It indicates the null hypothesis is very unlikely. | | It indicates the null hypothesis is very likely. | 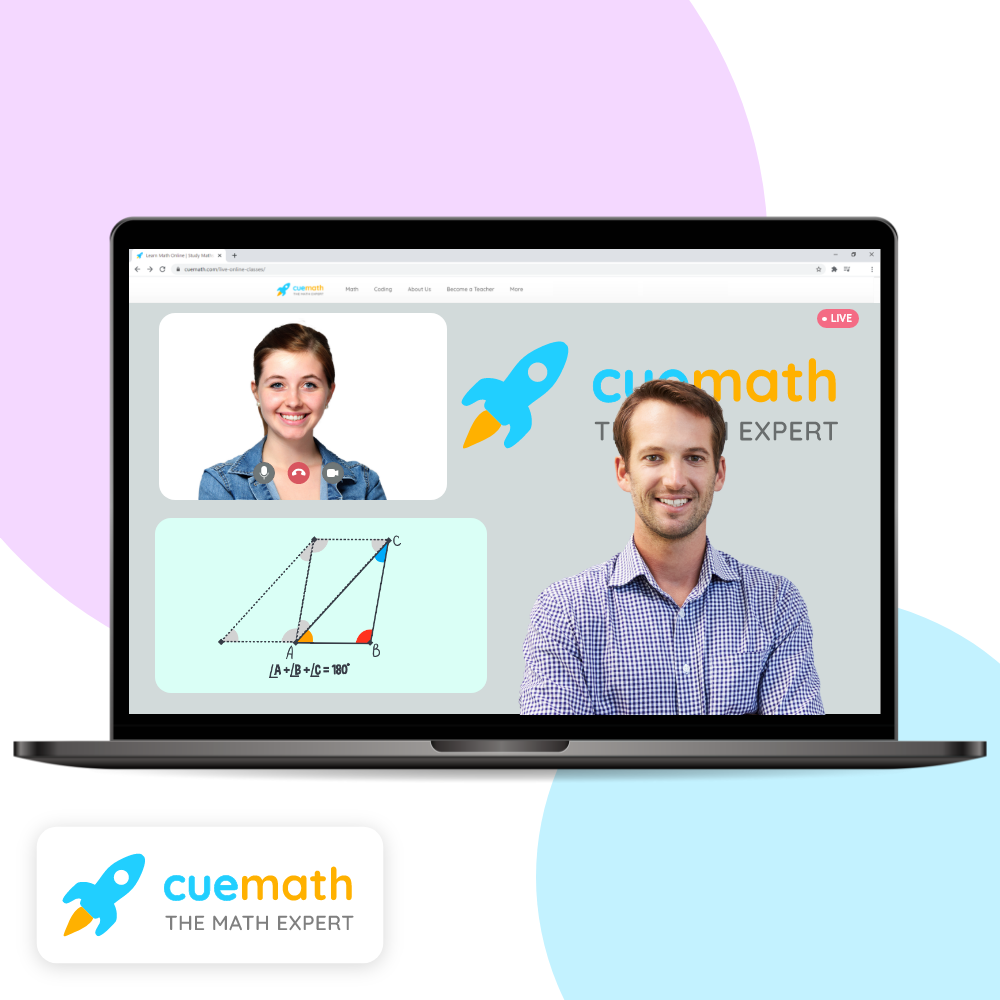 Book a Free Trial Class Examples Using P-value Formula Example 1: A statistician is testing the hypothesis H0: μ = 120 using the approach of alternative hypothesis Hα: μ > 120 and assuming that α = 0.05. The sample values that he took are as n =40, σ = 32.17 and x̄ = 105.37. What is the conclusion for this hypothesis? We know that, \(\sigma_{\bar{x}}=\dfrac{\sigma}{\sqrt{n}}\) Now substitute the given values \(\sigma_{\bar{x}}=\dfrac{32.17}{\sqrt{40}}=5.0865\) As per the test static formula, we get t = (105.37 – 120) / 5.0865 Therefore, t = -2.8762 Using the Z-Score table, finding the value of P(t > -2.8762) P (t < -2.8762) = P(t > 2.8762) = 0.003 If P(t > -2.8762) =1 - 0.003 =0.997 P- value =0.997 > 0.05 As the value of p > 0.05, the null hypothesis is accepted. Therefore, the null hypothesis is accepted. Example 2: P-value is 0.3105. If the level of significance is 5%, find if we can reject the null hypothesis. Solution: Looking at the P-value table, the p-value of 0.3105 is greater than the level of significance of 0.05 (5%), we fail to reject the null hypothesis. Example 3: P-value is 0.0219. If the level of significance is 5%, find if we can reject the null hypothesis. Solution: Looking at the P-value table, the p-value of 0.0219 is less than the level of significance of 0.05, we reject the null hypothesis. FAQs on P-value Formula What is meant by p-value formula. The P-value formula is short for probability value. P-value defines the probability of getting a result that is either the same or more extreme than the other actual observations. The P-value represents the probability of occurrence of the given event. The formula to calculate the p-value is: \(Z = \frac{\hat{p}-p 0}{\sqrt{\frac{p 0(1-p 0)}{n}}}\) What is the Formula to Calculate the P-value?What is the p-value formula table . The P-value formula table is: Using the P-value Formula Table, Check if the Hypothesis is Rejected or not when the P-value is 0.354 with 5% Level of Significance.Looking at the table, the p-value of 0.354 is greater than the level of significance of 0.05 (5%), we fail to reject the null hypothesis. - Skip to secondary menu
- Skip to main content
- Skip to primary sidebar
Statistics By Jim Making statistics intuitive P-Values, Error Rates, and False PositivesBy Jim Frost 41 Comments In my post about how to interpret p-values , I emphasize that p-values are not an error rate. The number one misinterpretation of p-values is that they are the probability of the null hypothesis being correct. The correct interpretation is that p-values indicate the probability of observing your sample data, or more extreme, when you assume the null hypothesis is true. If you don’t solidly grasp that correct interpretation, please take a moment to read that post first. Hopefully, that’s clear. Unfortunately, one part of that blog post confuses some readers. In that post, I explain how p-values are not a probability, or error rate, of a hypothesis. I then show how that misinterpretation is dangerous because it overstates the evidence against the null hypothesis.  The logical question is, if p-values aren’t an error rate, how can you report those higher false positive rates (an error rate)? That’s a reasonable question and it’s the topic of this post! A Quick Note about This PostThis post might be a bit of a mind-bender. P-values are already confusing! And in this post, we look at p-values differently using a different branch of statistics and methodology. I’ve hesitated writing this post because it feels like a deep, dark rabbit hole! However, the ideas from this exploration of p-values have strongly influenced how I view and use p-values. While I’m writing this post after other posts and an entire book chapter about p-values, the line of reasoning I present here strongly influenced how I wrote that earlier content. Buckle up! Frequentist StatisticsBefore calculating the false positive rate, you need to understand frequentist statistics, also known as frequentist inference. Frequentist statistics are what you learned, or are learning, in your Introduction to Statistics course. This methodology is a type of inferential statistics containing the familiar hypothesis testing framework where you compare your p-values to the significance level to determine statistical significance. It also includes using confidence intervals to estimate effects. Frequentist inference focuses on frequencies that make it possible to use samples to draw conclusions about entire populations. The frequencies in question are the sampling distributions of test statistics. That goes beyond the scope of this post but click the related posts links below for the details. Frequentist methodology treats population parameters , such as the population mean (µ), as fixed but unknown characteristics. There are no probabilities associated with them. The null and alternative hypotheses are statements about population parameters. Consequently, frequentists can’t say that there is such and such probability that the null hypothesis is correct. It either is correct or incorrect, but you don’t know the answer. The relevant point here is that when you stick strictly to frequentist statistics, there is no way to calculate the probability that a hypothesis is correct. Related posts : How Hypothesis Tests Work , How t-Tests Work , How F-tests Work in ANOVA , and How the Chi-Squared Test of Independence Works Why Can’t Frequentists Calculate those Probabilities?There are mathematical reasons for that but let’s look at it intuitively. In frequentist inference, you take a single, random sample and draw conclusions about the population. The procedure does not use other information from the outside world or other studies. It’s all based on that single sample with no broader context. In that setting, it’s just not possible to know the probability that a hypothesis is correct without incorporating other information. There’s no way to tell whether your sample is unusual or representative. Frequentist methods have no way to include such information and, therefore, cannot calculate the probability that a hypothesis is correct. However, Bayesian statistics and simulation studies include additional information. Those are large areas of study, so I’ll only discuss the points relevant to our discussion. Bayesian StatisticsBayesian statistics can incorporate an entire framework of evidence that resides outside the sample. Does the overall fact pattern support a particular hypothesis? Does the larger picture indicate that a hypothesis is more likely to be correct before starting your study? This additional information helps you calculate probabilities for a hypothesis because it’s not limited to a single sample. Simulation StudiesWhen you perform a study in the real world, you do it just once. However, simulation studies allow statisticians to perform simulated studies thousands of times while changing the conditions. Importantly, you know the correct results, enabling you to calculate error rates, such as the false positive rate. Using frequentist methods, you can’t calculate error rates for hypotheses. There is no way to take a p-value and convert it to an error rate. It’s just not possible with the math behind frequentist statistics. However, by incorporating Bayesian and simulation methods, we can estimate error rates for p-values. Simulation Studies and False PositivesIn my post about interpreting p-values, I quote the results from Sellke et al. He used a Bayesian approach. But let’s start with simulation studies and see how they can help us understand the false positive rate. For this, we’ll look at the work of David Colquhoun, a professor in biostatistics, who lays it out here . Factors that influence the false-positive rate include the following: - Prevalence of real effects (higher is good)
- Power (higher is good)
- Significance level (lower is good)
“Good” indicates the conditions under which hypothesis tests are less likely to produce false positives. Click the links to learn more about each concept. The prevalence of real effects indicates the probability that an effect exists in the population before conducting your study. More on that later! Let’s see how to calculate the false positive rate for a particular set of conditions. Our scenario uses the following conditions: - Prevalence of real effects = 0.1
- Significance level (alpha) = 0.05
- Power = 80%
We’ll “perform” 1000 hypothesis tests under these conditions.  In this scenario, the total number of positive test results are 45 + 80 = 125. However, 45 of those positives are false. Consequently, the false positive rate is:  Mathematically, calculate the false positive rate using the following:  Where alpha is your significance level and P(real) is the prevalence of real effects. Simulation studies for P-valuesThe previous example and calculation incorporate the significance level to derive the false positive rate. However, we’re interested in p-values. That’s were the simulation studies come in! Using simulation methodology, Colquhoun runs studies many times and sets the values of the parameters above. He then focuses on the simulated studies that produce p-values between 0.045 and 0.05 and evaluates how many are false positives. For these studies, he estimates a false positive rate of at least 26%. The 26% error rate assumes the prevalence of real effects is 0.5, and power is 80%. Decreasing the prevalence to 0.1 causes the false positive rate to jump to 76%. Yikes! Let’s examine the prevalence of real effects more closely. As you saw, it can dramatically influence the error rate! P-Values and the Bayesian Prior ProbabilityThe property that Colquhoun names the prevalence of real effects (P(real)) is what the Bayesian approach refers to as the prior probability. It is the proportion of studies where a similar effect is present. In other words, the alternative hypothesis is correct. The researchers don’t know this, of course, but sometimes you have an idea. You can think of it as the plausibility of the alternative hypothesis. When your alternative hypothesis is implausible, or similar studies have rarely found an effect, the prior probability (P(real)) is low. For instance, a prevalence of 0.1 signifies that 10% of comparable alternative hypotheses were correct, while 90% of the null hypotheses were accurate (1 – 0.1 = 0.9). In this case, the alternative hypothesis is unusual, untested, or otherwise unlikely to be correct. When your alternative hypothesis is consistent with current theory, has a recognized process for producing the effect, or prior studies have already found significant results, the prior probability is higher. For instance, a prevalence of 0.90 suggests that the alternative is correct 90% of the time, while the null is right only 10% of the time. Your alternative hypothesis is plausible. When the prior probability is 0.5, you have a 50/50 chance that either the null or alternative hypothesis is correct at the beginning of the study. You never know this prior probability for sure, but theory, previous studies, and other information can give you clues. For this blog post, I’ll assess prior probabilities to see how they impact our interpretation of P values. Specifically, I’ll focus on the likelihood that the null hypothesis is correct (1 – P(real)) at the start of the study. When you have a high probability that the null is right, your alternative hypothesis is unlikely. Moving from the Prior Probability to the Posterior ProbabilityFrom a Bayesian perspective, studies begin with varying probabilities that the null hypothesis is correct, depending on the alternative hypothesis’s plausibility. This prior probability affects the likelihood the null is valid at the end of the study, the posterior probability. If P(real) = 0.9, there is only a 10% probability that the null is correct at the start. Therefore, the chance that the hypothesis test rejects a true null at the end of the study cannot be greater than 10%. However, if the study begins with a 90% probability that the null is right, the likelihood of rejecting a true null escalates because there are more true nulls. The following table uses Colquhoun and Sellke et al.’s calculations . Lower prior probabilities are associated with lower posterior probabilities. Additionally, notice how the likelihood that the null is correct decreases from the prior probability to the posterior probability. The precise value of the p-value affects the size of that decrease. Smaller p-values cause a larger decline. Finally, the posterior probability is also the false positive rate in this context because of the following: - the low p-values cause the hypothesis test to reject the null.
- the posterior probability indicates the likelihood that the null is correct even though the hypothesis test rejected it.
| | | 0.5 | 0.05 | 0.289 | 0.5 | 0.01 | 0.110 | 0.5 | 0.001 | 0.018 | 0.33 | 0.05 | 0.12 | 0.9 | 0.05 | 0.76 | Safely Using P-valuesMany combinations of factors affect the likelihood of rejecting a true null. Don’t try to remember these combinations and false-positive rates. When conducting a study, you probably will have only a vague sense of the prior probability that your null is true! Or maybe no sense of that probability at all! Just keep these two big takeaways in mind: - A single study that produces statistically significant test results can provide weak evidence that the null is false, especially when the P value is close to 0.05.
- Different studies can produce the same p-value but have vastly different false-positive rates. You need to understand the plausibility of the alternative hypothesis.
Carl Sagan’s quote embodies the second point, “Extraordinary claims require extraordinary evidence.” Suppose a new study has surprising results that astound scientists. It even has a significant p-value! Don’t trust the alternative hypothesis until another study replicates the results! As the last row of the table shows, a study with an implausible alternative hypothesis and a significant p-value can still have an error rate of 76%! I can hear some of you wondering. Ok, both Bayesian methodology and simulation studies support these points about p-values. But what about empirical research? Does this happen in the real world? A study that looks at the reproducibility of results from real experiments supports it all. Read my post about p-values and the reproducibility of experimental results . I know this post might make p-values seem more confusing. But don’t worry! I have another post that provides simple recommendations to help you navigate P values. Read my post: Five P-value Tips to Avoid Being Fooled by False Positives . Share this: Reader Interactions July 26, 2024 at 9:47 am Hi Jim, thank you for this very important work of explanation. I have fallen into the rabbit hole of the relationship between p-values and error rates because of some literature review I have been doing in sports science. In this field, researchers often use ANOVA to compare the effect of different training regimens on certain physical ability metrics such as endurance. To test endurance, they come up with tests for which they often don’t evaluate the test-retest reliability. My initial inquiry was: how often can an ANOVA incorrectly detect a difference with p <= 0.05 as a function of test-retest reliabiliyt (measured using an ICC), in other words, how is the error rate affected by measurement (un)reliability? I ended up finding a paper by Westfall and Yarkoni (2016) on the effect of reliability on controlling for confounding variables, but I don't think this translates to my inquiry. That is how I ended up reading your blog posts on p-values which have been very illuminating. However I believe the work you shared doesn't take into account measurement reliability. Would you happen to have some thoughts or references to share on the impact of measurement reliability on the rate of false positives (type I error rate) in ANOVA? Thank you very much.  August 1, 2024 at 7:56 pm All hypothesis tests, including ANOVA, assume that measurement error is small compared to the sampling error. If you can’t make that assumption, it raises questions about the results. Hypothesis testing does not account for measurement error, just sampling error. I don’t know of a way to factor in measurement error to the results. It’s not standard practice. Ideally, the researchers would have conducted an assessment of their measurements to make that determination. Unfortunately, I don’t have references on hand. But, if you have concerns about the data’s reliability, that is potentially a legitimate problem and I’d encourage you to look into it more. Sorry I can’t be more helpful with a reference though.  June 12, 2022 at 2:55 am Yes but I am not looking for the error rate after the simulation is done. I need a way to control the error rate before the algorithm run. And intuitively there must be a way to do it with a threshold p Value on which you base the decision. The lower the pValue threshold, the better for error rate. I am looking for a way to calculate the function that retrieve error rate from this “beforehand chosen pValue threshold”. The only way I can think for now is to run the simulation with different critical values, observe error rate, and interpolate points to get a continuous function. So I was hoping that you had a better idea.  June 9, 2022 at 2:57 am Thanks for this blog. I am not a mathematician, just a computer scientist. Thus I may misunderstand but you seem to say that we cant compute error rate from value. My problem is as follow. I have a set of inputs that follow random distributions. By design, all the distribution are equals except for one that is have a bigger mean (very litle difference). All have same variance. I try to find the quickest way (in amount of try) to isolate this particular input with user given probability x. One of my approach is based on critical pvalues over the difference of the best set of data compared with all the others. I stop when the difference reach a predefined pValue. I was really surprised by the difference between error rate and pvalue observe: pvalue = 0.0025 => 0.14 error rate. This is why I came here to try to understand. It’s clear thanks to you now that this is to be expected, but I still cant grasp that there is no way to link the two values when you control every parameter. Since I am doing simulation, I control every parameter. The prevalence of effect is one. This is really bogging me (I find it counterintuitive), that I cant control x with pValues, but I can using an other interval of trust technique. Specially because the pvalue method go a bit faster: pvalues: (x = 0.859: numberOfTry=127.881) intérvals: (x=0.864, numberOfTry=134.649 So my question is: Is there really nothing to do to anticipate error rate from critical pValue for my specific use case? Do you have recommendation on the best way to resolve my problem ? Ps: the interval technique finish when both sets of data interval and all the other data interval become disjoint. June 12, 2022 at 1:07 am Please understand that when I say you can’t link p-values to error rates, I’m referring to real studies using real data. In those cases, you only get one sample and you don’t know (and can’t control) the population parameters. However, when you’re performing simulation studies, you certainly do control the population parameters and can draw repeated samples from the populations as you define them. In those cases, yes, you can certainly know the error rates because you know all the necessary information. However, in real world studies, you don’t have all that necessary info. That’s a huge difference!  March 3, 2022 at 12:40 pm Thanks again, Jim. I have 3 comments: First, you said that the probabilities of the following four events do not sum to 1. I think they DO sum to 1 — it is just that two of them will have probability zero, because, as you said, either the null is true or it is false. So, point taken. 1. Reject a true null hypothesis. 2. Reject a false null hypothesis. 3. Fail to reject a true null hypothesis. 4. Fail to reject a false null hypothesis. Second, I guess I still don’t understand the definition of a Type I error rate, if you say it is hard to determine. I completely understand that the error rate is not equal to the P-value, even though it is in fact a probability — but how is that probability defined? Given what you have written, I don’t see how it is different than alpha. Finally, I was talking about these ideas with a friend, and he referred me to this interesting article. Evidently I am not alone in thinking that Type I errors don’t occur. See page 1000. https://www.sjsu.edu/faculty/gerstman/misc/Cohen1994.pdf The author makes a point that I had never seen before. We are all familiar with this logic: If A, then B; it follows that if B isn’t true, we assume A isn’t true. In our context, ff the null is true, we won’t get this data; we got this data, so the null is false. He then points out how this isn’t quite right, and it is more accurate to say: If the null is true, we probably don’t get this data. We then conclude that if we got this data, the null is probably false. But this is very bad logic, as shown in this example: If a person is an American, he is probably not a member of Congress. Since this person is a member of Congress, he is probably not an American. Such logic falls into the same trap of thinking that Prob(getting this sample data, given that the null is true) is equal to Prob(the null is true, given this sample data). March 3, 2022 at 2:10 pm We’re getting to the point where we’re going around in circles a bit. If you have questions after this reply, please use my contact me form. I’ll try not to be too repetitive below because I’ve addressed several of these points already. I suppose you could say that all four should sum to 1. However, only two of them will be valid for any given test. In my list below, only 1 & 2 or 3 & 4 will be valid possibilities for a given test. And, again, you should be listing them in a logical order like the following where you correctly group complementary pairs. The order you use doesn’t emphasize the natural pairings. 1. Reject a true null: error rate = α 2. Failing to reject a true null: correct decision rate = 1 – α. 3. Failing to reject a false null: error rate = β 4. Reject a false null: correct decision rate = 1 – β (aka statistical power) While you could say the invalid pair has a probability that sums to zero, it doesn’t really make sense to consider, say, the probability of rejecting a true null for a test where the null is false. Of course, you don’t know the answer to that, but in theory that’s the case. But, if you want to consider one pair to have a probability of zero and the other pair to have a probability of 1, I suppose that works. Maybe it even clarifies how one pair is invalid. I focus on the interpretation of p-values . Click the link to read. I specifically cover what the probability represents. And read the following for graphical comparison between significance levels and p-values . I’ve already covered in detail in my previous replies why it’s not a problem if type I errors don’t exist. I have heard of this thinking before, but I don’t buy it personally. It’s easy enough to imagine a totally ineffective treatment where both populations are by definition the same. But, even if you assume that there is always some minimal effect, it’s not a problem for all the reasons I explained before. Then it just becomes a case of having a large enough sample size to detect meaningful effects and to produce a sufficiently precise confidence interval. That’s already built into the power analysis process. So, even if you’re right, it’s a not a problem. I do want to address your logic example. I actually addressed this idea in a previous reply. Yes, that is bad logic. And hypothesis testing specifically addresses that. That’s why when your results are not significant, we say that you “fail to reject the null.” You are NOT accepting the null. A non-significant hypothesis test isn’t proving that there is no effect (i.e., not proving the null is true). Instead, it’s saying that you have insufficient evidence to conclude that an effect exists in the population. Similar to your logic example, that is NOT the same as saying there is no effect. I’ve written a post about that topic exactly. I included in a previous reply, and I suggest you read it this time! 🙂 Failing to Reject the Null Hypothesis . February 25, 2022 at 12:07 pm Wait, one more post. Perhaps I just had an epiphany. By the error rate of “rejecting a true null”, do you mean the probability that the null is true, given that we rejected it? And this is what can be has high as 0.23 when P = .05 ? This is in contrast to the probability of a Type I error, alpha, which is the probability of rejecting a null, given that it is true? If this is what is meant, then my confusion is removed, and it explains why the error rate and alpha are not equal — they are different conditional probabilities. Of course these two probabilities are related to each other via Baye’s Theorem. By the way, if this is correct, then I change my initial objection from Type I errors hardly ever occurring to claim that the error rate is almost always 0, since the null is hardly ever true, unless we have some error tolerance built into the statement of the null :). March 1, 2022 at 1:16 am Type I errors can only occur when the null is true by definition. You’re rejecting a null that is true. That’s an error and can, obviously, only occur when the null is true. When the null is false, you can’t reject it incorrectly. The p-value error rate is also the same idea. You can only incorrectly reject the null when the null is true. So, yes, both cases are conditional on a true null. You can’t incorrectly reject a false null. As I write in my other reply, The type I error rate equals the significance level and applies to a range of p-values for a class of studies. For individual p-values from a single study, you need to use other methodologies just to estimate the false positive error rate. February 25, 2022 at 9:07 am Maybe we should continue, if you are willing, to do this via private email. I feel I have hijacked your thread here! So, I’ll just give one last response. My point was that those four scenarios partition the space of outcomes from experiments, so all four should add up to 1, and it doesn’t matter what order we list them. If we want to look at the probability that we make an error, in my list we can add them: P(error) = P(Case 1) + P(Case 4). In your list, you have written them as conditional probabilities, so they can’t be added. The probability of making an error is not α + β. This is why when errors type I and Type II errors are discussed, I think they should ALWAYS be described as conditional probabilities. To me, saying “rejecting a true null” is too likely to be interpreted as “rejecting and true null” rather than “rejecting | true null”. I’ve read your other pieces, and want to make sure I understand something. Above, you say the Type I error rate is simply α. However, in the article, you say the Type I error rate can be as high as 23% when P = 0.05. Does this just mean that apriori the error rate is α, but after you take your sample and get P=.05, you have new information, and the error rate has now climbed to 0.23? Thanks again. March 1, 2022 at 1:08 am I think this is a good discussion that others will benefit from. That’s why I always prefer discussion in the comments rather than via email! But that’s not correct thinking that those four scenarios should sum to 1. Perhaps we need to teach that better. However, the null hypothesis is either true or false. We don’t know the answer, but we do know that it’s one or the other. When the null is false, there is no chance of a false negative. And when the null is true, there is no chance of a false positive. I show two distribution curves in my post about the types of error. In actuality, only one of those curves exist, we just don’t know which one. As you say, they are conditional probabilities. Although, I think that’s baked right into the names as I’ve mentioned, but I can see the need to emphasize it. Getting to your questions about the error, there are a few complications! For one thing, the type I error rate equals the significance level (α), which applies to a range of p-values. Using frequentist methodology, there is no way to obtain an error rate for a single p-value from a study. However, using other methodologies, such as Bayesian statistics and simulation studies, you can estimate error rates for individual p-values. You do need to make some assumptions but it’s possible to come up with ballpark figures. And when I talk about error rates as high as 23% for a p-value of 0.05, it’s using those other methodologies. That’s why I consider a p-value around 0.05 (either above or below) to be fairly weak evidence on their own. I think I use an a priori probability of 0.5 for whether the null is true for the 23%. Obviously, the false positive rate will be higher when that probability is higher. But there was no reason to have assumed that a p-value of 0.05 should produce an error rate of 0.05 to begin with. That’s the common misinterpretation I discuss in my article about interpreting p-values. Many people link p-values to that type of error rate, but it’s just not true. And my point is that using conservative a priori probabilities, you can see that the true error rate is typically higher. Again, the Type I error rate equals the significance level, not an individual p-value. February 24, 2022 at 9:45 pm You wrote: “I still don’t quite understand what you’re saying about the vagueness of the Type I error rate. The type I error rate is the probability of rejecting a true null hypothesis. Therefore, by definition we’re talking about cases where the null hypothesis is correct.” This is what I meant. There are four non-overlapping possibilities, each with its own probability. 1. Reject a true null hypothesis. 2. Reject a false null hypothesis. 3. Fail to reject a true null hypothesis. 4. Fail to reject a false null hypothesis. It would be reasonable for one to conclude that the sum of these four probabilities is 1. However, when you say that the Type 1 error rate is the probability of rejecting a true null hypothesis, you actually mean the sum of the probabilities in 1 and 3 equals 1, and that the error rate is P(1)/( P(1) + P(3) ). February 25, 2022 at 3:05 am I guess if you write the list in that particular order, you’d need to sum non-adjacent items. Consequently, I wouldn’t list them in that order. It’s more logical to group them by whether the null hypothesis is true or not rather than by the rejection decision. But I do agree that we need to be clear when teaching this subject! 1. Reject a true null: error rate = α 2. Failing to reject a true null: correct decision rate = 1 – α. 3. Failing to reject a false null: error rate = β 4. Reject a false null: correct decision rate = 1 – β (aka statistical power) For more information on this topic, read my post about the two types of error in hypothesis testing . In that post, I put these in a table and I also show them on sampling distributions. February 24, 2022 at 8:10 am I completely resonate with what you say here. In fact, I’ve long thought that hypotheses should actually have an error tolerance built into them that somehow includes the effect size that is considered negligible. For example, it should be stated as an interval: mu = 100 +/- 1, if all values of mu in that range would be considered indistinguishable in any practical sense for the given context. Of course, this would make the calculation of the P-value a bit more complicated, and one would have to assume some type of distribution of the values of the parameter (probably normal or uniform) within the interval, but with technology this wouldn’t be a problem. I have never actually taken the step to see what effect such an approach would have on the P-values. Maybe none. Finally, I didn’t mean to imply that I think the definition of a Type I error is vague — I agree it is well-defined. What I meant is that I think that when the probability of a Type I error is discussed, we could all do a better job of clarifying that the sample space is all experiments for which the null is true. (Of course, that gets me back to my earlier issue, because I think the sample space is so small!) Thank you again for your responses. I want to read some of your other articles. I’m a mathematician who teaches statistics, and this is all very helpful to me. February 24, 2022 at 5:15 pm There is actually a standard way of doing just that. It involves using confidence intervals to evaluate both the magnitude and precision of the estimate effect. For more details, read my post about practical vs statistical significance . The nice thing is that CI approach is entirely consistent with p-values. I still don’t quite understand what you’re saying about the vagueness of the Type I error rate. The type I error rate is the probability of rejecting a true null hypothesis. Therefore, by definition we’re talking about cases where the null hypothesis is correct. And, even if that sample space is small, it’s not really a problem. Thanks for the interesting discussion! February 23, 2022 at 9:54 pm Thank you for the comment. That is a good point about the possibility of the null hypothesis being true with an equal sign for two-sample tests when considering the effect of a bogus drug. I guess I was mostly thinking of one-sample tests with a fixed standard in the null. Having said that, in your example, yes, it is easy to believe in a theoretically worthless treatment. In practice, if every subject of the population were tested (i.e., our sample is the population), an effect would likely always be observed, however small it is. In this case, then, it seems we probably need to define exactly what we mean when we refer to a population. To make my case (that the null is never true), I would define the population as an actual group of subjects who could conceivably be tested, not as an idealized theoretical group of all possible subjects. It seems the logic is backwards to say “The treatment is worthless, so the parameter must exactly equal zero.” On my other point, I realize that “probability of rejecting a null hypothesis that is true” is the usual definition. But I find this to be vague, because it can logically be interpreted by students as the probability of the intersection of two events: (1) Rejecting the null, and (2) The null is true. That is a very different than the conditional probability of Rejecting the null given that the null is true. I do realize these comments of mine are a bit pedantic. However, they have troubled me for some time, so I appreciate having your ear for a moment! February 23, 2022 at 10:45 pm For the sake of discussion, let’s go beyond the question of whether the null can be true exactly or not but ponder only those cases where it’s not exactly true but close. We’ll assume those cases exist to one degree or another even if we’re not sure how often. In those cases, it’s still not a problem. If the null is always false to some degree, then you don’t need to worry about Type I errors because that deals with true nulls. Instead, you’re worrying about Type II errors (failing to reject a false null) because that is applicable to false nulls. An effect exists but the test is not catching it. That sounds like a problem, but it isn’t necessarily. If the true population effect exists but is trivial, it’s not a problem if you fail to detect it. When you fail to reject the null in that case, you’re not missing out on an important finding. In fact, when you perform a power analysis before a test, you need to know the minimum effect size that is not trivial. This process helps you obtain a large enough sample so you have reasonable chance of detecting an effect that you’d consider important if it exists. (It also prevents you from obtain such a large sample size that you’ll detect a trivial effect.) In this scenario, you just want to have a reasonable chance of detecting an effect that is important. If you fail to reject the null in this case, it doesn’t matter whether the null is true or minimally false. In a practical sense that doesn’t matter. And remember, failing to reject the null doesn’t mean you’re proving the null is true. You can read my article about why we use the convoluted wording of failing to reject the null . So, in the scenario you describe, you wouldn’t worry about type I errors, only type II. And in that context, you want to detect important effects but it’s fine to fail to detect trivial effects. And that comes down to power analysis. I probably made that as clear as mud, but I hope you get my point. To learn more about how and why a power analysis builds in the idea of a practically significant effect size, read my post about power analysis . Finally, I don’t think the definition of a type I error is vague at all (or type II). They’re very specific. “It’s an error if you reject a null hypothesis that is true.” That statement is true by definition and has very precise meaning in the context of a hypothesis test where you define the null hypothesis. It’s certainly true that students can misinterpret that but that’s a point of education rather than a vague definition. It is an interesting discussion! February 23, 2022 at 10:38 am Could you clarify what you mean by the error rate? I think you said it is the conditional probability of Rejecting the null, given that the null is true? However, the null hypothesis is actually NEVER true if when we write = we really mean equal. It might be very close to being true, or it might be true to the level of precision with which we can measure, but it won’t actually be true. (In the same way that no matter how many decimals someone gives me for the value of the number pi, the value they give will still not actually equal pi.) However, in our hypotheses, we do not stipulate the level of accuracy for which we need to agree that two numbers are equal. So, my question: How does it make sense to talk about the conditional probability of an event when the underlying condition never happens? February 23, 2022 at 8:55 pm That’s correct that the error rate, more specifically, the Type I error rate, is the probability of rejecting a null hypothesis that is true. However, I’d disagree that the null hypothesis is never true when using an equal sign. For example, imagine that you’re testing a medication that truly is worthless. It has no effect whatsoever. If you perform an experiment with a treatment and control group, the null hypothesis is that the outcomes for the treatment group equals the control group. If the medication truly has zero effect, then at the population level, the outcomes should be equal. Of course, your sample means are unlikely to be exactly equal due to random sampling error. However, I would agree that there are many cases where, using the medication example, it has some effect but not a practically meaningful effect. In that case, the null hypothesis is not correct. But that’s not a problem. If you reject the null hypothesis when the treatment group is marginally better than the control group, it’s not an error. The hypothesis test made the correct decision by rejecting the null. At that point, it becomes a distinction between statistical significance and practical significance (i.e., importance in the real world). So, what you’re asking about is a concern, but a different type of concern than what you mention. The null hypothesis using equals is just fine. The real concern is whether after rejecting the null if the effect is practically significant.  February 23, 2021 at 2:40 pm Hi Jim, thank you for this explanation. I have one question. It is a probably a dumb question, but I am going to ask it anyway… Suppose I define the alpha as 5%. Does this mean that I have decided to reject the null hypothesis if p<0.05? Or when I define alpha as 5% I could use another threshold for the p-value? February 23, 2021 at 2:54 pm Hi Carolina, Yes, that’s correct! Technically, you reject the null if the p-value is less than or equal to 0.05 when you use an alpha of 0.05. So, basically what you said, but it’s less than or equal to.  February 23, 2021 at 2:59 am I found this blogpost by googling for “significance false positive rate”. I noticed that what you call “false positive rate” is apparently called “false discovery rate” elsewhere. According to Wikipedia, the false positive rate is the number of false positives (FP) divided by the number of negatives (TN + FP). So FP is _not_ divided by the number of positives (TP + FP); doing this, you would get (according to Wikipedia) just the “false discovery rate”. https://en.wikipedia.org/wiki/False_positive_rate https://en.wikipedia.org/wiki/False_discovery_rate Now I fully understand that the p value is not the same as the false discovery rate, as you correctly show. But how is the p value related to the false positive rate (defined as FP/(TN + FP))? February 23, 2021 at 3:20 pm Hi Andreas, The False Discovery Rate (FDR) and the False Positive Rate (FPR) are synonymous in this context. In statistics, one concept will sometimes have several different names. For example, alpha, the significance level, and the Type I error rate all mean the same thing! As you have found, analysts from different backgrounds will sometimes use these terms differently. It does make it a bit confusing! That’s why it’s good practice to include the calculations, as I do in this post. Thanks for writing!  January 12, 2021 at 10:33 am Many moons ago, when I was a junior electrical engineer, I wrote a white paper (for the US Navy). At the time, there was a big push to inject all sorts of Built-In Test (BIT) and Built-in Test Electronics (BITE) into avionics (i.e., aircraft weapon systems). The rapid pace of miniaturization of electronics made this a very attractive idea. In the paper I recommended we should slow down and inject BIT/E very judiciously, mainly for the reasons illustrated in your post. Specifically, if the actual failure rate of a weapon system is very low (i.e., the Prevalence of Real Effects is very small), and the Significance Level is too large, we will get a very high False Positive rate, which will result in the “pulling” of numerous “black boxes” for repair that don’t require maintenance. (BTW, this is what, in fact, happened. The incidence of “No Fault Found” on systems sent in for repair has gone up drastically.) And the Bayesian logic illustrated above is why certain medical diagnostic tests aren’t (or shouldn’t be) given to the general public: The prevalence in the general population is too low. The tests must be reserved for a sub-group of persons who are high risk for disease. Cheers, Joe January 12, 2021 at 3:15 pm Thanks so much for your insightful comment! These issues have real-world implications and I appreciate you sharing your experiences with us. Whenever anyone analyzes data, it’s crucial to know the underlying processes and subject area to understand correctly the implications, particularly when basing decisions on the analysis!  December 9, 2020 at 9:34 am Hello Jim, I have been binge reading the blogs/articles written by you. It is very helpful. I have a question related to prevalence. Is the concept of prevalence applicable to all scenarios and end goals (for which the analysis is performed) similar to the way alpha and beta are. For example, in the example that is relate to change in per capita income (from 260 to 330), my understanding is that prevalence does not hold true, Is that correct? If not, how to interpret/understand prevalence in that example? Your inputs will be helpful. December 10, 2020 at 12:13 am In this context, the prevalence is the probability that the effect exists in the population. You’d need to be able to come up with some probability that the per capita income has changed from 260 to 330. I think coming up with a good estimate can often be difficult. It becomes easier as a track record develops. Is that size change typical or unusual in previous years? Does it fit other economic observations? Etc. Coming up with a rough estimate can help you evaluate p-values.  November 23, 2020 at 8:55 am Thank you so much Jim. This was even better than what I expected when I asked you to explain: Sellke et al. I am going to suggest to all my fellow (Data) Scientists that this be a must read. November 24, 2020 at 12:25 am Thanks, Steven! I appreciate the kind words and sharing! November 23, 2020 at 8:36 am Looking forward to that.  November 21, 2020 at 1:47 pm This is a nice post. The language is not just elementary, it also made complex concepts intuitively easier to grasp. I have read these concepts several times in many textbooks, for the first time I have a better understanding of the lay application behind the erstwhile difficult topics.  November 21, 2020 at 12:24 am Thanks a lot Jim. It will be better, if you take this in the context of Panel data  November 19, 2020 at 1:59 pm Jim, thank you. As always, so informative and you are constantly challenging me with different ways of approaching concepts. Have you or do you know of any studies that applies this approach to COVID testing? I’m thinking about recent news from Elon Musk in which he said he had 4 tests done in the same day, same test, same health professional. Two came back positive and two negative. Is there a substantial error rate on these tests? November 19, 2020 at 11:09 am Dear Sir My question is that I have a dep variable say X and a variable of interest Y with some control variables(Z) Now when I run following regressions 1) X at time t , Y & Z at t-1 2) X at time t , Y at t-1 & Z at t 3) X at time t , Y & Z at t The sign of my variable of interest changes(significance too). If there are not any theory to guide me with respect lag specification of variable of interest and control variables, which one from the above model should I use? What is the general principle November 21, 2020 at 12:08 am A good method for identify lags to include is to use the cross correlation function (CCF). This helps find lags of on time series that can predict the current value of your time series of interest. You can also use the autocorrelation function (ACF) and partial autocorrelation function (PACF) to identify lags within one time series. These functions simply look for correlations between observations of a time series that are separated by k time units. CCF is between different sets of time series data while ACF and PACF are within one set of time series data. I don’t currently have posts about these topics but they’re on my list!  November 17, 2020 at 10:43 am Thanks so much for your great post. It’s always been tremendously helpful. I have one simple question about the difference between a significance level and a false positive rate. I have read your comment in one of your p-value posts: “When you’re talking significance levels and the Type I error rate, you’re talking about an entire class of studies. You can’t apply that to individual studies.” But, in this post, we simulated a test 1000 times, and in my humble opinion, it seemed like we treated 1000 tests as a kind of “a class of studies.” However, the false positive rate, 0.36, is still pretty different from the initial significance level setup, 0.05. I think this is a silly question, but could you please kindly clarify this? November 17, 2020 at 3:48 pm That’s a great question. And there’s a myriad of details details like that which are crucial to understand. That’s why it’s such a deep, dark rabbit hole! What you’re asking about gets to the heart of a major difference Frequentist and Bayesian statistics. Using Frequentist methodology, there’s no probability associated with the null hypothesis. It’s true or not true but you don’t know. The significance level is part of the Frequentist methodology. So, it can’t calculate a probability about whether the null is true. Instead, the significance level assumes the null hypothesis is true and goes from there. The significance level indicates the probability of the hypothesis producing significant results when the null is true. So, you don’t know whether the null is true or not, but you do know that IF it is true, your test is unlikely to be significant. Think of the significance level as a conditional probability based on the null being true. Compare that to the Bayesian approach, where you can have probabilities associated with the null hypothesis. The example I work through is akin to the Bayesian approach because we’re stating that the null has a 90% chance of being correct and a 10% chance of being incorrect. That’s a different scenario than Frequentist methodology where you assume the null is true. That’s why the numbers are different because they’re assessing different scenarios and assumptions. In a nutshell, yes, the 1000 tests can be a class of studies but this class includes cases where the null is both true and false at some assumed proportion. For significance levels, the class of studies contains only studies where the null hypothesis is true (e.g., 5% of all studies where the null is true). I hope that clarifies that point!  November 17, 2020 at 10:32 am Idea! It is not necessary to use the notation α for the threshold (critical) value of the random variable P ̃_v=Pr[(T ̃≤-|t|│H_0 )+(T ̃≥+|t|│H_0 )] and call it the significance level. For it a different notation, for instance, p_crit should be used. There is no direct relationship between the observed p-value (p_val) and the probability of the null hypothesis P(H_0│data), just as there is no direct relationship between the critical p-value p_crit and the significance level α (the probability of a type I error)! November 17, 2020 at 3:49 pm I don’t follow your comment. Is this just your preference for the notation or something more? Alpha is the usual notation for this concept.  November 17, 2020 at 3:40 am Very informative and useful. Thank you November 17, 2020 at 4:04 pm You’re very welcome! I’m glad it was helpful! Comments and Questions Cancel replyStack Exchange NetworkStack Exchange network consists of 183 Q&A communities including Stack Overflow , the largest, most trusted online community for developers to learn, share their knowledge, and build their careers. Q&A for work Connect and share knowledge within a single location that is structured and easy to search. Understanding p-values using an exampleDefinition of p-values: A p-value is a probability that provides a measure of the evidence against the null hypothesis provided by the sample. Smaller p-values indicate more evidence against null hypothesis. Can someone please explain this in simpler terms or in a language easy to understand? I know there might already be tons of questions around understanding the interpretation of p-values, however I would ask the question in a very limited form and with the use of a specific example: A manufacturing company fills up can with mean weight of 3 pounds, the level of significance is assumed to be 0.01 H(0) : u >= 3 -- Null hypotheses H(a) : u < 3 -- Alternate hypotheses We are trying to perform a one tailed test for the case where the population standard deviation is known, so for a sample mean of 2.92 and a standard error of 0.03 , we get the z-score as -2.67 , giving us the probability (p-value) of 0.0038 or 0.38% that the sample mean would be equal to or lower than 2.92 . Since the probability of getting a sample mean equal or less than 2.92 is 0.38% , which is very small, doesn't it mean that we should accept the null hypotheses? As the chances of getting a mean of 2.92 from a sample is only 0.38%. Or am I completely missing something here? Edit - It has been three days now since I tried understanding hypothesis testing and I think I am almost there, I will try to articulate what I have understood so far and then let me know if there are still any gaps in my understanding p-values measure the likelihood of obtaining the sample mean that we obtained given that the null hypothesis is true. So for the example that I mentioned, the probability of obtaining a sample mean of 2.92 is 0.038 if that population's mean is 3 (as assumed by the null hypothesis). Now there could be two reasons for obtaining means of 2.92: - The assumed population mean (i.e., the null hypothesis) is not correct, or
- the population mean is 3 but due to a sampling error / an unlikely sample we got a mean of 2.92.
Now if we select statement 1, we run the chance of making type 1 error and this is where the level of significance comes into play. Using the level of significance we can see if we can reject the null hypothesis or cannot reject null hypothesis. - hypothesis-testing
- statistical-significance
 - $\begingroup$ You say the population standard deviation $\sigma$ is known. Can you provide the known value? The terminology "correction factor" is not familiar to me; can you give a formula for finding that? // The sample mean $\bar X = 2.92$ is below the hypothetical population mean $\mu_0 = 3.$ The issue is whether it is enough smaller to warrant rejecting the null hypothesis. $\endgroup$ – BruceET Commented Apr 20, 2019 at 13:20
- $\begingroup$ The population standard deviation is .18 and sample size is 36, hence the correction factor is 0.18/sqrt(36) equals 0.03 $\endgroup$ – Rohit Saluja Commented Apr 20, 2019 at 13:24
- 3 $\begingroup$ Thanks for the additional information. The usual terminology is to call $\sigma/\sqrt{n}$ the 'standard error'. $\endgroup$ – BruceET Commented Apr 20, 2019 at 13:27
- $\begingroup$ @BruceET - the issue is if it is enough smaller to reject the null hypotheses, however the probability of sample mean of being less than or equal to 2.92 is only 0.0038, so can't we say that the probability of sample mean of less than 3 is very less hence we support null hypotheses. $\endgroup$ – Rohit Saluja Commented Apr 20, 2019 at 13:39
4 Answers 4Imagine you could measure the weight of all cans that the manufacturing company has ever made and the mean would be $2.87$ pounds. Then imagine you would take 10 cans randomly and see how much they weight. It is unlikely to get the exact mean of all cans ( $2.87$ pounds), hence you end up with a mean of $2.65$ , for example. If you would do that again and again - taking 10 cans and measuring the weight - you would get a distribution of means. The best guess about the true mean is the mean of the distribution you obtained. Extreme values like $1.9$ or $3.5$ pounds will be unlikely and even more extreme values will be even more unlikely. Doing significance tests usually means that you look how likely the mean you observed is if you assume that your sample was drawn from a population with mean zero. If the mean that you observed is very unlikely you would decide to discard the null hypothesis. The only difference between what I have said so far and your example is that you assume the null hypothesis a mean of $\ge 3$ . So the $0.38\%$ you report say that the probability of getting your mean of $2.92$ from a population with a mean of $\ge 3$ is so unlikely that you would discard the null hypothesis and accept the alternative hypothesis which is $<3$ . Your evidence indicate that the cans weight less than $3$ pounds. This means it is the opposite: having a $p$ of $0.38\%$ as you report doesn't mean you have to keep the null hypothesis because your result is so unlikely but it means that you can discard the null hypothesis because your data was very unlikely a randomly drawn sample from a population with a mean of $3$ (i.e., your data would be very unlikely given that the null hypothesis is true). - $\begingroup$ Comments are not for extended discussion; this conversation has been moved to chat . $\endgroup$ – gung - Reinstate Monica Commented Apr 23, 2019 at 14:51
Here is a figure that shows your problem on two scales: at left is the original scale in terms of pounds; at right is the standard of z-scale often used in testing.  To begin, let's look at your problem in terms of the fixed significance level $\alpha = 0.01 = 1\%.$ In the right-hand panel, your $Z$ -score is shown at the heavy vertical bar at $-2.67.$ The "critical value" for a test at the 1% level is shown by the vertical dotted line at $-2.326,$ which cuts 1% of the probability from the lower tail of the standard normal distribution. Because the $Z$ -score is to the left of the critical value, one rejects the null hypothesis at level $\alpha = 1\%.$ The P-value is the probability under the standard normal curve to the left of the heavy blue line. That area is smaller than $1\%,$ so in terms of P-values, we reject $H_0$ when the P-value is smaller than $1\%.$ You can see that the left-hand plot is the same as the right-hand plot, except for scale. It is not possible to make a printed normal table for all possible normal distributions. By converting to $Z$ -scores we can always use a single printed table for the 'standard' normal distribution, which has mean 0 and standard deviation 1. If we were going to do this production-monitoring procedure repeatedly with $n = 36$ observations each time, then we could find the critical value on the 'pound' scale; it is at 2.581 pounds. (That's because $(2.581 - 3)/.18 = -2.236,$ where the $0.18$ is the standard error.) Then we could turn the testing job over to a non-statistician, with instructions: "If the average weight for 36 cans is less than 2.581 pounds, let me know because we aren't putting enough stuff in our cans." (Or if we can't even trust the non-statistician with averages, the criterion might be a total weight less than 92.92 pounds.)  Since your question is actually quite precise, I would like to keep it rather concise. Definition of p-value: the p-value is the probability of the data (or even more extrem data) given the null hypothesis is actually true. If this probability is high, then there is no reason why we should reject the null hypothesis: the data is perfectly in line with the null hypothesis. If the p-value is small, then the data seems implausible given the null hypothesis. The more implausible the data, the stronger our evidence against the null. A level of significance of 0.01 means: to reject the null hypothesis, the probability of the data must be less than 1%. If the null hypothesis is actually true, we have therefore 1% chance to see data, which is so implausible that we would wrongly reject the null hypothesis. Regarding your example: there is only 0.38% chance to see this data, if the null hypothesis is true, which is below our threshold of significance. Hence, the data seems very unlikely, and therefore we conclude that we don't believe in the null hypothesis anymore.  Assume the significance level is $\alpha$ , which when talking about the null hypothesis, we are usually looking at 5% or 1% and so on. In simple terms: p-value is the smallest $\alpha$ at which we reject the null hypothesis. So, when your p-value is 0.15, then we accept the null hypothesis when $\alpha$ is 5% (or our confidence interval is 90%). But change that to only have a confidence interval of 60% and you reject your null hypothesis. Similarly, when your p-value=0.0038, it means you accept the null hypothesis under any value smaller than < 0.38%. That's why you compare p-value with $\alpha$ and if p-value < $\alpha$ , you say that you cannot accept the null hypothesis.  Your AnswerSign up or log in, post as a guest. Required, but never shown By clicking “Post Your Answer”, you agree to our terms of service and acknowledge you have read our privacy policy . Not the answer you're looking for? Browse other questions tagged hypothesis-testing statistical-significance p-value or ask your own question .- Featured on Meta
- We've made changes to our Terms of Service & Privacy Policy - July 2024
- Bringing clarity to status tag usage on meta sites
Hot Network Questions- How soon to fire rude and chaotic PhD student?
- Does the overall mAh of the battery add up when batteries are parallel?
- how replicate this effect with geometry nodes
- How does a closed-cycle rocket engine keep the chamber pressure from stalling the pump turbine?
- Four out puzzle: Get rid of a six digit number in 4 moves
- What is the default font of report documentclass?
- How should I respond to a former student from my old institution asking for a reference?
- "Knocking it out of the park" sports metaphor American English vs British English?
- Is the Shroud of Turin about 2000 years old?
- MySQL 5.7: Is it relevant/risk to have external hosts in the mysql.Hosts column if "skip-networking" is enabled?
- Fast circular buffer
- Is there anything that stops the majority shareholder(s) from destroying company value?
- Why do combinatorists care about Kazhdan–Lusztig polynomials?
- Who gave God the name 'Jealous' as referred to in Exodus 34:14?
- SF novel where the story, or part of it, is narrated by two linked brains taking turns
- Reed-Solomon error count if errors cannot be corrected
- What sort of impact did the discovery that water could be broken down (via electrolysis) into gas have?
- Fitting 10 pieces of pizza in a box
- A binary sequence such that sum of every 10 consecutive terms is divisible by 3 is necessarily periodic?
- Highlight shortest path between two clickable graph vertices
- Why don't we observe protons deflecting in J.J. Thomson's experiment?
- One IO to control two LEDs. When one is lit, the other is not
- Can you successfully substitute pickled onions for baby onions in Coq Au Vin?
- Reference request: "Higher order eigentuples" as generalized eigenvectors?
 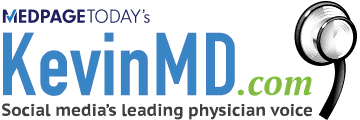 How physicians can fix media bias with scienceThe assassination attempt is the straw that breaks the camel’s back. The “gaslighting” is over. The rules for truth by legacy media are never examined for objectivity. We do not have the Inquisition in the United States; we have the legacy media. One “fact-checker” measures truth by “Pinocchios.” There is a better way—hypothesis testing. Who better to know about hypothesis testing than a physician? What if the facts about how Medicare is represented by two media outlets are tested? Hypothesis testing follows four rules: 1. Identify the truth: The truth is out there. Truth-telling has nine phases, each representing a specific duty that pertains to an ideal storyteller. - The initiation phase: The duty to collect all the facts.
- The acceptance phase: The duty to accept a fact verifiable by objective evidence.
- The rejection phase: The duty to reject an artifact not verifiable by objective evidence.
- The attribution phase: The duty to source the facts.
- The external review phase: The duty to examine the motives of others to influence facts.
- The internal review phase: The duty to examine a personal motive to influence facts.
- The discrimination phase: The duty to distinguish an opinion from a fact. Opinions, even a consensus by authorities, are not facts.
- The equanimity phase: The duty not to contaminate a fact with emotion.
- The analysis phase: The duty to use facts, and only facts, to arrive at a conclusion.
2. State the subject matter: It is the actual storyteller’s version of reality. The subject matter contains the same facts, but some may be subtly misrepresented, just enough to satisfy the conclusion. The subject matter is divided into the same nine phases as they pertain to the actual storyteller. 3. The Test: Each phase of the subject matter is compared to its counterpart in the truth. The comparison measures the “relative risk” resulting from the misrepresentation of a fact by the actual storyteller. - If there is no difference, the relative risk equals 1.0.
- If there is a difference, the relative risk is greater than 1.0. A relative risk greater than 1.0 is a Risk of Bias. For the sake of transparency, the assignments of Risk of Bias are documented for anyone to see and, if need be, to dispute.
A single sample of nine relative risks emerges representing each phase in the subject matter. Some are 1.0, and some are greater than 1.0. Because storytellers naturally tend to exaggerate a fact, producing a relative risk greater than 1.0, this discrepancy itself is not proof of a departure from the truth. Bias is intentional. For proof, the collective difference among the nine relative risks in all phases of the subject matter must be statistically significant. 4. Analysis: To determine a statistically significant difference, the sample is analyzed using the single-sample T-test, found in any statistical software. The level of significance, or alpha, is 0.05, which corresponds to 95 percent confidence. The population mean, or mu, is 1.0, which corresponds to the truth. The result is the p-value. - If the p-value is equal to or greater than 0.05, there is no statistically significant difference between the subject matter and the truth. Although there may be a phase that contains an exaggeration, the risk of bias is not sufficient for it to misrepresent reality. Therefore, there is no bias. This is the null hypothesis. If the null hypothesis is retained, the subject matter is the null hypothesis.
- If the p-value is less than 0.05, there is a statistically significant difference. Therefore, there is quantifiable proof of bias. This is the alternate hypothesis. The alternate hypothesis is accepted by default. If the null hypothesis is rejected, the subject matter is the alternate hypothesis.
Hypothesis testing, unlike “Pinocchios,” objectively makes a valid comparison between truth and facsimile. A Pinocchio, while quantitative, has no level of confidence. However, a p-value has a level of confidence of 95 percent. For a rational person, 95 percent confidence stands in stark contrast to a Pinocchio. As an example of hypothesis testing, the truth consists of the verifiable facts about Medicare that are publicly available in government documents. The subject matter consists of two media outlets’ versions of the truth. One storyteller is Fox News. The sample is 1.0, 1.0, 1.0, 1.0, 1.0, 1.5, 1.5, 2.0, 1.0, and the p-value is 0.051893. The collective risk of bias is not sufficient to misrepresent reality. The other storyteller is MSNBC. The sample is 1.5, 1.5, 1.5, 2.0, 1.5, 1.5, 1.5, 2.0, 2.0, and the p-value is 0.000022. The collective risk of bias is sufficient to misrepresent reality. The difference between the two p-values shows that MSNBC’s version of Medicare is 99.9 percent less reliable than Fox’s version. Howard Smith is an obstetrics-gynecology physician.  Why I won't let my wife see her EOBs anymore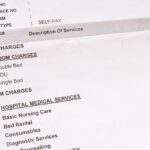 How compassionate communities can transform the lives of schizophrenia patients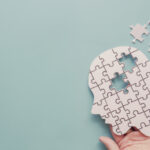 Tagged as: Mainstream media More by Howard Smith, MD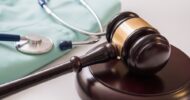 The truth about medical lawsuits: What the numbers revealThe flaw with medical malpractice litigation, why most medical malpractice claims never see a courtroom, related posts. 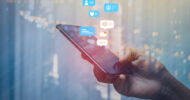 Social media: Striking a balance for physicians and parents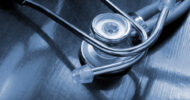 I was trolled by another physician on social media. I am happy I did not respond.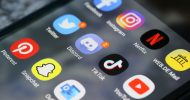 Are negative news cycles and social media injurious to our health?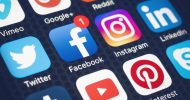 How I used social media to get promoted to professor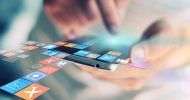 Sharing mental health issues on social media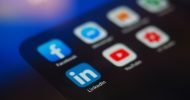 How physicians can engage on social mediaMore in physician. 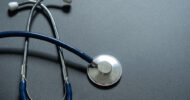 How a doctor transformed grief into personal growth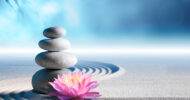 How embracing vulnerability transforms pain into power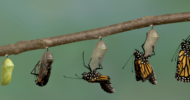 A life of purpose: free from societal pressures that lead us astray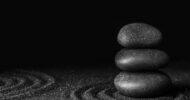 The resilience of international medical graduates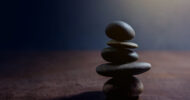 The practice of delayed gratification in medical training: a double-edged sword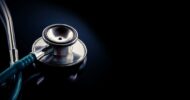 When a patient’s story hits close to home: a doctor’s emotional journeyMost popular. 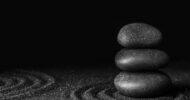 A doctor’s eye-opening journey as a patient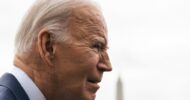 President Biden: a closer look at leadership, dignity, and aging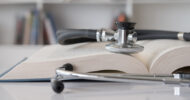 How medical school curricula perpetuate inequality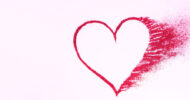 Unlock your child’s potential: the power of nurturing hidden talents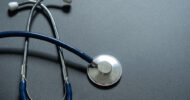 The surprising secret to success in medical schoolPast 6 months. 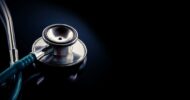 Struggles of navigating prestigious medical systems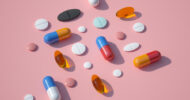 The truth behind opioid use disorder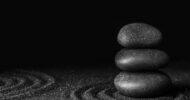 Creating a subspecialty track for experienced hospitalists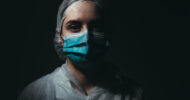 The sham peer review: a hidden contributor to the doctor shortage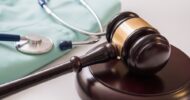 The unseen dangers of faulty expert witness testimonyRecent posts. 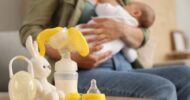 From plant milk to human milk: the untapped climate solution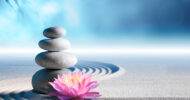 Tax-free income with the Augusta rule [PODCAST]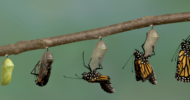 Subscribe to KevinMD and never miss a story!Get free updates delivered free to your inbox.  Find jobs at Careers by KevinMD.comSearch thousands of physician, PA, NP, and CRNA jobs now.  CME Spotlights Leave a CommentComments are moderated before they are published. Please read the comment policy .  Docker is an open platform for developing, shipping, and running applications. Docker allows you to separate your applications from your infrastructure so you can deliver software quickly. With Docker, you can manage your infrastructure in the same ways you manage your applications. By taking advantage of Docker’s methodologies for shipping, testing, and deploying code quickly, you can significantly reduce the delay between writing code and running it in production. You can download and install Docker on multiple platforms. Refer to the following section and choose the best installation path for you. Docker Desktop terms Commercial use of Docker Desktop in larger enterprises (more than 250 employees OR more than $10 million USD in annual revenue) requires a paid subscription . Note If you're looking for information on how to install Docker Engine, see Docker Engine installation overview . 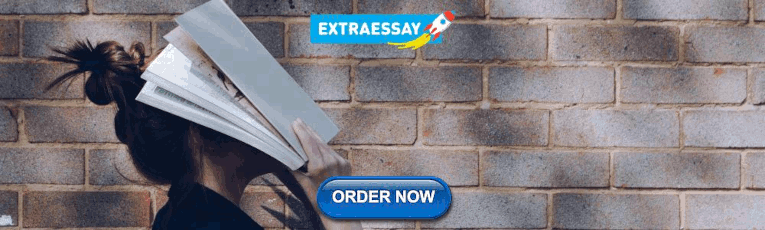 |
IMAGES
COMMENTS
The p value is a proportion: if your p value is 0.05, that means that 5% of the time you would see a test statistic at least as extreme as the one you found if the null hypothesis was true. Example: Test statistic and p value If the mice live equally long on either diet, then the test statistic from your t test will closely match the test ...
One of the most commonly used p-value is 0.05. If the calculated p-value turns out to be less than 0.05, the null hypothesis is considered to be false, or nullified (hence the name null hypothesis). And if the value is greater than 0.05, the null hypothesis is considered to be true. Let me elaborate a bit on that.
Reject the null hypothesis when the p-value is less than or equal to your significance level. Your sample data favor the alternative hypothesis, which suggests that the effect exists in the population. ... Below are typical examples of writing a null hypothesis for various parameters and hypothesis tests. Related posts: Descriptive vs ...
A p-value, or probability value, is a number describing how likely it is that your data would have occurred by random chance (i.e., that the null hypothesis is true). The level of statistical significance is often expressed as a p-value between 0 and 1. The smaller the p -value, the less likely the results occurred by random chance, and the ...
Two-Tailed. In our example concerning the mean grade point average, suppose again that our random sample of n = 15 students majoring in mathematics yields a test statistic t* instead of equaling -2.5.The P-value for conducting the two-tailed test H 0: μ = 3 versus H A: μ ≠ 3 is the probability that we would observe a test statistic less than -2.5 or greater than 2.5 if the population mean ...
The p-value represents the strength of your sample evidence against the null. Lower p-values represent stronger evidence. Like the significance level, the p-value is stated in terms of the likelihood of your sample evidence if the null is true. For example, a p-value of 0.03 indicates that the sample effect you observe, or more extreme, had a 3 ...
In this case, our t-value of 2.289 produces a p value between 0.02 and 0.05 for a two-tailed test. Our results are statistically significant, and they are consistent with the calculator's more precise results. Displaying the P value in a Chart. In the example above, you saw how to calculate a p-value starting with the sample statistics.
The p value is the evidence against a null hypothesis. The smaller the p-value, the stronger the evidence that you should reject the null hypothesis. P values are expressed as decimals although it may be easier to understand what they are if you convert them to a percentage. For example, a p value of 0.0254 is 2.54%.
The null hypothesis states that there is no statistical difference between groups based on the stated research hypothesis. ... For example, a p-value from a double-blinded randomized clinical trial (designed to minimize bias) should be weighted higher than one from a retrospective observational study .
In the first part of this example, we rejected the null hypothesis when \(\alpha = 0.05\). And, in the second part of this example, we failed to reject the null hypothesis when \(\alpha = 0.01\). There must be some level of \(\alpha\), then, in which we cross the threshold from rejecting to not rejecting the null hypothesis.
The p -value is used in the context of null hypothesis testing in order to quantify the statistical significance of a result, the result being the observed value of the chosen statistic . [ note 2] The lower the p -value is, the lower the probability of getting that result if the null hypothesis were true. A result is said to be statistically ...
H 0 (Null Hypothesis): Population parameter =, ≤, ≥ some value. H A (Alternative Hypothesis): Population parameter <, >, ≠ some value. Note that the null hypothesis always contains the equal sign. We interpret the hypotheses as follows: Null hypothesis: The sample data provides no evidence to support some claim being made by an individual.
The observed value is statistically significant (p ≤ 0.05), so the null hypothesis (N0) is rejected, and the alternative hypothesis (Ha) is accepted. Usually, a researcher uses a confidence level of 95% or 99% (p-value of 0.05 or 0.01) as general guidelines to decide if you should reject or keep the null.
The way to interpret that p-value is: observing 38 heads or less out of the 100 tosses could have happened in only 1% of infinitely many series of 100 fair coin tosses. The null hypothesis in this case is defined as the coin being fair, therefore having a 50% chance for heads and 50% chance for tails on each toss.. Assuming the null hypothesis is true allows the comparison of the observed data ...
In technical terms, a P value is the probability of obtaining an effect at least as extreme as the one in your sample data, assuming the truth of the null hypothesis. For example, suppose that a vaccine study produced a P value of 0.04. This P value indicates that if the vaccine had no effect, you'd obtain the observed difference or more in 4 ...
The p-value (or the observed level of significance) is the smallest level of significance at which you can reject the null hypothesis, assuming the null hypothesis is true. You can also think about the p-value as the total area of the region of rejection. Remember that in a one-tailed test, the regi
How to calculate p-value. Below are steps you can use to help calculate the p-value for a data sample: 1. State the null and alternative hypotheses. The first step to calculating the p-value of a sample is to look at your data and create a null and alternative hypothesis. For example, you could state that a hypothesized mean "μ" is equal to 10 ...
This probability is called the P-value. If the value of p-value is smaller than the level of significance, it provides evidence against the null hypothesis. For example, let's say we are testing the claim that the students studying more than 6 hours on average get more than 75% marks. Here are the steps followed:
This example shows that the p-value is entirely dependent on the test statistic used and that p-values can only be used to reject a null hypothesis, not to explore an alternate hypothesis. P-value Formula. We Know that P-value is a statistical measure, that helps to determine whether the hypothesis is correct or not. P-value is a number that ...
Therefore, the null hypothesis is accepted. Example 2: P-value is 0.3105. If the level of significance is 5%, find if we can reject the null hypothesis. Solution: Looking at the P-value table, the p-value of 0.3105 is greater than the level of significance of 0.05 (5%), we fail to reject the null hypothesis. Example 3: P-value is 0.0219.
The precise value of the p-value affects the size of that decrease. Smaller p-values cause a larger decline. Finally, the posterior probability is also the false positive rate in this context because of the following: the low p-values cause the hypothesis test to reject the null.
To decide if we should reject or fail to reject each null hypothesis, we must refer to the p-values in the output of the two-way ANOVA table. The following examples show how to decide to reject or fail to reject the null hypothesis in both a one-way ANOVA and two-way ANOVA. Example 1: One-Way ANOVA
p-values measure the likelihood of obtaining the sample mean that we obtained given that the null hypothesis is true. So for the example that I mentioned, the probability of obtaining a sample mean of 2.92 is 0.038 if that population's mean is 3 (as assumed by the null hypothesis). Now there could be two reasons for obtaining means of 2.92: The ...
Answer: Given that the null hypothesis is true, a p-value is the probability that you would see a result at least as extreme as the one observed. P-values are typically calculated to determine whether the result of a statistical test is significant. In simple words, the p-value tells us whether there is enough evidence to reject the null ...
Therefore, there is no bias. This is the null hypothesis. If the null hypothesis is retained, the subject matter is the null hypothesis. If the p-value is less than 0.05, there is a statistically significant difference. Therefore, there is quantifiable proof of bias. This is the alternate hypothesis. The alternate hypothesis is accepted by default.
Download and install Docker on the platform of your choice, including Mac, Linux, or Windows.