Examples of data management plans
These examples of data management plans (DMPs) were provided by University of Minnesota researchers. They feature different elements. One is concise and the other is detailed. One utilizes secondary data, while the other collects primary data. Both have explicit plans for how the data is handled through the life cycle of the project.
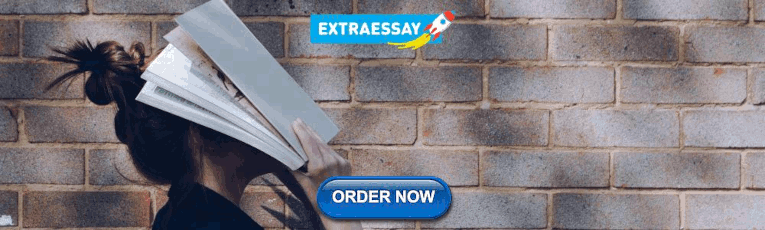
School of Public Health featuring data use agreements and secondary data analysis
All data to be used in the proposed study will be obtained from XXXXXX; only completely de-identified data will be obtained. No new data collection is planned. The pre-analysis data obtained from the XXX should be requested from the XXX directly. Below is the contact information provided with the funding opportunity announcement (PAR_XXX).
Types of data : Appendix # contains the specific variable list that will be used in the proposed study. The data specification including the size, file format, number of files, data dictionary and codebook will be documented upon receipt of the data from the XXX. Any newly created variables from the process of data management and analyses will be updated to the data specification.
Data use for others : The post-analysis data may be useful for researchers who plan to conduct a study in WTC related injuries and personal economic status and quality of life change. The Injury Exposure Index that will be created from this project will also be useful for causal analysis between WTC exposure and injuries among WTC general responders.
Data limitations for secondary use : While the data involve human subjects, only completely de-identified data will be available and used in the proposed study. Secondary data use is not expected to be limited, given the permission obtained to use the data from the XXX, through the data use agreement (Appendix #).
Data preparation for transformations, preservation and sharing : The pre-analysis data will be delivered in Stata format. The post-analysis data will also be stored in Stata format. If requested, other data formats, including comma-separated-values (CSV), Excel, SAS, R, and SPSS can be transformed.
Metadata documentation : The Data Use Log will document all data-related activities. The proposed study investigators will have access to a highly secured network drive controlled by the University of Minnesota that requires logging of any data use. For specific data management activities, Stata “log” function will record all activities and store in relevant designated folders. Standard file naming convention will be used with a format: “WTCINJ_[six letter of data indication]_mmddyy_[initial of personnel]”.
Data sharing agreement : Data sharing will require two steps of permission. 1) data use agreement from the XXXXXX for pre-analysis data use, and 2) data use agreement from the Principal Investigator, Dr. XXX XXX ([email protected] and 612-xxx-xxxx) for post-analysis data use.
Data repository/sharing/archiving : A long-term data sharing and preservation plan will be used to store and make publicly accessible the data beyond the life of the project. The data will be deposited into the Data Repository for the University of Minnesota (DRUM), http://hdl.handle.net/11299/166578. This University Libraries’ hosted institutional data repository is an open access platform for dissemination and archiving of university research data. Date files in DRUM are written to an Isilon storage system with two copies, one local to each of the two geographically separated University of Minnesota Data Centers. The local Isilon cluster stores the data in such a way that the data can survive the loss of any two disks or any one node of the cluster. Within two hours of the initial write, data replication to the 2nd Isilon cluster commences. The 2nd cluster employs the same protections as the local cluster, and both verify with a checksum procedure that data has not altered on write. In addition, DRUM provides long-term preservation of digital data files for at least 10 years using services such as migration (limited format types), secure backup, bit-level checksums, and maintains a persistent DOIs for data sets, facilitating data citations. In accordance to DRUM policies, the de-identified data will be accompanied by the appropriate documentation, metadata, and code to facilitate reuse and provide the potential for interoperability with similar data sets.
Expected timeline : Preparation for data sharing will begin with completion of planned publications and anticipated data release date will be six months prior.
Back to top
College of Education and Human Development featuring quantitative and qualitative data
Types of data to be collected and shared The following quantitative and qualitative data (for which we have participant consent to share in de-identified form) will be collected as part of the project and will be available for sharing in raw or aggregate form. Specifically, any individual level data will be de-identified before sharing. Demographic data may only be shared at an aggregated level as needed to maintain confidentiality.
Student-level data including
- Pre- and posttest data from proximal and distal writing measures
- Demographic data (age, sex, race/ethnicity, free or reduced price lunch status, home language, special education and English language learning services status)
- Pre/post knowledge and skills data (collected via secure survey tools such as Qualtrics)
- Teacher efficacy data (collected via secure survey tools such as Qualtrics)
- Fidelity data (teachers’ accuracy of implementation of Data-Based Instruction; DBI)
- Teacher logs of time spent on DBI activities
- Demographic data (age, sex, race/ethnicity, degrees earned, teaching certification, years and nature of teaching experience)
- Qualitative field notes from classroom observations and transcribed teacher responses to semi-structured follow-up interview questions.
- Coded qualitative data
- Audio and video files from teacher observations and interviews (participants will sign a release form indicating that they understand that sharing of these files may reveal their identity)
Procedures for managing and for maintaining the confidentiality of the data to be shared
The following procedures will be used to maintain data confidentiality (for managing confidentiality of qualitative data, we will follow additional guidelines ).
- When participants give consent and are enrolled in the study, each will be assigned a unique (random) study identification number. This ID number will be associated with all participant data that are collected, entered, and analyzed for the study.
- All paper data will be stored in locked file cabinets in locked lab/storage space accessible only to research staff at the performance sites. Whenever possible, paper data will only be labeled with the participant’s study ID. Any direct identifiers will be redacted from paper data as soon as it is processed for data entry.
- All electronic data will be stripped of participant names and other identifiable information such as addresses, and emails.
- During the active project period (while data are being collected, coded, and analyzed), data from students and teachers will be entered remotely from the two performance sites into the University of Minnesota’s secure BOX storage (box.umn.edu), which is a highly secure online file-sharing system. Participants’ names and any other direct identifiers will not be entered into this system; rather, study ID numbers will be associated with the data entered into BOX.
- Data will be downloaded from BOX for analysis onto password protected computers and saved only on secure University servers. A log (saved in BOX) will be maintained to track when, at which site, and by whom data are entered as well as downloaded for analysis (including what data are downloaded and for what specific purpose).
Roles and responsibilities of project or institutional staff in the management and retention of research data
Key personnel on the project (PIs XXXXX and XXXXX; Co-Investigator XXXXX) will be the data stewards while the data are “active” (i.e., during data collection, coding, analysis, and publication phases of the project), and will be responsible for documenting and managing the data throughout this time. Additional project personnel (cost analyst, project coordinators, and graduate research assistants at each site) will receive human subjects and data management training at their institutions, and will also be responsible for adhering to the data management plan described above.
Project PIs will develop study-specific protocols and will train all project staff who handle data to follow these protocols. Protocols will include guidelines for managing confidentiality of data (described above), as well as protocols for naming, organizing, and sharing files and entering and downloading data. For example, we will establish file naming conventions and hierarchies for file and folder organization, as well as conventions for versioning files. We will also develop a directory that lists all types of data and where they are stored and entered. As described above, we will create a log to track data entry and downloads for analysis. We will designate one project staff member (e.g., UMN project coordinator) to ensure that these protocols are followed and documentation is maintained. This person will work closely with Co-Investigator XXXXX, who will oversee primary data analysis activities.
At the end of the grant and publication processes, the data will be archived and shared (see Access below) and the University of Minnesota Libraries will serve as the steward of the de-identified, archived dataset from that point forward.
Expected schedule for data access
The complete dataset is expected to be accessible after the study and all related publications are completed, and will remain accessible for at least 10 years after the data are made available publicly. The PIs and Co-Investigator acknowledge that each annual report must contain information about data accessibility, and that the timeframe of data accessibility will be reviewed as part of the annual progress reviews and revised as necessary for each publication.
Format of the final dataset
The format of the final dataset to be available for public access is as follows: De-identified raw paper data (e.g., student pre/posttest data) will be scanned into pdf files. Raw data collected electronically (e.g., via survey tools, field notes) will be available in MS Excel spreadsheets or pdf files. Raw data from audio/video files will be in .wav format. Audio/video materials and field notes from observations/interviews will also be transcribed and coded onto paper forms and scanned into pdf files. The final database will be in a .csv file that can be exported into MS Excel, SAS, SPSS, or ASCII files.
Dataset documentation to be provided
The final data file to be shared will include (a) raw item-level data (where applicable to recreate analyses) with appropriate variable and value labels, (b) all computed variables created during setup and scoring, and (c) all scale scores for the demographic, behavioral, and assessment data. These data will be the de-identified and individual- or aggregate-level data used for the final and published analyses.
Dataset documentation will consist of electronic codebooks documenting the following information: (a) a description of the research questions, methodology, and sample, (b) a description of each specific data source (e.g., measures, observation protocols), and (c) a description of the raw data and derived variables, including variable lists and definitions.
To aid in final dataset documentation, throughout the project, we will maintain a log of when, where, and how data were collected, decisions related to methods, coding, and analysis, statistical analyses, software and instruments used, where data and corresponding documentation are stored, and future research ideas and plans.
Method of data access
Final peer-reviewed publications resulting from the study/grant will be accompanied by the dataset used at the time of publication, during and after the grant period. A long-term data sharing and preservation plan will be used to store and make publicly accessible the data beyond the life of the project. The data will be deposited into the Data Repository for the University of Minnesota (DRUM), http://hdl.handle.net/11299/166578 . This University Libraries’ hosted institutional data repository is an open access platform for dissemination and archiving of university research data. Date files in DRUM are written to an Isilon storage system with two copies, one local to each of the two geographically separated University of Minnesota Data Centers. The local Isilon cluster stores the data in such a way that the data can survive the loss of any two disks or any one node of the cluster. Within two hours of the initial write, data replication to the 2nd Isilon cluster commences. The 2nd cluster employs the same protections as the local cluster, and both verify with a checksum procedure that data has not altered on write. In addition, DRUM provides long-term preservation of digital data files for at least 10 years using services such as migration (limited format types), secure backup, bit-level checksums, and maintains persistent DOIs for datasets, facilitating data citations. In accordance to DRUM policies, the de-identified data will be accompanied by the appropriate documentation, metadata, and code to facilitate reuse and provide the potential for interoperability with similar datasets.
The main benefit of DRUM is whatever is shared through this repository is public; however, a completely open system is not optimal if any of the data could be identifying (e.g., certain types of demographic data). We will work with the University of MN Library System to determine if DRUM is the best option. Another option available to the University of MN, ICPSR ( https://www.icpsr.umich.edu/icpsrweb/ ), would allow us to share data at different levels. Through ICPSR, data are available to researchers at member institutions of ICPSR rather than publicly. ICPSR allows for various mediated forms of sharing, where people interested in getting less de-identified individual level would sign data use agreements before receiving the data, or would need to use special software to access it directly from ICPSR rather than downloading it, for security proposes. ICPSR is a good option for sensitive or other kinds of data that are difficult to de-identify, but is not as open as DRUM. We expect that data for this project will be de-identifiable to a level that we can use DRUM, but will consider ICPSR as an option if needed.
Data agreement
No specific data sharing agreement will be needed if we use DRUM; however, DRUM does have a general end-user access policy ( conservancy.umn.edu/pages/drum/policies/#end-user-access-policy ). If we go with a less open access system such as ICPSR, we will work with ICPSR and the Un-funded Research Agreements (UFRA) coordinator at the University of Minnesota to develop necessary data sharing agreements.
Circumstances preventing data sharing
The data for this study fall under multiple statutes for confidentiality including multiple IRB requirements for confidentiality and FERPA. If it is not possible to meet all of the requirements of these agencies, data will not be shared.
For example, at the two sites where data will be collected, both universities (University of Minnesota and University of Missouri) and school districts have specific requirements for data confidentiality that will be described in consent forms. Participants will be informed of procedures used to maintain data confidentiality and that only de-identified data will be shared publicly. Some demographic data may not be sharable at the individual level and thus would only be provided in aggregate form.
When we collect audio/video data, participants will sign a release form that provides options to have data shared with project personnel only and/or for sharing purposes. We will not share audio/video data from people who do not consent to share it, and we will not publicly share any data that could identify an individual (these parameters will be specified in our IRB-approved informed consent forms). De-identifying is also required for FERPA data. The level of de-identification needed to meet these requirements is extensive, so it may not be possible to share all raw data exactly as collected in order to protect privacy of participants and maintain confidentiality of data.
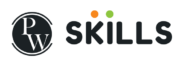
Data Analysis Techniques in Research – Methods, Tools & Examples
Varun Saharawat is a seasoned professional in the fields of SEO and content writing. With a profound knowledge of the intricate aspects of these disciplines, Varun has established himself as a valuable asset in the world of digital marketing and online content creation.
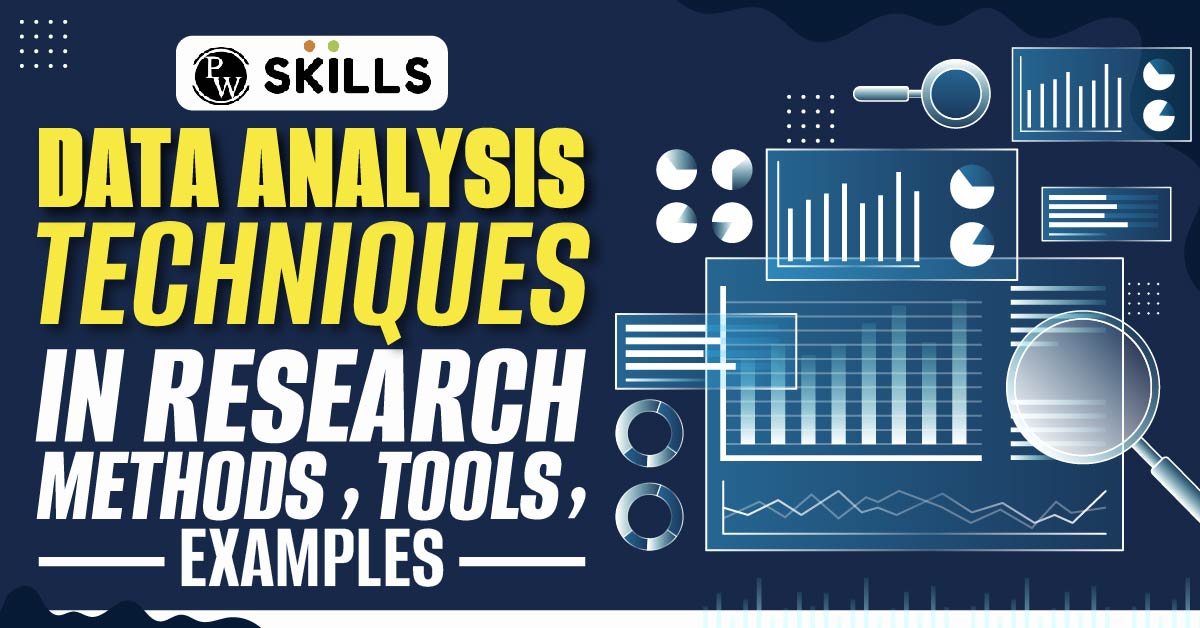
Data analysis techniques in research are essential because they allow researchers to derive meaningful insights from data sets to support their hypotheses or research objectives.
Data Analysis Techniques in Research : While various groups, institutions, and professionals may have diverse approaches to data analysis, a universal definition captures its essence. Data analysis involves refining, transforming, and interpreting raw data to derive actionable insights that guide informed decision-making for businesses.
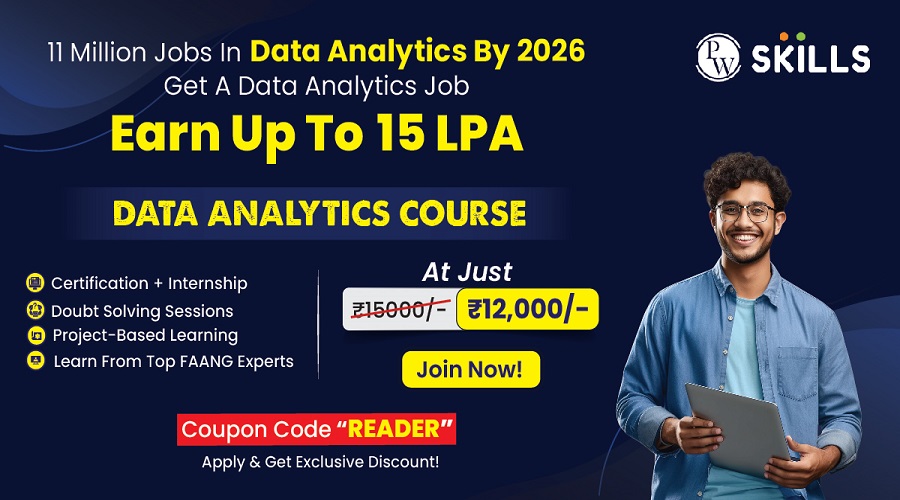
A straightforward illustration of data analysis emerges when we make everyday decisions, basing our choices on past experiences or predictions of potential outcomes.
If you want to learn more about this topic and acquire valuable skills that will set you apart in today’s data-driven world, we highly recommend enrolling in the Data Analytics Course by Physics Wallah . And as a special offer for our readers, use the coupon code “READER” to get a discount on this course.
Table of Contents
What is Data Analysis?
Data analysis is the systematic process of inspecting, cleaning, transforming, and interpreting data with the objective of discovering valuable insights and drawing meaningful conclusions. This process involves several steps:
- Inspecting : Initial examination of data to understand its structure, quality, and completeness.
- Cleaning : Removing errors, inconsistencies, or irrelevant information to ensure accurate analysis.
- Transforming : Converting data into a format suitable for analysis, such as normalization or aggregation.
- Interpreting : Analyzing the transformed data to identify patterns, trends, and relationships.
Types of Data Analysis Techniques in Research
Data analysis techniques in research are categorized into qualitative and quantitative methods, each with its specific approaches and tools. These techniques are instrumental in extracting meaningful insights, patterns, and relationships from data to support informed decision-making, validate hypotheses, and derive actionable recommendations. Below is an in-depth exploration of the various types of data analysis techniques commonly employed in research:
1) Qualitative Analysis:
Definition: Qualitative analysis focuses on understanding non-numerical data, such as opinions, concepts, or experiences, to derive insights into human behavior, attitudes, and perceptions.
- Content Analysis: Examines textual data, such as interview transcripts, articles, or open-ended survey responses, to identify themes, patterns, or trends.
- Narrative Analysis: Analyzes personal stories or narratives to understand individuals’ experiences, emotions, or perspectives.
- Ethnographic Studies: Involves observing and analyzing cultural practices, behaviors, and norms within specific communities or settings.
2) Quantitative Analysis:
Quantitative analysis emphasizes numerical data and employs statistical methods to explore relationships, patterns, and trends. It encompasses several approaches:
Descriptive Analysis:
- Frequency Distribution: Represents the number of occurrences of distinct values within a dataset.
- Central Tendency: Measures such as mean, median, and mode provide insights into the central values of a dataset.
- Dispersion: Techniques like variance and standard deviation indicate the spread or variability of data.
Diagnostic Analysis:
- Regression Analysis: Assesses the relationship between dependent and independent variables, enabling prediction or understanding causality.
- ANOVA (Analysis of Variance): Examines differences between groups to identify significant variations or effects.
Predictive Analysis:
- Time Series Forecasting: Uses historical data points to predict future trends or outcomes.
- Machine Learning Algorithms: Techniques like decision trees, random forests, and neural networks predict outcomes based on patterns in data.
Prescriptive Analysis:
- Optimization Models: Utilizes linear programming, integer programming, or other optimization techniques to identify the best solutions or strategies.
- Simulation: Mimics real-world scenarios to evaluate various strategies or decisions and determine optimal outcomes.
Specific Techniques:
- Monte Carlo Simulation: Models probabilistic outcomes to assess risk and uncertainty.
- Factor Analysis: Reduces the dimensionality of data by identifying underlying factors or components.
- Cohort Analysis: Studies specific groups or cohorts over time to understand trends, behaviors, or patterns within these groups.
- Cluster Analysis: Classifies objects or individuals into homogeneous groups or clusters based on similarities or attributes.
- Sentiment Analysis: Uses natural language processing and machine learning techniques to determine sentiment, emotions, or opinions from textual data.
Also Read: AI and Predictive Analytics: Examples, Tools, Uses, Ai Vs Predictive Analytics
Data Analysis Techniques in Research Examples
To provide a clearer understanding of how data analysis techniques are applied in research, let’s consider a hypothetical research study focused on evaluating the impact of online learning platforms on students’ academic performance.
Research Objective:
Determine if students using online learning platforms achieve higher academic performance compared to those relying solely on traditional classroom instruction.
Data Collection:
- Quantitative Data: Academic scores (grades) of students using online platforms and those using traditional classroom methods.
- Qualitative Data: Feedback from students regarding their learning experiences, challenges faced, and preferences.
Data Analysis Techniques Applied:
1) Descriptive Analysis:
- Calculate the mean, median, and mode of academic scores for both groups.
- Create frequency distributions to represent the distribution of grades in each group.
2) Diagnostic Analysis:
- Conduct an Analysis of Variance (ANOVA) to determine if there’s a statistically significant difference in academic scores between the two groups.
- Perform Regression Analysis to assess the relationship between the time spent on online platforms and academic performance.
3) Predictive Analysis:
- Utilize Time Series Forecasting to predict future academic performance trends based on historical data.
- Implement Machine Learning algorithms to develop a predictive model that identifies factors contributing to academic success on online platforms.
4) Prescriptive Analysis:
- Apply Optimization Models to identify the optimal combination of online learning resources (e.g., video lectures, interactive quizzes) that maximize academic performance.
- Use Simulation Techniques to evaluate different scenarios, such as varying student engagement levels with online resources, to determine the most effective strategies for improving learning outcomes.
5) Specific Techniques:
- Conduct Factor Analysis on qualitative feedback to identify common themes or factors influencing students’ perceptions and experiences with online learning.
- Perform Cluster Analysis to segment students based on their engagement levels, preferences, or academic outcomes, enabling targeted interventions or personalized learning strategies.
- Apply Sentiment Analysis on textual feedback to categorize students’ sentiments as positive, negative, or neutral regarding online learning experiences.
By applying a combination of qualitative and quantitative data analysis techniques, this research example aims to provide comprehensive insights into the effectiveness of online learning platforms.
Also Read: Learning Path to Become a Data Analyst in 2024
Data Analysis Techniques in Quantitative Research
Quantitative research involves collecting numerical data to examine relationships, test hypotheses, and make predictions. Various data analysis techniques are employed to interpret and draw conclusions from quantitative data. Here are some key data analysis techniques commonly used in quantitative research:
1) Descriptive Statistics:
- Description: Descriptive statistics are used to summarize and describe the main aspects of a dataset, such as central tendency (mean, median, mode), variability (range, variance, standard deviation), and distribution (skewness, kurtosis).
- Applications: Summarizing data, identifying patterns, and providing initial insights into the dataset.
2) Inferential Statistics:
- Description: Inferential statistics involve making predictions or inferences about a population based on a sample of data. This technique includes hypothesis testing, confidence intervals, t-tests, chi-square tests, analysis of variance (ANOVA), regression analysis, and correlation analysis.
- Applications: Testing hypotheses, making predictions, and generalizing findings from a sample to a larger population.
3) Regression Analysis:
- Description: Regression analysis is a statistical technique used to model and examine the relationship between a dependent variable and one or more independent variables. Linear regression, multiple regression, logistic regression, and nonlinear regression are common types of regression analysis .
- Applications: Predicting outcomes, identifying relationships between variables, and understanding the impact of independent variables on the dependent variable.
4) Correlation Analysis:
- Description: Correlation analysis is used to measure and assess the strength and direction of the relationship between two or more variables. The Pearson correlation coefficient, Spearman rank correlation coefficient, and Kendall’s tau are commonly used measures of correlation.
- Applications: Identifying associations between variables and assessing the degree and nature of the relationship.
5) Factor Analysis:
- Description: Factor analysis is a multivariate statistical technique used to identify and analyze underlying relationships or factors among a set of observed variables. It helps in reducing the dimensionality of data and identifying latent variables or constructs.
- Applications: Identifying underlying factors or constructs, simplifying data structures, and understanding the underlying relationships among variables.
6) Time Series Analysis:
- Description: Time series analysis involves analyzing data collected or recorded over a specific period at regular intervals to identify patterns, trends, and seasonality. Techniques such as moving averages, exponential smoothing, autoregressive integrated moving average (ARIMA), and Fourier analysis are used.
- Applications: Forecasting future trends, analyzing seasonal patterns, and understanding time-dependent relationships in data.
7) ANOVA (Analysis of Variance):
- Description: Analysis of variance (ANOVA) is a statistical technique used to analyze and compare the means of two or more groups or treatments to determine if they are statistically different from each other. One-way ANOVA, two-way ANOVA, and MANOVA (Multivariate Analysis of Variance) are common types of ANOVA.
- Applications: Comparing group means, testing hypotheses, and determining the effects of categorical independent variables on a continuous dependent variable.
8) Chi-Square Tests:
- Description: Chi-square tests are non-parametric statistical tests used to assess the association between categorical variables in a contingency table. The Chi-square test of independence, goodness-of-fit test, and test of homogeneity are common chi-square tests.
- Applications: Testing relationships between categorical variables, assessing goodness-of-fit, and evaluating independence.
These quantitative data analysis techniques provide researchers with valuable tools and methods to analyze, interpret, and derive meaningful insights from numerical data. The selection of a specific technique often depends on the research objectives, the nature of the data, and the underlying assumptions of the statistical methods being used.
Also Read: Analysis vs. Analytics: How Are They Different?
Data Analysis Methods
Data analysis methods refer to the techniques and procedures used to analyze, interpret, and draw conclusions from data. These methods are essential for transforming raw data into meaningful insights, facilitating decision-making processes, and driving strategies across various fields. Here are some common data analysis methods:
- Description: Descriptive statistics summarize and organize data to provide a clear and concise overview of the dataset. Measures such as mean, median, mode, range, variance, and standard deviation are commonly used.
- Description: Inferential statistics involve making predictions or inferences about a population based on a sample of data. Techniques such as hypothesis testing, confidence intervals, and regression analysis are used.
3) Exploratory Data Analysis (EDA):
- Description: EDA techniques involve visually exploring and analyzing data to discover patterns, relationships, anomalies, and insights. Methods such as scatter plots, histograms, box plots, and correlation matrices are utilized.
- Applications: Identifying trends, patterns, outliers, and relationships within the dataset.
4) Predictive Analytics:
- Description: Predictive analytics use statistical algorithms and machine learning techniques to analyze historical data and make predictions about future events or outcomes. Techniques such as regression analysis, time series forecasting, and machine learning algorithms (e.g., decision trees, random forests, neural networks) are employed.
- Applications: Forecasting future trends, predicting outcomes, and identifying potential risks or opportunities.
5) Prescriptive Analytics:
- Description: Prescriptive analytics involve analyzing data to recommend actions or strategies that optimize specific objectives or outcomes. Optimization techniques, simulation models, and decision-making algorithms are utilized.
- Applications: Recommending optimal strategies, decision-making support, and resource allocation.
6) Qualitative Data Analysis:
- Description: Qualitative data analysis involves analyzing non-numerical data, such as text, images, videos, or audio, to identify themes, patterns, and insights. Methods such as content analysis, thematic analysis, and narrative analysis are used.
- Applications: Understanding human behavior, attitudes, perceptions, and experiences.
7) Big Data Analytics:
- Description: Big data analytics methods are designed to analyze large volumes of structured and unstructured data to extract valuable insights. Technologies such as Hadoop, Spark, and NoSQL databases are used to process and analyze big data.
- Applications: Analyzing large datasets, identifying trends, patterns, and insights from big data sources.
8) Text Analytics:
- Description: Text analytics methods involve analyzing textual data, such as customer reviews, social media posts, emails, and documents, to extract meaningful information and insights. Techniques such as sentiment analysis, text mining, and natural language processing (NLP) are used.
- Applications: Analyzing customer feedback, monitoring brand reputation, and extracting insights from textual data sources.
These data analysis methods are instrumental in transforming data into actionable insights, informing decision-making processes, and driving organizational success across various sectors, including business, healthcare, finance, marketing, and research. The selection of a specific method often depends on the nature of the data, the research objectives, and the analytical requirements of the project or organization.
Also Read: Quantitative Data Analysis: Types, Analysis & Examples
Data Analysis Tools
Data analysis tools are essential instruments that facilitate the process of examining, cleaning, transforming, and modeling data to uncover useful information, make informed decisions, and drive strategies. Here are some prominent data analysis tools widely used across various industries:
1) Microsoft Excel:
- Description: A spreadsheet software that offers basic to advanced data analysis features, including pivot tables, data visualization tools, and statistical functions.
- Applications: Data cleaning, basic statistical analysis, visualization, and reporting.
2) R Programming Language:
- Description: An open-source programming language specifically designed for statistical computing and data visualization.
- Applications: Advanced statistical analysis, data manipulation, visualization, and machine learning.
3) Python (with Libraries like Pandas, NumPy, Matplotlib, and Seaborn):
- Description: A versatile programming language with libraries that support data manipulation, analysis, and visualization.
- Applications: Data cleaning, statistical analysis, machine learning, and data visualization.
4) SPSS (Statistical Package for the Social Sciences):
- Description: A comprehensive statistical software suite used for data analysis, data mining, and predictive analytics.
- Applications: Descriptive statistics, hypothesis testing, regression analysis, and advanced analytics.
5) SAS (Statistical Analysis System):
- Description: A software suite used for advanced analytics, multivariate analysis, and predictive modeling.
- Applications: Data management, statistical analysis, predictive modeling, and business intelligence.
6) Tableau:
- Description: A data visualization tool that allows users to create interactive and shareable dashboards and reports.
- Applications: Data visualization , business intelligence , and interactive dashboard creation.
7) Power BI:
- Description: A business analytics tool developed by Microsoft that provides interactive visualizations and business intelligence capabilities.
- Applications: Data visualization, business intelligence, reporting, and dashboard creation.
8) SQL (Structured Query Language) Databases (e.g., MySQL, PostgreSQL, Microsoft SQL Server):
- Description: Database management systems that support data storage, retrieval, and manipulation using SQL queries.
- Applications: Data retrieval, data cleaning, data transformation, and database management.
9) Apache Spark:
- Description: A fast and general-purpose distributed computing system designed for big data processing and analytics.
- Applications: Big data processing, machine learning, data streaming, and real-time analytics.
10) IBM SPSS Modeler:
- Description: A data mining software application used for building predictive models and conducting advanced analytics.
- Applications: Predictive modeling, data mining, statistical analysis, and decision optimization.
These tools serve various purposes and cater to different data analysis needs, from basic statistical analysis and data visualization to advanced analytics, machine learning, and big data processing. The choice of a specific tool often depends on the nature of the data, the complexity of the analysis, and the specific requirements of the project or organization.
Also Read: How to Analyze Survey Data: Methods & Examples
Importance of Data Analysis in Research
The importance of data analysis in research cannot be overstated; it serves as the backbone of any scientific investigation or study. Here are several key reasons why data analysis is crucial in the research process:
- Data analysis helps ensure that the results obtained are valid and reliable. By systematically examining the data, researchers can identify any inconsistencies or anomalies that may affect the credibility of the findings.
- Effective data analysis provides researchers with the necessary information to make informed decisions. By interpreting the collected data, researchers can draw conclusions, make predictions, or formulate recommendations based on evidence rather than intuition or guesswork.
- Data analysis allows researchers to identify patterns, trends, and relationships within the data. This can lead to a deeper understanding of the research topic, enabling researchers to uncover insights that may not be immediately apparent.
- In empirical research, data analysis plays a critical role in testing hypotheses. Researchers collect data to either support or refute their hypotheses, and data analysis provides the tools and techniques to evaluate these hypotheses rigorously.
- Transparent and well-executed data analysis enhances the credibility of research findings. By clearly documenting the data analysis methods and procedures, researchers allow others to replicate the study, thereby contributing to the reproducibility of research findings.
- In fields such as business or healthcare, data analysis helps organizations allocate resources more efficiently. By analyzing data on consumer behavior, market trends, or patient outcomes, organizations can make strategic decisions about resource allocation, budgeting, and planning.
- In public policy and social sciences, data analysis is instrumental in developing and evaluating policies and interventions. By analyzing data on social, economic, or environmental factors, policymakers can assess the effectiveness of existing policies and inform the development of new ones.
- Data analysis allows for continuous improvement in research methods and practices. By analyzing past research projects, identifying areas for improvement, and implementing changes based on data-driven insights, researchers can refine their approaches and enhance the quality of future research endeavors.
However, it is important to remember that mastering these techniques requires practice and continuous learning. That’s why we highly recommend the Data Analytics Course by Physics Wallah . Not only does it cover all the fundamentals of data analysis, but it also provides hands-on experience with various tools such as Excel, Python, and Tableau. Plus, if you use the “ READER ” coupon code at checkout, you can get a special discount on the course.
For Latest Tech Related Information, Join Our Official Free Telegram Group : PW Skills Telegram Group
Data Analysis Techniques in Research FAQs
What are the 5 techniques for data analysis.
The five techniques for data analysis include: Descriptive Analysis Diagnostic Analysis Predictive Analysis Prescriptive Analysis Qualitative Analysis
What are techniques of data analysis in research?
Techniques of data analysis in research encompass both qualitative and quantitative methods. These techniques involve processes like summarizing raw data, investigating causes of events, forecasting future outcomes, offering recommendations based on predictions, and examining non-numerical data to understand concepts or experiences.
What are the 3 methods of data analysis?
The three primary methods of data analysis are: Qualitative Analysis Quantitative Analysis Mixed-Methods Analysis
What are the four types of data analysis techniques?
The four types of data analysis techniques are: Descriptive Analysis Diagnostic Analysis Predictive Analysis Prescriptive Analysis
- Top 15 SAS Courses For Aspiring Data Analysts
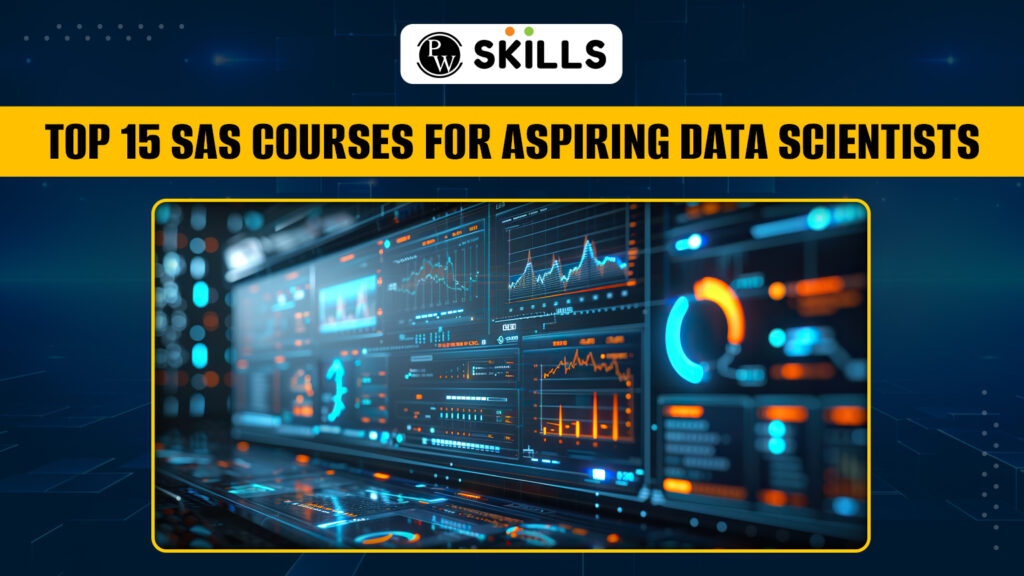
The top 15 SAS Courses for aspiring data scientists include- 1. SAS Professional Programmer Certificate, 2. SAS Advanced Programmer Certificate…
- How To Become Data Analyst In 2023
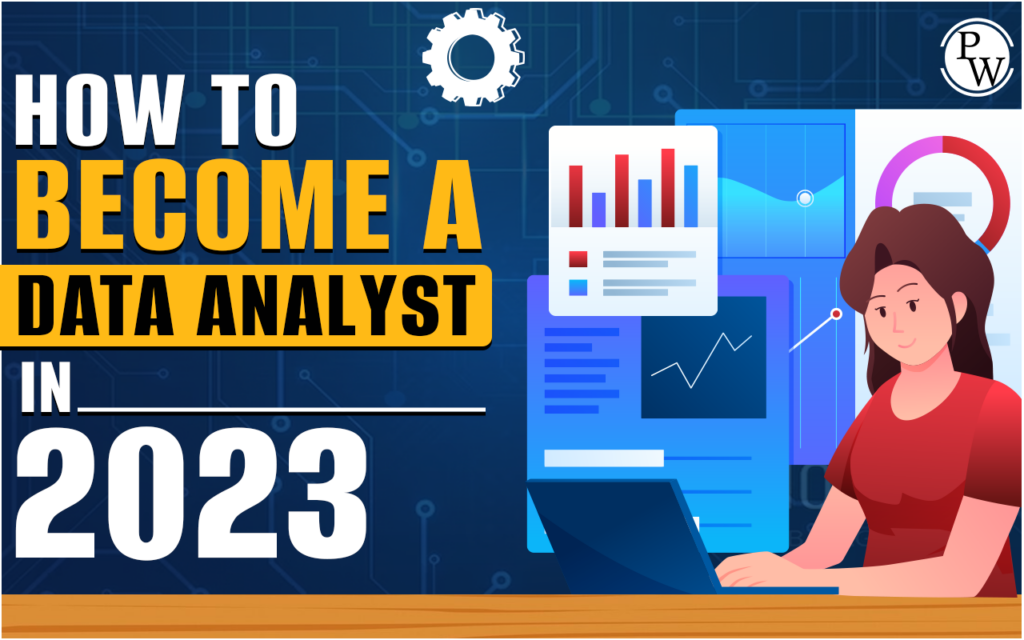
Data Analyst in 2023: In 2023, becoming a data analyst is not just a career choice but is also the…
- Top 25 Big Data Interview Questions and Answers
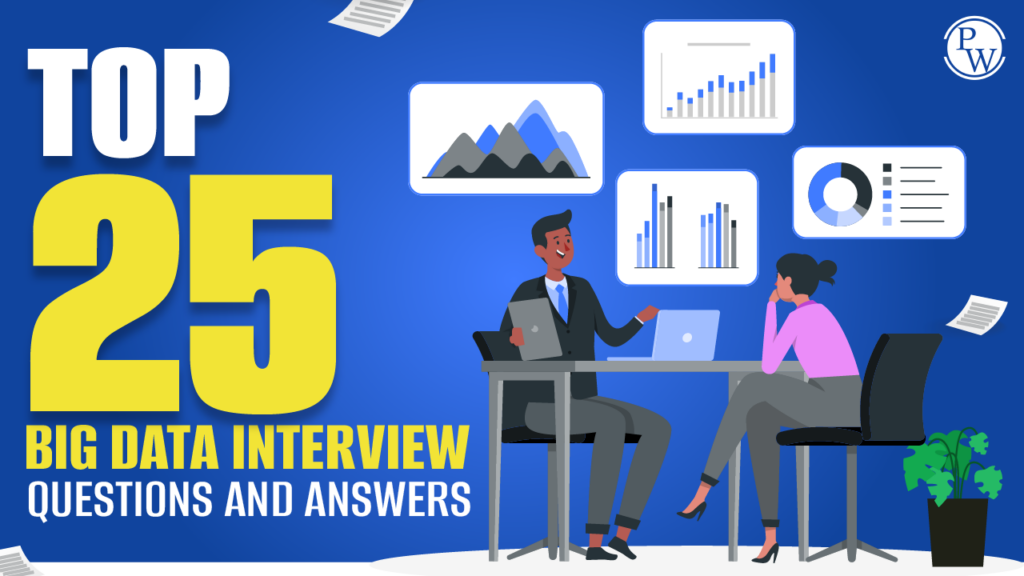
Big Data Interview Questions and Answers: In the fast-paced digital age, data multiplies rapidly. Big Data is the hidden hero,…
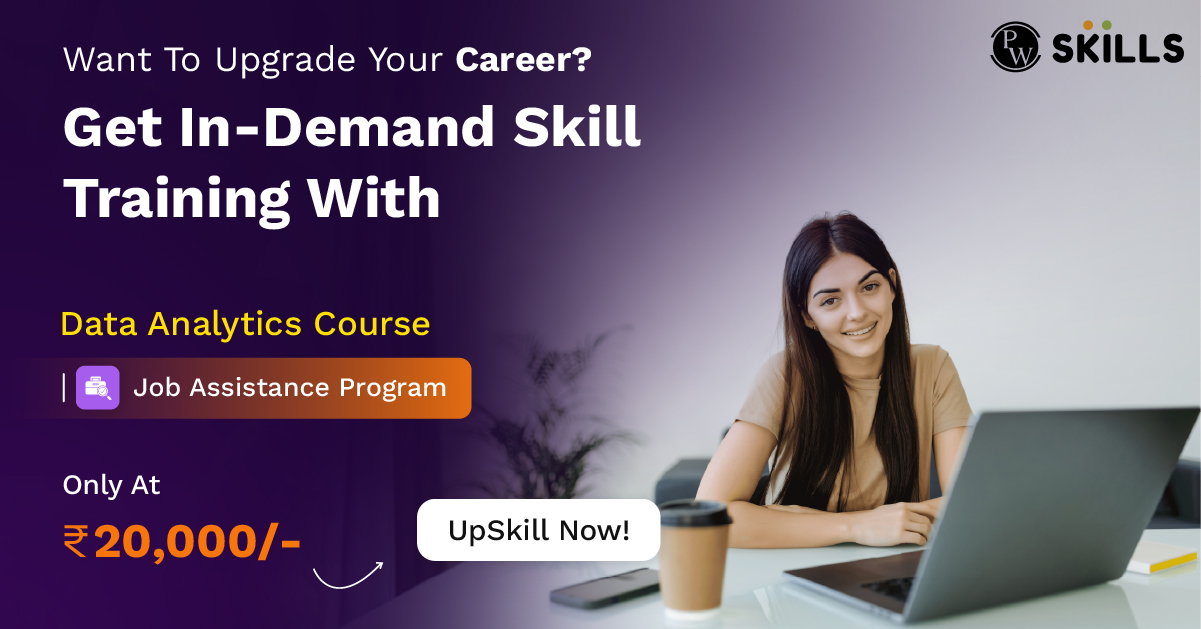
Related Articles
- 10 Best Companies For Data Analysis Internships 2024
- Finance Data Analysis: What is a Financial Data Analysis?
- Data Analytics Meaning, Importance, Techniques, Examples
- 5 BI Business Intelligence Tools You Must Know in 2024
- What Is Business BI?
- What Is Predictive Data Analytics, Definition, Tools, How Does It Work?
- What Is Big Data Analytics? Definition, Benefits, and More
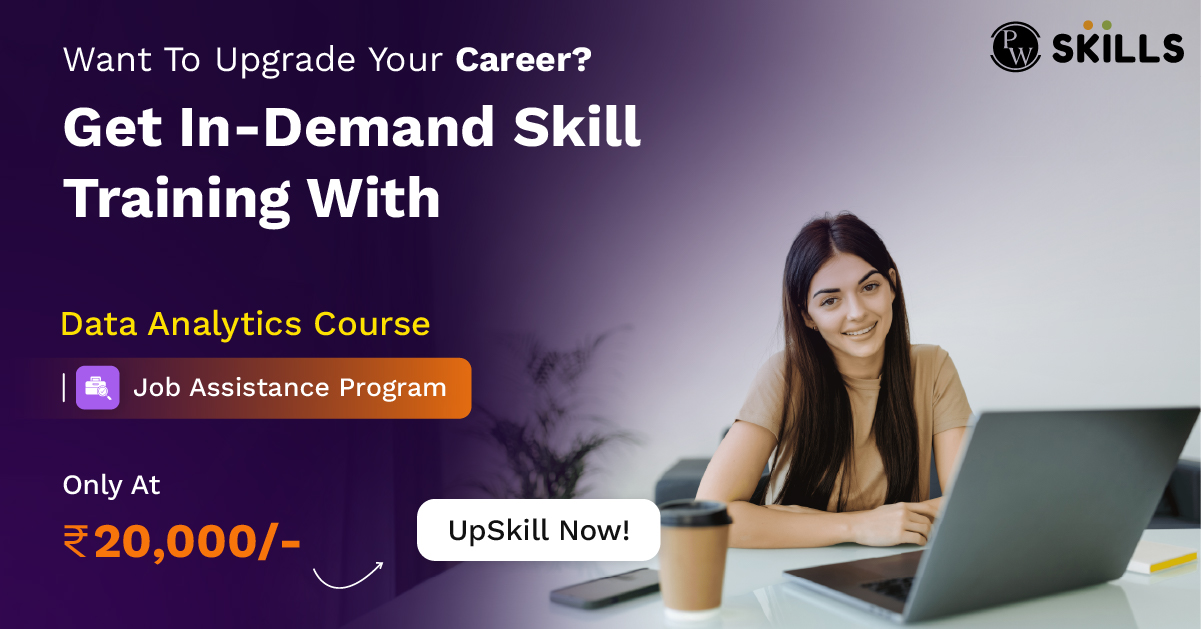
Ask Yale Library
My Library Accounts
Find, Request, and Use
Help and Research Support
Visit and Study
Explore Collections
Research Data Management: Plan for Data
- Plan for Data
- Organize & Document Data
- Store & Secure Data
- Validate Data
- Share & Re-use Data
- Data Use Agreements
- Research Data Policies
What is a Data Management Plan?
Data management plans (DMPs) are documents that outline how data will be collected , stored , secured , analyzed , disseminated , and preserved over the lifecycle of a research project. They are typically created in the early stages of a project, and they are typically short documents that may evolve over time. Increasingly, they are required by funders and institutions alike, and they are a recommended best practice in research data management.
Tab through this guide to consider each stage of the research data management process, and each correlated section of a data management plan.
Tools for Data Management Planning
DMPTool is a collaborative effort between several universities to streamline the data management planning process.
The DMPTool supports the majority of federal and many non-profit and private funding agencies that require data management plans as part of a grant proposal application. ( View the list of supported organizations and corresponding templates.) If the funder you're applying to isn't listed or you just want to create one as good practice, there is an option for a generic plan.
Key features:
Data management plan templates from most major funders
Guided creation of a data management plan with click-throughs and helpful questions and examples
Access to public plans , to review ahead of creating your own
Ability to share plans with collaborators as well as copy and reuse existing plans
How to get started:
Log in with your yale.edu email to be directed to a NetID sign-in, and review the quick start guide .
Research Data Lifecycle
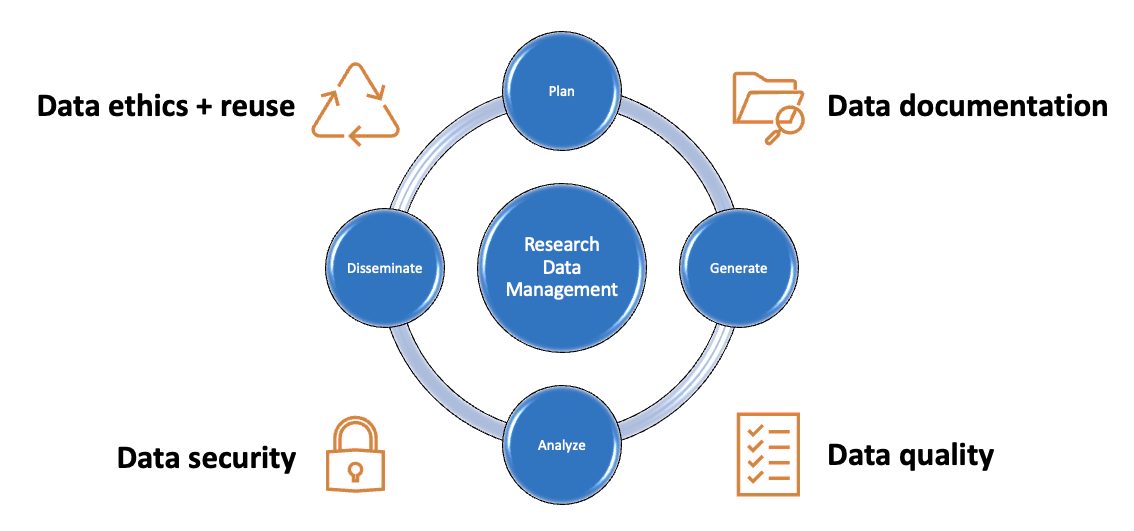
Additional Resources for Data Management Planning
- << Previous: Overview
- Next: Organize & Document Data >>
- Last Updated: Sep 27, 2023 1:15 PM
- URL: https://guides.library.yale.edu/datamanagement

Site Navigation
P.O. BOX 208240 New Haven, CT 06250-8240 (203) 432-1775
Yale's Libraries
Bass Library
Beinecke Rare Book and Manuscript Library
Classics Library
Cushing/Whitney Medical Library
Divinity Library
East Asia Library
Gilmore Music Library
Haas Family Arts Library
Lewis Walpole Library
Lillian Goldman Law Library
Marx Science and Social Science Library
Sterling Memorial Library
Yale Center for British Art
SUBSCRIBE TO OUR NEWSLETTER
@YALELIBRARY

Yale Library Instagram
Accessibility Diversity, Equity, and Inclusion Giving Privacy and Data Use Contact Our Web Team
© 2022 Yale University Library • All Rights Reserved
- Data Management Plans
What is a Data Management Plan (DMP)?
For nih (recently updated), what about other government agencies, writing a plan, having a plan reviewed, what generally goes into a dmp, anticipate the storage, infrastructure, and software needs of the project, create or adopt standard terminology and file-naming practices, set a schedule for your data management activities, assign responsibilities, think long-term.
A DMP (or DMSP, Data Management and Sharing Plan) describes what data will be acquired or generated as part of a research project, how the data will be managed, described, analyzed, and stored, and what mechanisms will be used to at the end of your project to share and preserve the data.
One of the key advantages to writing a DMP is that it helps you think concretely about your process, identify potential weaknesses in your plans, and provide a record of what you intend to do. Developing a DMP can prompt valuable discussion among collaborators that uncovers and resolves unspoken assumptions, and provide a framework for documentation that keeps graduate students, postdocs, and collaborators on the same page with respect to practices, expectations, and policies.
Data management planning is most effective in the early stages of a research project, but it is never too late to develop a data management plan.
How can I find out what my funding agency requires?
Most funding agencies require a DMP as part of an application for funding, but the specific requirements differ across and even within agencies. Many agencies, including the NSF and NIH, have requirements that apply generally, with some additional considerations depending on the specific funding announcement or the directorate/institute.
Here are some resources to help identify what you’ll need:
- DMP Requirements (PAPPG, Chapter 2, Proposal prep instructions)
- Data sharing policy (PAPPG, Chapter 11, Post-award requirements)
- Links to directorate-specific requirements
- Data Management and Sharing Policy Overview
- Research Covered Under the Data Management and Sharing Policy
- Writing a Data Management and Sharing Plan
- Final NIH Policy for Data Management and Sharing
- NOTE : Some specific NIH Institutes, Centers, or Offices have additional requirements for DMSPs. For example, applications to NIMH require a data validation schedule. Please check with your institute and your funding announcement to ensure all aspects expected are included in your DMSP.
- Data Sharing Policies
- General guidance and examples
- Data Sharing and Management Policies for US Federal Agencies
Need help figuring out what your agency needs? Ask a PRDS team member !
Where can I get help with writing a DMP?
With recent and upcoming changes to the research landscape, it can be tricky to determine what information is needed for your Data Management (and Sharing) Plan. As a Princeton researcher, you have several ways of obtaining support in this area
You have free access to an online tool for writing DMPs: DMPTool . You just need to sign in as a Princeton researcher, and you’ll be able to use and adapt templates, example DMPs, and Princeton-specific guidance. You can find some helpful public guidance on using DMPTool created by Arizona State University.
You are also welcome to schedule an appointment with a member of the PRDS team. While we are unable to write your DMP for you, we are happy to review your funding call and guide you through the information you will need to provide as part of your DMP
PRDS also offers free and confidential feedback on draft DMPs. If you would like to request feedback, we require:
- Your draft DMP (either via email [[email protected]] or by selecting the “Request Feedback” option on the last page of your DMP template in the DMPTool ).
- Your funding announcement.
- Your deadline to submit your grant proposal.
NOTE: Reviewing DMPs is a process and may involve several rounds of edits or a conversation between you and our team. The timeline for requesting a DMP review is as follows:
- Single-lab or single-PI grants: no fewer than 5 business days ;
- Complex, multi-institution grants, including Centers: no fewer than 10 business days .
We will make every effort to review all DMPs submitted to us, however, we cannot guarantee a thorough review if submitted after our requested time frame.
Details will vary from funder to funder, but the Digital Curation Centre’s Checklist for a Data Management Plan provides a useful list of questions to consider when writing a DMP:
- What data will you collect or create? Type of data, e.g., observation, experimental, simulation, derived/compiled Form of data, e.g., text, numeric, audiovisual, discipline- or instrument-specific File Formats, ideally using research community standards or open format (e.g., txt, csv, pdf, jpg)
- How will the data be collected or created?
- What documentation and metadata will accompany the data?
- How will you manage any ethical issues?
- How will you manage copyright and intellectual property rights issues?
- How will the data be stored and backed up during research?
- How will you manage access and security?
- Which data should be retained, shared, and/or preserved?
- What is the long-term preservation plan for the dataset?
- How will you share the data?
- Are any restrictions on data sharing required?
- Who will be responsible for data management?
- What resources will you require to implement your plan?
Additional key things to consider
Consider the types of data that will be created or used in the project. For example, will your project…
- generate large amounts of data?
- require coordinated effort between offsite collaborators?
- use data that has licensing agreements or other restrictions on its use?
- Involve human or non-human animal subjects?
Answers to questions like these will help you accurately assess what you’ll need during the project and prevent delays during crucial stages.
Decide on file and directory naming conventions and stick to them. Document them (either independently or as part of a standard operating procedure (SOP) document) so that any new graduate students, post-docs, or collaborators can transition smoothly into the project.
Plan and implement a back-up schedule onto shared storage in order to ensure that more than one copy of the data exists. Periodic file and/or directory clean-ups will help keep “publication quality” data safe and accessible.
Make it clear who is responsible for what. For example, assign a data manager who can check that backup clients are functional, monitor shared directories for clean-up or archiving maintenance, and follow up with project members as needed.
Decide where your data will go after the end of the project. Data that are associated with publications need to be preserved long-term, and so it’s good to decide early on where the data will be stored (e.g. a discipline or institutional repository) and when and how it will get there. Other data may need this level of preservation as well. PRDS can help you find places to store your data and provide advice about what kinds of data to plan to keep.
- Events and Training
- Getting Started
- Guides for Good Data Management
- Data Curation
- Data Ownership
- Large Data Resources
- Data Use Agreements
- Human Subjects
- Finding Datasets
- Non-Human Animal Subjects
- Data Security
- File Organization
- READMEs for Research Data
- Research Computing Resources
- Collaboration Options
- Help with Statistics
- Publisher Requirements
- Data Repositories
- Princeton Data Commons
- Getting Started as a Princeton Data Commons Describe Contributor
- Publishing Large Datasets
- Using Globus with Princeton Data Commons (PDC)
- Storage Options
- Tools and Resources
- Data Management in Research: A Comprehensive Guide
Research Data Management (RDM) is an important part of any research project. Learn about RDM examples from University of Minnesota & how it helps researchers manage & share their research data.

Research data management (RDM) is a term that describes the organization, storage, preservation, and sharing of data collected and used in a research project. It involves the daily management of research data throughout the life of a research project, such as using consistent file naming conventions. Examples of data management plans (DMPs) can be found in universities, such as the University of Minnesota . These plans can be concise or detailed, depending on the type of data used (secondary or primary).
Research data can include video, sound, or text data, as long as it is used for systematic analysis. For example, a collection of video interviews used to collect and identify facial gestures and expressions in a study of emotional responses to stimuli would be considered research data. Making this data accessible to everyone in the group, even those who are not on the team but who are in the same discipline, can open up enormous opportunities to advance their own research. The Stata logging function can record all activities and store them in the relevant designated folders.
Qualitative data is subjective and exists only in relation to the observer (McLeod, 201). The Stanford Digital Repository (SDR) provides digital preservation, hosting and access services that allow researchers to preserve, manage and share research data in a secure environment for citation, access and long-term reuse. In addition, DRUM provides long-term preservation of digital data files for at least 10 years using services such as migration (limited format types), secure backups, bit-level checksums, and maintains persistent DOIs for data sets. The DMPTool includes data management plan templates along with a wealth of information and assistance to guide you through the process of creating a ready-to-use DMP for your specific research project and funding agency.
Some demographics may not be shareable on an individual level and would therefore only be provided in aggregate form. In accordance with DRUM policies, unidentified data will be accompanied by appropriate documentation, metadata and code to facilitate reuse and provide the potential for interoperability with similar data sets. It is important to remember that you can generate data at any point in your research but if you don't document it properly it will become useless. For example, observation data should be recorded immediately to avoid data loss while reference data is not as time-sensitive.
Research data management describes a way to organize and store the data that a research project has accumulated in the most efficient way possible. The willingness of researchers to manage and share their data has been evolving under increasing pressure from government mandates of the National Institutes of Health and the data exchange policies of major publishers that now require researchers to share their data and the processes they took to collect the data if they want to continue receiving funding or have their articles published. Librarians have begun to provide a range of services in this area and are now teaching data management to researchers, working with individual researchers to improve their data management practices, create thematic data management guides, and help support agencies' data requirements funding and publishers. Some operating systems also support embedding metadata in this way, such as Microsoft Document Properties.
Related Posts

- The Benefits of Data Management in Biology
Data management in biology is an essential process that involves the acquisition, modeling, storage, integration, analysis, and interpretation of various types of data. Learn more about its benefits & best practices here.

- What are the data management principles?
The first and most important guiding principle of data management is data modeling. One of the most important data management principles is to develop a data management plan.

- The Benefits of Good Data Management Explained
Data management helps minimize potential errors by establishing usage processes and policies and building trust in the data used to make decisions across the organization. Learn more about how good MDM can improve transaction efficiency.

- The Benefits of Data Management for Businesses
Data management is an essential practice for businesses of all sizes. It helps minimize potential errors by establishing usage processes & policies while ensuring accuracy & protection. Learn how industries around the world are using it.
More articles

- Data Management Services: A Comprehensive Guide

- Who will approve data management plan?

Data Management: A Comprehensive Guide

- Data Management: An Overview of the 5 Key Functions
- What is the Role of Data Management in Organizations?
- What are the 5 basic steps in data analysis?
- Data Management Softwares: A Comprehensive Guide
- A Comprehensive Guide to Data Management Plans
- What are data management skills?
- The Benefits of Data Management Tools
- Types of Data Management Functions Explained
- What is Data Services and How Does it Work?
- Data Management: Unlocking the Potential of Your Data
- Data Management Plans: A Comprehensive Guide for Researchers
- The Benefits of Enterprise Data Management for Businesses
- The Ultimate Guide to Enterprise Data Management
- Data Management: What You Need to Know
- Data Management: What is it and How to Implement it?
- Why is tools management important?
- The Ultimate Guide to Data Management
- The Benefits of Data Management: Unlocking the Value of Data
- 6 Major Areas for Best Practices in Data Management
- What should a data management plan include?
- The Benefits of Enterprise Data Management for Every Enterprise
- What is a data principle?
- The Essential Role of Data Management in Business
- The Advantages of Enterprise Data Management
- Who created data management?
- What are the 5 importance of data processing?
- The Benefits of Data Management in Research
- Data Management Tools: A Comprehensive Guide
- What are the best practices in big data adoption?
- Types of Management Tools: A Comprehensive Guide
- Data Management in Quantitative Research: An Expert's Guide
- Data Management: A Comprehensive Guide to Maximize Benefits
- How is big data used in biology?
- The Benefits of Data Management in Research: A Comprehensive Guide
- The Essential Role of Enterprise Data Management
- A Comprehensive Guide to Different Types of Data Management
- What is managing the data?
- What is a data standard?
- What are data standards and interoperability?
- What software create and manage data base?
- Which of the following is api management tool?
- Types of Data in Research: A Comprehensive Guide
- A Comprehensive Guide to Writing a Data Management Research Plan
- What Are the Different Types of Management Tools?
Data Processing: Exploring the 3 Main Methods
- The Benefits of Research Data Management
- What tools are used in data analysis?
- 9 Best Practices of Master Data Management
- What is data management framework in d365?
- How do you create a data standard?
- Data Management: A Comprehensive Guide to Activities Involved
- 10 Steps to Master Data Management
- What is Enterprise Data Management and How Can It Help Your Organization?
- Data Management Standards: A Comprehensive Guide
- Data Management: A Comprehensive Guide to Unlocking Data Potential
- Data Management Best Practices: A Comprehensive Guide
- The Benefits of Data Management: Unlocking the Potential of Data
- Why is data management and analysis important?
- The Difference Between Enterprise Data Management and Data Management
- The Benefits of Understanding Project Data and Processes
- Data Management Principles: A Comprehensive Guide
- What is Enterprise Data Management Framework?
- What is a value in data management?
- Data Management Tools: An Expert's Guide
- The Benefits of Enterprise Data Management
- What are database systems?
- Data Management: Functions, Storage, Security and More
- Four Essential Best Practices for Big Data Governance
- Data Management: What is it and How to Use it?
- What is the Purpose of a Data Management Plan?
- The Benefits of Data Management: Unlocking the Power of Your Data
- Data Management: Unlocking the Potential of Data
- How does data governance add value?
- A Comprehensive Guide to Database Management Systems
- 4 Types of Data Management Explained
- Data Management: Unlocking the Power of Data Analysis
- Data Management in Research Studies: An Expert's Guide
- The Significance of Data Management in Mathematics
- What is data standard?
- Do You Need Math to Become a Data Scientist?
- What is Data Management and How to Manage it Effectively?
- Data Governance vs Data Management: What's the Difference?
New Articles

Which cookies do you want to accept?
What is Data Analysis? An Expert Guide With Examples
What is data analysis.
Data analysis is a comprehensive method of inspecting, cleansing, transforming, and modeling data to discover useful information, draw conclusions, and support decision-making. It is a multifaceted process involving various techniques and methodologies to interpret data from various sources in different formats, both structured and unstructured.
Data analysis is not just a mere process; it's a tool that empowers organizations to make informed decisions, predict trends, and improve operational efficiency. It's the backbone of strategic planning in businesses, governments, and other organizations.
Consider the example of a leading e-commerce company. Through data analysis, they can understand their customers' buying behavior, preferences, and patterns. They can then use this information to personalize customer experiences, forecast sales, and optimize marketing strategies, ultimately driving business growth and customer satisfaction.
Learn more about how to become a data analyst in our separate article, which covers everything you need to know about launching your career in this field and the skills you’ll need to master.
AI Upskilling for Beginners
The importance of data analysis in today's digital world.
In the era of digital transformation, data analysis has become more critical than ever. The explosion of data generated by digital technologies has led to the advent of what we now call 'big data.' This vast amount of data, if analyzed correctly, can provide invaluable insights that can revolutionize businesses.
Data analysis is the key to unlocking the potential of big data. It helps organizations to make sense of this data, turning it into actionable insights. These insights can be used to improve products and services, enhance experiences, streamline operations, and increase profitability.
A good example is the healthcare industry . Through data analysis, healthcare providers can predict disease outbreaks, improve patient care, and make informed decisions about treatment strategies. Similarly, in the finance sector, data analysis can help in risk assessment, fraud detection, and investment decision-making.
The Data Analysis Process: A Step-by-Step Guide
The process of data analysis is a systematic approach that involves several stages, each crucial to ensuring the accuracy and usefulness of the results. Here, we'll walk you through each step, from defining objectives to data storytelling. You can learn more about how businesses analyze data in a separate guide.

The data analysis process in a nutshell
Step 1: Defining objectives and questions
The first step in the data analysis process is to define the objectives and formulate clear, specific questions that your analysis aims to answer. This step is crucial as it sets the direction for the entire process. It involves understanding the problem or situation at hand, identifying the data needed to address it, and defining the metrics or indicators to measure the outcomes.
Step 2: Data collection
Once the objectives and questions are defined, the next step is to collect the relevant data. This can be done through various methods such as surveys, interviews, observations, or extracting from existing databases. The data collected can be quantitative (numerical) or qualitative (non-numerical), depending on the nature of the problem and the questions being asked.
Step 3: Data cleaning
Data cleaning, also known as data cleansing, is a critical step in the data analysis process. It involves checking the data for errors and inconsistencies, and correcting or removing them. This step ensures the quality and reliability of the data, which is crucial for obtaining accurate and meaningful results from the analysis.
Step 4: Data analysis
Once the data is cleaned, it's time for the actual analysis. This involves applying statistical or mathematical techniques to the data to discover patterns, relationships, or trends. There are various tools and software available for this purpose, such as Python, R, Excel, and specialized software like SPSS and SAS.
Step 5: Data interpretation and visualization
After the data is analyzed, the next step is to interpret the results and visualize them in a way that is easy to understand. This could involve creating charts, graphs, or other visual representations of the data. Data visualization helps to make complex data more understandable and provides a clear picture of the findings.
Step 6: Data storytelling
The final step in the data analysis process is data storytelling. This involves presenting the findings of the analysis in a narrative form that is engaging and easy to understand. Data storytelling is crucial for communicating the results to non-technical audiences and for making data-driven decisions.
The Types of Data Analysis
Data analysis can be categorized into four main types, each serving a unique purpose and providing different insights. These are descriptive, diagnostic, predictive, and prescriptive analyses.
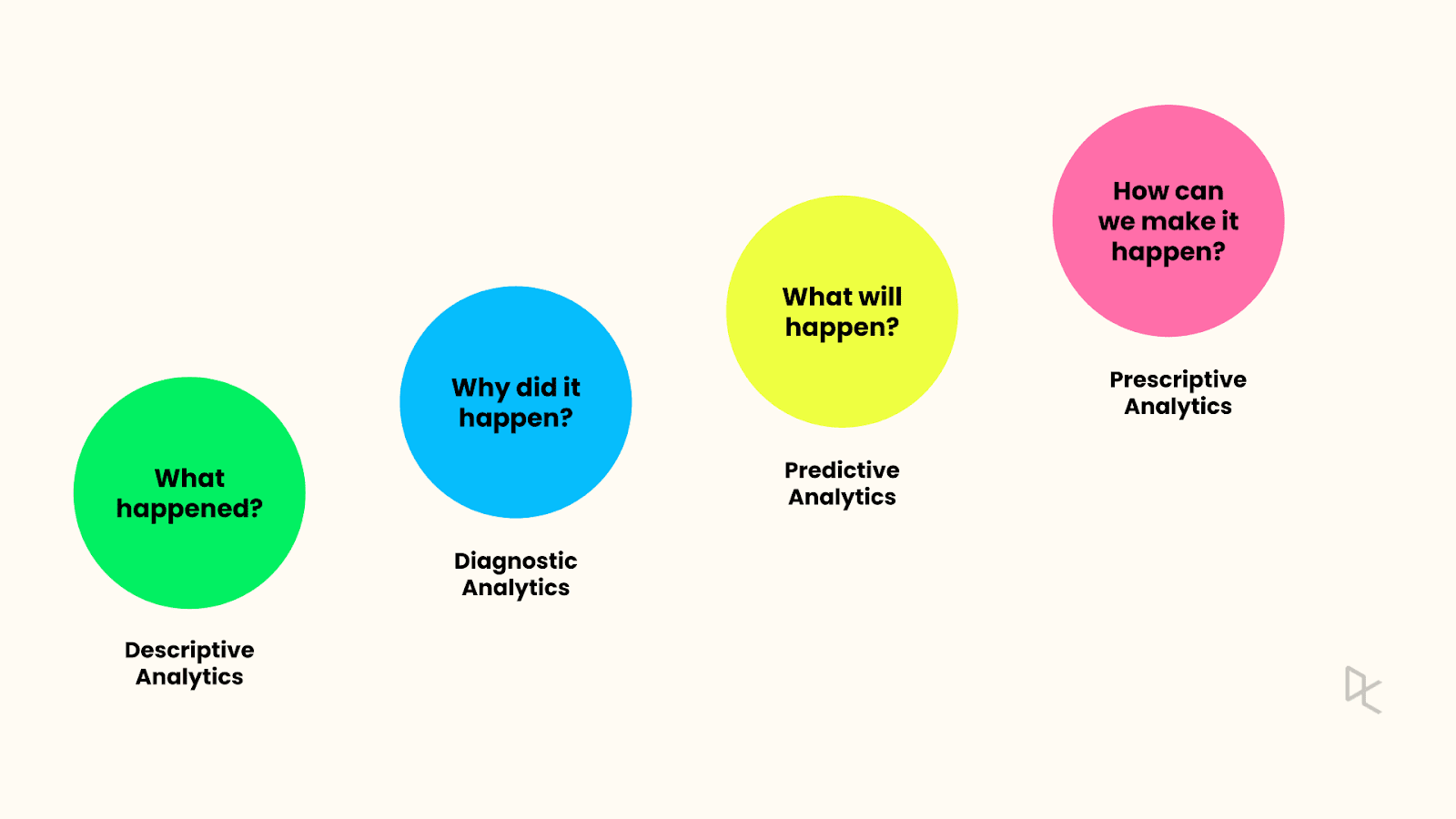
The four types of analytics
Descriptive analysis
Descriptive analysis , as the name suggests, describes or summarizes raw data and makes it interpretable. It involves analyzing historical data to understand what has happened in the past.
This type of analysis is used to identify patterns and trends over time.
For example, a business might use descriptive analysis to understand the average monthly sales for the past year.
Diagnostic analysis
Diagnostic analysis goes a step further than descriptive analysis by determining why something happened. It involves more detailed data exploration and comparing different data sets to understand the cause of a particular outcome.
For instance, if a company's sales dropped in a particular month, diagnostic analysis could be used to find out why.
Predictive analysis
Predictive analysis uses statistical models and forecasting techniques to understand the future. It involves using data from the past to predict what could happen in the future. This type of analysis is often used in risk assessment, marketing, and sales forecasting.
For example, a company might use predictive analysis to forecast the next quarter's sales based on historical data.
Prescriptive analysis
Prescriptive analysis is the most advanced type of data analysis. It not only predicts future outcomes but also suggests actions to benefit from these predictions. It uses sophisticated tools and technologies like machine learning and artificial intelligence to recommend decisions.
For example, a prescriptive analysis might suggest the best marketing strategies to increase future sales.
Data Analysis Techniques
There are numerous techniques used in data analysis, each with its unique purpose and application. Here, we will discuss some of the most commonly used techniques, including exploratory analysis, regression analysis, Monte Carlo simulation, factor analysis, cohort analysis, cluster analysis, time series analysis, and sentiment analysis.
Exploratory analysis
Exploratory analysis is used to understand the main characteristics of a data set. It is often used at the beginning of a data analysis process to summarize the main aspects of the data, check for missing data, and test assumptions. This technique involves visual methods such as scatter plots, histograms, and box plots.
You can learn more about exploratory data analysis with our course, covering how to explore, visualize, and extract insights from data using Python.
Regression analysis
Regression analysis is a statistical method used to understand the relationship between a dependent variable and one or more independent variables. It is commonly used for forecasting, time series modeling, and finding the causal effect relationships between variables.
We have a tutorial exploring the essentials of linear regression , which is one of the most widely used regression algorithms in areas like machine learning.
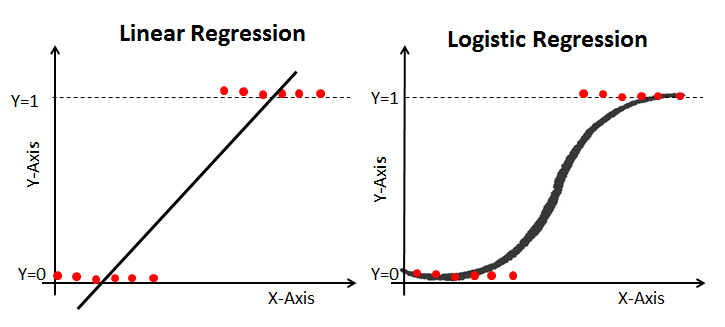
Linear and logistic regression
Factor analysis
Factor analysis is a technique used to reduce a large number of variables into fewer factors. The factors are constructed in such a way that they capture the maximum possible information from the original variables. This technique is often used in market research, customer segmentation, and image recognition.
Learn more about factor analysis in R with our course, which explores latent variables, such as personality, using exploratory and confirmatory factor analyses.
Monte Carlo simulation
Monte Carlo simulation is a technique that uses probability distributions and random sampling to estimate numerical results. It is often used in risk analysis and decision-making where there is significant uncertainty.
We have a tutorial that explores Monte Carlo methods in R , as well as a course on Monte Carlo simulations in Python , which can estimate a range of outcomes for uncertain events.
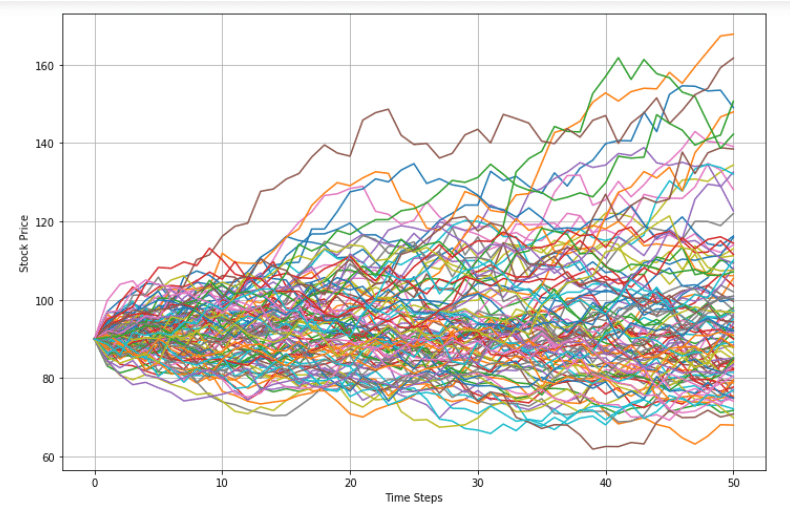
Example of a Monte Carlo simulation
Cluster analysis
Cluster analysis is a technique used to group a set of objects in such a way that objects in the same group (called a cluster) are more similar to each other than to those in other groups. It is often used in market segmentation, image segmentation, and recommendation systems.
You can explore a range of clustering techniques, including hierarchical clustering and k-means clustering, in our Cluster Analysis in R course.
Cohort analysis
Cohort analysis is a subset of behavioral analytics that takes data from a given dataset and groups it into related groups for analysis. These related groups, or cohorts, usually share common characteristics within a defined time span. This technique is often used in marketing, user engagement, and customer lifecycle analysis.
Our course, Customer Segmentation in Python , explores a range of techniques for segmenting and analyzing customer data, including cohort analysis.
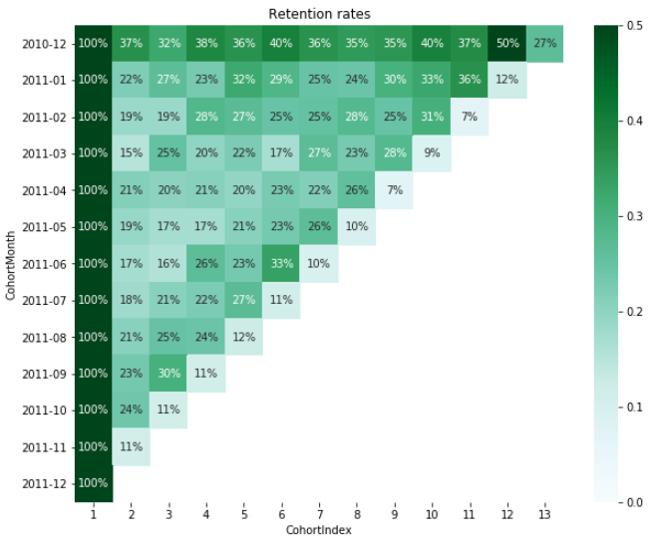
Graph showing an example of cohort analysis
Time series analysis
Time series analysis is a statistical technique that deals with time series data, or trend analysis. It is used to analyze the sequence of data points to extract meaningful statistics and other characteristics of the data. This technique is often used in sales forecasting, economic forecasting, and weather forecasting.
Our Time Series with Python skill track takes you through how to manipulate and analyze time series data, working with a variety of Python libraries.
Sentiment analysis
Sentiment analysis, also known as opinion mining, uses natural language processing, text analysis, and computational linguistics to identify and extract subjective information from source materials. It is often used in social media monitoring, brand monitoring, and understanding customer feedback.
To get familiar with sentiment analysis in Python , you can take our online course, which will teach you how to perform an end-to-end sentiment analysis.
Data Analysis Tools
In the realm of data analysis, various tools are available that cater to different needs, complexities, and levels of expertise. These tools range from programming languages like Python and R to visualization software like Power BI and Tableau. Let's delve into some of these tools.
Python is a high-level, general-purpose programming language that has become a favorite among data analysts and data scientists. Its simplicity and readability, coupled with a wide range of libraries like pandas , NumPy , and Matplotlib , make it an excellent tool for data analysis and data visualization.
" dir="ltr">Resources to get you started
- You can start learning Python today with our Python Fundamentals skill track, which covers all the foundational skills you need to understand the language.
- You can also take out Data Analyst with Python career track to start your journey to becoming a data analyst.
- Check out our Python for beginners cheat sheet as a handy reference guide.
R is a programming language and free software environment specifically designed for statistical computing and graphics. It is widely used among statisticians and data miners for developing statistical software and data analysis. R provides a wide variety of statistical and graphical techniques, including linear and nonlinear modeling, classical statistical tests, time-series analysis, and more.
- Our R Programming skill track will introduce you to R and help you develop the skills you’ll need to start coding in R.
- With the Data Analyst with R career track, you’ll gain the skills you need to start your journey to becoming a data analyst.
- Our Getting Started with R cheat sheet helps give an overview of how to start learning R Programming.
SQL (Structured Query Language) is a standard language for managing and manipulating databases. It is used to retrieve and manipulate data stored in relational databases. SQL is essential for tasks that involve data management or manipulation within databases.
- To get familiar with SQL, consider taking our SQL Fundamentals skill track, where you’ll learn how to interact with and query your data.
- SQL for Business Analysts will boost your business SQL skills.
- Our SQL Basics cheat sheet covers a list of functions for querying data, filtering data, aggregation, and more.
Power BI is a business analytics tool developed by Microsoft. It provides interactive visualizations with self-service business intelligence capabilities. Power BI is used to transform raw data into meaningful insights through easy-to-understand dashboards and reports.
- Explore the power of Power BI with our Power BI Fundamentals skill track, where you’ll learn to get the most from the business intelligence tool.
- With Exploratory Data Analysis in Power BI you’ll learn how to enhance your reports with EDA.
- We have a Power BI cheat sheet which covers many of the basics you’ll need to get started.
Tableau is a powerful data visualization tool used in the Business Intelligence industry. It allows you to create interactive and shareable dashboards, which depict trends, variations, and density of the data in the form of charts and graphs.
- The Tableau Fundamentals skill track will introduce you to the business intelligence tool and how you can use it to clear, analyze, and visualize data.
- Analyzing Data in Tableau will give you some of the advanced skills needed to improve your analytics and visualizations.
- Check out our Tableau cheat sheet , which runs you through the essentials of how to get started using the tool.
Microsoft Excel is one of the most widely used tools for data analysis. It offers a range of features for data manipulation, statistical analysis, and visualization. Excel's simplicity and versatility make it a great tool for both simple and complex data analysis tasks.
- Check out our Data Analysis in Excel course to build functional skills in Excel.
- For spreadsheet skills in general, check out Marketing Analytics in Spreadsheets .
- The Excel Basics cheat sheet covers many of the basic formulas and operations you’ll need to make a start.
Understanding the Impact of Data Analysis
Data analysis, whether on a small or large scale, can have a profound impact on business performance. It can drive significant changes, leading to improved efficiency, increased profitability, and a deeper understanding of market trends and customer behavior.
Informed decision-making
Data analysis allows businesses to make informed decisions based on facts, figures, and trends, rather than relying on guesswork or intuition. It provides a solid foundation for strategic planning and policy-making, ensuring that resources are allocated effectively and that efforts are directed towards areas that will yield the most benefit.
Impact on small businesses
For small businesses, even simple data analysis can lead to significant improvements. For example, analyzing sales data can help identify which products are performing well and which are not. This information can then be used to adjust marketing strategies, pricing, and inventory management, leading to increased sales and profitability.
Impact on large businesses
For larger businesses, the impact of data analysis can be even more profound. Big data analysis can uncover complex patterns and trends that would be impossible to detect otherwise. This can lead to breakthrough insights, driving innovation and giving the business a competitive edge.
For example, a large retailer might use data analysis to optimize its supply chain, reducing costs and improving efficiency. Or a tech company might use data analysis to understand user behavior, leading to improved product design and better user engagement.
The critical role of data analysis
In today's data-driven world, the ability to analyze and interpret data is a critical skill. Businesses that can harness the power of data analysis are better positioned to adapt to changing market conditions, meet customer needs, and drive growth and profitability.
Get started with DataCamp for Business
Build a data-driven workforce with DataCamp for business
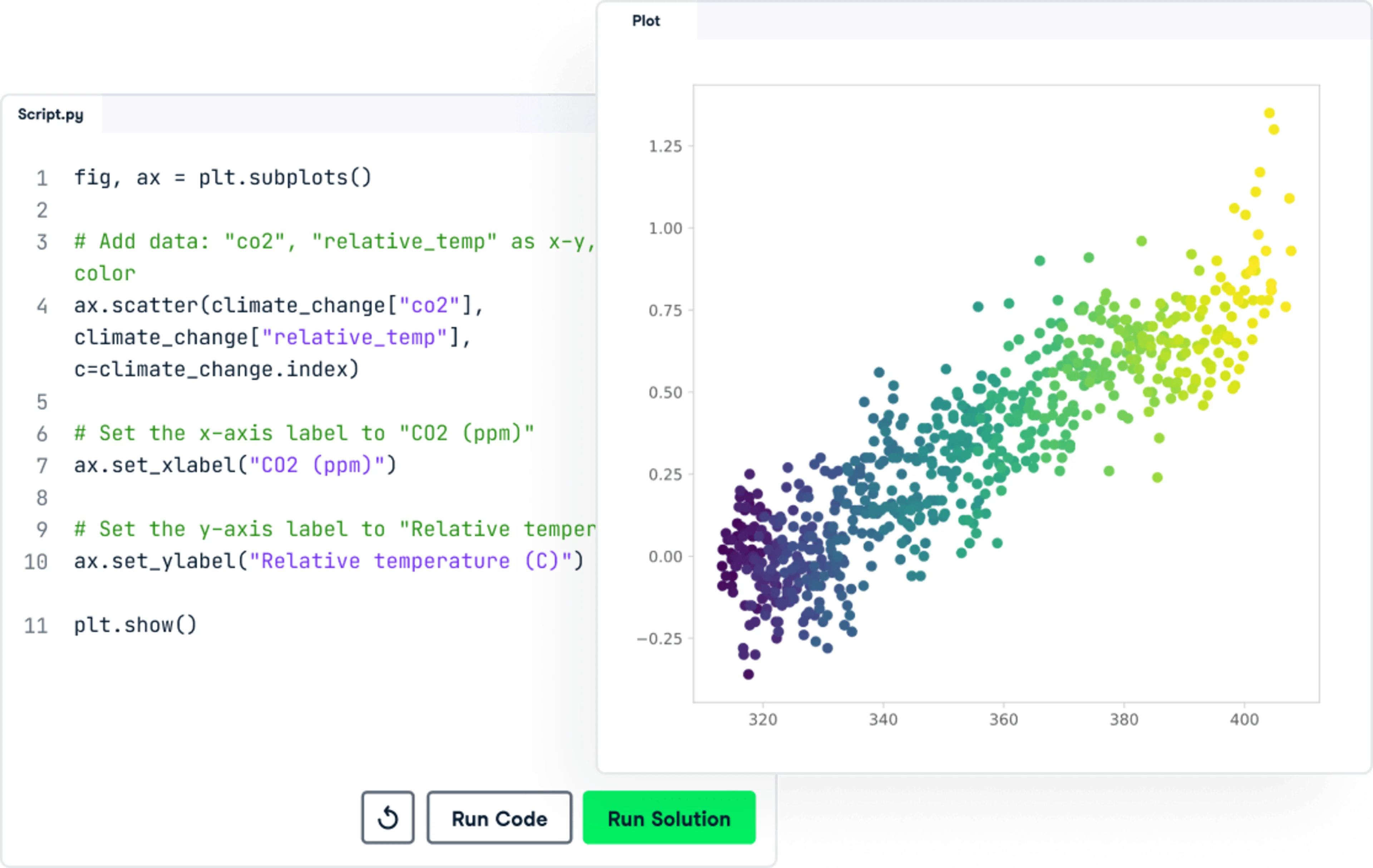
Top Careers in Data Analysis in 2023
In the era of Big Data, careers in data analysis are flourishing. With the increasing demand for data-driven insights, these professions offer promising prospects. Here, we will discuss some of the top careers in data analysis in 2023, referring to our full guide on the top ten analytics careers .
1. Data scientist
Data scientists are the detectives of the data world, uncovering patterns, insights, and trends from vast amounts of information. They use a combination of programming, statistical skills, and machine learning to make sense of complex data sets. Data scientists not only analyze data but also use their insights to influence strategic decisions within their organization.
We’ve got a complete guide on how to become a data scientist , which outlines everything you need to know about starting your career in the industry.
Key skills :
- Proficiency in programming languages like Python or R
- Strong knowledge of statistics and probability
- Familiarity with machine learning algorithms
- Data wrangling and data cleaning skills
- Ability to communicate complex data insights in a clear and understandable manner
Essential tools :
- Jupyter Notebook
- Machine learning libraries like Scikit-learn, TensorFlow
- Data visualization libraries like Matplotlib, Seaborn
2. Business intelligence analyst
Business intelligence analysts are responsible for providing a clear picture of a business's performance by analyzing data related to market trends, business processes, and industry competition. They use tools and software to convert complex data into digestible reports and dashboards, helping decision-makers to understand the business's position and make informed decisions.
- Strong analytical skills
- Proficiency in SQL and other database technologies
- Understanding of data warehousing and ETL processes
- Ability to create clear visualizations and reports
- Business acumen
- Power BI, Tableau
3. Data engineer
Data engineers are the builders and maintainers of the data pipeline. They design, construct, install, test, and maintain highly scalable data management systems. They also ensure that data is clean, reliable, and preprocessed for data scientists to perform analysis.
Read more about what a data engineer does and how you can become a data engineer in our separate guide.
- Proficiency in SQL and NoSQL databases
- Knowledge of distributed systems and data architecture
- Familiarity with ETL tools and processes
- Programming skills, particularly in Python and Java
- Understanding of machine learning algorithms
- Hadoop, Spark
- Python, Java
4. Business analyst
Business analysts are the bridge between IT and business stakeholders. They use data to assess processes, determine requirements, and deliver data-driven recommendations and reports to executives and stakeholders. They are involved in strategic planning, business model analysis, process design, and system analysis.
- Understanding of business processes and strategies
- Proficiency in SQL
- Ability to communicate effectively with both IT and business stakeholders
- Project management skills
|
|
|
| Proficiency in programming, strong statistical knowledge, familiarity with machine learning, data wrangling skills, and effective communication. | Python, R, SQL, Scikit-learn, TensorFlow, Matplotlib, Seaborn |
| Strong analytical skills, proficiency in SQL, understanding of data warehousing and ETL, ability to create visualizations and reports, and business acumen. | SQL, Power BI, Tableau, Excel, Python |
| Proficiency in SQL and NoSQL, knowledge of distributed systems and data architecture, familiarity with ETL, programming skills, and understanding of machine learning. | SQL, NoSQL, Hadoop, Spark, Python, Java, ETL tools |
| Strong analytical skills, understanding of business processes, proficiency in SQL, effective communication, and project management skills. | SQL, Excel,Power BI, Tableau, Python |
A table outlining different data analysis careers
How to Get Started with Data Analysis
Embarking on your journey into data analysis might seem daunting at first, but with the right resources and guidance, you can develop the necessary skills and knowledge. Here are some steps to help you get started, focusing on the resources available at DataCamp.
.css-138yw8m{-webkit-align-self:start;-ms-flex-item-align:start;align-self:start;-webkit-flex-shrink:0;-ms-flex-negative:0;flex-shrink:0;width:-webkit-max-content;width:-moz-max-content;width:max-content;} .css-b8zm5p{box-sizing:border-box;margin:0;min-width:0;-webkit-align-self:start;-ms-flex-item-align:start;align-self:start;-webkit-flex-shrink:0;-ms-flex-negative:0;flex-shrink:0;width:-webkit-max-content;width:-moz-max-content;width:max-content;} .css-i98n7q{-webkit-flex-shrink:0;-ms-flex-negative:0;flex-shrink:0;margin-top:5px;}.css-i98n7q .quote-new_svg__quote{fill:#7933ff;} .css-xfsibo{-webkit-align-self:center;-ms-flex-item-align:center;align-self:center;color:#000820;-webkit-flex-direction:column;-ms-flex-direction:column;flex-direction:column;-webkit-box-flex:1;-webkit-flex-grow:1;-ms-flex-positive:1;flex-grow:1;-webkit-box-pack:space-evenly;-ms-flex-pack:space-evenly;-webkit-justify-content:space-evenly;justify-content:space-evenly;margin-left:16px;} .css-gt3aw7{display:-webkit-box;display:-webkit-flex;display:-ms-flexbox;display:flex;-webkit-align-self:center;-ms-flex-item-align:center;align-self:center;color:#000820;-webkit-flex-direction:column;-ms-flex-direction:column;flex-direction:column;-webkit-box-flex:1;-webkit-flex-grow:1;-ms-flex-positive:1;flex-grow:1;-webkit-box-pack:space-evenly;-ms-flex-pack:space-evenly;-webkit-justify-content:space-evenly;justify-content:space-evenly;margin-left:16px;} .css-n2j7xo{box-sizing:border-box;margin:0;min-width:0;display:-webkit-box;display:-webkit-flex;display:-ms-flexbox;display:flex;-webkit-align-self:center;-ms-flex-item-align:center;align-self:center;color:#000820;-webkit-flex-direction:column;-ms-flex-direction:column;flex-direction:column;-webkit-box-flex:1;-webkit-flex-grow:1;-ms-flex-positive:1;flex-grow:1;-webkit-box-pack:space-evenly;-ms-flex-pack:space-evenly;-webkit-justify-content:space-evenly;justify-content:space-evenly;margin-left:16px;} .css-1yf55a1{margin-bottom:8px;}.css-1yf55a1 a{color:#05192d;font-weight:700;line-height:1.5;-webkit-text-decoration:none;text-decoration:none;}.css-1yf55a1 a:active,.css-1yf55a1 a:focus,.css-1yf55a1 a:hover{-webkit-text-decoration:underline;text-decoration:underline;}.css-1yf55a1 p{font-size:16px;font-weight:800;line-height:24px;} .css-xjjmwi{box-sizing:border-box;margin:0;min-width:0;font-size:1.5rem;letter-spacing:-0.5px;line-height:1.2;margin-top:0;margin-bottom:8px;}.css-xjjmwi a{color:#05192d;font-weight:700;line-height:1.5;-webkit-text-decoration:none;text-decoration:none;}.css-xjjmwi a:active,.css-xjjmwi a:focus,.css-xjjmwi a:hover{-webkit-text-decoration:underline;text-decoration:underline;}.css-xjjmwi p{font-size:16px;font-weight:800;line-height:24px;} To thrive in data analysis, you must build a strong foundation of knowledge, sharpen practical skills, and accumulate valuable experience. Start with statistics, mathematics, and programming and tackle real-world projects. Then, gain domain expertise, and connect with professionals in the field. Combine expertise, skills, and experience for a successful data analysis career. .css-16mqoqa{color:#626D79;font-weight:400;} .css-1k1umiz{box-sizing:border-box;margin:0;min-width:0;font-size:0.875rem;line-height:1.5;margin-top:0;color:#626D79;font-weight:400;} Richie Cotton , Data Evangelist at DataCamp
Understand the basics
Before diving into data analysis, it's important to understand the basics. This includes familiarizing yourself with statistical concepts, data types, and data structures. DataCamp's Introduction to Data Science in Python or Introduction to Data Science in R courses are great starting points.
Learn a programming language
Data analysis requires proficiency in at least one programming language. Python and R are among the most popular choices due to their versatility and the vast array of libraries they offer for data analysis. We offer comprehensive learning paths for both Python and R .
Master data manipulation and visualization
Data manipulation and visualization are key components of data analysis. They allow you to clean, transform, and visualize your data, making it easier to understand and analyze. Courses like Data Manipulation with pandas or Data Visualization with ggplot2 can help you develop these skills.
Dive into Specific Data Analysis Techniques
Once you've mastered the basics, you can delve into specific data analysis techniques like regression analysis , time series analysis , or machine learning . We offer a wide range of courses across many topics, allowing you to specialize based on your interests and career goals.
Practice, Practice, Practice
The key to mastering data analysis is practice. DataCamp's practice mode and projects provide hands-on experience with real-world data, helping you consolidate your learning and apply your skills. You can find a list of 20 data analytics projects for all levels to give you some inspiration.
Remember, learning data analysis is a journey. It's okay to start small and gradually build up your skills over time. With patience, persistence, and the right resources, you'll be well on your way to becoming a proficient data analyst.
Become a ML Scientist
Final thoughts.
In the era of digital transformation, data analysis has emerged as a crucial skill, regardless of your field or industry. The ability to make sense of data, to extract insights, and to use those insights to make informed decisions can give you a significant advantage in today's data-driven world.
Whether you're a marketer looking to understand customer behavior, a healthcare professional aiming to improve patient outcomes, or a business leader seeking to drive growth and profitability, data analysis can provide the insights you need to succeed.
Remember, data analysis is not just about numbers and statistics. It's about asking the right questions, being curious about patterns and trends, and having the courage to make data-driven decisions. It's about telling a story with data, a story that can influence strategies, change perspectives, and drive innovation.
So, we encourage you to apply your understanding of data analysis in your respective fields. Harness the power of data to uncover insights, make informed decisions, and drive success. The world of data is at your fingertips, waiting to be explored.
Data Analyst with Python
.css-1531qan{-webkit-text-decoration:none;text-decoration:none;color:inherit;} data analyst, what is data analysis .css-18x2vi3{-webkit-flex-shrink:0;-ms-flex-negative:0;flex-shrink:0;height:18px;padding-top:6px;-webkit-transform:rotate(0.5turn) translate(21%, -10%);-moz-transform:rotate(0.5turn) translate(21%, -10%);-ms-transform:rotate(0.5turn) translate(21%, -10%);transform:rotate(0.5turn) translate(21%, -10%);-webkit-transition:-webkit-transform 0.3s cubic-bezier(0.85, 0, 0.15, 1);transition:transform 0.3s cubic-bezier(0.85, 0, 0.15, 1);width:18px;}.
Data analysis is a comprehensive method that involves inspecting, cleansing, transforming, and modeling data to discover useful information, make conclusions, and support decision-making. It's a process that empowers organizations to make informed decisions, predict trends, and improve operational efficiency.
What are the steps in the data analysis process? .css-167dpqb{-webkit-flex-shrink:0;-ms-flex-negative:0;flex-shrink:0;height:18px;padding-top:6px;-webkit-transform:none;-moz-transform:none;-ms-transform:none;transform:none;-webkit-transition:-webkit-transform 0.3s cubic-bezier(0.85, 0, 0.15, 1);transition:transform 0.3s cubic-bezier(0.85, 0, 0.15, 1);width:18px;}
The data analysis process involves several steps, including defining objectives and questions, data collection, data cleaning, data analysis, data interpretation and visualization, and data storytelling. Each step is crucial to ensuring the accuracy and usefulness of the results.
What are the different types of data analysis?
Data analysis can be categorized into four types: descriptive, diagnostic, predictive, and prescriptive analysis. Descriptive analysis summarizes raw data, diagnostic analysis determines why something happened, predictive analysis uses past data to predict the future, and prescriptive analysis suggests actions based on predictions.
What are some commonly used data analysis techniques?
There are various data analysis techniques, including exploratory analysis, regression analysis, Monte Carlo simulation, factor analysis, cohort analysis, cluster analysis, time series analysis, and sentiment analysis. Each has its unique purpose and application in interpreting data.
What are some of the tools used in data analysis?
Data analysis typically utilizes tools such as Python, R, SQL for programming, and Power BI, Tableau, and Excel for visualization and data management.
How can I start learning data analysis?
You can start learning data analysis by understanding the basics of statistical concepts, data types, and structures. Then learn a programming language like Python or R, master data manipulation and visualization, and delve into specific data analysis techniques.
How can I become a data analyst?
Becoming a Data Analyst requires a strong understanding of statistical techniques and data analysis tools. Mastery of software such as Python, R, Excel, and specialized software like SPSS and SAS is typically necessary. Read our full guide on how to become a Data Analyst and consider our Data Analyst Certification to get noticed by recruiters.
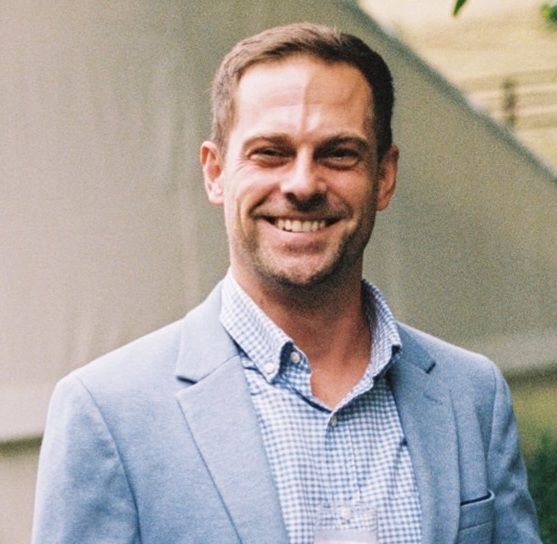
A writer and content editor in the edtech space. Committed to exploring data trends and enthusiastic about learning data science.
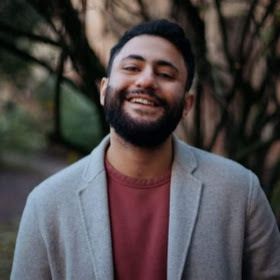
Adel is a Data Science educator, speaker, and Evangelist at DataCamp where he has released various courses and live training on data analysis, machine learning, and data engineering. He is passionate about spreading data skills and data literacy throughout organizations and the intersection of technology and society. He has an MSc in Data Science and Business Analytics. In his free time, you can find him hanging out with his cat Louis.
What is Business Analytics? Everything You Need to Know

Joleen Bothma
How to Analyze Data For Your Business in 5 Steps
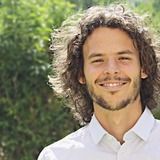
Javier Canales Luna
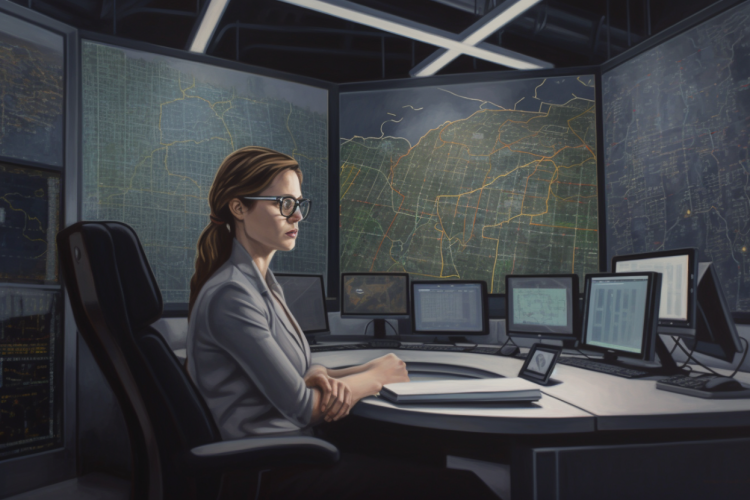
What is Data Science? Definition, Examples, Tools & More
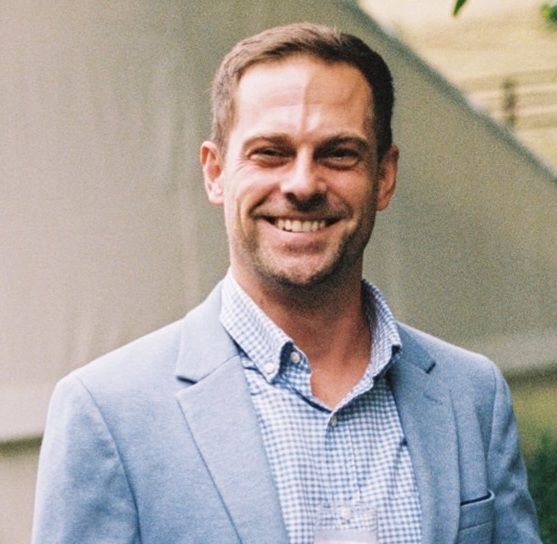
Matt Crabtree
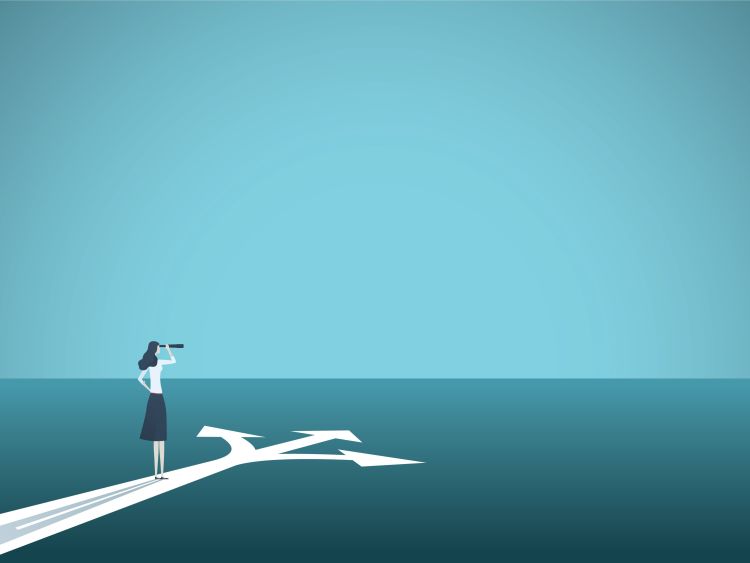
Data Analyst vs. Data Scientist: A Comparative Guide For 2024
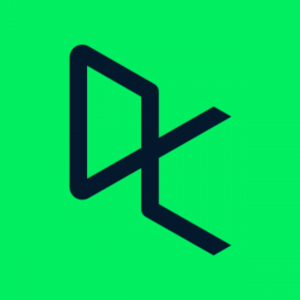
DataCamp Team
A Beginner's Guide to Predictive Analytics
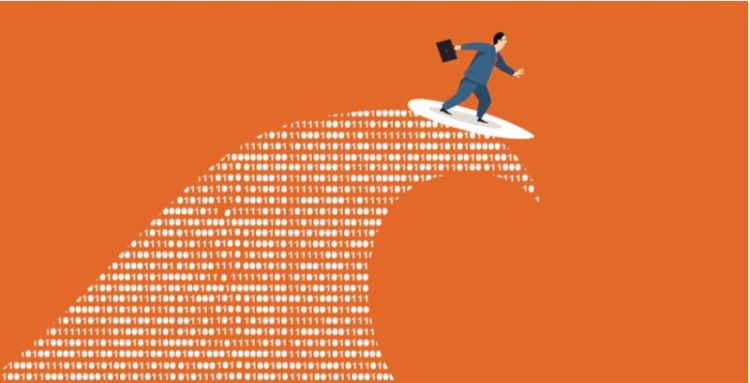
9 Essential Data Analyst Skills: A Comprehensive Career Guide

An official website of the United States government
The .gov means it’s official. Federal government websites often end in .gov or .mil. Before sharing sensitive information, make sure you’re on a federal government site.
The site is secure. The https:// ensures that you are connecting to the official website and that any information you provide is encrypted and transmitted securely.
- Publications
- Account settings
Preview improvements coming to the PMC website in October 2024. Learn More or Try it out now .
- Advanced Search
- Journal List
- PLoS Comput Biol
- v.11(10); 2015 Oct

Ten Simple Rules for Creating a Good Data Management Plan
William k. michener.
College of University Libraries & Learning Sciences, University of New Mexico, Albuquerque, New Mexico, United States of America
Introduction
Research papers and data products are key outcomes of the science enterprise. Governmental, nongovernmental, and private foundation sponsors of research are increasingly recognizing the value of research data. As a result, most funders now require that sufficiently detailed data management plans be submitted as part of a research proposal. A data management plan (DMP) is a document that describes how you will treat your data during a project and what happens with the data after the project ends. Such plans typically cover all or portions of the data life cycle—from data discovery, collection, and organization (e.g., spreadsheets, databases), through quality assurance/quality control, documentation (e.g., data types, laboratory methods) and use of the data, to data preservation and sharing with others (e.g., data policies and dissemination approaches). Fig 1 illustrates the relationship between hypothetical research and data life cycles and highlights the links to the rules presented in this paper. The DMP undergoes peer review and is used in part to evaluate a project’s merit. Plans also document the data management activities associated with funded projects and may be revisited during performance reviews.

As part of the research life cycle (A), many researchers (1) test ideas and hypotheses by (2) acquiring data that are (3) incorporated into various analyses and visualizations, leading to interpretations that are then (4) published in the literature and disseminated via other mechanisms (e.g., conference presentations, blogs, tweets), and that often lead back to (1) new ideas and hypotheses. During the data life cycle (B), researchers typically (1) develop a plan for how data will be managed during and after the project; (2) discover and acquire existing data and (3) collect and organize new data; (4) assure the quality of the data; (5) describe the data (i.e., ascribe metadata); (6) use the data in analyses, models, visualizations, etc.; and (7) preserve and (8) share the data with others (e.g., researchers, students, decision makers), possibly leading to new ideas and hypotheses.
Earlier articles in the Ten Simple Rules series of PLOS Computational Biology provided guidance on getting grants [ 1 ], writing research papers [ 2 ], presenting research findings [ 3 ], and caring for scientific data [ 4 ]. Here, I present ten simple rules that can help guide the process of creating an effective plan for managing research data—the basis for the project’s findings, research papers, and data products. I focus on the principles and practices that will result in a DMP that can be easily understood by others and put to use by your research team. Moreover, following the ten simple rules will help ensure that your data are safe and sharable and that your project maximizes the funder’s return on investment.
Rule 1: Determine the Research Sponsor Requirements
Research communities typically develop their own standard methods and approaches for managing and disseminating data. Likewise, research sponsors often have very specific DMP expectations. For instance, the Wellcome Trust, the Gordon and Betty Moore Foundation (GBMF), the United States National Institutes of Health (NIH), and the US National Science Foundation (NSF) all fund computational biology research but differ markedly in their DMP requirements. The GBMF, for instance, requires that potential grantees develop a comprehensive DMP in conjunction with their program officer that answers dozens of specific questions. In contrast, NIH requirements are much less detailed and primarily ask that potential grantees explain how data will be shared or provide reasons as to why the data cannot be shared. Furthermore, a single research sponsor (such as the NSF) may have different requirements that are established for individual divisions and programs within the organization. Note that plan requirements may not be labeled as such; for example, the National Institutes of Health guidelines focus largely on data sharing and are found in a document entitled “NIH Data Sharing Policy and Implementation Guidance” ( http://grants.nih.gov/grants/policy/data_sharing/data_sharing_guidance.htm ).
Significant time and effort can be saved by first understanding the requirements set forth by the organization to which you are submitting a proposal. Research sponsors normally provide DMP requirements in either the public request for proposals (RFP) or in an online grant proposal guide. The DMPTool ( https://dmptool.org/ ) and DMPonline ( https://dmponline.dcc.ac.uk/ ) websites are also extremely valuable resources that provide updated funding agency plan requirements (for the US and United Kingdom, respectively) in the form of templates that are usually accompanied with annotated advice for filling in the template. The DMPTool website also includes numerous example plans that have been published by DMPTool users. Such examples provide an indication of the depth and breadth of detail that are normally included in a plan and often lead to new ideas that can be incorporated in your plan.
Regardless of whether you have previously submitted proposals to a particular funding program, it is always important to check the latest RFP, as well as the research sponsor’s website, to verify whether requirements have recently changed and how. Furthermore, don’t hesitate to contact the responsible program officer(s) that are listed in a specific solicitation to discuss sponsor requirements or to address specific questions that arise as you are creating a DMP for your proposed project. Keep in mind that the principle objective should be to create a plan that will be useful for your project. Thus, good data management plans can and often do contain more information than is minimally required by the research sponsor. Note, though, that some sponsors constrain the length of DMPs (e.g., two-page limit); in such cases, a synopsis of your more comprehensive plan can be provided, and it may be permissible to include an appendix, supplementary file, or link.
Rule 2: Identify the Data to Be Collected
Every component of the DMP depends upon knowing how much and what types of data will be collected. Data volume is clearly important, as it normally costs more in terms of infrastructure and personnel time to manage 10 terabytes of data than 10 megabytes. But, other characteristics of the data also affect costs as well as metadata, data quality assurance and preservation strategies, and even data policies. A good plan will include information that is sufficient to understand the nature of the data that is collected, including:
- Types. A good first step is to list the various types of data that you expect to collect or create. This may include text, spreadsheets, software and algorithms, models, images and movies, audio files, and patient records. Note that many research sponsors define data broadly to include physical collections, software and code, and curriculum materials.
- Sources. Data may come from direct human observation, laboratory and field instruments, experiments, simulations, and compilations of data from other studies. Reviewers and sponsors may be particularly interested in understanding if data are proprietary, are being compiled from other studies, pertain to human subjects, or are otherwise subject to restrictions in their use or redistribution.
- Volume. Both the total volume of data and the total number of files that are expected to be collected can affect all other data management activities.
- Data and file formats. Technology changes and formats that are acceptable today may soon be obsolete. Good choices include those formats that are nonproprietary, based upon open standards, and widely adopted and preferred by the scientific community (e.g., Comma Separated Values [CSV] over Excel [.xls, xlsx]). Data are more likely to be accessible for the long term if they are uncompressed, unencrypted, and stored using standard character encodings such as UTF-16.
The precise types, sources, volume, and formats of data may not be known beforehand, depending on the nature and uniqueness of the research. In such case, the solution is to iteratively update the plan (see Rule 9 ).
Rule 3: Define How the Data Will Be Organized
Once there is an understanding of the volume and types of data to be collected, a next obvious step is to define how the data will be organized and managed. For many projects, a small number of data tables will be generated that can be effectively managed with commercial or open source spreadsheet programs like Excel and OpenOffice Calc. Larger data volumes and usage constraints may require the use of relational database management systems (RDBMS) for linked data tables like ORACLE or mySQL, or a Geographic Information System (GIS) for geospatial data layers like ArcGIS, GRASS, or QGIS.
The details about how the data will be organized and managed could fill many pages of text and, in fact, should be recorded as the project evolves. However, in drafting a DMP, it is most helpful to initially focus on the types and, possibly, names of the products that will be used. The software tools that are employed in a project should be amenable to the anticipated tasks. A spreadsheet program, for example, would be insufficient for a project in which terabytes of data are expected to be generated, and a sophisticated RDMBS may be overkill for a project in which only a few small data tables will be created. Furthermore, projects dependent upon a GIS or RDBMS may entail considerable software costs and design and programming effort that should be planned and budgeted for upfront (see Rules 9 and 10 ). Depending on sponsor requirements and space constraints, it may also be useful to specify conventions for file naming, persistent unique identifiers (e.g., Digital Object Identifiers [DOIs]), and versioning control (for both software and data products).
Rule 4: Explain How the Data Will Be Documented
Rows and columns of numbers and characters have little to no meaning unless they are documented in some fashion. Metadata—the details about what, where, when, why, and how the data were collected, processed, and interpreted—provide the information that enables data and files to be discovered, used, and properly cited. Metadata include descriptions of how data and files are named, physically structured, and stored as well as details about the experiments, analytical methods, and research context. It is generally the case that the utility and longevity of data relate directly to how complete and comprehensive the metadata are. The amount of effort devoted to creating comprehensive metadata may vary substantially based on the complexity, types, and volume of data.
A sound documentation strategy can be based on three steps. First, identify the types of information that should be captured to enable a researcher like you to discover, access, interpret, use, and cite your data. Second, determine whether there is a community-based metadata schema or standard (i.e., preferred sets of metadata elements) that can be adopted. As examples, variations of the Dublin Core Metadata Initiative Abstract Model are used for many types of data and other resources, ISO (International Organization for Standardization) 19115 is used for geospatial data, ISA-Tab file format is used for experimental metadata, and Ecological Metadata Language (EML) is used for many types of environmental data. In many cases, a specific metadata content standard will be recommended by a target data repository, archive, or domain professional organization. Third, identify software tools that can be employed to create and manage metadata content (e.g., Metavist, Morpho). In lieu of existing tools, text files (e.g., readme.txt) that include the relevant metadata can be included as headers to the data files.
A best practice is to assign a responsible person to maintain an electronic lab notebook, in which all project details are maintained. The notebook should ideally be routinely reviewed and revised by another team member, as well as duplicated (see Rules 6 and 9 ). The metadata recorded in the notebook provide the basis for the metadata that will be associated with data products that are to be stored, reused, and shared.
Rule 5: Describe How Data Quality Will Be Assured
Quality assurance and quality control (QA/QC) refer to the processes that are employed to measure, assess, and improve the quality of products (e.g., data, software, etc.). It may be necessary to follow specific QA/QC guidelines depending on the nature of a study and research sponsorship; such requirements, if they exist, are normally stated in the RFP. Regardless, it is good practice to describe the QA/QC measures that you plan to employ in your project. Such measures may encompass training activities, instrument calibration and verification tests, double-blind data entry, and statistical and visualization approaches to error detection. Simple graphical data exploration approaches (e.g., scatterplots, mapping) can be invaluable for detecting anomalies and errors.
Rule 6: Present a Sound Data Storage and Preservation Strategy
A common mistake of inexperienced (and even many experienced) researchers is to assume that their personal computer and website will live forever. They fail to routinely duplicate their data during the course of the project and do not see the benefit of archiving data in a secure location for the long term. Inevitably, though, papers get lost, hard disks crash, URLs break, and tapes and other media degrade, with the result that the data become unavailable for use by both the originators and others. Thus, data storage and preservation are central to any good data management plan. Give careful consideration to three questions:
- How long will the data be accessible?
- How will data be stored and protected over the duration of the project?
- How will data be preserved and made available for future use?
The answer to the first question depends on several factors. First, determine whether the research sponsor or your home institution have any specific requirements. Usually, all data do not need to be retained, and those that do need not be retained forever. Second, consider the intrinsic value of the data. Observations of phenomena that cannot be repeated (e.g., astronomical and environmental events) may need to be stored indefinitely. Data from easily repeatable experiments may only need to be stored for a short period. Simulations may only need to have the source code, initial conditions, and verification data stored. In addition to explaining how data will be selected for short-term storage and long-term preservation, remember to also highlight your plans for the accompanying metadata and related code and algorithms that will allow others to interpret and use the data (see Rule 4 ).
Develop a sound plan for storing and protecting data over the life of the project. A good approach is to store at least three copies in at least two geographically distributed locations (e.g., original location such as a desktop computer, an external hard drive, and one or more remote sites) and to adopt a regular schedule for duplicating the data (i.e., backup). Remote locations may include an offsite collaborator’s laboratory, an institutional repository (e.g., your departmental, university, or organization’s repository if located in a different building), or a commercial service, such as those offered by Amazon, Dropbox, Google, and Microsoft. The backup schedule should also include testing to ensure that stored data files can be retrieved.
Your best bet for being able to access the data 20 years beyond the life of the project will likely require a more robust solution (i.e., question 3 above). Seek advice from colleagues and librarians to identify an appropriate data repository for your research domain. Many disciplines maintain specific repositories such as GenBank for nucleotide sequence data and the Protein Data Bank for protein sequences. Likewise, many universities and organizations also host institutional repositories, and there are numerous general science data repositories such as Dryad ( http://datadryad.org/ ), figshare ( http://figshare.com/ ), and Zenodo ( http://zenodo.org/ ). Alternatively, one can easily search for discipline-specific and general-use repositories via online catalogs such as http://www.re3data.org/ (i.e., REgistry of REsearch data REpositories) and http://www.biosharing.org (i.e., BioSharing). It is often considered good practice to deposit code in a host repository like GitHub that specializes in source code management as well as some types of data like large files and tabular data (see https://github.com/ ). Make note of any repository-specific policies (e.g., data privacy and security, requirements to submit associated code) and costs for data submission, curation, and backup that should be included in the DMP and the proposal budget.
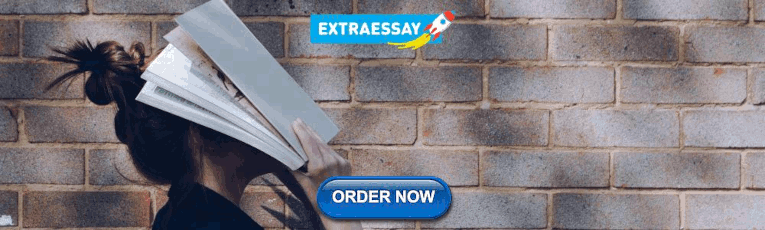
Rule 7: Define the Project’s Data Policies
Despite what may be a natural proclivity to avoid policy and legal matters, researchers cannot afford to do so when it comes to data. Research sponsors, institutions that host research, and scientists all have a role in and obligation for promoting responsible and ethical behavior. Consequently, many research sponsors require that DMPs include explicit policy statements about how data will be managed and shared. Such policies include:
- licensing or sharing arrangements that pertain to the use of preexisting materials;
- plans for retaining, licensing, sharing, and embargoing (i.e., limiting use by others for a period of time) data, code, and other materials; and
- legal and ethical restrictions on access and use of human subject and other sensitive data.
Unfortunately, policies and laws often appear or are, in fact, confusing or contradictory. Furthermore, policies that apply within a single organization or in a given country may not apply elsewhere. When in doubt, consult your institution’s office of sponsored research, the relevant Institutional Review Board, or the program officer(s) assigned to the program to which you are applying for support.
Despite these caveats, it is usually possible to develop a sound policy by following a few simple steps. First, if preexisting materials, such as data and code, are being used, identify and include a description of the relevant licensing and sharing arrangements in your DMP. Explain how third party software or libraries are used in the creation and release of new software. Note that proprietary and intellectual property rights (IPR) laws and export control regulations may limit the extent to which code and software can be shared.
Second, explain how and when the data and other research products will be made available. Be sure to explain any embargo periods or delays such as publication or patent reasons. A common practice is to make data broadly available at the time of publication, or in the case of graduate students, at the time the graduate degree is awarded. Whenever possible, apply standard rights waivers or licenses, such as those established by Open Data Commons (ODC) and Creative Commons (CC), that guide subsequent use of data and other intellectual products (see http://creativecommons.org/ and http://opendatacommons.org/licenses/pddl/summary/ ). The CC0 license and the ODC Public Domain Dedication and License, for example, promote unrestricted sharing and data use. Nonstandard licenses and waivers can be a significant barrier to reuse.
Third, explain how human subject and other sensitive data will be treated (e.g., see http://privacyruleandresearch.nih.gov/ for information pertaining to human health research regulations set forth in the US Health Insurance Portability and Accountability Act). Many research sponsors require that investigators engaged in human subject research approaches seek or receive prior approval from the appropriate Institutional Review Board before a grant proposal is submitted and, certainly, receive approval before the actual research is undertaken. Approvals may require that informed consent be granted, that data are anonymized, or that use is restricted in some fashion.
Rule 8: Describe How the Data Will Be Disseminated
The best-laid preservation plans and data sharing policies do not necessarily mean that a project’s data will see the light of day. Reviewers and research sponsors will be reassured that this will not be the case if you have spelled out how and when the data products will be disseminated to others, especially people outside your research group. There are passive and active ways to disseminate data. Passive approaches include posting data on a project or personal website or mailing or emailing data upon request, although the latter can be problematic when dealing with large data and bandwidth constraints. More active, robust, and preferred approaches include: (1) publishing the data in an open repository or archive (see Rule 6 ); (2) submitting the data (or subsets thereof) as appendices or supplements to journal articles, such as is commonly done with the PLOS family of journals; and (3) publishing the data, metadata, and relevant code as a “data paper” [ 5 ]. Data papers can be published in various journals, including Scientific Data (from Nature Publishing Group), the GeoScience Data Journal (a Wiley publication on behalf of the Royal Meteorological Society), and GigaScience (a joint BioMed Central and Springer publication that supports big data from many biology and life science disciplines).
A good dissemination plan includes a few concise statements. State when, how, and what data products will be made available. Generally, making data available to the greatest extent and with the fewest possible restrictions at the time of publication or project completion is encouraged. The more proactive approaches described above are greatly preferred over mailing or emailing data and will likely save significant time and money in the long run, as the data curation and sharing will be supported by the appropriate journals and repositories or archives. Furthermore, many journals and repositories provide guidelines and mechanisms for how others can appropriately cite your data, including digital object identifiers, and recommended citation formats; this helps ensure that you receive credit for the data products you create. Keep in mind that the data will be more usable and interpretable by you and others if the data are disseminated using standard, nonproprietary approaches and if the data are accompanied by metadata and associated code that is used for data processing.
Rule 9: Assign Roles and Responsibilities
A comprehensive DMP clearly articulates the roles and responsibilities of every named individual and organization associated with the project. Roles may include data collection, data entry, QA/QC, metadata creation and management, backup, data preparation and submission to an archive, and systems administration. Consider time allocations and levels of expertise needed by staff. For small to medium size projects, a single student or postdoctoral associate who is collecting and processing the data may easily assume most or all of the data management tasks. In contrast, large, multi-investigator projects may benefit from having a dedicated staff person(s) assigned to data management.
Treat your DMP as a living document and revisit it frequently (e.g., quarterly basis). Assign a project team member to revise the plan, reflecting any new changes in protocols and policies. It is good practice to track any changes in a revision history that lists the dates that any changes were made to the plan along with the details about those changes, including who made them.
Reviewers and sponsors may be especially interested in knowing how adherence to the data management plan will be assessed and demonstrated, as well as how, and by whom, data will be managed and made available after the project concludes. With respect to the latter, it is often sufficient to include a pointer to the policies and procedures that are followed by the repository where you plan to deposit your data. Be sure to note any contributions by nonproject staff, such as any repository, systems administration, backup, training, or high-performance computing support provided by your institution.
Rule 10: Prepare a Realistic Budget
Creating, managing, publishing, and sharing high-quality data is as much a part of the 21st century research enterprise as is publishing the results. Data management is not new—rather, it is something that all researchers already do. Nonetheless, a common mistake in developing a DMP is forgetting to budget for the activities. Data management takes time and costs money in terms of software, hardware, and personnel. Review your plan and make sure that there are lines in the budget to support the people that manage the data (see Rule 9 ) as well as pay for the requisite hardware, software, and services. Check with the preferred data repository (see Rule 6 ) so that requisite fees and services are budgeted appropriately. As space allows, facilitate reviewers by pointing to specific lines or sections in the budget and budget justification pages. Experienced reviewers will be on the lookout for unfunded components, but they will also recognize that greater or lesser investments in data management depend upon the nature of the research and the types of data.
A data management plan should provide you and others with an easy-to-follow road map that will guide and explain how data are treated throughout the life of the project and after the project is completed. The ten simple rules presented here are designed to aid you in writing a good plan that is logical and comprehensive, that will pass muster with reviewers and research sponsors, and that you can put into practice should your project be funded. A DMP provides a vehicle for conveying information to and setting expectations for your project team during both the proposal and project planning stages, as well as during project team meetings later, when the project is underway. That said, no plan is perfect. Plans do become better through use. The best plans are “living documents” that are periodically reviewed and revised as necessary according to needs and any changes in protocols (e.g., metadata, QA/QC, storage), policy, technology, and staff, as well as reused, in that the most successful parts of the plan are incorporated into subsequent projects. A public, machine-readable, and openly licensed DMP is much more likely to be incorporated into future projects and to have higher impact; such increased transparency in the research funding process (e.g., publication of proposals and DMPs) can assist researchers and sponsors in discovering data and potential collaborators, educating about data management, and monitoring policy compliance [ 6 ].
Acknowledgments
This article is the outcome of a series of training workshops provided for new faculty, postdoctoral associates, and graduate students.
Funding Statement
This work was supported by NSF IIA-1301346, IIA-1329470, and ACI-1430508 ( http://nsf.gov ). The funders had no role in study design, data collection and analysis, decision to publish, or preparation of the manuscript.
Have a language expert improve your writing
Run a free plagiarism check in 10 minutes, generate accurate citations for free.
- Knowledge Base
The Beginner's Guide to Statistical Analysis | 5 Steps & Examples
Statistical analysis means investigating trends, patterns, and relationships using quantitative data . It is an important research tool used by scientists, governments, businesses, and other organizations.
To draw valid conclusions, statistical analysis requires careful planning from the very start of the research process . You need to specify your hypotheses and make decisions about your research design, sample size, and sampling procedure.
After collecting data from your sample, you can organize and summarize the data using descriptive statistics . Then, you can use inferential statistics to formally test hypotheses and make estimates about the population. Finally, you can interpret and generalize your findings.
This article is a practical introduction to statistical analysis for students and researchers. We’ll walk you through the steps using two research examples. The first investigates a potential cause-and-effect relationship, while the second investigates a potential correlation between variables.
Table of contents
Step 1: write your hypotheses and plan your research design, step 2: collect data from a sample, step 3: summarize your data with descriptive statistics, step 4: test hypotheses or make estimates with inferential statistics, step 5: interpret your results, other interesting articles.
To collect valid data for statistical analysis, you first need to specify your hypotheses and plan out your research design.
Writing statistical hypotheses
The goal of research is often to investigate a relationship between variables within a population . You start with a prediction, and use statistical analysis to test that prediction.
A statistical hypothesis is a formal way of writing a prediction about a population. Every research prediction is rephrased into null and alternative hypotheses that can be tested using sample data.
While the null hypothesis always predicts no effect or no relationship between variables, the alternative hypothesis states your research prediction of an effect or relationship.
- Null hypothesis: A 5-minute meditation exercise will have no effect on math test scores in teenagers.
- Alternative hypothesis: A 5-minute meditation exercise will improve math test scores in teenagers.
- Null hypothesis: Parental income and GPA have no relationship with each other in college students.
- Alternative hypothesis: Parental income and GPA are positively correlated in college students.
Planning your research design
A research design is your overall strategy for data collection and analysis. It determines the statistical tests you can use to test your hypothesis later on.
First, decide whether your research will use a descriptive, correlational, or experimental design. Experiments directly influence variables, whereas descriptive and correlational studies only measure variables.
- In an experimental design , you can assess a cause-and-effect relationship (e.g., the effect of meditation on test scores) using statistical tests of comparison or regression.
- In a correlational design , you can explore relationships between variables (e.g., parental income and GPA) without any assumption of causality using correlation coefficients and significance tests.
- In a descriptive design , you can study the characteristics of a population or phenomenon (e.g., the prevalence of anxiety in U.S. college students) using statistical tests to draw inferences from sample data.
Your research design also concerns whether you’ll compare participants at the group level or individual level, or both.
- In a between-subjects design , you compare the group-level outcomes of participants who have been exposed to different treatments (e.g., those who performed a meditation exercise vs those who didn’t).
- In a within-subjects design , you compare repeated measures from participants who have participated in all treatments of a study (e.g., scores from before and after performing a meditation exercise).
- In a mixed (factorial) design , one variable is altered between subjects and another is altered within subjects (e.g., pretest and posttest scores from participants who either did or didn’t do a meditation exercise).
- Experimental
- Correlational
First, you’ll take baseline test scores from participants. Then, your participants will undergo a 5-minute meditation exercise. Finally, you’ll record participants’ scores from a second math test.
In this experiment, the independent variable is the 5-minute meditation exercise, and the dependent variable is the math test score from before and after the intervention. Example: Correlational research design In a correlational study, you test whether there is a relationship between parental income and GPA in graduating college students. To collect your data, you will ask participants to fill in a survey and self-report their parents’ incomes and their own GPA.
Measuring variables
When planning a research design, you should operationalize your variables and decide exactly how you will measure them.
For statistical analysis, it’s important to consider the level of measurement of your variables, which tells you what kind of data they contain:
- Categorical data represents groupings. These may be nominal (e.g., gender) or ordinal (e.g. level of language ability).
- Quantitative data represents amounts. These may be on an interval scale (e.g. test score) or a ratio scale (e.g. age).
Many variables can be measured at different levels of precision. For example, age data can be quantitative (8 years old) or categorical (young). If a variable is coded numerically (e.g., level of agreement from 1–5), it doesn’t automatically mean that it’s quantitative instead of categorical.
Identifying the measurement level is important for choosing appropriate statistics and hypothesis tests. For example, you can calculate a mean score with quantitative data, but not with categorical data.
In a research study, along with measures of your variables of interest, you’ll often collect data on relevant participant characteristics.
Variable | Type of data |
---|---|
Age | Quantitative (ratio) |
Gender | Categorical (nominal) |
Race or ethnicity | Categorical (nominal) |
Baseline test scores | Quantitative (interval) |
Final test scores | Quantitative (interval) |
Parental income | Quantitative (ratio) |
---|---|
GPA | Quantitative (interval) |
Prevent plagiarism. Run a free check.
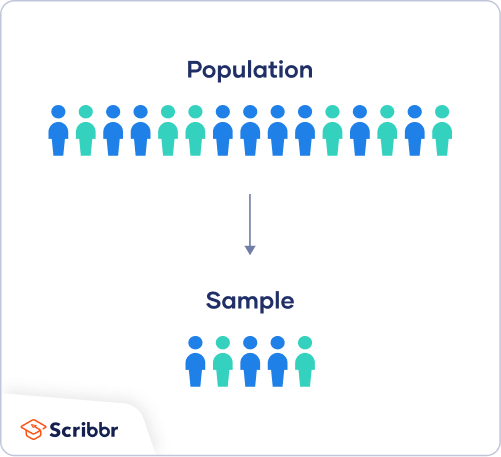
In most cases, it’s too difficult or expensive to collect data from every member of the population you’re interested in studying. Instead, you’ll collect data from a sample.
Statistical analysis allows you to apply your findings beyond your own sample as long as you use appropriate sampling procedures . You should aim for a sample that is representative of the population.
Sampling for statistical analysis
There are two main approaches to selecting a sample.
- Probability sampling: every member of the population has a chance of being selected for the study through random selection.
- Non-probability sampling: some members of the population are more likely than others to be selected for the study because of criteria such as convenience or voluntary self-selection.
In theory, for highly generalizable findings, you should use a probability sampling method. Random selection reduces several types of research bias , like sampling bias , and ensures that data from your sample is actually typical of the population. Parametric tests can be used to make strong statistical inferences when data are collected using probability sampling.
But in practice, it’s rarely possible to gather the ideal sample. While non-probability samples are more likely to at risk for biases like self-selection bias , they are much easier to recruit and collect data from. Non-parametric tests are more appropriate for non-probability samples, but they result in weaker inferences about the population.
If you want to use parametric tests for non-probability samples, you have to make the case that:
- your sample is representative of the population you’re generalizing your findings to.
- your sample lacks systematic bias.
Keep in mind that external validity means that you can only generalize your conclusions to others who share the characteristics of your sample. For instance, results from Western, Educated, Industrialized, Rich and Democratic samples (e.g., college students in the US) aren’t automatically applicable to all non-WEIRD populations.
If you apply parametric tests to data from non-probability samples, be sure to elaborate on the limitations of how far your results can be generalized in your discussion section .
Create an appropriate sampling procedure
Based on the resources available for your research, decide on how you’ll recruit participants.
- Will you have resources to advertise your study widely, including outside of your university setting?
- Will you have the means to recruit a diverse sample that represents a broad population?
- Do you have time to contact and follow up with members of hard-to-reach groups?
Your participants are self-selected by their schools. Although you’re using a non-probability sample, you aim for a diverse and representative sample. Example: Sampling (correlational study) Your main population of interest is male college students in the US. Using social media advertising, you recruit senior-year male college students from a smaller subpopulation: seven universities in the Boston area.
Calculate sufficient sample size
Before recruiting participants, decide on your sample size either by looking at other studies in your field or using statistics. A sample that’s too small may be unrepresentative of the sample, while a sample that’s too large will be more costly than necessary.
There are many sample size calculators online. Different formulas are used depending on whether you have subgroups or how rigorous your study should be (e.g., in clinical research). As a rule of thumb, a minimum of 30 units or more per subgroup is necessary.
To use these calculators, you have to understand and input these key components:
- Significance level (alpha): the risk of rejecting a true null hypothesis that you are willing to take, usually set at 5%.
- Statistical power : the probability of your study detecting an effect of a certain size if there is one, usually 80% or higher.
- Expected effect size : a standardized indication of how large the expected result of your study will be, usually based on other similar studies.
- Population standard deviation: an estimate of the population parameter based on a previous study or a pilot study of your own.
Once you’ve collected all of your data, you can inspect them and calculate descriptive statistics that summarize them.
Inspect your data
There are various ways to inspect your data, including the following:
- Organizing data from each variable in frequency distribution tables .
- Displaying data from a key variable in a bar chart to view the distribution of responses.
- Visualizing the relationship between two variables using a scatter plot .
By visualizing your data in tables and graphs, you can assess whether your data follow a skewed or normal distribution and whether there are any outliers or missing data.
A normal distribution means that your data are symmetrically distributed around a center where most values lie, with the values tapering off at the tail ends.
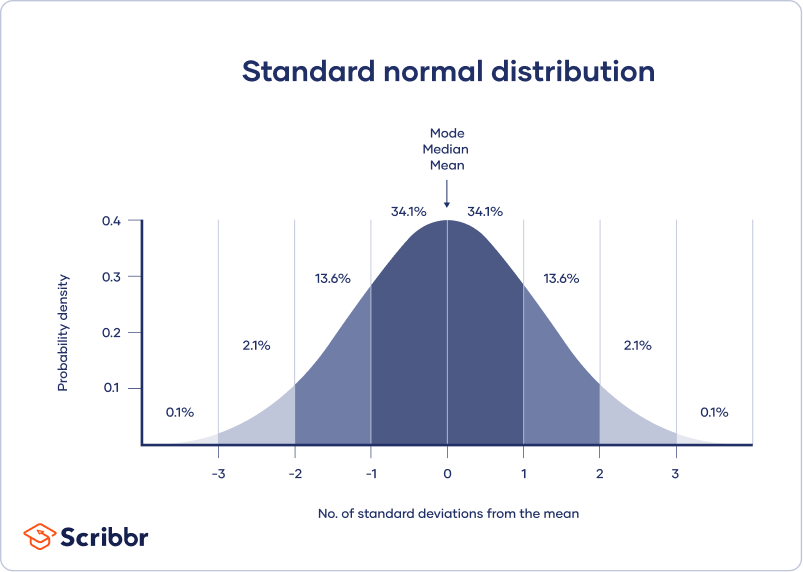
In contrast, a skewed distribution is asymmetric and has more values on one end than the other. The shape of the distribution is important to keep in mind because only some descriptive statistics should be used with skewed distributions.
Extreme outliers can also produce misleading statistics, so you may need a systematic approach to dealing with these values.
Calculate measures of central tendency
Measures of central tendency describe where most of the values in a data set lie. Three main measures of central tendency are often reported:
- Mode : the most popular response or value in the data set.
- Median : the value in the exact middle of the data set when ordered from low to high.
- Mean : the sum of all values divided by the number of values.
However, depending on the shape of the distribution and level of measurement, only one or two of these measures may be appropriate. For example, many demographic characteristics can only be described using the mode or proportions, while a variable like reaction time may not have a mode at all.
Calculate measures of variability
Measures of variability tell you how spread out the values in a data set are. Four main measures of variability are often reported:
- Range : the highest value minus the lowest value of the data set.
- Interquartile range : the range of the middle half of the data set.
- Standard deviation : the average distance between each value in your data set and the mean.
- Variance : the square of the standard deviation.
Once again, the shape of the distribution and level of measurement should guide your choice of variability statistics. The interquartile range is the best measure for skewed distributions, while standard deviation and variance provide the best information for normal distributions.
Using your table, you should check whether the units of the descriptive statistics are comparable for pretest and posttest scores. For example, are the variance levels similar across the groups? Are there any extreme values? If there are, you may need to identify and remove extreme outliers in your data set or transform your data before performing a statistical test.
Pretest scores | Posttest scores | |
---|---|---|
Mean | 68.44 | 75.25 |
Standard deviation | 9.43 | 9.88 |
Variance | 88.96 | 97.96 |
Range | 36.25 | 45.12 |
30 |
From this table, we can see that the mean score increased after the meditation exercise, and the variances of the two scores are comparable. Next, we can perform a statistical test to find out if this improvement in test scores is statistically significant in the population. Example: Descriptive statistics (correlational study) After collecting data from 653 students, you tabulate descriptive statistics for annual parental income and GPA.
It’s important to check whether you have a broad range of data points. If you don’t, your data may be skewed towards some groups more than others (e.g., high academic achievers), and only limited inferences can be made about a relationship.
Parental income (USD) | GPA | |
---|---|---|
Mean | 62,100 | 3.12 |
Standard deviation | 15,000 | 0.45 |
Variance | 225,000,000 | 0.16 |
Range | 8,000–378,000 | 2.64–4.00 |
653 |
A number that describes a sample is called a statistic , while a number describing a population is called a parameter . Using inferential statistics , you can make conclusions about population parameters based on sample statistics.
Researchers often use two main methods (simultaneously) to make inferences in statistics.
- Estimation: calculating population parameters based on sample statistics.
- Hypothesis testing: a formal process for testing research predictions about the population using samples.
You can make two types of estimates of population parameters from sample statistics:
- A point estimate : a value that represents your best guess of the exact parameter.
- An interval estimate : a range of values that represent your best guess of where the parameter lies.
If your aim is to infer and report population characteristics from sample data, it’s best to use both point and interval estimates in your paper.
You can consider a sample statistic a point estimate for the population parameter when you have a representative sample (e.g., in a wide public opinion poll, the proportion of a sample that supports the current government is taken as the population proportion of government supporters).
There’s always error involved in estimation, so you should also provide a confidence interval as an interval estimate to show the variability around a point estimate.
A confidence interval uses the standard error and the z score from the standard normal distribution to convey where you’d generally expect to find the population parameter most of the time.
Hypothesis testing
Using data from a sample, you can test hypotheses about relationships between variables in the population. Hypothesis testing starts with the assumption that the null hypothesis is true in the population, and you use statistical tests to assess whether the null hypothesis can be rejected or not.
Statistical tests determine where your sample data would lie on an expected distribution of sample data if the null hypothesis were true. These tests give two main outputs:
- A test statistic tells you how much your data differs from the null hypothesis of the test.
- A p value tells you the likelihood of obtaining your results if the null hypothesis is actually true in the population.
Statistical tests come in three main varieties:
- Comparison tests assess group differences in outcomes.
- Regression tests assess cause-and-effect relationships between variables.
- Correlation tests assess relationships between variables without assuming causation.
Your choice of statistical test depends on your research questions, research design, sampling method, and data characteristics.
Parametric tests
Parametric tests make powerful inferences about the population based on sample data. But to use them, some assumptions must be met, and only some types of variables can be used. If your data violate these assumptions, you can perform appropriate data transformations or use alternative non-parametric tests instead.
A regression models the extent to which changes in a predictor variable results in changes in outcome variable(s).
- A simple linear regression includes one predictor variable and one outcome variable.
- A multiple linear regression includes two or more predictor variables and one outcome variable.
Comparison tests usually compare the means of groups. These may be the means of different groups within a sample (e.g., a treatment and control group), the means of one sample group taken at different times (e.g., pretest and posttest scores), or a sample mean and a population mean.
- A t test is for exactly 1 or 2 groups when the sample is small (30 or less).
- A z test is for exactly 1 or 2 groups when the sample is large.
- An ANOVA is for 3 or more groups.
The z and t tests have subtypes based on the number and types of samples and the hypotheses:
- If you have only one sample that you want to compare to a population mean, use a one-sample test .
- If you have paired measurements (within-subjects design), use a dependent (paired) samples test .
- If you have completely separate measurements from two unmatched groups (between-subjects design), use an independent (unpaired) samples test .
- If you expect a difference between groups in a specific direction, use a one-tailed test .
- If you don’t have any expectations for the direction of a difference between groups, use a two-tailed test .
The only parametric correlation test is Pearson’s r . The correlation coefficient ( r ) tells you the strength of a linear relationship between two quantitative variables.
However, to test whether the correlation in the sample is strong enough to be important in the population, you also need to perform a significance test of the correlation coefficient, usually a t test, to obtain a p value. This test uses your sample size to calculate how much the correlation coefficient differs from zero in the population.
You use a dependent-samples, one-tailed t test to assess whether the meditation exercise significantly improved math test scores. The test gives you:
- a t value (test statistic) of 3.00
- a p value of 0.0028
Although Pearson’s r is a test statistic, it doesn’t tell you anything about how significant the correlation is in the population. You also need to test whether this sample correlation coefficient is large enough to demonstrate a correlation in the population.
A t test can also determine how significantly a correlation coefficient differs from zero based on sample size. Since you expect a positive correlation between parental income and GPA, you use a one-sample, one-tailed t test. The t test gives you:
- a t value of 3.08
- a p value of 0.001
Receive feedback on language, structure, and formatting
Professional editors proofread and edit your paper by focusing on:
- Academic style
- Vague sentences
- Style consistency
See an example
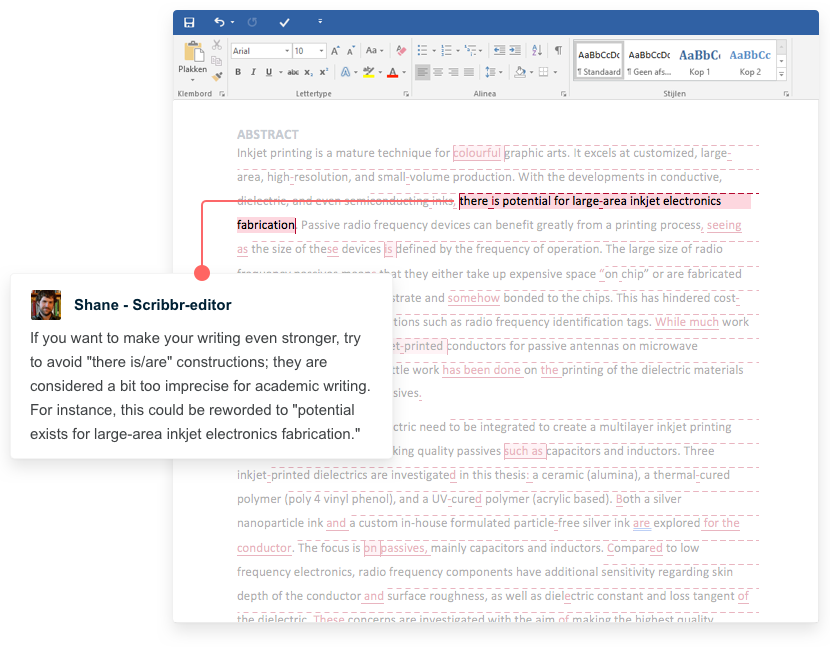
The final step of statistical analysis is interpreting your results.
Statistical significance
In hypothesis testing, statistical significance is the main criterion for forming conclusions. You compare your p value to a set significance level (usually 0.05) to decide whether your results are statistically significant or non-significant.
Statistically significant results are considered unlikely to have arisen solely due to chance. There is only a very low chance of such a result occurring if the null hypothesis is true in the population.
This means that you believe the meditation intervention, rather than random factors, directly caused the increase in test scores. Example: Interpret your results (correlational study) You compare your p value of 0.001 to your significance threshold of 0.05. With a p value under this threshold, you can reject the null hypothesis. This indicates a statistically significant correlation between parental income and GPA in male college students.
Note that correlation doesn’t always mean causation, because there are often many underlying factors contributing to a complex variable like GPA. Even if one variable is related to another, this may be because of a third variable influencing both of them, or indirect links between the two variables.
Effect size
A statistically significant result doesn’t necessarily mean that there are important real life applications or clinical outcomes for a finding.
In contrast, the effect size indicates the practical significance of your results. It’s important to report effect sizes along with your inferential statistics for a complete picture of your results. You should also report interval estimates of effect sizes if you’re writing an APA style paper .
With a Cohen’s d of 0.72, there’s medium to high practical significance to your finding that the meditation exercise improved test scores. Example: Effect size (correlational study) To determine the effect size of the correlation coefficient, you compare your Pearson’s r value to Cohen’s effect size criteria.
Decision errors
Type I and Type II errors are mistakes made in research conclusions. A Type I error means rejecting the null hypothesis when it’s actually true, while a Type II error means failing to reject the null hypothesis when it’s false.
You can aim to minimize the risk of these errors by selecting an optimal significance level and ensuring high power . However, there’s a trade-off between the two errors, so a fine balance is necessary.
Frequentist versus Bayesian statistics
Traditionally, frequentist statistics emphasizes null hypothesis significance testing and always starts with the assumption of a true null hypothesis.
However, Bayesian statistics has grown in popularity as an alternative approach in the last few decades. In this approach, you use previous research to continually update your hypotheses based on your expectations and observations.
Bayes factor compares the relative strength of evidence for the null versus the alternative hypothesis rather than making a conclusion about rejecting the null hypothesis or not.
If you want to know more about statistics , methodology , or research bias , make sure to check out some of our other articles with explanations and examples.
- Student’s t -distribution
- Normal distribution
- Null and Alternative Hypotheses
- Chi square tests
- Confidence interval
Methodology
- Cluster sampling
- Stratified sampling
- Data cleansing
- Reproducibility vs Replicability
- Peer review
- Likert scale
Research bias
- Implicit bias
- Framing effect
- Cognitive bias
- Placebo effect
- Hawthorne effect
- Hostile attribution bias
- Affect heuristic
Is this article helpful?
Other students also liked.
- Descriptive Statistics | Definitions, Types, Examples
- Inferential Statistics | An Easy Introduction & Examples
- Choosing the Right Statistical Test | Types & Examples
More interesting articles
- Akaike Information Criterion | When & How to Use It (Example)
- An Easy Introduction to Statistical Significance (With Examples)
- An Introduction to t Tests | Definitions, Formula and Examples
- ANOVA in R | A Complete Step-by-Step Guide with Examples
- Central Limit Theorem | Formula, Definition & Examples
- Central Tendency | Understanding the Mean, Median & Mode
- Chi-Square (Χ²) Distributions | Definition & Examples
- Chi-Square (Χ²) Table | Examples & Downloadable Table
- Chi-Square (Χ²) Tests | Types, Formula & Examples
- Chi-Square Goodness of Fit Test | Formula, Guide & Examples
- Chi-Square Test of Independence | Formula, Guide & Examples
- Coefficient of Determination (R²) | Calculation & Interpretation
- Correlation Coefficient | Types, Formulas & Examples
- Frequency Distribution | Tables, Types & Examples
- How to Calculate Standard Deviation (Guide) | Calculator & Examples
- How to Calculate Variance | Calculator, Analysis & Examples
- How to Find Degrees of Freedom | Definition & Formula
- How to Find Interquartile Range (IQR) | Calculator & Examples
- How to Find Outliers | 4 Ways with Examples & Explanation
- How to Find the Geometric Mean | Calculator & Formula
- How to Find the Mean | Definition, Examples & Calculator
- How to Find the Median | Definition, Examples & Calculator
- How to Find the Mode | Definition, Examples & Calculator
- How to Find the Range of a Data Set | Calculator & Formula
- Hypothesis Testing | A Step-by-Step Guide with Easy Examples
- Interval Data and How to Analyze It | Definitions & Examples
- Levels of Measurement | Nominal, Ordinal, Interval and Ratio
- Linear Regression in R | A Step-by-Step Guide & Examples
- Missing Data | Types, Explanation, & Imputation
- Multiple Linear Regression | A Quick Guide (Examples)
- Nominal Data | Definition, Examples, Data Collection & Analysis
- Normal Distribution | Examples, Formulas, & Uses
- Null and Alternative Hypotheses | Definitions & Examples
- One-way ANOVA | When and How to Use It (With Examples)
- Ordinal Data | Definition, Examples, Data Collection & Analysis
- Parameter vs Statistic | Definitions, Differences & Examples
- Pearson Correlation Coefficient (r) | Guide & Examples
- Poisson Distributions | Definition, Formula & Examples
- Probability Distribution | Formula, Types, & Examples
- Quartiles & Quantiles | Calculation, Definition & Interpretation
- Ratio Scales | Definition, Examples, & Data Analysis
- Simple Linear Regression | An Easy Introduction & Examples
- Skewness | Definition, Examples & Formula
- Statistical Power and Why It Matters | A Simple Introduction
- Student's t Table (Free Download) | Guide & Examples
- T-distribution: What it is and how to use it
- Test statistics | Definition, Interpretation, and Examples
- The Standard Normal Distribution | Calculator, Examples & Uses
- Two-Way ANOVA | Examples & When To Use It
- Type I & Type II Errors | Differences, Examples, Visualizations
- Understanding Confidence Intervals | Easy Examples & Formulas
- Understanding P values | Definition and Examples
- Variability | Calculating Range, IQR, Variance, Standard Deviation
- What is Effect Size and Why Does It Matter? (Examples)
- What Is Kurtosis? | Definition, Examples & Formula
- What Is Standard Error? | How to Calculate (Guide with Examples)
What is your plagiarism score?
- Skip to main content
- Skip to primary sidebar
- Skip to footer
- QuestionPro

- Solutions Industries Gaming Automotive Sports and events Education Government Travel & Hospitality Financial Services Healthcare Cannabis Technology Use Case AskWhy Communities Audience Contactless surveys Mobile LivePolls Member Experience GDPR Positive People Science 360 Feedback Surveys
- Resources Blog eBooks Survey Templates Case Studies Training Help center

Home Market Research Research Tools and Apps
Research data management: What it is + benefits with examples
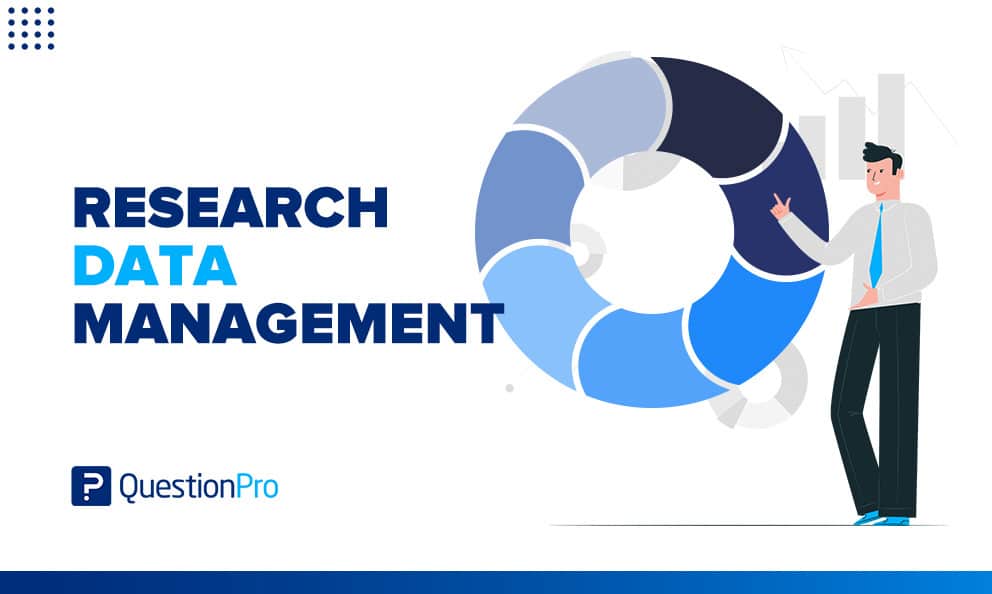
With the proliferation of data and the need for agile and fast insights, research teams, researchers, and organizations worldwide need quicker access to the correct data, which is possible with research data management .
Research is conducted for various reasons, including academic research, pricing research, brand tracking, monitoring, competitive research, ad testing , longitudinal tracking, product and service upgrades, customer satisfaction, etc. The data generated during the research process is diverse and vast.
LEARN ABOUT: Pricing Research
Accessing the correct data in the proper format allows for the data democratization of insights, reduces the silo in research, and eliminates tribal knowledge with mature insights management tools such as InsightsHub . A recent Statista report stated that the global revenue from the market research industry exceeded $74.6 billion in 2021 , and that number is only expected to grow.
With data at this scale, it is imperative to have systems in place to make the most of the data in the shortest possible time, and that’s where research data management comes in.
LEARN ABOUT: Research Process Steps
What is research data management?
Research data management (or RDM) is the action of vigorously organizing, storing, and preserving data during the market research process. RDM covers the data lifecycle from planning through to people, process and technology, and long-term monitoring and access to the data. This is a continuous ongoing cycle during the process of data.
Research data comes in many forms and types, especially with various types of research that include qualitative and quantitative research. This means the data also can be of multiple scales and types. RDM helps to classify, categorize and store this information in a way that’s easy to understand, reference, and draw inferences from.
Data management in research follows the fundamentals of the data life cycle, which are critical steps in research data management as listed below:
- Plan: The plan includes involving stakeholders, defining processes, picking the tools, defining data owners, and how the data is shared.
- Create: Researchers and research teams create the data in the form of data collection techniques defined by projects and then put together this data in structured formats with relevant tags and meta-descriptions.
- Process: This raw data is then converted into digital data in the organization’s structure. The information is cleaned, scrubbed, and structured to eliminate time for insights.
- Analyze: A critical component of RDM is analyzing research dat a to derive actionable insights from the data that has been collected. This data can then be structured into consumable data.
- Preserve: The raw and analyzed data is then preserved in formats defined in the earlier process to maintain the quality of information.
- Share: Distribution of insights to the right stakeholders in role-based access control is required so that the insights are then actioned upon to match business and research goals.
- Reuse: With the correct metadata, tagging and categorization, it is possible to reuse research data to draw correlations, increase ROI and reduce time to research studies.
All the above steps aid in innovative research data management and are critical to market research and insights management success.
LEARN ABOUT: Action Research
Research data management benefits
Following good research data management practices has multiple benefits. Some of the most important ones, however, are:
Maintain the sanctity of data and increase accountability
An essential benefit of RDM is that it allows the ability to maintain the sanctity of the collected data and increases accountability across the board and for all stakeholders. There is absolute transparency in how the information is collected, stored, tracked, shared, and more, along with the additional benefits of following data storage compliances and regulations. Defined processes also lead to lesser ambiguity about stakeholders and data owners and how it is to be monitored.
Eliminate tribal knowledge
Since there is an expectation that data is to be managed in a specific manner, everyone follows the same process. This eliminates tribal knowledge when people either leave organizations or new members come into the fold. It also ensures that stakeholders and researchers from cross-functional teams can lean in on past data to make inferences.
Democratize insights
Insights are powerful when they’re accessible to the right teams at the right time. With research data management, there is an assurance that even if it is role-based access, a larger pool of members has access to the data regardless of the research design and type. There is greater visibility in the tools used; the audience reached, granular, and analyzed data, which in turn helps to democratize insights.
Enable longitudinal monitoring and quick turnaround studies
No matter what type of research is being conducted, RDM allows users to either draw comparisons from past studies or use the past data to validate or disprove hypotheses. With easy access to data, there is also the ability to conduct longitudinal studies or quick turnaround studies by leaning on past, structured data.
Avoid duplication of efforts and research
Brands and organizations use a market research platform to conduct research studies. With a data management software plan in place, you can avoid redoing the same or similar research and reducing the research’s geo-location borders and validity. It also helps reduce duplication of efforts as you do not have to start from scratch.
Reduce time and increase the ROI of research
With easy access to structured data and insights, the time to insights is reduced as there is a reduction of duplicity in research projects. There’s also the scope of inferences to past data and data across demographics and regions. There’s scope to do more with less. All of the above aids in increasing the ROI of research as the effort spent is lesser, but the output is higher, which aids with continuous discovery .
Research data management examples
As seen above, there is research data management is forming an integral part of organizations and research teams to derive the most out of their research processes.
To illustrate this better with an example, consider a retail giant with a presence in many countries. To stay above the competition, create sticky customers, and to constantly co-create with customers, multiple research techniques and methods are used continuously.
This research helps to understand the brand value, consumer behavior, pricing sensitivity, product upgrades, customer satisfaction, etc. By implementing a solid RDM strategy, the brand can lean on past and existing studies, both qualitative and quantitative, to draw inferences about pricing preferences across markets, seasonal launches, what works in different needs, perception of brand vs. their competitors, etc. There is also the ability to look at historical data to manage inventory or budget for marketing spending.
When done well, a good research data management strategy and the right knowledge discovery tools can work wonders for brands and organizations alike.
Get the most out of your research data management
With QuestionPro, you have access to the most mature market research platform and tool that helps you collect and analyze the insights that matter the most. By leveraging InsightsHub , the unified hub for data management, you can leverage the consolidated platform to organize, explore, search, and discover your research data in one organized repository.
LEARN ABOUT: Customer data management
Get started now
MORE LIKE THIS
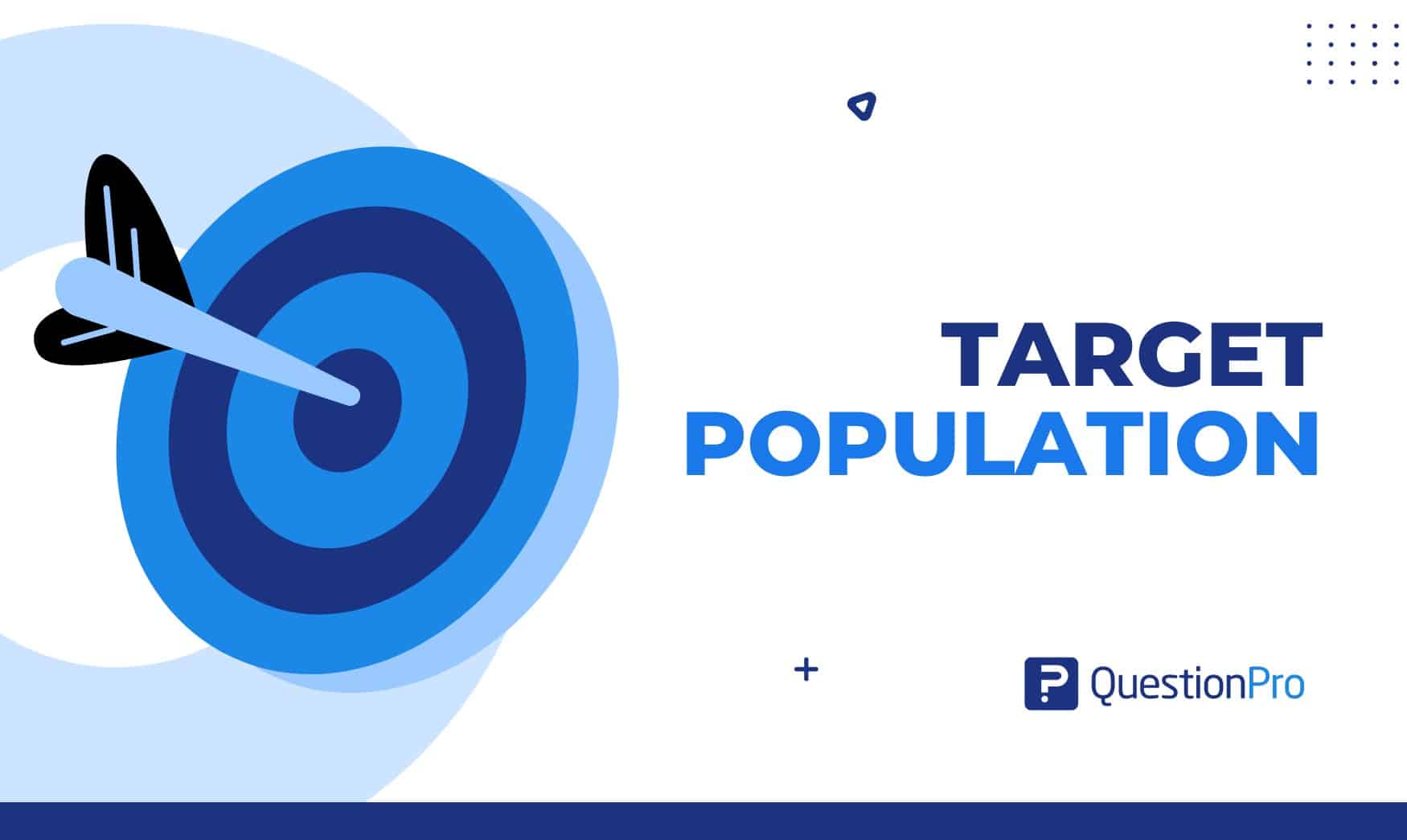
Target Population: What It Is + Strategies for Targeting
Aug 29, 2024
Microsoft Customer Voice vs QuestionPro: Choosing the Best
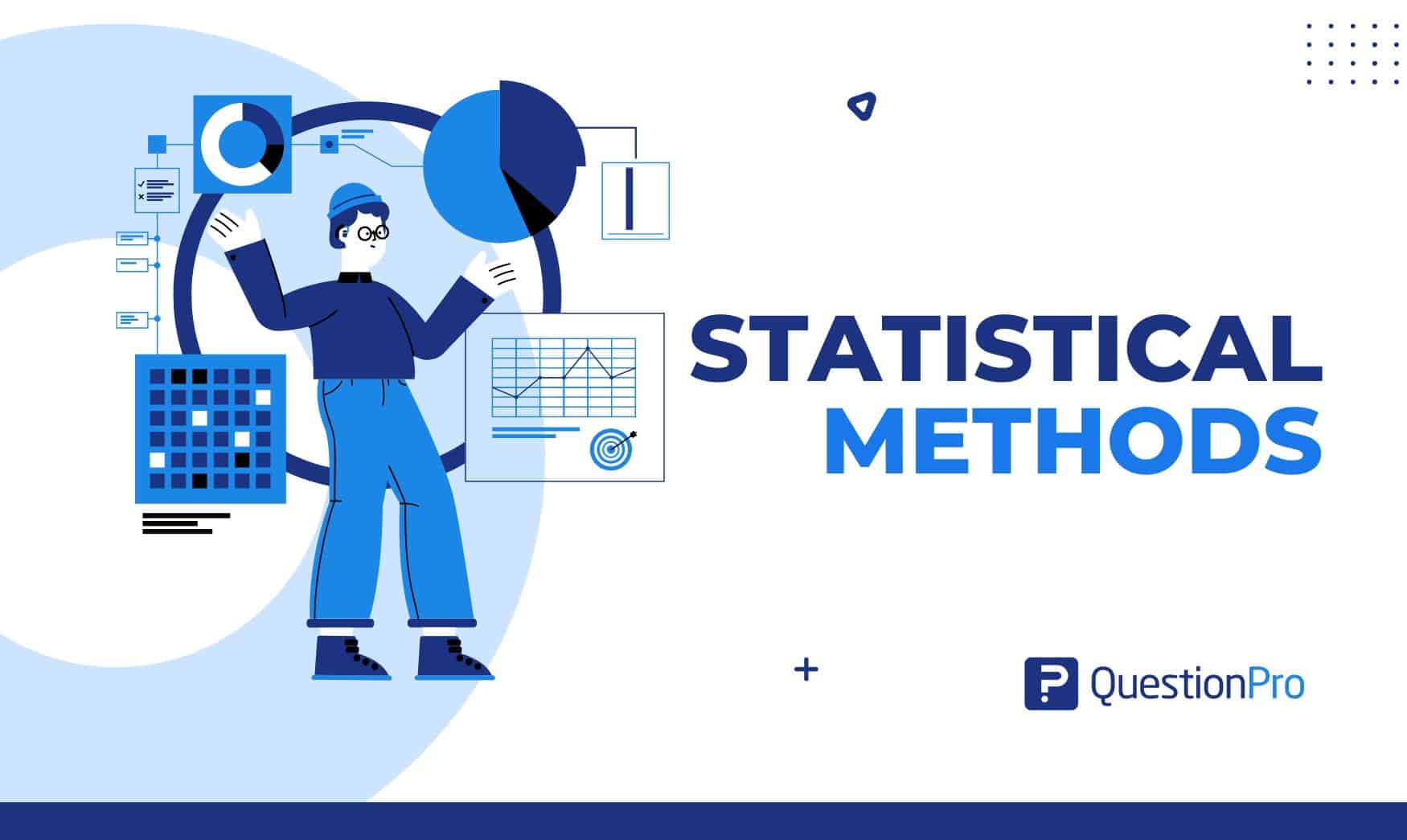
Statistical Methods: What It Is, Process, Analyze & Present
Aug 28, 2024
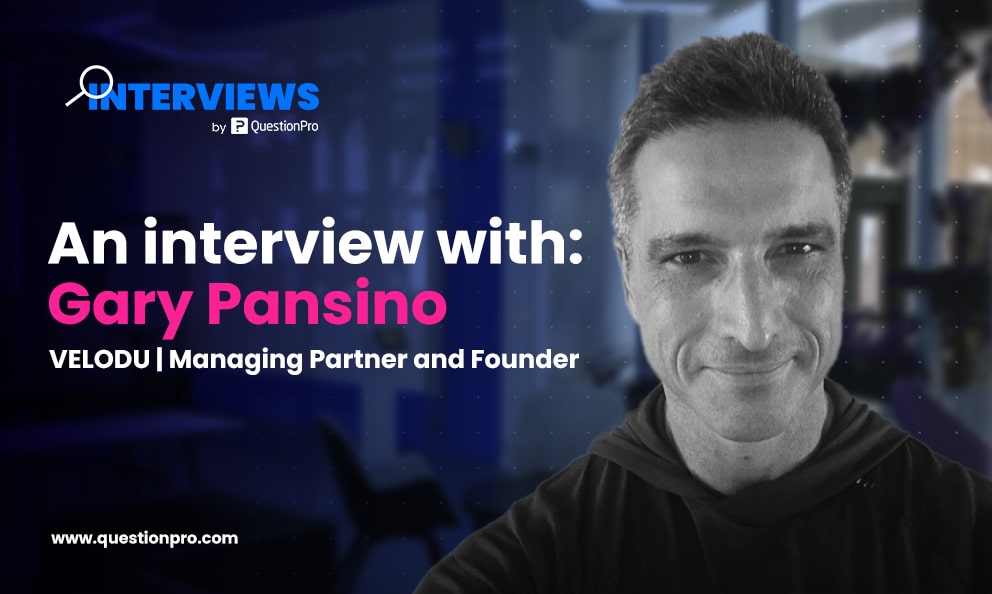
Velodu and QuestionPro: Connecting Data with a Human Touch
Other categories.
- Academic Research
- Artificial Intelligence
- Assessments
- Brand Awareness
- Case Studies
- Communities
- Consumer Insights
- Customer effort score
- Customer Engagement
- Customer Experience
- Customer Loyalty
- Customer Research
- Customer Satisfaction
- Employee Benefits
- Employee Engagement
- Employee Retention
- Friday Five
- General Data Protection Regulation
- Insights Hub
- Life@QuestionPro
- Market Research
- Mobile diaries
- Mobile Surveys
- New Features
- Online Communities
- Question Types
- Questionnaire
- QuestionPro Products
- Release Notes
- Research Tools and Apps
- Revenue at Risk
- Survey Templates
- Training Tips
- Tuesday CX Thoughts (TCXT)
- Uncategorized
- What’s Coming Up
- Workforce Intelligence
Evaluation and analysis of Data Management Plan tools: A parametric approach
- February 2021
- Information Processing & Management 58(3)

- Indian Statistical Institute, Bangalore Center

- Indian Statistical Institute, Bangalore, India

- SOL, University of Delhi

- Indian Statistical Institute, Bengaluru
Abstract and Figures

Discover the world's research
- 25+ million members
- 160+ million publication pages
- 2.3+ billion citations

- Dominik Brilhaus

- Patricia Henning
- Isabella Henrique Lima Pereira

- Carmela Freda

- Christopher Brewster

- J LIBR INF SCI

- Cristian-Alejandro Chisaba-Pereira

- Larry Schmidt
- Jennifer Kaari

- J INSECT SCI

- Paulo Augusto Cauchick-Miguel

- Recruit researchers
- Join for free
- Login Email Tip: Most researchers use their institutional email address as their ResearchGate login Password Forgot password? Keep me logged in Log in or Continue with Google Welcome back! Please log in. Email · Hint Tip: Most researchers use their institutional email address as their ResearchGate login Password Forgot password? Keep me logged in Log in or Continue with Google No account? Sign up
Business growth
Business tips
What is data analysis? Examples and how to get started
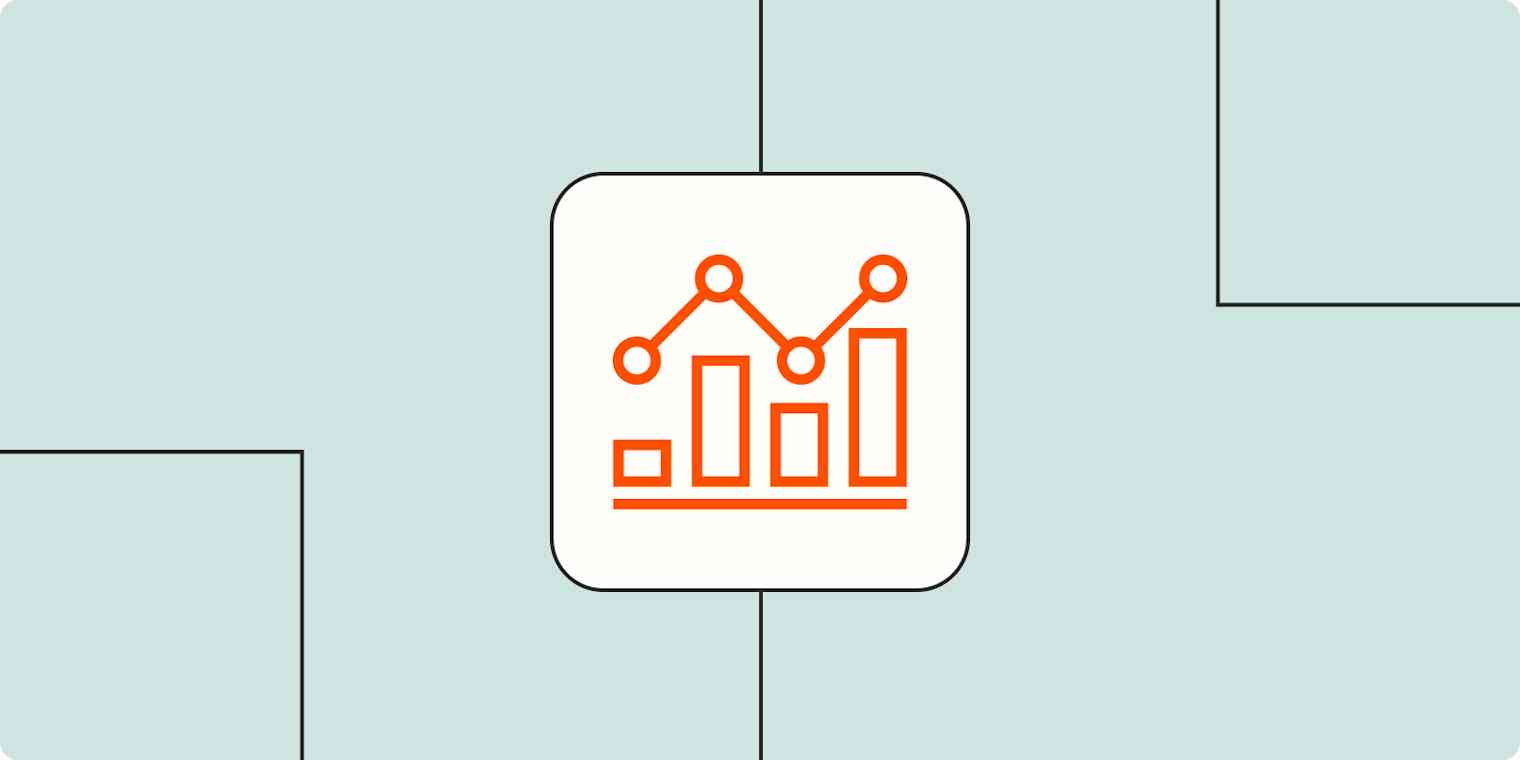
Even with years of professional experience working with data, the term "data analysis" still sets off a panic button in my soul. And yes, when it comes to serious data analysis for your business, you'll eventually want data scientists on your side. But if you're just getting started, no panic attacks are required.
Table of contents:
Quick review: What is data analysis?
Data analysis is the process of examining, filtering, adapting, and modeling data to help solve problems. Data analysis helps determine what is and isn't working, so you can make the changes needed to achieve your business goals.
Keep in mind that data analysis includes analyzing both quantitative data (e.g., profits and sales) and qualitative data (e.g., surveys and case studies) to paint the whole picture. Here are two simple examples (of a nuanced topic) to show you what I mean.
An example of quantitative data analysis is an online jewelry store owner using inventory data to forecast and improve reordering accuracy. The owner looks at their sales from the past six months and sees that, on average, they sold 210 gold pieces and 105 silver pieces per month, but they only had 100 gold pieces and 100 silver pieces in stock. By collecting and analyzing inventory data on these SKUs, they're forecasting to improve reordering accuracy. The next time they order inventory, they order twice as many gold pieces as silver to meet customer demand.
An example of qualitative data analysis is a fitness studio owner collecting customer feedback to improve class offerings. The studio owner sends out an open-ended survey asking customers what types of exercises they enjoy the most. The owner then performs qualitative content analysis to identify the most frequently suggested exercises and incorporates these into future workout classes.
Why is data analysis important?
Here's why it's worth implementing data analysis for your business:
Understand your target audience: You might think you know how to best target your audience, but are your assumptions backed by data? Data analysis can help answer questions like, "What demographics define my target audience?" or "What is my audience motivated by?"
Inform decisions: You don't need to toss and turn over a decision when the data points clearly to the answer. For instance, a restaurant could analyze which dishes on the menu are selling the most, helping them decide which ones to keep and which ones to change.
Adjust budgets: Similarly, data analysis can highlight areas in your business that are performing well and are worth investing more in, as well as areas that aren't generating enough revenue and should be cut. For example, a B2B software company might discover their product for enterprises is thriving while their small business solution lags behind. This discovery could prompt them to allocate more budget toward the enterprise product, resulting in better resource utilization.
Identify and solve problems: Let's say a cell phone manufacturer notices data showing a lot of customers returning a certain model. When they investigate, they find that model also happens to have the highest number of crashes. Once they identify and solve the technical issue, they can reduce the number of returns.
Types of data analysis (with examples)
There are five main types of data analysis—with increasingly scary-sounding names. Each one serves a different purpose, so take a look to see which makes the most sense for your situation. It's ok if you can't pronounce the one you choose.
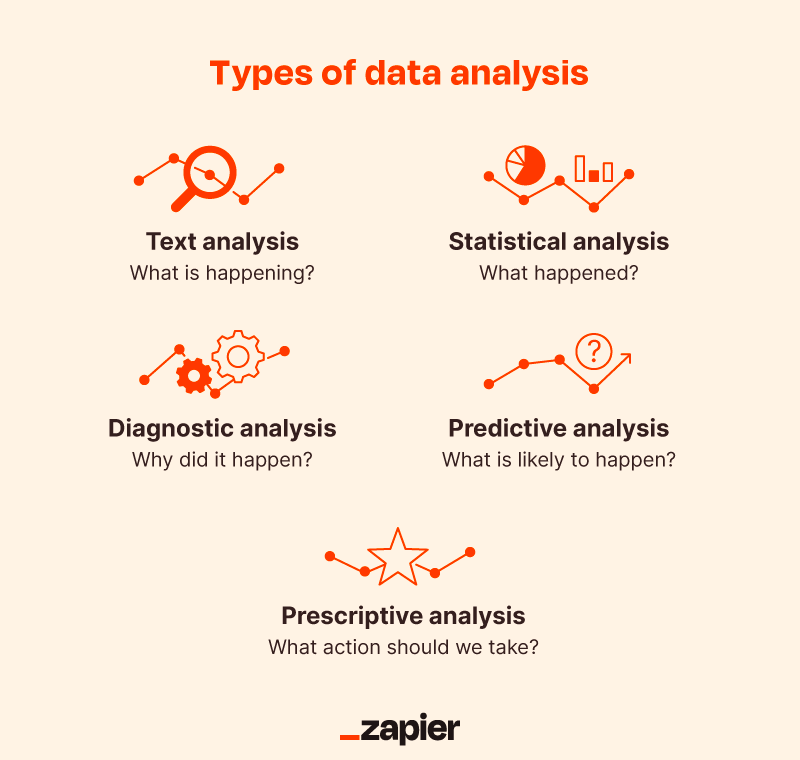
Text analysis: What is happening?
Here are a few methods used to perform text analysis, to give you a sense of how it's different from a human reading through the text:
Word frequency identifies the most frequently used words. For example, a restaurant monitors social media mentions and measures the frequency of positive and negative keywords like "delicious" or "expensive" to determine how customers feel about their experience.
Language detection indicates the language of text. For example, a global software company may use language detection on support tickets to connect customers with the appropriate agent.
Keyword extraction automatically identifies the most used terms. For example, instead of sifting through thousands of reviews, a popular brand uses a keyword extractor to summarize the words or phrases that are most relevant.
Statistical analysis: What happened?
Statistical analysis pulls past data to identify meaningful trends. Two primary categories of statistical analysis exist: descriptive and inferential.
Descriptive analysis
Here are a few methods used to perform descriptive analysis:
Measures of frequency identify how frequently an event occurs. For example, a popular coffee chain sends out a survey asking customers what their favorite holiday drink is and uses measures of frequency to determine how often a particular drink is selected.
Measures of central tendency use mean, median, and mode to identify results. For example, a dating app company might use measures of central tendency to determine the average age of its users.
Measures of dispersion measure how data is distributed across a range. For example, HR may use measures of dispersion to determine what salary to offer in a given field.
Inferential analysis
Inferential analysis uses a sample of data to draw conclusions about a much larger population. This type of analysis is used when the population you're interested in analyzing is very large.
Here are a few methods used when performing inferential analysis:
Hypothesis testing identifies which variables impact a particular topic. For example, a business uses hypothesis testing to determine if increased sales were the result of a specific marketing campaign.
Regression analysis shows the effect of independent variables on a dependent variable. For example, a rental car company may use regression analysis to determine the relationship between wait times and number of bad reviews.
Diagnostic analysis: Why did it happen?
Diagnostic analysis, also referred to as root cause analysis, uncovers the causes of certain events or results.
Here are a few methods used to perform diagnostic analysis:
Time-series analysis analyzes data collected over a period of time. A retail store may use time-series analysis to determine that sales increase between October and December every year.
Correlation analysis determines the strength of the relationship between variables. For example, a local ice cream shop may determine that as the temperature in the area rises, so do ice cream sales.
Predictive analysis: What is likely to happen?
Predictive analysis aims to anticipate future developments and events. By analyzing past data, companies can predict future scenarios and make strategic decisions.
Here are a few methods used to perform predictive analysis:
Decision trees map out possible courses of action and outcomes. For example, a business may use a decision tree when deciding whether to downsize or expand.
Prescriptive analysis: What action should we take?
The highest level of analysis, prescriptive analysis, aims to find the best action plan. Typically, AI tools model different outcomes to predict the best approach. While these tools serve to provide insight, they don't replace human consideration, so always use your human brain before going with the conclusion of your prescriptive analysis. Otherwise, your GPS might drive you into a lake.
Here are a few methods used to perform prescriptive analysis:
Algorithms are used in technology to perform specific tasks. For example, banks use prescriptive algorithms to monitor customers' spending and recommend that they deactivate their credit card if fraud is suspected.
Data analysis process: How to get started
The actual analysis is just one step in a much bigger process of using data to move your business forward. Here's a quick look at all the steps you need to take to make sure you're making informed decisions.
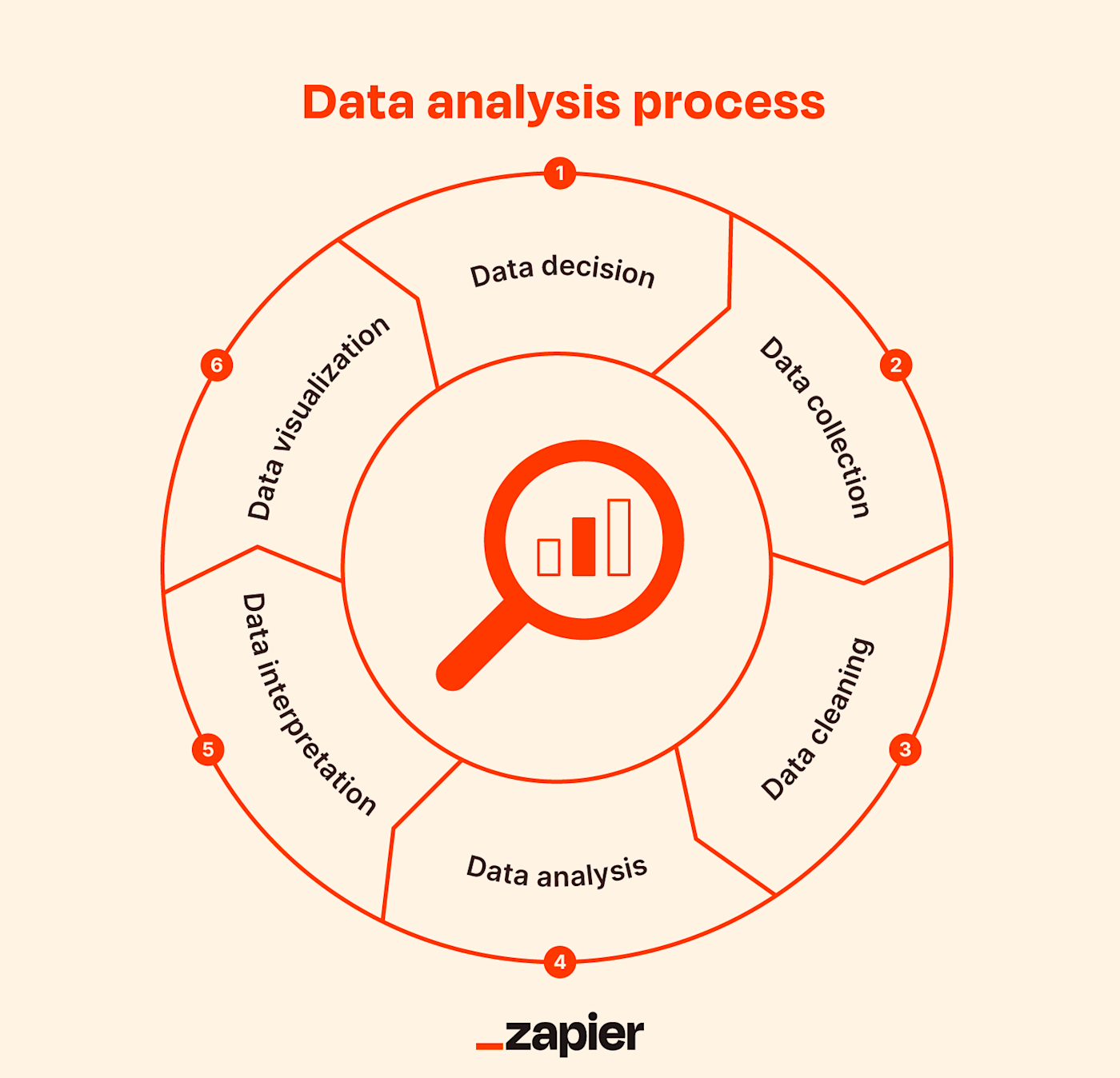
Data decision
As with almost any project, the first step is to determine what problem you're trying to solve through data analysis.
Make sure you get specific here. For example, a food delivery service may want to understand why customers are canceling their subscriptions. But to enable the most effective data analysis, they should pose a more targeted question, such as "How can we reduce customer churn without raising costs?"
Data collection
Next, collect the required data from both internal and external sources.
Internal data comes from within your business (think CRM software, internal reports, and archives), and helps you understand your business and processes.
External data originates from outside of the company (surveys, questionnaires, public data) and helps you understand your industry and your customers.
Data cleaning
Data can be seriously misleading if it's not clean. So before you analyze, make sure you review the data you collected. Depending on the type of data you have, cleanup will look different, but it might include:
Removing unnecessary information
Addressing structural errors like misspellings
Deleting duplicates
Trimming whitespace
Human checking for accuracy
Data analysis
Now that you've compiled and cleaned the data, use one or more of the above types of data analysis to find relationships, patterns, and trends.
Data analysis tools can speed up the data analysis process and remove the risk of inevitable human error. Here are some examples.
Spreadsheets sort, filter, analyze, and visualize data.
Structured query language (SQL) tools manage and extract data in relational databases.
Data interpretation
After you analyze the data, you'll need to go back to the original question you posed and draw conclusions from your findings. Here are some common pitfalls to avoid:
Correlation vs. causation: Just because two variables are associated doesn't mean they're necessarily related or dependent on one another.
Confirmation bias: This occurs when you interpret data in a way that confirms your own preconceived notions. To avoid this, have multiple people interpret the data.
Small sample size: If your sample size is too small or doesn't represent the demographics of your customers, you may get misleading results. If you run into this, consider widening your sample size to give you a more accurate representation.
Data visualization
Automate your data collection, frequently asked questions.
Need a quick summary or still have a few nagging data analysis questions? I'm here for you.
What are the five types of data analysis?
The five types of data analysis are text analysis, statistical analysis, diagnostic analysis, predictive analysis, and prescriptive analysis. Each type offers a unique lens for understanding data: text analysis provides insights into text-based content, statistical analysis focuses on numerical trends, diagnostic analysis looks into problem causes, predictive analysis deals with what may happen in the future, and prescriptive analysis gives actionable recommendations.
What is the data analysis process?
The data analysis process involves data decision, collection, cleaning, analysis, interpretation, and visualization. Every stage comes together to transform raw data into meaningful insights. Decision determines what data to collect, collection gathers the relevant information, cleaning ensures accuracy, analysis uncovers patterns, interpretation assigns meaning, and visualization presents the insights.
What is the main purpose of data analysis?
In business, the main purpose of data analysis is to uncover patterns, trends, and anomalies, and then use that information to make decisions, solve problems, and reach your business goals.
Related reading:
This article was originally published in October 2022 and has since been updated with contributions from Cecilia Gillen. The most recent update was in September 2023.
Get productivity tips delivered straight to your inbox
We’ll email you 1-3 times per week—and never share your information.
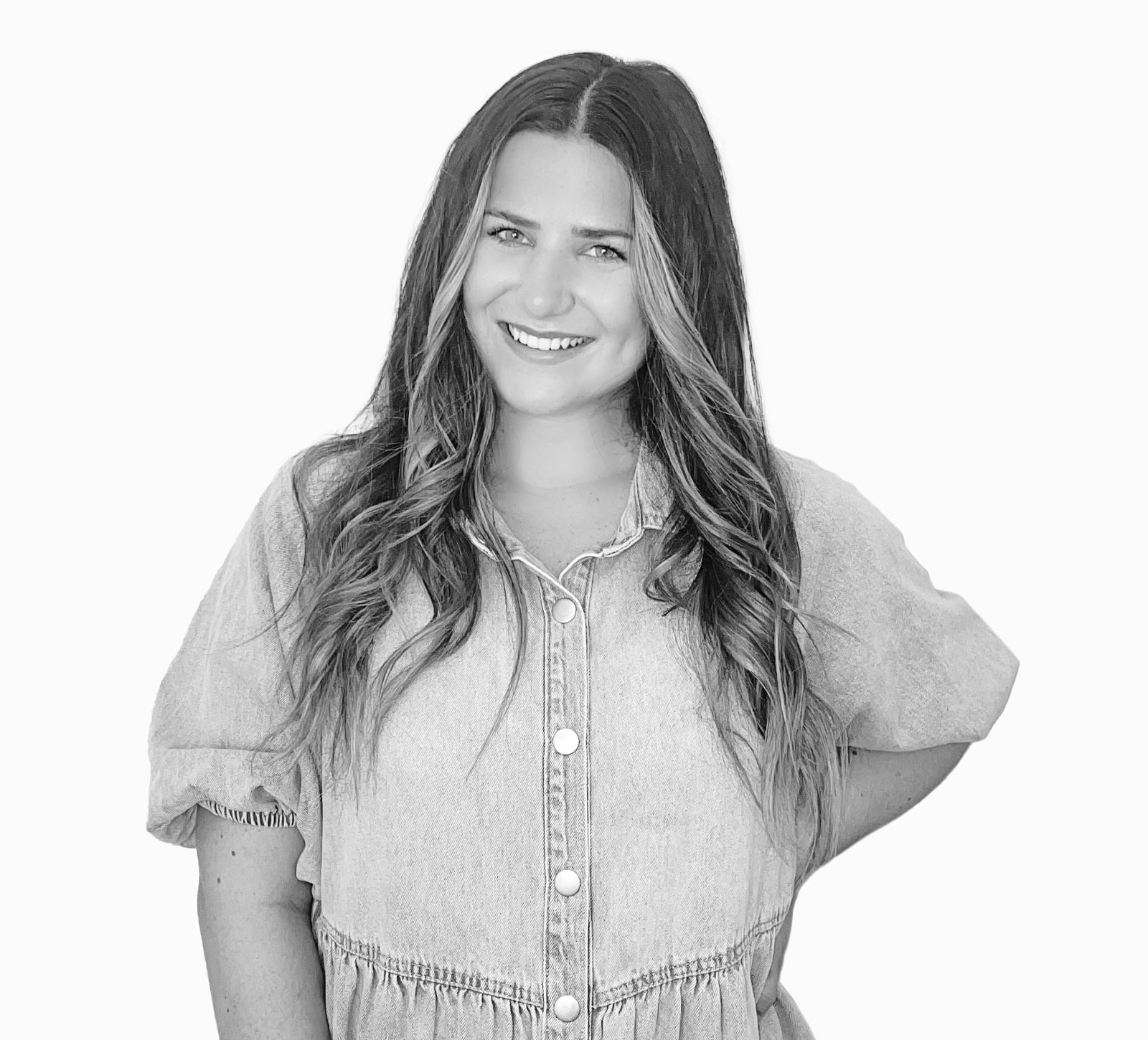
Shea Stevens
Shea is a content writer currently living in Charlotte, North Carolina. After graduating with a degree in Marketing from East Carolina University, she joined the digital marketing industry focusing on content and social media. In her free time, you can find Shea visiting her local farmers market, attending a country music concert, or planning her next adventure.
- Data & analytics
- Small business
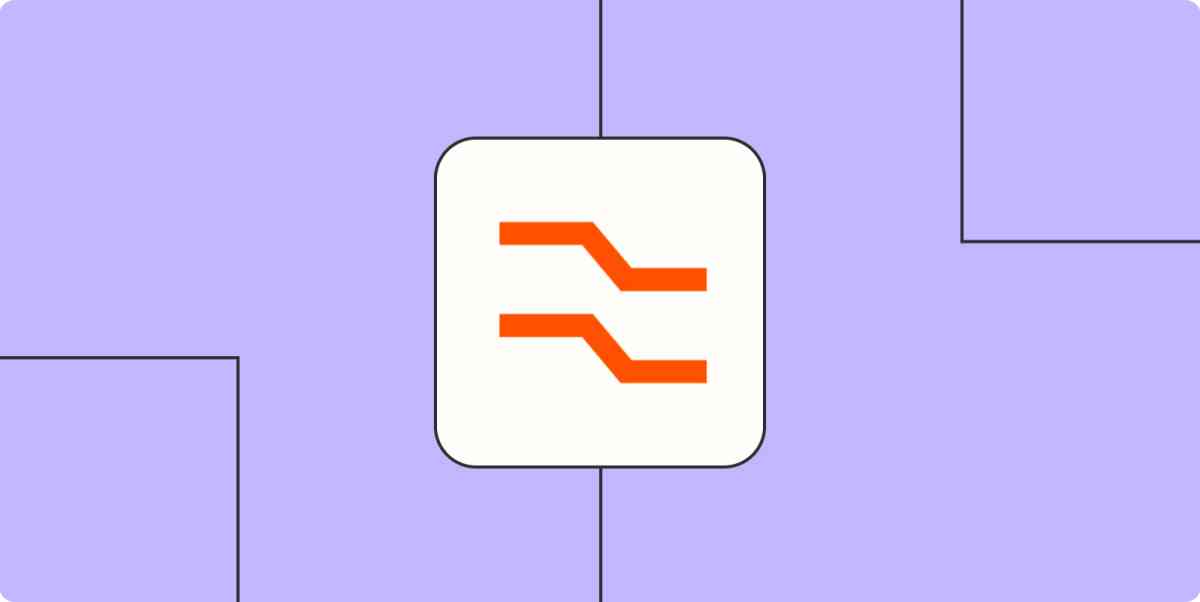
Data extraction is the process of taking actionable information from larger, less structured sources to be further refined or analyzed. Here's how to do it.
Related articles
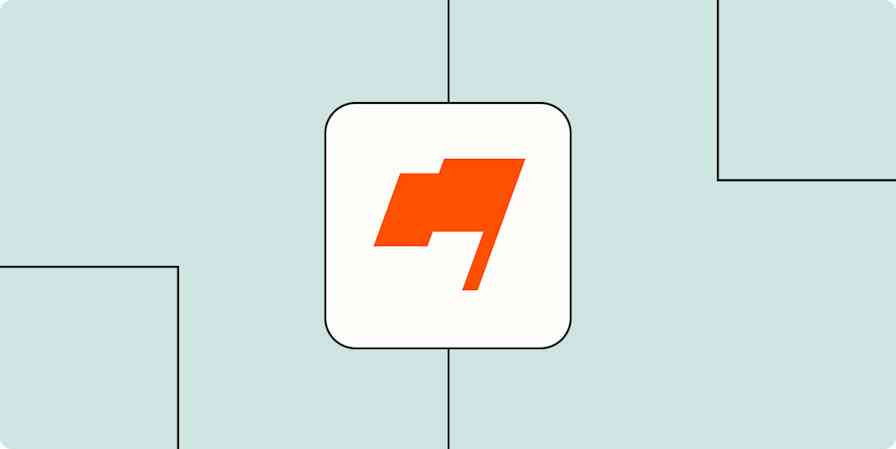
Project milestones for improved project management
Project milestones for improved project...
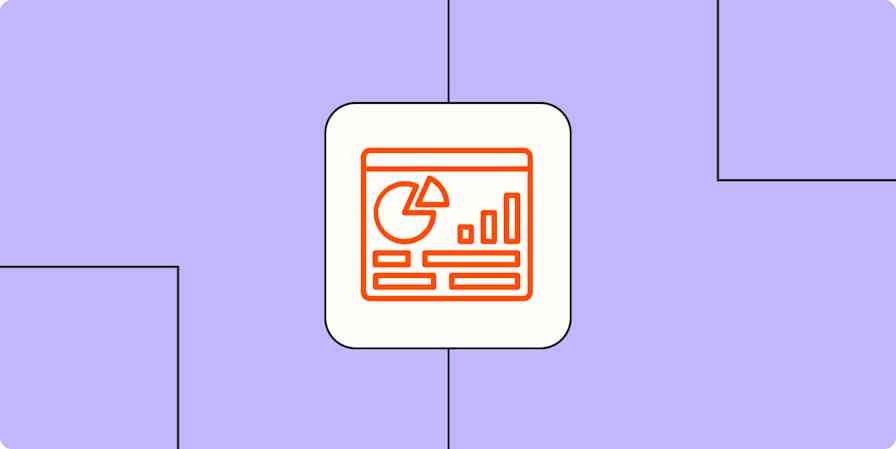
14 data visualization examples to captivate your audience
14 data visualization examples to captivate...
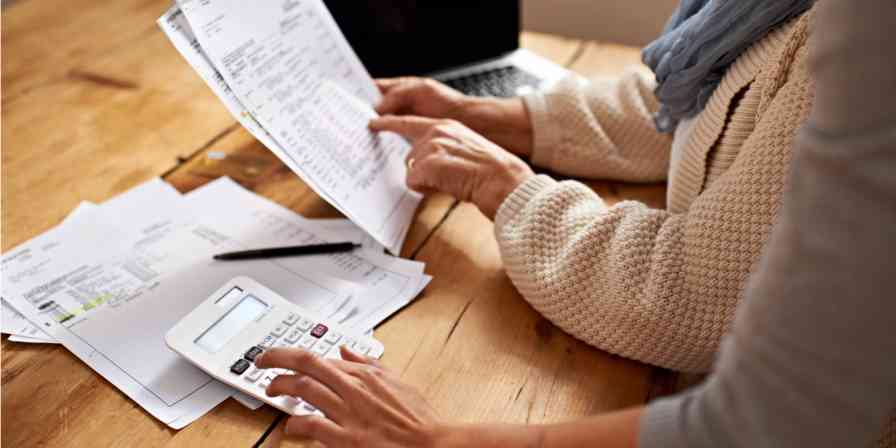
61 best businesses to start with $10K or less
61 best businesses to start with $10K or...
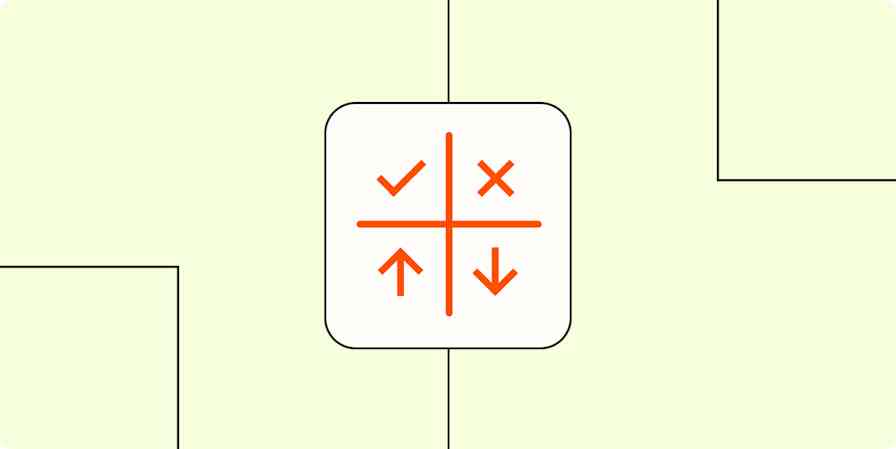
SWOT analysis: A how-to guide and template (that won't bore you to tears)
SWOT analysis: A how-to guide and template...
Improve your productivity automatically. Use Zapier to get your apps working together.
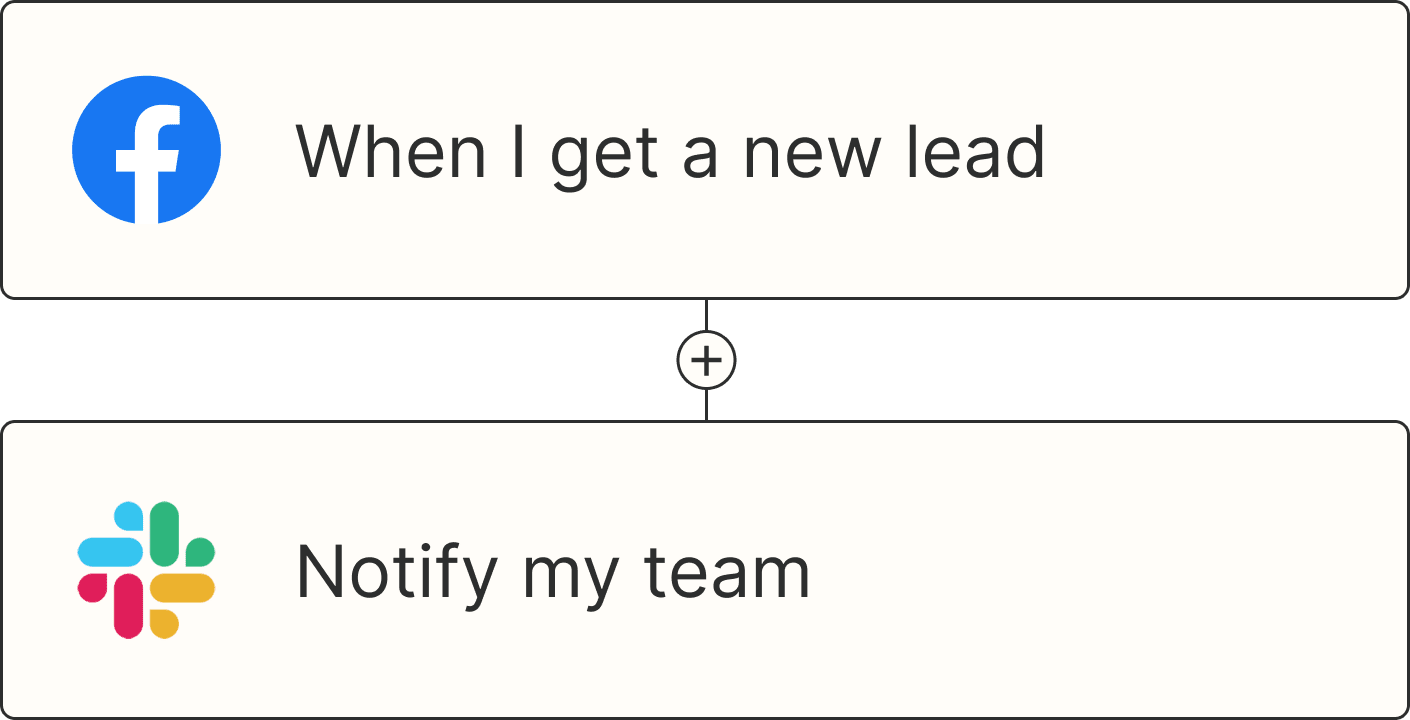
- Awards Season
- Big Stories
- Pop Culture
- Video Games
- Celebrities
Understanding the Key Features of LIMS System Software for Research Laboratories
In today’s fast-paced and data-driven research laboratories, managing and organizing vast amounts of information can be a daunting task. That’s where Laboratory Information Management System (LIMS) software comes into play. LIMS system software is designed to streamline laboratory operations, improve efficiency, and ensure compliance with industry regulations. In this article, we will explore the key features of LIMS system software and how it can benefit research laboratories.
Sample Tracking and Management
One of the primary functions of LIMS system software is sample tracking and management. With this feature, researchers can easily track the status and location of samples throughout their lifecycle – from collection to analysis and disposal. This eliminates the risk of misplacing or losing valuable samples, ensuring accurate results and reducing costly rework.
Additionally, LIMS system software allows for efficient sample registration by automating data entry processes. Researchers can simply scan or input barcode labels to quickly record sample information such as sample type, storage location, date collected, and more. This not only saves time but also minimizes human error in data entry.
Workflow Automation
Another crucial feature of LIMS system software is workflow automation. Research laboratories often have complex workflows involving multiple steps and personnel. Manual tracking of these workflows can be time-consuming and prone to errors.
LIMS system software offers automated workflows that streamline laboratory processes from start to finish. It allows researchers to define standard operating procedures (SOPs) for various experiments or tests, ensuring consistency across different projects. The software then guides users through each step of the workflow, providing prompts or reminders when necessary.
Moreover, LIMS system software enables real-time collaboration among team members by facilitating communication and task assignments within the platform itself. This promotes transparency and enhances productivity by eliminating delays caused by manual handoffs or miscommunication.
Data Management and Analysis
Accurate and reliable data management is crucial for research laboratories. LIMS system software provides a centralized database where researchers can store, organize, and analyze their experimental data. This eliminates the need for multiple spreadsheets or disparate systems, reducing the risk of data duplication or loss.
With LIMS system software, researchers can easily retrieve specific data sets or generate comprehensive reports with just a few clicks. The software also allows for customizable data visualization, making it easier to identify trends or patterns in large datasets.
Furthermore, LIMS system software often integrates with other analytical tools such as statistical analysis software or laboratory instruments. This seamless integration enables direct transfer of data from instruments to the LIMS platform, eliminating manual transcription errors and accelerating the analysis process.
Regulatory Compliance
Compliance with industry regulations is of utmost importance in research laboratories. Failure to meet regulatory requirements can result in severe consequences such as loss of accreditation or legal penalties. LIMS system software plays a crucial role in ensuring regulatory compliance by providing features such as audit trails and electronic signatures.
Audit trails allow laboratories to track every action taken within the LIMS system, providing a comprehensive record of all activities related to sample handling, data entry, and analysis. This not only ensures transparency but also facilitates traceability in case of any discrepancies or audits.
Electronic signatures provide an additional layer of security by verifying the identity of users and ensuring that only authorized personnel can access sensitive information within the LIMS platform. This feature helps laboratories maintain compliance with regulations pertaining to data security and privacy.
In conclusion, LIMS system software offers numerous key features that enhance efficiency and productivity in research laboratories. From sample tracking and management to workflow automation, data management and analysis to regulatory compliance – these features make LIMS system software an invaluable tool for modern-day laboratories seeking optimal performance and adherence to industry standards.
This text was generated using a large language model, and select text has been reviewed and moderated for purposes such as readability.
MORE FROM ASK.COM
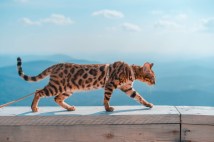

Style and Grammar Guidelines
APA Style provides a foundation for effective scholarly communication because it helps writers present their ideas in a clear, concise, and inclusive manner. When style works best, ideas flow logically, sources are credited appropriately, and papers are organized predictably. People are described using language that affirms their worth and dignity. Authors plan for ethical compliance and report critical details of their research protocol to allow readers to evaluate findings and other researchers to potentially replicate the studies. Tables and figures present information in an engaging, readable manner.
The style and grammar guidelines pages present information about APA Style as described in the Publication Manual of the American Psychological Association, Seventh Edition and the Concise Guide to APA Style, Seventh Edition . Any updates to APA Style are noted on the applicable topic pages. If you are still using the sixth edition, helpful resources are available in the sixth edition archive .
Looking for more style?
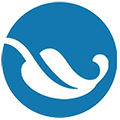
- Accessibility of APA Style
- Line Spacing
- Order of Pages
- Page Header
- Paragraph Alignment and Indentation
- Sample Papers
- Title Page Setup
- Appropriate Level of Citation
- Basic Principles of Citation
- Classroom or Intranet Sources
- Paraphrasing
- Personal Communications
- Quotations From Research Participants
- Secondary Sources
- Abbreviations
- Capitalization
- Italics and Quotation Marks
- Punctuation
- Spelling and Hyphenation
- General Principles for Reducing Bias
- Historical Context
- Intersectionality
- Participation in Research
- Racial and Ethnic Identity
- Sexual Orientation
- Socioeconomic Status
- Accessible Use of Color in Figures
- Figure Setup
- Sample Figures
- Sample Tables
- Table Setup
- Archival Documents and Collections
- Basic Principles of Reference List Entries
- Database Information in References
- DOIs and URLs
- Elements of Reference List Entries
- Missing Reference Information
- Reference Examples
- References in a Meta-Analysis
- Reference Lists Versus Bibliographies
- Works Included in a Reference List
- Active and Passive Voice
- Anthropomorphism
- First-Person Pronouns
- Logical Comparisons
- Plural Nouns
- Possessive Adjectives
- Possessive Nouns
- Singular “They”
- Adapting a Dissertation or Thesis Into a Journal Article
- Correction Notices
- Cover Letters
- Journal Article Reporting Standards (JARS)
- Open Science
- Response to Reviewers
Examining the Effect of Research Engagement on the Interest in Integrating Research into Future Career Paths Among Medical Graduates in China: An Instrumental Variable Analysis
- Original Research
- Published: 29 August 2024
Cite this article
- Guoyang Zhang ORCID: orcid.org/0000-0002-2335-1984 1 ,
- Xuanxuan Ma 3 , 5 &
- Hongbin Wu ORCID: orcid.org/0000-0002-4425-9845 4 , 5 , 6
While the importance of physician research has been underscored, a shortage of qualified physicians engaged in research persists. Early exposure to research could potentially ignite medical students’ interest in research, thereby motivating them to pursue research-related careers.
The study aims to examine early research engagement and medical graduates’ interest in incorporating research into their future career paths.
This was a national cross-sectional survey administered in 2020, with 152,624 medical students from 119 medical schools in China completing it. We selected and resampled the graduates’ data, and the final sample included 17,451 respondents graduating from 101 medical schools.
For graduates engaged in research, 63.4% (3054) had the interest in integrating research into their future careers. Such interest in research did differ between medical graduates with and without research engagement by linear probability regression ( β , 0.50; 95%CI, 0.48 to 0.52), but did not differ in instrumental variable regression analysis ( β , 0.31; 95%CI, − 0.18 to 0.80). Furthermore, engaging in research significantly increased the top 50% of academically ranked graduates’ research interest in instrumental variable regression analysis ( β , 0.44; 95%CI, 0.01 to 0.86).
Conclusions
Contrary to expectations, research engagement does not necessarily enhance medical graduates’ interest in integrating research into their future careers. However, graduates with strong academic performance are more inclined to develop this research interest. In light of these findings, we propose recommendations for nurturing research interest within medical education.
This is a preview of subscription content, log in via an institution to check access.
Access this article
Subscribe and save.
- Get 10 units per month
- Download Article/Chapter or eBook
- 1 Unit = 1 Article or 1 Chapter
- Cancel anytime
Price includes VAT (Russian Federation)
Instant access to the full article PDF.
Rent this article via DeepDyve
Institutional subscriptions
Data Availability
Data will be made available on request.
Cianciolo AT, et al. Physician-scientist or basic scientist? Exploring the nature of clinicians’ research engagement. Adv Health Sci Educ Theory Pract. 2021;26(2):353–67.
Article Google Scholar
Mokresh ME, et al. Knowledge, attitudes, and barriers toward research among medical students: a cross-sectional study in Turkey. Med Sci Educ. 2024;34(2):387–95.
Association of American Medical Colleges. Physician-scientists. 2023; https://www.aamc.org/what-we-do/mission-areas/medical-research/physician-scientist .
Schafer AI. The vanishing physician-scientist? Transl Res. 2010;155(1):1.
Paracha M, et al. Scholarly impact of student participation in radiation oncology research. Int J Radiat Oncol Biol Phys. 2018;101(4):779–83.
Amgad M, et al. Medical student research: an integrated mixed-methods systematic review and meta-analysis. PLoS One. 2015;10(6). https://doi.org/10.1371/journal.pone.0127470
Anderson DJ. Population and the environment: time for another contraception revolution. N Engl J Med. 2019;381(5):397–9.
Milewicz DM, et al. Rescuing the physician-scientist workforce: the time for action is now. J Clin Invest. 2015;125(10):3742–7.
Schroeder AR, et al. Outcomes of follow-up visits after bronchiolitis hospitalizations. JAMA Pediatr. 2018;172(3):296–7.
Ommering BWC, et al. Future physician-scientists: could we catch them young? Factors influencing intrinsic and extrinsic motivation for research among first-year medical students. Perspect Med Educ. 2018;7(4):248–55.
Goldacre M, et al. Junior doctors’ views about careers in academic medicine. Med Educ. 1999;33(5):318–26.
Rosenzweig EQ, et al Inside the STEM pipeline: changes in students’ biomedical career plans across the college years. Sci Adv 2021:7(18). https://doi.org/10.1126/sciadv.abe0985
Burgoyne LN, O’Flynn S, Boylan GB. Undergraduate medical research: the student perspective. Med Educ Online. 2010;15. https://doi.org/10.3402/meo.v15i0.5212
DiBiase RM, et al. A medical student scholarly concentrations program: scholarly self-efficacy and impact on future research activities. Med Educ Online. 2020;25(1):1786210.
Funston G, et al. Medical student perceptions of research and research-orientated careers: an international questionnaire study. Med Teach. 2016;38(10):1041–8.
Greenberg RB, et al. Medical student interest in academic medical careers: a multi-institutional study. Perspect Med Educ. 2013;2(5–6):298–316.
Ommering BWC, et al. Promoting positive perceptions of and motivation for research among undergraduate medical students to stimulate future research involvement: a grounded theory study. BMC Med Educ. 2020;20(1):204.
Adeboye W, et al. Predictors of self-reported research engagement and academic-career interest amongst medical students in the United Kingdom: a national cross-sectional survey. Postgrad Med J. 2023;99(1177):1189–96.
Colmenares C, Bierer SB, Graham LM. Impact of a 5-year research-oriented medical school curriculum on medical student research interest, scholarly output, and career intentions. Med Sci Educ. 2013;23(1):88–91.
Laskowitz DT, et al. Engaging students in dedicated research and scholarship during medical school: the long-term experiences at Duke and Stanford. Acad Med. 2010;85(3):419–28.
Stevenson MD, et al. Increasing scholarly activity productivity during residency: a systematic review. Acad Med. 2017;92(2):250–66.
Yuan HF, Xu WD, Hu HY. Young Chinese doctors and the pressure of publication. Lancet. 2013;381(9864). https://doi.org/10.1016/S0140-6736(13)60174-9
Philibert I, et al. Scholarly activity in the next accreditation system: moving from structure and process to outcomes. J Grad Med Educ. 2013;5(4):714–7.
Stone C, et al. Contemporary global perspectives of medical students on research during undergraduate medical education: a systematic literature review. Med Educ Online. 2018;23(1):1537430.
Hidi S, Renninger KA. The four-phase model of interest development. Educ Psychol. 2006;41(2):111–27.
Krapp A. An educational-psychological theory of interest and its relation to SDT. In: Deci EL, Ryan RM, editors. Handbook of self-determination research. Rochester (NY): University of Rochester Press; 2002. p. 405–27.
Google Scholar
Schiefele U, Krapp A, Winteler A. Interest as a predictor of academic achievement: a meta-analysis of research. In: Renninger KA, Hidi S, Krapp A, editors. The role of interest in learning and development. Hillsdale (NJ): Lawrence Erlbaum Associates; 1992. p. 183–212.
Houlden RL, et al. Medical students’ perceptions of an undergraduate research elective. Med Teach. 2004;26(7):659–61.
Burge SK, Hill JH. The medical student summer research program in family medicine. Fam Med. 2014;46(1):45–8.
Cain L, Kramer G, Ferguson M. The Medical Student Summer Research Program at the University of Texas Medical Branch at Galveston: building research foundations. Med Educ Online. 2019;24(1):1581523.
Association of American Medical Colleges. Medical School Graduation Questionnaire: 2020 All Schools Summary Report. 2021. Available from: https://www.aamc.org/media/46851/download .
Center for Postsecondary Research in the Indiana University School of Education. NSSE Survey instruments. 2013. Available from: https://nsse.indiana.edu/nsse/survey-instruments/index.html .
Zhang G, et al. The association between medical student research engagement with learning outcomes. Med Educ Online. 2022;27(1):2100039.
National Centre for Health Professions Education Development. China Medical Students Survey 2020 All Schools Summary Report. 2020. Available from: https://medu.bjmu.edu.cn/cms/show.action?code=publish_4028801e6bb6cf11016be526c0dc0014&siteid=100000&newsid=50947b6c789248b39fca817dfb9ba3ee&channelid=0000000008 .
Schwarz MR, Wojtczak A, Zhou T. Medical education in China’s leading medical schools. Med Teach. 2004;26(3):215–22.
Zhu J, Li W, Chen L. Doctors in China: improving quality through modernisation of residency education. Lancet. 2016;388(10054):1922–9.
Horrace WC, Oaxaca RL. Results on the bias and inconsistency of ordinary least squares for the linear probability model. Econ Lett. 2006;90(3):321–7.
Ministry of Education. Accreditation Standards for Basic Medical Education in China. 2016. Available from: http://ime.bjmu.edu.cn/cgzs/197708.htm .
de Oliveira NA, et al. Student views of research training programmes in medical schools. Med Educ. 2011;45(7):748–55.
Wolfson RK, et al. The impact of a scholarly concentration program on student interest in career-long research: a longitudinal study. Acad Med. 2017;92(8):1196–203.
O’Sullivan PS, et al. Becoming an academic doctor: perceptions of scholarly careers. Med Educ. 2009;43(4):335–41.
Baig SA, et al. Reasons behind the increase in research activities among medical students of Karachi, Pakistan, a low-income country. Educ Health (Abingdon). 2013;26(2):117.
Siemens DR, et al. A survey on the attitudes towards research in medical school. BMC Med Educ. 2010;10(1):1–7.
Mina S, et al. Perceived influential factors toward participation in undergraduate research activities among medical students at Alfaisal University—College of Medicine: a Saudi Arabian perspective. Med Teach. 2016;38(sup1). https://doi.org/10.3109/0142159X.2016.1142508
Ommering BWC, Dekker FW. Medical students’ intrinsic versus extrinsic motivation to engage in research as preparation for residency. Perspect Med Educ. 2017;6(6):366–8.
Download references
The research project was funded by the National Natural Science Foundation of China (Grant No. 72174013) and the China Scholarship Council (File No. 202208310033). The funders had no role in the design and development of the study protocol or the decision to publish.
Author information
Authors and affiliations.
School of Health Professions Education, Maastricht University, Maastricht, the Netherlands
Guoyang Zhang
Department of Critical Care Medicine, Zhongnan Hospital of Wuhan University, Wuhan, Hubei, People’s Republic of China
School of Public Health, Peking University, Beijing, People’s Republic of China
Xuanxuan Ma
Institute of Medical Education, Peking University, Beijing, People’s Republic of China
National Centre for Health Professions Education Development, Peking University, Beijing, People’s Republic of China
Xuanxuan Ma & Hongbin Wu
School of Medicine, The Fourth Affiliated Hospital of Zhejiang University, Yiwu, Zhejiang, People’s Republic of China
You can also search for this author in PubMed Google Scholar
Contributions
HBW made substantial contributions to the study conception and design. HBW and GYZ conducted the data analyses and drafted the manuscript. HBW interpreted the results with the support from GYZ, LL, and XXM. XXM made editing contributions. All authors reviewed the final manuscript and have approved the final version.
Corresponding author
Correspondence to Hongbin Wu .
Ethics declarations
Conflict of interest.
The authors report there are no competing interests to declare.
Additional information
Publisher's note.
Springer Nature remains neutral with regard to jurisdictional claims in published maps and institutional affiliations.
Supplementary Information
Below is the link to the electronic supplementary material.
Supplementary file1 (DOCX 15 KB)
Rights and permissions.
Springer Nature or its licensor (e.g. a society or other partner) holds exclusive rights to this article under a publishing agreement with the author(s) or other rightsholder(s); author self-archiving of the accepted manuscript version of this article is solely governed by the terms of such publishing agreement and applicable law.
Reprints and permissions
About this article
Zhang, G., Li, L., Ma, X. et al. Examining the Effect of Research Engagement on the Interest in Integrating Research into Future Career Paths Among Medical Graduates in China: An Instrumental Variable Analysis. Med.Sci.Educ. (2024). https://doi.org/10.1007/s40670-024-02152-3
Download citation
Accepted : 21 August 2024
Published : 29 August 2024
DOI : https://doi.org/10.1007/s40670-024-02152-3
Share this article
Anyone you share the following link with will be able to read this content:
Sorry, a shareable link is not currently available for this article.
Provided by the Springer Nature SharedIt content-sharing initiative
- Research engagement
- Research interest
- Medical graduates
- Instrumental variable analysis
- Find a journal
- Publish with us
- Track your research
Advisory boards aren’t only for executives. Join the LogRocket Content Advisory Board today →
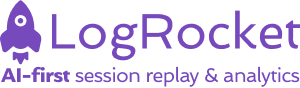
- Product Management
- Solve User-Reported Issues
- Find Issues Faster
- Optimize Conversion and Adoption
How to conduct a feasibility study: Template and examples
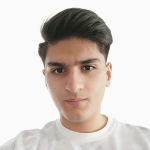
Editor’s note : This article was last updated on 27 August 2004 to bolster the step-by-step guide with more detailed instructions, more robust examples, and a downloadable, customizable template.
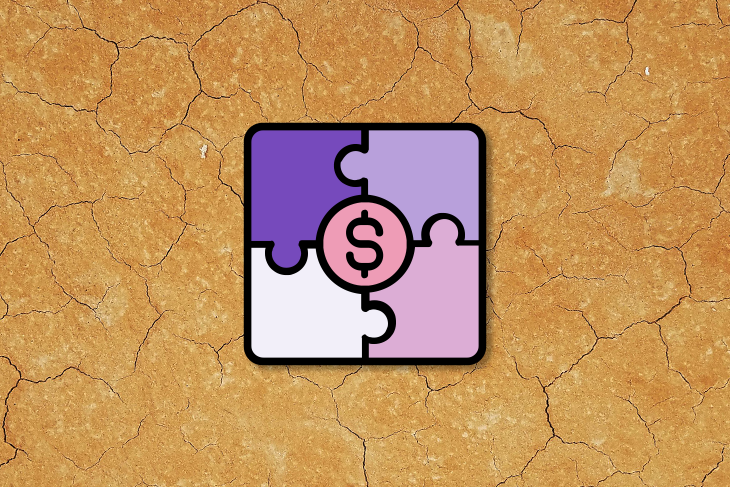
Opportunities are everywhere. Some opportunities are small and don’t require many resources. Others are massive and need further analysis and evaluation.
One of your key responsibilities as a product manager is to evaluate the potential success of those opportunities before investing significant money, time, and resources. A feasibility study, also known as a feasibility assessment or feasibility analysis, is a critical tool that can help product managers determine whether a product idea or opportunity is viable, feasible, and profitable.
So, what is a feasibility analysis? Why should product managers use it? And how do you conduct one?
Click here to download our customizable feasibility study template .
What is a feasibility study?
A feasibility study is a systematic analysis and evaluation of a product opportunity’s potential to succeed. It aims to determine whether a proposed opportunity is financially and technically viable, operationally feasible, and commercially profitable.
A feasibility study typically includes an assessment of a wide range of factors, including the technical requirements of the product, resources needed to develop and launch the product, the potential market gap and demand, the competitive landscape, and economic and financial viability. These factors can be broken down into different types of feasibility studies:
- Technical feasibility — Evaluates the technical resources and expertise needed to develop the product and identifies any technical challenges that could arise
- Financial feasibility — Analyzes the costs involved, potential revenue, and overall financial viability of the opportunity
- Market feasibility — Assesses the demand for the product, market trends, target audience, and competitive landscape
- Operational feasibility — Looks at the organizational structure, logistics, and day-to-day operations required to launch and sustain the product
- Legal feasibility — Examines any legal considerations, including regulations, patents, and compliance requirements that could affect the opportunity
Based on the analysis’s findings, the product manager and their product team can decide whether to proceed with the product opportunity, modify its scope, or pursue another opportunity and solve a different problem.
Conducting a feasibility study helps PMs ensure that resources are invested in opportunities that have a high likelihood of success and align with the overall objectives and goals of the product strategy .
What are feasibility analyses used for?
Feasibility studies are particularly useful when introducing entirely new products or verticals. Product managers can use the results of a feasibility study to:
- Assess the technical feasibility of a product opportunity — Evaluate whether the proposed product idea or opportunity can be developed with the available technology, tools, resources, and expertise
- Determine a project’s financial viability — By analyzing the costs of development, manufacturing, and distribution, a feasibility study helps you determine whether your product is financially viable and can generate a positive return on investment (ROI)
- Evaluate customer demand and the competitive landscape — Assessing the potential market size, target audience, and competitive landscape for the product opportunity can inform decisions about the overall product positioning, marketing strategies, and pricing
- Identify potential risks and challenges — Identify potential obstacles or challenges that could impact the success of the identified opportunity, such as regulatory hurdles, operational and legal issues, and technical limitations
- Refine the product concept — The insights gained from a feasibility study can help you refine the product’s concept, make necessary modifications to the scope, and ultimately create a better product that is more likely to succeed in the market and meet users’ expectations
How to conduct a feasibility study
The activities involved in conducting a feasibility study differ from one organization to another. Also, the threshold, expectations, and deliverables change from role to role. However, a general set of guidelines can help you get started.
Here are some basic steps to conduct and report a feasibility study for major product opportunities or features:
1. Clearly define the opportunity
Imagine your user base is facing a significant problem that your product doesn’t solve. This is an opportunity. Define the opportunity clearly, support it with data, talk to your stakeholders to understand the opportunity space, and use it to define the objective.
2. Define the objective and scope
Each opportunity should be coupled with a business objective and should align with your product strategy.
Determine and clearly communicate the business goals and objectives of the opportunity. Align those objectives with company leaders to make sure everyone is on the same page. Lastly, define the scope of what you plan to build.
3. Conduct market and user research
Now that you have everyone on the same page and the objective and scope of the opportunity clearly defined, gather data and insights on the target market.
Include elements like the total addressable market (TAM) , growth potential, competitors’ insights, and deep insight into users’ problems and preferences collected through techniques like interviews, surveys, observation studies, contextual inquiries, and focus groups.
4. Analyze technical feasibility
Suppose your market and user research have validated the problem you are trying to solve. The next step should be to, alongside your engineers, assess the technical resources and expertise needed to launch the product to the market.
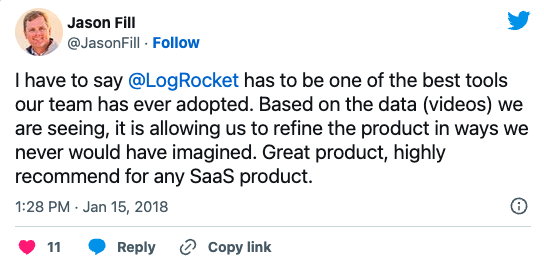
Over 200k developers and product managers use LogRocket to create better digital experiences

Dig deeper into the proposed solution and try to comprehend the technical limitations and estimated time required for the product to be in your users’ hands. A detailed assessment might include:
- Technical requirements — What technology stack is needed? Does your team have the necessary expertise? Are there any integration challenges?
- Development timeline — How long will it take to develop the solution? What are the critical milestones?
- Resource allocation — What resources (hardware, software, personnel) are required? Can existing resources be repurposed?
5. Assess financial viability
If your company has a product pricing team, work closely with them to determine the willingness to pay (WTP) and devise a monetization strategy for the new feature.
Conduct a comprehensive financial analysis, including the total cost of development, revenue streams, and the expected return on investment (ROI) based on the agreed-upon monetization strategy. Key elements to include:
- Cost analysis — Breakdown of development, production, and operational costs
- Revenue projections — Estimated revenue from different pricing models
- ROI calculation — Expected return on investment and payback period
6. Evaluate potential risks
Now that you have almost a complete picture, identify the risks associated with building and launching the opportunity. Risks may include things like regulatory hurdles, technical limitations, and any operational risks.
A thorough risk assessment should cover:
- Technical risks — Potential issues with technology, integration, or scalability.
- Market risks — Changes in market conditions, customer preferences, or competitive landscape.
- Operational risks — Challenges in logistics, staffing, or supply chain management.
- Regulatory risks — Legal or compliance issues that could affect the product’s launch. For more on regulatory risks, check out this Investopedia article .
7. Decide, prepare, and share
Based on the steps above, you should end up with a comprehensive report that helps you decide whether to pursue the opportunity, modify its scope, or explore alternative options. Here’s what you should do next:
- Prepare your report — Compile all your findings, including the feasibility analysis, market research, technical assessment, financial viability, and risk analysis into a detailed report. This document should provide a clear recommendation on whether to move forward with the project
- Create an executive summary — Summarize the key findings and recommendations in a concise executive summary , tailored for stakeholders such as the C-suite. The executive summary should capture the essence of your report, focusing on the most critical points
- Present to stakeholders — Share your report with stakeholders, ensuring you’re prepared to discuss the analysis and defend your recommendations. Make sure to involve key stakeholders early in the process to build buy-in and address any concerns they may have
- Prepare for next steps — Depending on the decision, be ready to either proceed with the project, implement modifications, or pivot to another opportunity. Outline the action plan, resource requirements, and timeline for the next phase
Feasibility study template
The following feasibility study report template is designed to help you evaluate the feasibility of a product opportunity and provide a comprehensive report to inform decision-making and guide the development process.
Note: You can customize this template to fit your specific needs. Click here to download and customize this feasibility study report template .
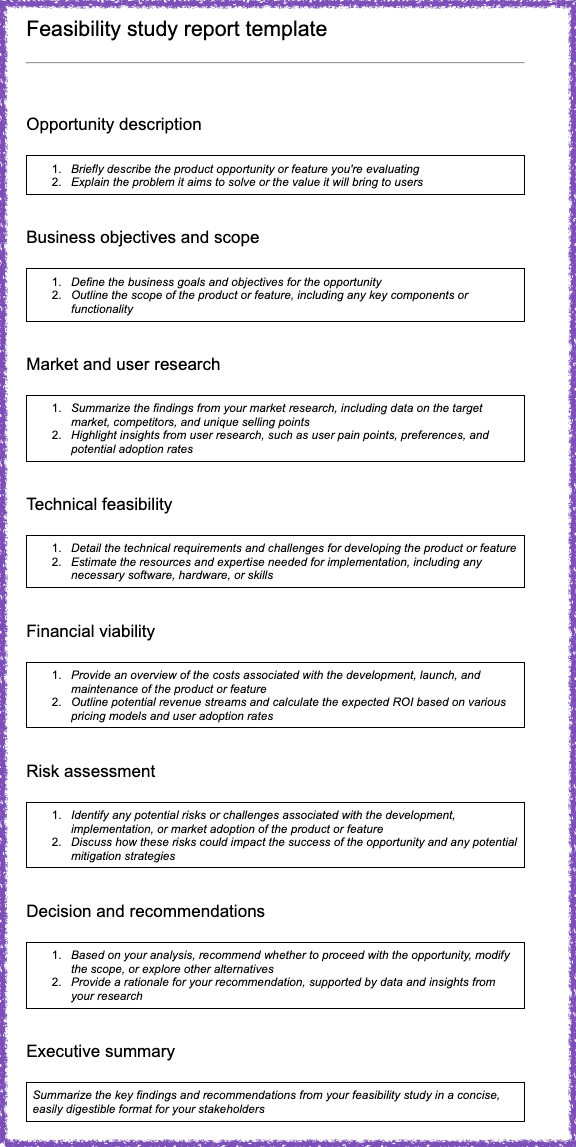
Feasibility study example
Imagine you’re a product manager at a company that specializes in project management tools. Your team has identified a potential opportunity to expand the product offering by developing a new AI-powered feature that can automatically prioritize tasks for users based on their deadlines, workload, and importance.
A feasibility study can help you assess the viability of this opportunity. Here’s how you might approach it according to the template above:
- Opportunity description — The opportunity lies in creating an AI-powered feature that automatically prioritizes tasks based on user-defined parameters such as deadlines, workload, and task importance. This feature is expected to enhance user productivity by helping teams focus on high-priority tasks and ensuring timely project completion
- Problem statement — Many users of project management tools struggle with managing and prioritizing tasks effectively, leading to missed deadlines and project delays. Current solutions often require manual input or lack sophisticated algorithms to adjust priorities dynamically. The proposed AI-powered feature aims to solve this problem by automating the prioritization process, thereby reducing manual effort and improving overall project efficiency
- Business objective — The primary objective is to increase user engagement and satisfaction by offering a feature that addresses a common pain point. The feature is also intended to increase customer retention by providing added value and driving user adoption
- Scope — The scope includes the development of an AI algorithm capable of analyzing task parameters (e.g., deadlines, workload) and dynamically prioritizing tasks. The feature will be integrated into the existing project management tool interface, with minimal disruption to current users. Additionally, the scope covers user training and support for the new feature
Market analysis:
- Total addressable market (TAM) — The TAM for this feature includes all users who actively manage projects and could benefit from enhanced task prioritization
- Competitor analysis — Competitor products such as Asana and Trello offer basic task prioritization features, but none use advanced AI algorithms. This presents a unique opportunity to differentiate this product by offering a more sophisticated solution
- User pain points — Surveys and interviews with current users reveal that 65 percent struggle with manual task prioritization, leading to inefficiencies and missed deadlines. Users expressed a strong interest in an automated solution that could save time and improve project outcomes
Technical requirements:
- AI algorithm development — The core of the feature is an AI algorithm that can analyze multiple factors to prioritize tasks. This requires expertise in machine learning, data processing, and AI integration
- Integration with existing infrastructure — The feature must seamlessly integrate with the existing architecture without causing significant disruptions. This includes data compatibility, API development, and UI/UX considerations
- Data handling and privacy — The feature will process sensitive project data, so robust data privacy and security measures must be implemented to comply with regulations like GDPR
Development timeline:
- Phase 1 (3 months) — Research and development of the AI algorithm, including training with sample datasets
- Phase 2 (2 months) — Integration with the platform, including UI/UX design adjustments
- Phase 3 (1 month) — Testing, quality assurance, and bug fixing
- Phase 4 (1 month) — User training materials and documentation preparation
Resource allocation:
- Development team — Two AI specialists, three backend developers, two frontend developers, one project manager
- Hardware/software — Additional cloud computing resources for AI processing, development tools for machine learning, testing environments
Cost analysis:
- Development costs — Estimated at $300,000, including salaries, cloud computing resources, and software licenses
- Marketing and launch costs — $50,000 for promotional activities, user onboarding, and initial support
- Operational costs — $20,000/year for maintenance, AI model updates, and ongoing support
Revenue projections:
- Pricing model — The AI-powered feature will be offered as part of a premium subscription tier, with an additional monthly fee of $10/user
- User adoption — Based on user surveys, an estimated 25 percent of the current user base (10,000 users) is expected to upgrade to the premium tier within the first year
- Projected revenue — First-year revenue is projected at $1.2 million, with an expected growth rate of 10 percent annually
ROI calculation:
- Break-even point — The project is expected to break even within 6 months of launch
- Five-year ROI — The feature is projected to generate a 200% ROI over five years, driven by increased subscription fees and user retention
Technical risks:
- AI algorithm complexity — Developing an accurate and reliable AI algorithm is challenging and may require multiple iterations
- Integration issues — There is a risk that integrating the new feature could disrupt the existing platform, leading to user dissatisfaction
Market risks:
- User adoption — There’s a risk that users may not perceive sufficient value in the AI feature to justify the additional cost, leading to lower-than-expected adoption rates
Operational risks:
- Support and maintenance — Maintaining the AI feature requires continuous updates and monitoring, which could strain the development and support teams
Regulatory risks:
- Data privacy compliance — Handling sensitive project data requires strict adherence to data privacy regulations. Noncompliance could lead to legal challenges and damage to the company’s reputation
- Decision — Based on the comprehensive analysis, the recommendation is to proceed with the development and launch of the AI-powered task prioritization feature. The potential for increased user engagement, differentiation from competitors, and positive ROI justifies the investment
- Prepare the report — A detailed report will be compiled, including all findings from the feasibility study, cost-benefit analysis, and risk assessments. This report will be presented to key stakeholders for approval
- Create an executive summary — A concise executive summary will be prepared for the C-suite, highlighting the key benefits, expected ROI, and strategic alignment with the company’s goals
- Next steps — Upon approval, the project will move into the development phase, following the timeline and resource allocation outlined in the study. Continuous monitoring and iterative improvements will be made based on user feedback and performance metrics
8. Executive summary
This feasibility study evaluates the potential for developing and launching an AI-powered task prioritization feature within our project management tool. The feature is intended to automatically prioritize tasks based on deadlines, workload, and task importance, thus improving user productivity and project efficiency. The study concludes that the feature is both technically and financially viable, with a projected ROI of 200 percent over five years. The recommendation is to proceed with development, as the feature offers a significant opportunity for product differentiation and user satisfaction.
Mock feasibility study report
Now let’s see what a feasibility study report based on the above example scenario would look like ( download an example here ):
Introduction
The purpose of this feasibility study is to assess the viability of introducing an AI-powered task prioritization feature into our existing project management software. This feature aims to address the common user challenge of manually prioritizing tasks, which often leads to inefficiencies and missed deadlines. By automating this process, we expect to enhance user productivity, increase customer retention, and differentiate our product in a competitive market.
Market and user research
The total addressable market (TAM) for this AI-powered task prioritization feature includes all current and potential users of project management tools who manage tasks and projects regularly. Based on market analysis, the current user base primarily consists of mid-sized enterprises and large organizations, where task management is a critical component of daily operations.
- Competitor analysis — Key competitors in the project management space, such as Asana and Trello, offer basic task prioritization features. However, these solutions lack advanced AI capabilities that dynamically adjust task priorities based on real-time data. This gap in the market presents an opportunity for us to differentiate our product by offering a more sophisticated, AI-driven solution
- User pain points — Surveys and interviews conducted with our current user base reveal that 65 percent of users experience challenges with manual task prioritization. Common issues include difficulty in maintaining focus on high-priority tasks, inefficient use of time, and the tendency to miss deadlines due to poor task management. Users expressed a strong interest in an automated solution that could alleviate these challenges, indicating a high demand for the proposed feature
Technical feasibility
- AI algorithm development — The core component of the feature is an AI algorithm capable of analyzing multiple task parameters, such as deadlines, workload, and task importance. The development of this algorithm requires expertise in machine learning, particularly in natural language processing (NLP) and predictive analytics. Additionally, data processing capabilities will need to be enhanced to handle the increased load from real-time task prioritization
- Integration with existing infrastructure — The AI-powered feature must be integrated into our existing project management tool with minimal disruption. This includes ensuring compatibility with current data formats, APIs, and the user interface. The integration will also require modifications to the UI/UX to accommodate the new functionality while maintaining ease of use for existing features
- Data handling and privacy — The feature will process sensitive project data, making robust data privacy and security measures critical. Compliance with regulations such as GDPR is mandatory, and the data flow must be encrypted end-to-end to prevent unauthorized access. Additionally, user consent will be required for data processing related to the AI feature
- Phase 1 (3 months) — Research and development of the AI algorithm, including dataset acquisition, model training, and initial testing
- Phase 2 (2 months) — Integration with the existing platform, focusing on backend development and UI/UX adjustments
- Phase 3 (1 month) — Extensive testing, quality assurance, and bug fixing to ensure stability and performance
- Phase 4 (1 month) — Development of user training materials, documentation, and preparation for the product launch
Financial analysis
- Development costs — Estimated at $300,000, covering salaries, cloud computing resources, machine learning tools, and necessary software licenses
- Marketing and launch costs — $50,000 allocated for promotional campaigns, user onboarding programs, and initial customer support post-launch
- Operational costs — $20,000 annually for ongoing maintenance, AI model updates, and customer support services
- Pricing model — The AI-powered task prioritization feature will be included in a premium subscription tier, with an additional monthly fee of $10 per user
- User adoption — Market research suggests that approximately 25% of the current user base (estimated at 10,000 users) is likely to upgrade to the premium tier within the first year
- Projected revenue — First-year revenue is estimated at $1.2 million, with an anticipated annual growth rate of 10% as more users adopt the feature
- Break-even point — The project is expected to reach its break-even point within 6 months of the feature’s launch
- Five-year ROI — Over a five-year period, the feature is projected to generate a return on investment (ROI) of 200 percent, driven by steady subscription revenue and enhanced user retention
Risk assessment
- AI algorithm complexity — Developing a sophisticated AI algorithm poses significant technical challenges, including the risk of inaccuracies in task prioritization. Multiple iterations and extensive testing will be required to refine the algorithm
- Integration issues — Integrating the new feature into the existing platform could potentially cause compatibility issues, resulting in performance degradation or user dissatisfaction
- User adoption — There is a possibility that users may not perceive enough value in the AI-powered feature to justify the additional cost, leading to lower-than-expected adoption rates and revenue
- Support and maintenance — The ongoing support and maintenance required for the AI feature, including regular updates and monitoring, could place a significant burden on the development and customer support teams, potentially leading to resource constraints
- Data privacy compliance — Handling sensitive user data for AI processing necessitates strict adherence to data privacy regulations such as GDPR. Failure to comply could result in legal repercussions and damage to the company’s reputation
Conclusion and recommendations
The feasibility study demonstrates that the proposed AI-powered task prioritization feature is both technically and financially viable. The feature addresses a significant user pain point and has the potential to differentiate the product in a competitive market. With an estimated ROI of 200 percent over five years and strong user interest, it is recommended that the project move forward into the development phase.
Next steps include finalizing the development plan, securing approval from key stakeholders, and initiating the development process according to the outlined timeline and resource allocation. Continuous monitoring and iterative improvements will be essential to ensure the feature meets user expectations and achieves the projected financial outcomes.
Overcoming stakeholder management challenges
The ultimate challenge that faces most product managers when conducting a feasibility study is managing stakeholders .
Stakeholders may interfere with your analysis, jumping to conclusions that your proposed product or feature won’t work and deeming it a waste of resources. They may even try to prioritize your backlog for you.
Here are some tips to help you deal with even the most difficult stakeholders during a feasibility study:
- Use hard data to make your point — Never defend your opinion based on your assumptions. Always show them data and evidence based on your user research and market analysis
- Learn to say no — You are the voice of customers, and you know their issues and how to monetize them. Don’t be afraid to say no and defend your team’s work as a product manager
- Build stakeholder buy-in early on — Engage stakeholders from the beginning of the feasibility study process by involving them in discussions and seeking their input. This helps create a sense of ownership and ensures that their concerns and insights are considered throughout the study
- Provide regular updates and maintain transparency — Keep stakeholders informed about the progress of the feasibility study by providing regular updates and sharing key findings. This transparency can help build trust, foster collaboration, and prevent misunderstandings or misaligned expectations
- Leverage stakeholder expertise — Recognize and utilize the unique expertise and knowledge that stakeholders bring to the table. By involving them in specific aspects of the feasibility study where their skills and experience can add value, you can strengthen the study’s outcomes and foster a more collaborative working relationship
Final thoughts
A feasibility study is a critical tool to use right after you identify a significant opportunity. It helps you evaluate the potential success of the opportunity, analyze and identify potential challenges, gaps, and risks in the opportunity, and provides a data-driven approach in the market insights to make an informed decision.
By conducting a feasibility study, product teams can determine whether a product idea is profitable, viable, feasible, and thus worth investing resources into. It is a crucial step in the product development process and when considering investments in significant initiatives such as launching a completely new product or vertical.
For a more detailed approach and ready-to-use resources, consider using the feasibility study template provided in this post. If you’re dealing with challenging stakeholders, remember the importance of data-driven decisions, maintaining transparency, and leveraging the expertise of your team.
LogRocket generates product insights that lead to meaningful action
Get your teams on the same page — try LogRocket today.
Share this:
- Click to share on Twitter (Opens in new window)
- Click to share on Reddit (Opens in new window)
- Click to share on LinkedIn (Opens in new window)
- Click to share on Facebook (Opens in new window)
- #product strategy
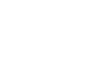
Stop guessing about your digital experience with LogRocket
Recent posts:.
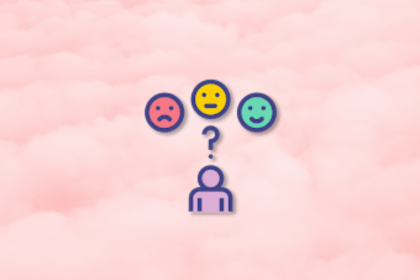
Feedback management tools and strategies
Feedback management refers to a structured process for gathering and analyzing feedback to improve products, services, or processes.
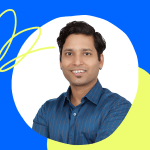
An overview of feature-driven development (FDD)
FDD is an agile framework for software development that emphasizes incremental and iterative progress on product features development.
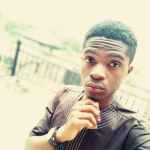
Leader Spotlight: Making the customer feel like a regular, with Judy Yao
Judy Yao talks about creating a digital experience that makes customers feel as if they were repeat, familiar customers in a physical store.
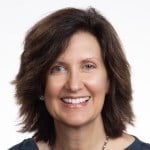
How can PMs benefit from generative AI
AI amplifies your potential when you use it as a co-pilot. However, don’t forget you’re the driver, not the passenger.
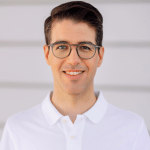
Leave a Reply Cancel reply
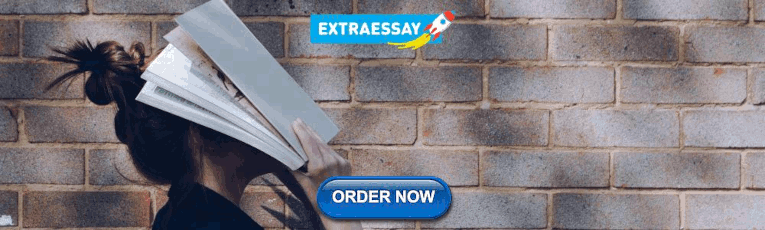
IMAGES
VIDEO
COMMENTS
What Is Data Analysis? (With Examples) Data analysis is the practice of working with data to glean useful information, which can then be used to make informed decisions. "It is a capital mistake to theorize before one has data. Insensibly one begins to twist facts to suit theories, instead of theories to suit facts," Sherlock Holme's proclaims ...
Will all data be used in the analysis, or will subsets of the data be analyzed? To speed analysis, the researcher will sometimes want to work with a subset of fields rather than all database fields within a record at once. In other cases, only a subset of records will be analyzed. For example, a research may investigate traffic flow and speed
Examples of data management plans. These examples of data management plans (DMPs) were provided by University of Minnesota researchers. They feature different elements. One is concise and the other is detailed. One utilizes secondary data, while the other collects primary data. Both have explicit plans for how the data is handled through the ...
This article is a practical guide to conducting data analysis in general literature reviews. The general literature review is a synthesis and analysis of published research on a relevant clinical issue, and is a common format for academic theses at the bachelor's and master's levels in nursing, physiotherapy, occupational therapy, public health and other related fields.
INTRODUCTION. In an earlier paper, 1 we presented an introduction to using qualitative research methods in pharmacy practice. In this article, we review some principles of the collection, analysis, and management of qualitative data to help pharmacists interested in doing research in their practice to continue their learning in this area.
Data analysis techniques in research are essential because they allow researchers to derive meaningful insights from data sets to support their hypotheses or research objectives.. Data Analysis Techniques in Research: While various groups, institutions, and professionals may have diverse approaches to data analysis, a universal definition captures its essence.
The first step in a data analysis plan is to describe the data collected in the study. This can be done using figures to give a visual presentation of the data and statistics to generate numeric descriptions of the data. Selection of an appropriate figure to represent a particular set of data depends on the measurement level of the variable.
Why Manage Data?Research today not only has to be rigorous, innovative, and insightful - it also has to be organized! As improved technology creates more capacity to create and store data, it increases the challenge of making data FAIR: Findable, Accessible, Interoperable, and Reusable (The FAIR Guiding Principles for scientific data management and stewardship).
The DMPTool allows you to create data management plans from templates based on funder requirements using a quick-and-easy click-through wizard.. DMPTool is a collaborative effort between several universities to streamline the data management planning process. The DMPTool supports the majority of federal and many non-profit and private funding agencies that require data management plans as part ...
A DMP (or DMSP, Data Management and Sharing Plan) describes what data will be acquired or generated as part of a research project, how the data will be managed, described, analyzed, and stored, and what mechanisms will be used to at the end of your project to share and preserve the data. One of the key advantages to writing a DMP is that it ...
Definition of research in data analysis: According to LeCompte and Schensul, research data analysis is a process used by researchers to reduce data to a story and interpret it to derive insights. The data analysis process helps reduce a large chunk of data into smaller fragments, which makes sense. Three essential things occur during the data ...
Research data management (RDM) is a term that describes the organization, storage, preservation, and sharing of data collected and used in a research project. It involves the daily management of research data throughout the life of a research project, such as using consistent file naming conventions. Examples of data management plans (DMPs) can ...
Data analysis is a comprehensive method of inspecting, cleansing, transforming, and modeling data to discover useful information, draw conclusions, and support decision-making. It is a multifaceted process involving various techniques and methodologies to interpret data from various sources in different formats, both structured and unstructured.
Earlier articles in the Ten Simple Rules series of PLOS Computational Biology provided guidance on getting grants [], writing research papers [], presenting research findings [], and caring for scientific data [].Here, I present ten simple rules that can help guide the process of creating an effective plan for managing research data—the basis for the project's findings, research papers ...
Analyse the data. By manipulating the data using various data analysis techniques and tools, you can find trends, correlations, outliers, and variations that tell a story. During this stage, you might use data mining to discover patterns within databases or data visualisation software to help transform data into an easy-to-understand graphical ...
Data Analysis. Definition: Data analysis refers to the process of inspecting, cleaning, transforming, and modeling data with the goal of discovering useful information, drawing conclusions, and supporting decision-making. It involves applying various statistical and computational techniques to interpret and derive insights from large datasets.
Table of contents. Step 1: Write your hypotheses and plan your research design. Step 2: Collect data from a sample. Step 3: Summarize your data with descriptive statistics. Step 4: Test hypotheses or make estimates with inferential statistics.
In this analysis we showcase RDM as consisting of (i) alignment of research management and data management, (ii) resourcing, (iii) researcher openness; and (iv) research data governance. While providing some additional insights the current analysis of a subset of the literature is limited in scope and a more comprehensive review of the ...
Data analytics is the collection, transformation, and organization of data in order to draw conclusions, make predictions, and drive informed decision making. Data analytics is often confused with data analysis. While these are related terms, they aren't exactly the same. In fact, data analysis is a subcategory of data analytics that deals ...
Research data management (or RDM) is the action of vigorously organizing, storing, and preserving data during the market research process. RDM covers the data lifecycle from planning through to people, process and technology, and long-term monitoring and access to the data. This is a continuous ongoing cycle during the process of data.
Abstract. This paper explores the openly available DMP tools and forms a comparative. analysis aimed at assisting researchers and data managers to formulate effec-. tive data management plans ...
Data analysis is the process of examining, filtering, adapting, and modeling data to help solve problems. Data analysis helps determine what is and isn't working, so you can make the changes needed to achieve your business goals. Keep in mind that data analysis includes analyzing both quantitative data (e.g., profits and sales) and qualitative ...
Data Management and Analysis. Accurate and reliable data management is crucial for research laboratories. LIMS system software provides a centralized database where researchers can store, organize, and analyze their experimental data. This eliminates the need for multiple spreadsheets or disparate systems, reducing the risk of data duplication ...
The long-term effects of COVID-19 are still being studied, and the incidence rate of LC may change over time. In the UK, studies have explored LC symptoms and risk factors in non-hospitalised individuals using primary care records 4 and consolidated evidence on persistent symptoms and their associations in broader populations. 5 Additionally, there has been significant interest in Patient ...
People are described using language that affirms their worth and dignity. Authors plan for ethical compliance and report critical details of their research protocol to allow readers to evaluate findings and other researchers to potentially replicate the studies. Tables and figures present information in an engaging, readable manner.
The value of physician research has been made clear; however, there exists a shortage of physician researchers, including physician-scientists and clinical researchers [1,2,3,4,5,6].Physician-scientists are uniquely positioned to bridge the gap between laboratory-based research and clinical practice, translating scientific discoveries into innovative medical treatments [3,4,5,6], and clinical ...
Please browse through our webpages to find the latest available health statistics and data analysis for Pennsylvania. Our statistical products cover a wide variety of health topics suitable for community health assessments, research, and public inquiry.
Accurate epidemiological data including changes over time for the UK are lacking, particularly in adults. Previous reports have analysed hospital admissions for food-induced anaphylaxis, and reported a three-fold increase (from 1·23 to 4·04 per 100 000 population per year) between 1998 and 2018, with the largest increase in children. 6 However, this is likely an underestimate as only a ...
Whether you are part of a small or large organization, learning how to effectively utilize data analytics can help you take advantage of the wide range of data-driven benefits. 1. RapidMiner. Primary use: Data mining. RapidMiner is a comprehensive package for data mining and model development.
Data handling and privacy — The feature will process sensitive project data, so robust data privacy and security measures must be implemented to comply with regulations like GDPR; Development timeline: Phase 1 (3 months) — Research and development of the AI algorithm, including training with sample datasets