PID controller is designed in MATLAB 2019a, utilizing genetic algorithms to optimize the PID parameter tuning process.
A hybrid genetic algorithm for multidepot and periodic vehicle routing problems. Operations Research, 5(60), 611-624. Article Google Scholar Vidal, T., Crainic, T. G., Gendreau, M., & Prins, C. (2013). A hybrid genetic algorithm with adaptive diversity management for a large class of vehicle routing problems with time-windows.