Academia.edu no longer supports Internet Explorer.
To browse Academia.edu and the wider internet faster and more securely, please take a few seconds to upgrade your browser .
Enter the email address you signed up with and we'll email you a reset link.
- We're Hiring!
- Help Center
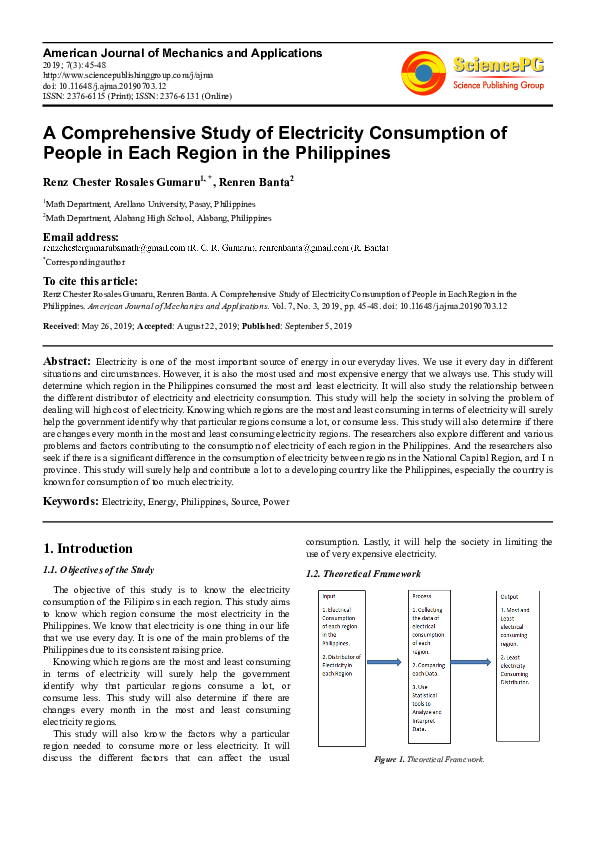
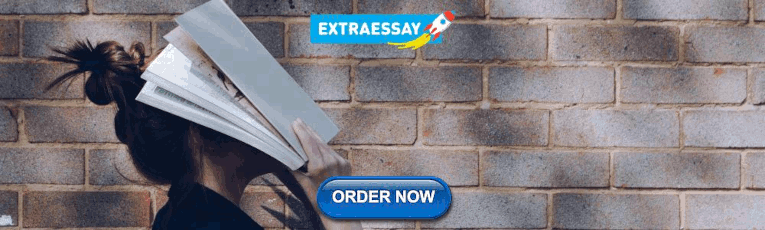
A Comprehensive Study of Electricity Consumption of People in Each Region in the Philippines

2019, American Journal of Mechanics and Applications
Related Papers
Elizabeth Remedio
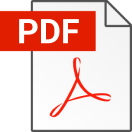
Eddie S. See , Mary Ann M. See
International Social Science Journal
RONALDO CABAUATAN
Energy for Sustainable Development
Muhammad Arif Wijaya
JIET (Jurnal Ilmu Ekonomi Terapan)
Jurnal Ilmu Ekonomi Terapan Universitas Airlangga
Electrical energy is one energy source that plays an essential role in human life daily, such as industrial, commercial, government, and household activities. All processes related to public activities can dash effectively and efficiently with electricity. The electricity consumption in Indonesia has reportedly increased every year. This enhancement can be caused by several factors, one of which is the population. The increasing electricity consumption in Indonesia has shown that electricity is the primary driving sector for the development that supports productivity and public activities. It is hoped that the economy will also increase. Based on this statement, this study aimed to analyze the factors which affect electricity consumption in Indonesia during the 2015-2019 period. This study used secondary data by taking four independent variables, including GDRP per capita, population, installed power capacity, and electricity tariffs. The dependent variable used in this study is electricity consumption. The research used the estimation technique of the Fixed Effect Model, which was selected based on the result of the Chow Test. The results in the regression analysis showed that the GDRP per capita and population variable both resulted in positive and insignificant effects on the electricity consumption in Indonesia during the 2015-2019 period. The installed power capacity variable had a positive and significant influence on the electricity consumption in Indonesia during the 2015-2019 period. Meanwhile, the electricity rate variable had a negative and insignificant effect on the electricity consumption in Indonesia during the 2015-2019 period.
International Journal of Social and Local Economic Governance
ghozali maski
asmarashid ponniran
Sustainability
Electricity-saving strategies are an essential solution to overcoming increasing global CO2 emission and electricity consumption problems; therefore, the determinant factors of electricity consumption in households need to be assessed. Most previous studies were conducted in developed countries of subtropical regions that had different household characteristic factors from those in developing countries of tropical regions. A field survey was conducted on electricity consumption for Malaysian households to investigate the factors affecting electricity consumption that focused on technology perspective (building and appliance characteristics) and socio-economic perspective (socio-demographics and occupant behaviour). To analyse the determinant factors of electricity consumption, direct and indirect questionnaire surveys were conducted from November 2017 to January 2018 among 214 university students. Direct questionnaire surveys were performed in order to obtain general information tha...
The Online Journal of Science and Technology
Enock Duodu
The study assessed the efficient utilization of electricity by domestic consumers in the Agona District. Descriptive survey design was employed in the study. Purposive and simple random sampling techniques were used in selecting five (5) towns and 100 respondents, respectively. A questionnaire as well as interview and observation methods were used in data collection. The data obtained from respondents were analyzed using frequencies and percentages. The study revealed that almost two-thirds (63%) of the respondents in the condominium consume electricity from a single central credit meters. Again, the study showed that lack of access to energy efficient technologies have contributed to the waste of electricity in the households. The results also revealed that consumers have little or no knowledge about some basic energy conservation tips. It is recommended that all households in such condominium should be provided with separate meters preferably the pre-paid meters so as to encourag...
Sekiguchi Global Research Association Forum in Waseda University, Tokyo - Seminar Proceedings
Stephanie N Gilles
Loading Preview
Sorry, preview is currently unavailable. You can download the paper by clicking the button above.
RELATED PAPERS
Anser Bashir
Global Journal of Science Frontier Research
P. Villeneuve
Immaculate Conception School of Malolos– Senior High School (ICSM-SHS)
Mark Lester S Chico
International Journal of Machine Learning and Computing
Allemar Jhone Delima
Roderick J . Lawrence
Forecasting Ten-years of Electricity Consumption in the Philippines Using ARIMA Model
Mekko Paul Malicay
International Journal of Renewable Energy Research
Hendra Pachri
Naila Nazir , Muhammad Rehman
International Journal of Energy Economics and Policy
Nidhi Tewathia
Bunda Kaysha , Karin Bestarina
Azahan Awang
The Explorer Islamabad
Energy Policy
Mehboob Ahmad
Indonesian Journal of Electrical Engineering and Computer Science
Indonesian Journal of Electrical Engineering and Computer Science , Mohammed Hussain
Alyssa Mari Catanjal
The Pakistan Development Review
Muhammad Nasir
- We're Hiring!
- Help Center
- Find new research papers in:
- Health Sciences
- Earth Sciences
- Cognitive Science
- Mathematics
- Computer Science
- Academia ©2024
Information
- Author Services
Initiatives
You are accessing a machine-readable page. In order to be human-readable, please install an RSS reader.
All articles published by MDPI are made immediately available worldwide under an open access license. No special permission is required to reuse all or part of the article published by MDPI, including figures and tables. For articles published under an open access Creative Common CC BY license, any part of the article may be reused without permission provided that the original article is clearly cited. For more information, please refer to https://www.mdpi.com/openaccess .
Feature papers represent the most advanced research with significant potential for high impact in the field. A Feature Paper should be a substantial original Article that involves several techniques or approaches, provides an outlook for future research directions and describes possible research applications.
Feature papers are submitted upon individual invitation or recommendation by the scientific editors and must receive positive feedback from the reviewers.
Editor’s Choice articles are based on recommendations by the scientific editors of MDPI journals from around the world. Editors select a small number of articles recently published in the journal that they believe will be particularly interesting to readers, or important in the respective research area. The aim is to provide a snapshot of some of the most exciting work published in the various research areas of the journal.
Original Submission Date Received: .
- Active Journals
- Find a Journal
- Proceedings Series
- For Authors
- For Reviewers
- For Editors
- For Librarians
- For Publishers
- For Societies
- For Conference Organizers
- Open Access Policy
- Institutional Open Access Program
- Special Issues Guidelines
- Editorial Process
- Research and Publication Ethics
- Article Processing Charges
- Testimonials
- Preprints.org
- SciProfiles
- Encyclopedia
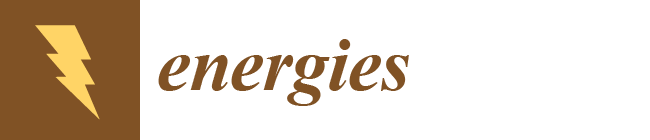
Article Menu
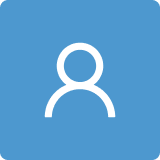
- Subscribe SciFeed
- Recommended Articles
- Google Scholar
- on Google Scholar
- Table of Contents
Find support for a specific problem in the support section of our website.
Please let us know what you think of our products and services.
Visit our dedicated information section to learn more about MDPI.
JSmol Viewer
Methods of forecasting electric energy consumption: a literature review.
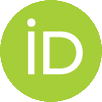
1. Introduction
- Conducted a comparative analysis of the methods by determining the main advantages and disadvantages, the scope, and specific features of the most popular methods used in forecasting electricity consumption.
- Conducted a comparative analysis of the accuracy of existing forecasting methods using the magnitude of the average absolute error in percent (MAPE).
- Conducted a comparative analysis of forecasting methods by the complexity of the implementation of the methods under consideration based on the level of requirements for computational capabilities and the complexity of reproducing the method.
- Conducted a comparative analysis of the methods of the requirements for the initial data set required for the application of the method.
- Identified the most promising and productive methods.
2. Methods and Materials
3. comparative analysis of forecasting methods, 3.1. operative forecasting, 3.2. short-term forecasting, 3.3. medium-term forecasting, 3.4. long-term forecasting, 4. conclusions, author contributions, data availability statement, conflicts of interest.
- Nadtoka, I.I. Development of the Theory and Methods of Modeling and Forecasting of Power Consumption Based on the Data of Accounting Automation and Tele-Measurements. Ph.D. Thesis, Platov South-Russian State Polytechnic University (NPI), Novocherkassk, Russia, 1998. [ Google Scholar ]
- Bunn, D.W.; Farmer, E.D. Comparative Models for Electrical Load Forecasting ; John Wiley & Sons: New York, NY, USA, 1985; p. 200. [ Google Scholar ]
- Zhang, Z.Z.; Hope, G.S.; Malik, O.P. Expert systems in electric power systems-a bibliographical survey. IEEE Trans. Power Syst. 1989 , 4 , 1355–1362. [ Google Scholar ] [ CrossRef ]
- Staroverov, B.A.; Shvedenko, V.N. Synthesis method of neural networks ensemble for electrical energy consumption forecast. Sci. Tech. Volga Reg. Bull. 2018 , 3 , 64–66. [ Google Scholar ] [ CrossRef ]
- Bouktif, S.; Fiaz, A.; Ouni, A.; Serhani, M.A. Optimal Deep Learning LSTM Model for Electric Load Forecasting using Feature Selection and Genetic Algorithm: Comparison with Machine Learning Approaches. Energies 2018 , 11 , 1636. [ Google Scholar ] [ CrossRef ] [ Green Version ]
- Meng, M.; Niu, D.; Sun, W. Forecasting Monthly Electric Energy Consumption Using Feature Extraction. Energies 2011 , 4 , 1495–1507. [ Google Scholar ] [ CrossRef ] [ Green Version ]
- Xiao, J.; Li, Y.; Xie, L.; Liu, D.; Huang, J. A hybrid model based on selective ensemble for energy consumption forecasting in China. Energy 2018 , 15915 , 534–546. [ Google Scholar ] [ CrossRef ]
- Hong, T.; Fan, S. Probabilistic electric load forecasting: A tutorial review. Int. J. Forecast. 2016 , 32 , 914–938. [ Google Scholar ] [ CrossRef ]
- Iria, J.; Soares, F.; Matos, M. Optimal bidding strategy for an aggregator of prosumers in energy and secondary reserve markets. Appl. Energy 2019 , 238 , 1361–1372. [ Google Scholar ] [ CrossRef ]
- Lee, D.; Chen, Y.-T.; Chao, S.-L. Universal workflow of artificial intelligence for energy saving. Energy Rep. 2022 , 8 , 1602–1633. [ Google Scholar ] [ CrossRef ]
- García-Martín, E.; Rodrigues, C.F.; Riley, G.; Grahn, H. Estimation of energy consumption in machine learning. J. Parallel Distrib. Comput. 2019 , 134 , 75–88. [ Google Scholar ] [ CrossRef ]
- Ghoddusi, H.; Creamer, G.G.; Rafizadeh, N. Machine learning in energy economics and finance: A review. Energy Econ. 2019 , 81 , 709–727. [ Google Scholar ] [ CrossRef ]
- González-Briones, A.; Hernandez, G.; Corchado, J.M.; Omatu, S.; Mohamad, M.S. Machine Learning Models for Electricity Consumption Forecasting: A Review. In Proceedings of the 2019 2nd International Conference on Computer Applications & Information Security (ICCAIS), Riyadh, Saudi Arabia, 1–3 May 2019; pp. 1–6. [ Google Scholar ] [ CrossRef ]
- von Scheidt, F.; Medinová, H.; Ludwig, N.; Richter, B.; Staudt, P.; Weinhardt, C. Data analytics in the electricity sector–A quantitative and qualitative literature review. Energy AI 2020 , 1 , 100009. [ Google Scholar ] [ CrossRef ]
- Shayuhov, T.T. Calculation of the specific norms and forecasting of energy consumption in industrial. Innov. Transp. 2016 , 3 , 8–12. [ Google Scholar ] [ CrossRef ]
- Biel, K.; Glock, C. Systematic literature review of decision support models for energy-efficient production planning. Comput. Ind. Eng. 2016 , 101 , 243–259. [ Google Scholar ] [ CrossRef ]
- Wei, N.; Li, C.; Peng, X.; Zeng, F.; Lu, X. Conventional models and artificial intelligence-based models for energy consumption forecasting: A review. J. Pet. Sci. Eng. 2019 , 181 , 106187. [ Google Scholar ] [ CrossRef ]
- Github.com. Available online: https://gist.github.com/ZohebAbai/266210c08da7894789d12d2f4a289238 (accessed on 30 October 2022).
- He, Y.; Zheng, Y.; Xu, Q. Forecasting energy consumption in Anhui province of China through two Box-Cox transformation quantile regression probability density methods. Measurement 2019 , 136 , 579–593. [ Google Scholar ] [ CrossRef ]
- Ning, Y.; Zhao, R.; Wang, S.; Yuan, B.; Wang, Y.; Zheng, D. Probabilistic short-term power load forecasting based on B-SCN. Energy Rep. 2022 , 8 , 646–655. [ Google Scholar ] [ CrossRef ]
- Li, Y.; Guo, X.; Gao, Y.; Yuan, B.; Wang, S. Short-term power load probabilistic interval multi-step forecasting based on ForecastNet. Energy Rep. 2022 , 8 , 133–140. [ Google Scholar ] [ CrossRef ]
- Henni, S.; Becker, J.; Staudt, P.; Scheidt, F.; Weinhardt, C. Industrial peak shaving with battery storage using a probabilistic forecasting approach: Economic evaluation of risk attitude. Appl. Energy 2022 , 327 , 120088. [ Google Scholar ] [ CrossRef ]
- Dumas, J.; Wehenkel, A.; Lanaspeze, D.; Cornélusse, B.; Sutera, A. A deep generative model for probabilistic energy forecasting in power systems: Normalizing flows. Appl. Energy 2021 , 305 , 117871. [ Google Scholar ] [ CrossRef ]
- Lu, S.; Xu, Q.; Jiang, C.; Liu, Y.; Kusiak, A. Probabilistic load forecasting with a non-crossing sparse-group Lasso-quantile regression deep neural network. Energy 2021 , 242 , 122955. [ Google Scholar ] [ CrossRef ]
- Zhang, Y.; Ma, R.; Liu, J.; Liu, X.; Petrosian, O.; Krinkin, K. Comparison and Explanation of Forecasting Algorithms for Energy Time Series. Mathematics 2021 , 9 , 2794. [ Google Scholar ] [ CrossRef ]
- Shumilova, G.P.; Gottman, N.E.; Starceva, T.B. Forecasting of Electrical Loads in the Operational Management of Electric Power Systems Based on Neural Network Structures ; KNC UrO RAS: Syktyvkar, Russia, 2008; p. 85. [ Google Scholar ]
- Hora, S.K.; Poongodan, R.; de Prado, R.P.; Wozniak, M.; Divakarachari, P.B. Long Short-Term Memory Network-Based Metaheuristic for Effective Electric Energy Consumption Prediction. Appl. Sci. 2021 , 11 , 11263. [ Google Scholar ] [ CrossRef ]
- Grant, J.; Eltoukhy, M.; Asfour, S. Short-Term Electrical Peak Demand Forecasting in a Large Government Building Using Artificial Neural Networks. Energies 2014 , 7 , 1935–1953. [ Google Scholar ] [ CrossRef ] [ Green Version ]
- Laayati, O.; Bouzi, M.; Chebak, A. Smart Energy Management System: Design of a Monitoring and Peak Load Forecasting System for an Experimental Open-Pit Mine. Appl. Syst. Innov. 2022 , 5 , 18. [ Google Scholar ] [ CrossRef ]
- Manusov, V.Z.; Matrenin, P.V.; Khasanzoda, N. Application of swarm intelligence algorithms to energy management by a generating consumer with renewable energy sources. Sci. Bull. Novosib. State Tech. Univ. 2019 , 3 , 115–134. [ Google Scholar ] [ CrossRef ]
- Jing, W.; Yu, J.; Luo, W.; Li, C.; Liu, X. Energy-saving diagnosis model of central air-conditioning refrigeration system in large shopping mall. Energy Rep. 2021 , 7 , 4035–4046. [ Google Scholar ] [ CrossRef ]
- Moradzadeh, A.; Moayyed, H.; Zakeri, S.; Mohammadi-Ivatloo, B.; Aguiar, A.P. Deep LearningAssisted Short-Term Load Forecasting for Sustainable Management of Energy in Microgrid. Inventions 2021 , 6 , 15. [ Google Scholar ] [ CrossRef ]
- Frikha, M.; Taouil, K.; Fakhfakh, A.; Derbel, F. Limitation of Deep-Learning Algorithm for Prediction of Power Consumption. Eng. Proc. 2022 , 18 , 26. [ Google Scholar ] [ CrossRef ]
- Lee, G.-C. Regression-Based Methods for Daily Peak Load Forecasting in South Korea. Sustainability 2022 , 14 , 3984. [ Google Scholar ] [ CrossRef ]
- Machado, E.; Pinto, T.; Guedes, V.; Morais, H. Electrical Load Demand Forecasting Using Feed-Forward Neural Networks. Energies 2021 , 14 , 7644. [ Google Scholar ] [ CrossRef ]
- Iruela, J.R.S.; Ruiz, L.G.B.; Capel, M.I.; Pegalajar, M.C. A TensorFlow Approach to Data Analysis for Time Series Forecasting in the Energy-Efficiency Realm. Energies 2021 , 14 , 4038. [ Google Scholar ] [ CrossRef ]
- Ibrahim, B.; Rabelo, L. A Deep Learning Approach for Peak Load Forecasting: A Case Study on Panama. Energies 2021 , 14 , 3039. [ Google Scholar ] [ CrossRef ]
- Szul, T.; Nęcka, K.; Lis, S. Application of the Takagi-Sugeno Fuzzy Modeling to Forecast Energy Efficiency in Real Buildings Undergoing Thermal Improvement. Energies 2021 , 14 , 1920. [ Google Scholar ] [ CrossRef ]
- Pîrjan, A.; Oprea, S.-V.; Căruțașu, G.; Petroșanu, D.-M.; Bâra, A.; Coculescu, C. Devising Hourly Forecasting Solutions Regarding Electricity Consumption in the Case of Commercial Center Type Consumers. Energies 2017 , 10 , 1727. [ Google Scholar ] [ CrossRef ] [ Green Version ]
- Iria, J.; Soares, F.; Matos, M. Optimal supply and demand bidding strategy for an aggregator of small prosumers. Appl. Energy 2018 , 213 , 658–669. [ Google Scholar ] [ CrossRef ]
- Ponoćko, J.; Milanović, J.V. Forecasting Demand Flexibility of Aggregated Residential Load Using Smart Meter Data. IEEE Trans. Power Syst. 2018 , 33 , 5446–5455. [ Google Scholar ] [ CrossRef ] [ Green Version ]
- Ponocko, J.; Cai, J.; Sun, Y.; Milanovic, J.V. Real-time visualisation of residential load flexibility for advanced demand side management. In Proceedings of the 19th IEEE Mediterranean Electrotechnical Conference (MELECON), Marrakech, Morocco, 2–7 May 2018; pp. 181–186. [ Google Scholar ] [ CrossRef ] [ Green Version ]
- Senchilo, N.; Babanova, I. Improving the Energy Efficiency of Electricity Distribution in the Mining Industry Using Distributed Generation by Forecasting Energy Consumption Using Machine Learning. In Proceedings of the International Multi-Conference on Industrial Engineering and Modern Technologies (FarEastCon), Vladivostok, Russia, 6–9 October 2020; pp. 1–7. [ Google Scholar ] [ CrossRef ]
- Rollert, K.E. The underlying factors in the uptake of electricity demand response: The case of Poland. Util. Policy 2018 , 54 , 11–21. [ Google Scholar ] [ CrossRef ]
- Zhukovskiy, Y.L.; Kovalchuk, M.S.; Batueva, D.E.; Senchilo, N.D. Development of an Algorithm for Regulating the Load Schedule of Educational Institutions Based on the Forecast of Electric Consumption within the Framework of Application of the Demand Response. Sustainability 2021 , 13 , 13801. [ Google Scholar ] [ CrossRef ]
- Singh, S.; Yassine, A. Big Data Mining of Energy Time Series for Behavioral Analytics and Energy Consumption Forecasting. Energies 2018 , 11 , 452. [ Google Scholar ] [ CrossRef ] [ Green Version ]
- Vyalkova, S.A.; Kornykova, O.A.; Nadtoka, I.I. Development of mathematical models for the short-term forecasting of daily consumption schedules of active power by Moscow. In Proceedings of the of the V International Scientific and Technical Conference «Problems of Machine Science», Omsk State Technical University, Omsk, Russia, 16–17 March 2021; pp. 166–173. [ Google Scholar ] [ CrossRef ]
- Rajbhandari, Y.; Marahatta, A.; Ghimire, B.; Shrestha, A.; Gachhadar, A.; Thapa, A.; Chapagain, K.; Korba, P. Impact Study of Temperature on the Time Series Electricity Demand of Urban Nepal for Short-Term Load Forecasting. Appl. Syst. Innov. 2021 , 4 , 43. [ Google Scholar ] [ CrossRef ]
- Oliveira, P.; Fernandes, B.; Analide, C.; Novais, P. Forecasting Energy Consumption of Wastewater Treatment Plants with a Transfer Learning Approach for Sustainable Cities. Electronics 2021 , 10 , 1149. [ Google Scholar ] [ CrossRef ]
- Tyunkov, D.A.; Gritsay, A.S.; Sapilova, A.A.; Blokhin, A.V.; Rodionov, V.S.; Potapov, V.I. Neirosetevaya model’ dlya kratkosrochnogo prognozirovaniya vyrabotki elektricheskoi energii solnechnymi elektrostantsiyami A neural network model for short-term forecasting of electricity generation by solar power plants. Sci. Bull. Novosib. State Tech. Univ. 2020 , 4 , 145–158. [ Google Scholar ] [ CrossRef ]
- Mokhov, V.G.; Tsimbol, V.I. Electrical Energy Consumption Prediction of the Federal District of Russia on the Based of the Reccurent Neural Network. J. Comput. Eng. Math. 2018 , 5 , 3–15. [ Google Scholar ] [ CrossRef ] [ Green Version ]
- Hernández, L.; Baladrón, C.; Aguiar, J.M.; Calavia, L.; Carro, B.; Sánchez-Esguevillas, A.; Pérez, F.; Fernández, Á.; Lloret, J. Artificial Neural Network for Short-Term Load Forecasting in Distribution Systems. Energies 2014 , 7 , 1576–1598. [ Google Scholar ] [ CrossRef ] [ Green Version ]
- Ryu, S.; Noh, J.; Kim, H. Deep Neural Network Based Demand Side Short Term Load Forecasting. Energies 2017 , 10 , 3. [ Google Scholar ] [ CrossRef ]
- Amber, K.P.; Aslam, M.W.; Mahmood, A.; Kousar, A.; Younis, M.Y.; Akbar, B.; Chaudhary, G.Q.; Hussain, S.K. Energy Consumption Forecasting for University Sector Buildings. Energies 2017 , 10 , 1579. [ Google Scholar ] [ CrossRef ]
- Alrasheedi, A.; Almalaq, A. Hybrid Deep Learning Applied on Saudi Smart Grids for Short-Term Load Forecasting. Mathematics 2022 , 10 , 2666. [ Google Scholar ] [ CrossRef ]
- Ikeda, S.; Nagai, T. A novel optimization method combining metaheuristics and machine learning for daily optimal operations in building energy and storage systems. Appl. Energy 2021 , 289 , 116716. [ Google Scholar ] [ CrossRef ]
- Amasyali, K.; El-Gohary, N. Machine learning for occupant-behavior-sensitive cooling energy consumption prediction in office buildings. Renew. Sustain. Energy Rev. 2021 , 142 , 110714. [ Google Scholar ] [ CrossRef ]
- Bagherzadeh, F.; Nouri, A.S.; Mehrani, M.-J.; Thennadil, S. Prediction of energy consumption and evaluation of affecting factors in a full-scale WWTP using a machine learning approach. Process Saf. Environ. Prot. 2021 , 154 , 458–466. [ Google Scholar ] [ CrossRef ]
- Flick, D.; Keck, C.; Herrmann, C.; Thiede, S. Machine learning based analysis of factory energy load curves with focus on transition times for anomaly detection. Procedia CIRP 2020 , 93 , 461–466. [ Google Scholar ] [ CrossRef ]
- Emaletdinova, L.Y.; Mukhametzyanov, Z.I.; Kataseva, D.V.; Kabirova, A.N. A method of constructing a predictive neural network model of a time series. Comput. Res. Model. 2020 , 12 , 737–756. [ Google Scholar ] [ CrossRef ]
- Serebryakov, N. Application of adaptive ensemble neural network method for short-term load forecasting electrical engineering complex of regional electric grid. Omsk. Sci. Bull. 2021 , 1 , 39–45. [ Google Scholar ] [ CrossRef ]
- Khomutov, S.O.; Stashko, V.I.; Serebryakov, N.A. Improving the accuracy of short-term load forecasting of delivery point cluster of the second level default provider. Bull. Tomsk. Polytech. Univ. Geo Assets Eng. 2020 , 331 , 128–140. [ Google Scholar ] [ CrossRef ]
- Vyalkova, S.A.; Nadtoka, I.I. Forecasting Daily Graphs Active Energy Consumption of a Megapolis Taking Into Account Forecast Data of Daylight Illumination. Russ. Electromechanics 2020 , 5 , 67–71. [ Google Scholar ] [ CrossRef ]
- Vyalkova, S.A.; Nadtoka, I.I. Analysis of the noise component of the daily schedules of active power energy systems and meteofactors at short-term forecasting. Smart Electr. Eng. 2018 , 4 , 25–34. [ Google Scholar ] [ CrossRef ]
- Skorokhodov, V.I.; Lysenko, O.A.; Simakov, A.V.; Gorovoy, S.A. Forecasting of electric energy consumption using the wavelet transform. Omsk. Sci. Bull. 2021 , 3 , 75–78. [ Google Scholar ] [ CrossRef ]
- Abu-Salih, B.; Wongthongtham, P.; Morrison, G.; Coutinho, K.; Al-Okaily, M.; Huneiti, A. Short-term renewable energy consumption and generation forecasting: A case study of Western Australia. Heliyon 2022 , 8 , e09152. [ Google Scholar ] [ CrossRef ]
- Aurangzeb, K. Short term power load forecasting using machine learning models for energy management in a smart community. In Proceedings of the 2019 International Conference on Computer and Information Sciences (ICCIS), Sakaka, Saudi Arabia, 3–4 April 2019; pp. 1–6. [ Google Scholar ] [ CrossRef ]
- Lago, J.; Marcjasz, G.; De Schutter, B.; Weron, R. Forecasting day-ahead electricity prices: A review of state-of-the-art algorithms, best practices and an open-access benchmark. Appl. Energy 2021 , 293 , 116983. [ Google Scholar ] [ CrossRef ]
- Shi, J.; Wang, Z. A Hybrid Forecast Model for Household Electric Power by Fusing Landmark-Based Spectral Clustering and Deep Learning. Sustainability 2022 , 14 , 9255. [ Google Scholar ] [ CrossRef ]
- Mahjoub, S.; Chrifi-Alaoui, L.; Marhic, B.; Delahoche, L. Predicting Energy Consumption Using LSTM, Multi-Layer GRU and Drop-GRU Neural Networks. Sensors 2022 , 22 , 4062. [ Google Scholar ] [ CrossRef ] [ PubMed ]
- López, M.; Valero, S.; Sans, C.; Senabre, C. Use of Available Daylight to Improve Short-Term Load Forecasting Accuracy. Energies 2021 , 14 , 95. [ Google Scholar ] [ CrossRef ]
- Xu, A.; Tian, M.-W.; Firouzi, B.; Alattas, K.A.; Mohammadzadeh, A.; Ghaderpour, E. A New Deep Learning Restricted Boltzmann Machine for Energy Consumption Forecasting. Sustainability 2022 , 14 , 10081. [ Google Scholar ] [ CrossRef ]
- Akhtar, S.; Sujod, M.Z.B.; Rizvi, S.S.H. An Intelligent Data-Driven Approach for Electrical Energy Load Management Using Machine Learning Algorithms. Energies 2022 , 15 , 5742. [ Google Scholar ] [ CrossRef ]
- Zhou, F.; Zhou, H.; Li, Z.; Zhao, K. Multi-Step Ahead Short-Term Electricity Load Forecasting Using VMD-TCN and Error Correction Strategy. Energies 2022 , 15 , 5375. [ Google Scholar ] [ CrossRef ]
- Pannakkong, W.; Harncharnchai, T.; Buddhakulsomsiri, J. Forecasting Daily Electricity Consumption in Thailand Using Regression, Artificial Neural Network, Support Vector Machine, and Hybrid Models. Energies 2022 , 15 , 3105. [ Google Scholar ] [ CrossRef ]
- Tian, M.-W.; Alattas, K.; El-Sousy, F.; Alanazi, A.; Mohammadzadeh, A.; Tavoosi, J.; Mobayen, S.; Skruch, P. A New Short Term Electrical Load Forecasting by Type-2 Fuzzy Neural Networks. Energies 2022 , 15 , 3034. [ Google Scholar ] [ CrossRef ]
- Zhao, Z.; Xia, C.; Chi, L.; Chang, X.; Li, W.; Yang, T.; Zomaya, A.Y. Short-Term Load Forecasting Based on the Transformer Model. Information 2021 , 12 , 516. [ Google Scholar ] [ CrossRef ]
- de Mattos Neto, P.S.G.; de Oliveira, J.F.L.; Bassetto, P.; Siqueira, H.V.; Barbosa, L.; Alves, E.P.; Marinho, M.H.N.; Rissi, G.F.; Li, F. Energy Consumption Forecasting for Smart Meters Using Extreme Learning Machine Ensemble. Sensors 2021 , 21 , 8096. [ Google Scholar ] [ CrossRef ]
- Hou, T.; Fang, R.; Tang, J.; Ge, G.; Yang, D.; Liu, J.; Zhang, W. A Novel Short-Term Residential Electric Load Forecasting Method Based on Adaptive Load Aggregation and Deep Learning Algorithms. Energies 2021 , 14 , 7820. [ Google Scholar ] [ CrossRef ]
- Arvanitidis, A.I.; Bargiotas, D.; Daskalopulu, A.; Laitsos, V.M.; Tsoukalas, L.H. Enhanced Short-Term Load Forecasting Using Artificial Neural Networks. Energies 2021 , 14 , 7788. [ Google Scholar ] [ CrossRef ]
- Aslam, S.; Ayub, N.; Farooq, U.; Alvi, M.J.; Albogamy, F.R.; Rukh, G.; Haider, S.I.; Azar, A.T.; Bukhsh, R. Towards Electric Price and Load Forecasting Using CNN-Based Ensembler in Smart Grid. Sustainability 2021 , 13 , 12653. [ Google Scholar ] [ CrossRef ]
- Bot, K.; Santos, S.; Laouali, I.; Ruano, A.; Ruano, M.d.G. Design of Ensemble Forecasting Models for Home Energy Management Systems. Energies 2021 , 14 , 7664. [ Google Scholar ] [ CrossRef ]
- Arahal, M.R.; Ortega, M.G.; Satué, M.G. Chiller Load Forecasting Using Hyper-Gaussian Nets. Energies 2021 , 14 , 3479. [ Google Scholar ] [ CrossRef ]
- Khan, A.-N.; Iqbal, N.; Rizwan, A.; Ahmad, R.; Kim, D.-H. An Ensemble Energy Consumption Forecasting Model Based on Spatial-Temporal Clustering Analysis in Residential Buildings. Energies 2021 , 14 , 3020. [ Google Scholar ] [ CrossRef ]
- Wang, Y.; Zhang, N.; Chen, X. A Short-Term Residential Load Forecasting Model Based on LSTM Recurrent Neural Network Considering Weather Features. Energies 2021 , 14 , 2737. [ Google Scholar ] [ CrossRef ]
- Dorado Rueda, F.; Durán Suárez, J.; del Real Torres, A. Short-Term Load Forecasting Using Encoder-Decoder WaveNet: Application to the French Grid. Energies 2021 , 14 , 2524. [ Google Scholar ] [ CrossRef ]
- Khan, S.; Aslam, S.; Mustafa, I.; Aslam, S. Short-Term Electricity Price Forecasting by Employing Ensemble Empirical Mode Decomposition and Extreme Learning Machine. Forecasting 2021 , 3 , 460–477. [ Google Scholar ] [ CrossRef ]
- Ramos, D.; Khorram, M.; Faria, P.; Vale, Z. Load Forecasting in an Office Building with Different Data Structure and Learning Parameters. Forecasting 2021 , 3 , 242–255. [ Google Scholar ] [ CrossRef ]
- Oprea, S.-V.; Pîrjan, A.; Căruțașu, G.; Petroșanu, D.-M.; Bâra, A.; Stănică, J.-L.; Coculescu, C. Developing a Mixed Neural Network Approach to Forecast the Residential Electricity Consumption Based on Sensor Recorded Data. Sensors 2018 , 18 , 1443. [ Google Scholar ] [ CrossRef ] [ Green Version ]
- Divina, F.; Gilson, A.; Goméz-Vela, F.; García Torres, M.; Torres, J.F. Stacking Ensemble Learning for Short-Term Electricity Consumption Forecasting. Energies 2018 , 11 , 949. [ Google Scholar ] [ CrossRef ] [ Green Version ]
- Li, C.; Ding, Z.; Yi, J.; Lv, Y.; Zhang, G. Deep Belief Network Based Hybrid Model for Building Energy Consumption Prediction. Energies 2018 , 11 , 242. [ Google Scholar ] [ CrossRef ] [ Green Version ]
- Massidda, L.; Marrocu, M. Decoupling Weather Influence from User Habits for an Optimal Electric Load Forecast System. Energies 2017 , 10 , 2171. [ Google Scholar ] [ CrossRef ] [ Green Version ]
- Bornschlegl, M.; Bregulla, M.; Franke, J. Methods-Energy Measurement–An approach for sustainable energy planning of manufacturing technologies. J. Clean. Prod. 2016 , 1351 , 644–656. [ Google Scholar ] [ CrossRef ]
- Spiliotis, E.; Petropoulos, F.; Kourentzes, N.; Assimakopoulos, V. Cross-temporal aggregation: Improving the forecast accuracy of hierarchical electricity consumption. Appl. Energy 2020 , 2611 , 114339. [ Google Scholar ] [ CrossRef ]
- Yuce, B.; Mourshed, M.; Rezgui, Y. A Smart Forecasting Approach to District Energy Management. Energies 2017 , 10 , 1073. [ Google Scholar ] [ CrossRef ] [ Green Version ]
- Gnatyuk, V.I. The Law of Optimal Building Technocenoses , 3rd ed.; KIC “Technocenosis”: Kaliningrad, Russia, 2019; p. 940. [ Google Scholar ]
- Morgoeva, A.D.; Morgoev, I.D.; Klyuev, R.V.; Gavrina, O.A. Forecasting of electric energy consumption by an industrial enterprise using machine learning methods. Bull. Tomsk. Polytech. Univ. Geo Assets Eng. 2022 , 333 , 115–125. [ Google Scholar ] [ CrossRef ]
- Morgoeva, A.D.; Morgoev, I.D.; Klyuev, R.V. Prediction of electrical load by autoregression and integrated moving average. Grozny Nat. Sci. Bull. 2022 , 2 , 111–117. [ Google Scholar ] [ CrossRef ]
- Morgoev, I.D.; Dzgoev, A.E.; Klyuev, R.V.; Morgoeva, A.D. Forecasting the consumption of electricity by enterprises of the national economy complex in conditions of incomplete information. News Kabard.-Balkar. Sci. Cent. RAS 2022 , 3 , 9–20. [ Google Scholar ] [ CrossRef ]
- Klyuev, R.V.; Gavrina, O.A.; Khetagurov, V.N.; Zaseev, S.G.; Umirov, B.Z. Prediction of specific electric energy consumption at processing plant. Min. Inf. Anal. Bull. (Sci. Tech. J.) 2020 , 11 , 135–145. [ Google Scholar ] [ CrossRef ]
- Albuquerque, P.C.; Cajueiro, D.O.; Rossi, M.D.C. Machine learning models for forecasting power electricity consumption using a high dimensional dataset. Expert Syst. Appl. 2022 , 187 , 115917. [ Google Scholar ] [ CrossRef ]
- Naji, A.; Al Tarhuni, B.; Choi, J.K.; Alshatshati, S.; Ajena, S. Ajena Toward cost-effective residential energy reduction andcommunity impacts: A data-based machine learning approach. Energy AI 2021 , 4 , 100068. [ Google Scholar ] [ CrossRef ]
- Ji, Q.; Zhang, S.; Duan, Q.; Gong, Y.; Li, Y.; Xie, X.; Bai, J.; Huang, C.; Zhao, X. Short- and Medium-Term Power Demand Forecasting with Multiple Factors Based on Multi-Model Fusion. Mathematics 2022 , 10 , 2148. [ Google Scholar ] [ CrossRef ]
- Khan, P.W.; Byun, Y.-C.; Lee, S.-J.; Kang, D.-H.; Kang, J.-Y.; Park, H.-S. Machine Learning-Based Approach to Predict Energy Consumption of Renewable and Nonrenewable Power Sources. Energies 2020 , 13 , 4870. [ Google Scholar ] [ CrossRef ]
- Liu, D.; Sun, K.; Huang, H.; Tang, P. Monthly Load Forecasting Based on Economic Data by Decomposition Integration Theory. Sustainability 2018 , 10 , 3282. [ Google Scholar ] [ CrossRef ]
- Senchilo, N.D.; Ustinov, D.A. Method for Determining the Optimal Capacity of Energy Storage Systems with a Long-Term Forecast of Power Consumption. Energies 2021 , 14 , 7098. [ Google Scholar ] [ CrossRef ]
- Giola, C.; Danti, P.; Magnani, S. Learning Curves: A Novel Approach for Robustness Improvement of Load Forecasting. Eng. Proc. 2021 , 5 , 38. [ Google Scholar ] [ CrossRef ]
- Yousaf, A.; Asif, R.M.; Shakir, M.; Rehman, A.U.; Adrees, M.S. An Improved Residential Electricity Load Forecasting Using a Machine-Learning-Based Feature Selection Approach and a Proposed Integration Strategy. Sustainability 2021 , 13 , 6199. [ Google Scholar ] [ CrossRef ]
- Oliveira, E.M.d.; Oliveira, F.; Cyrino, F. Forecasting mid-long term electric energy consumption through bagging ARIMA and exponential smoothing methods. Energy 2018 , 1441 , 776–788. [ Google Scholar ] [ CrossRef ]
- Mochalin, D.S.; Titov, V.G. Assessment and forecasting of power consumption of the device of air cooling of gas at compressor station. Bull. Chuvash Univ. 2014 , 2 , 41–46. [ Google Scholar ]
- Gnatyuk, V.I.; Kivchun, O.R.; Morozov, D.G. Electric consumption predictions objects of socio-economic systems based on the ranked values. Mar. Intellect. Technol. 2020 , 4 , 107–111. [ Google Scholar ] [ CrossRef ]
- Xie, Y.; Yang, Y.; Wu, L. Power Consumption Forecast of Three Major Industries in China Based on Fractional Grey Model. Axioms 2022 , 11 , 407. [ Google Scholar ] [ CrossRef ]
- Shirzadi, N.; Nizami, A.; Khazen, M.; Nik-Bakht, M. Medium-Term Regional Electricity Load Forecasting through Machine Learning and Deep Learning. Designs 2021 , 5 , 27. [ Google Scholar ] [ CrossRef ]
- Li, H.; Guo, S.; Zhao, H.; Su, C.; Wang, B. Annual Electric Load Forecasting by a Least Squares Support Vector Machine with a Fruit Fly Optimization Algorithm. Energies 2012 , 5 , 4430–4445. [ Google Scholar ] [ CrossRef ]
- Hasanov, F.J.; Hunt, L.C.; Mikayilov, C.I. Modeling and Forecasting Electricity Demand in Azerbaijan Using Cointegration Techniques. Energies 2016 , 9 , 1045. [ Google Scholar ] [ CrossRef ] [ Green Version ]
- Gitelman, L.D.; Dobrodey, V.V.; Kozhevnikov, M.V. Tools for sustainable development of regional energy systems. Econ. Reg. 2020 , 16 , 1208–1223. [ Google Scholar ] [ CrossRef ]
- Mokhov, V.G.; Demyanenko, T.S. Construction of trend component of additive long-term forecasting model of electricity consumption volume of the wholesale electric energy and power market of Russia, by the example of united power system of the Ural. Bull. South Ural. State Univ. Ser. Econ. Manag. 2018 , 12 , 80–87. (In Russian) [ Google Scholar ] [ CrossRef ] [ Green Version ]
- Hamed, M.M.; Ali, H.; Abdelal, Q. Forecasting annual electric power consumption using a random parameters model with heterogeneity in means and variances. Energy 2022 , 255 , 124510. [ Google Scholar ] [ CrossRef ]
- Zhou, C.; Chen, X. Predicting China’s energy consumption: Combining machine learning with three-layer decomposition approach. Energy Rep. 2021 , 7 , 5086–5099. [ Google Scholar ] [ CrossRef ]
- Ahmadi, M.; Soofiabadi, M.; Nikpour, M.; Naderi, H.; Abdullah, L.; Arandian, B. Developing a Deep Neural Network with Fuzzy Wavelets and Integrating an Inline PSO to Predict Energy Consumption Patterns in Urban Buildings. Mathematics 2022 , 10 , 1270. [ Google Scholar ] [ CrossRef ]
- Kanté, M.; Li, Y.; Deng, S. Scenarios Analysis on Electric Power Planning Based on Multi-Scale Forecast: A Case Study of Taoussa, Mali from 2020 to 2035. Energies 2021 , 14 , 8515. [ Google Scholar ] [ CrossRef ]
- Cui, X.; E, S.; Niu, D.; Wang, D.; Li, M. An Improved Forecasting Method and Application of China’s Energy Consumption under the Carbon Peak Target. Sustainability 2021 , 13 , 8670. [ Google Scholar ] [ CrossRef ]
- Khan, A.M.; Osińska, M. How to Predict Energy Consumption in BRICS Countries? Energies 2021 , 14 , 2749. [ Google Scholar ] [ CrossRef ]
- Rehman, S.A.U.; Cai, Y.; Fazal, R.; Das Walasai, G.; Mirjat, N.H. An Integrated Modeling Approach for Forecasting Long-Term Energy Demand in Pakistan. Energies 2017 , 10 , 1868. [ Google Scholar ] [ CrossRef ] [ Green Version ]
- Karabulut, K.; Alkan, A.; Yilmaz, A.S. Long Term Energy Consumption Forecasting Using Genetic Programming. Math. Comput. Appl. 2008 , 13 , 71–80. [ Google Scholar ] [ CrossRef ] [ Green Version ]
- Zhukovskiy, Y.; Tsvetkov, P.; Buldysko, A.; Malkova, Y.; Stoianova, A.; Koshenkova, A. Scenario Modeling of Sustainable Development of Energy Supply in the Arctic. Resources 2021 , 10 , 124. [ Google Scholar ] [ CrossRef ]
- Kaboli, S.; Selvaraj, J.; Rahim, N. Long-term electric energy consumption forecasting via artificial cooperative search algorithm. Energy 2016 , 115 , 857–871. [ Google Scholar ] [ CrossRef ]
- Carvallo, J.; Larsen, P.; Sanstad, A.; Goldman, C. Long term load forecasting accuracy in electric utility integrated resource planning. Energy Policy 2018 , 119 , 410–422. [ Google Scholar ] [ CrossRef ]
Click here to enlarge figure
Method | Scope of Application | Forecasting Error | Identified Problems | Advantages of the Method | The Possibility of Using the Model to Solve Short-Term Forecasting Problems (i.e., How it Will Behave for Long Intervals) | Main Publications | Initial Dataset | Performance (Forecast Execution Time, s) | |
---|---|---|---|---|---|---|---|---|---|
% | Estimation (+/−) | ||||||||
Perceptron | Forecasting of power consumption in regional control rooms, the building of justice in the USA | 1.25 (standard error per day) 3.9 | + | Overfitting; problems of obtaining detailed data; complexity of interpretation of results. | The random component is taken into account due to the presence of an adaptation contour, with the help of which deviations in the model are taken into account. | The model is applicable to the problem of short-term forecasting provided that previous temperature observations are taken into account. | [ , ] | 1. The actual load of the system at time t. 2. Previous load observations (t-n). 5760 fifteen-second data values of all input data for each type of day during a 90-day data period: heating, ventilation and air conditioning kW, type of day (weekend/working), time of day, external temperature, humidity. | No information available |
LSTM network using the “Butterfly” optimization algorithm | Private households | 9.5 (tested on two datasets) | + | The complexity of implementation, the need to take into account a large number of factors. | Productive (in terms of forecast execution time) compared to other models (ensemble-based deep-learning model, CNN with GRU model, multilayer bidirectional GRU with CNN) due to the use of the algorithm for selecting optimal hyperparameters of the Butterfly network. Tested on publicly available datasets. LSTM networks have a memory mechanism and effectively cope with the problem of gradient attenuation. | More research is needed | [ ] | Detailed data on power consumption: for the first data set, 29 parameters related to the energy consumption of appliances, lighting and weather information (pressure, temperature, dew point, humidity, wind speed, etc.); for the second data set, the original data set contains nine attributes, such as voltage, minutes, global intensity, month, global active power, year, global reactive power, day, and hour. Three more variables are obtained from sensors. | 12 and 13 for two datasets |
Quantile Regression Fast Forest | Mining industry: Ben Guerir Quarry, Morocco | 1% | + | Additional research of the model is needed for the possibility of real-time application. | Good performance of the method | The model is applicable for operational and short-term forecasting. | [ ] | The model is designed to function in SCADA systems as an intelligent module. It is possible to connect alternative energy sources. | 10 |
Autoregressions of the integrated moving average ARIMA | The building of justice in the USA | 6.9 | + | The need to re-evaluate the model when adding new data to the model, the low generalizing ability of the algorithm, the complexity of parameter selection. | A well-developed mathematical apparatus, a formalized method of checking the model for adequacy to experimental data | More research is needed. High probability of applicability of the method for the problem of short-term forecasting | [ ] | 5760 15-second data values of all input data for each type of day during a 90-day data period: heating, ventilation and air conditioning kW, type of day (output/working), time of day, external temperature, humidity. | No information available |
Linear regression | The building of justice in the USA | 15.2 | − | Inefficient in the case of complex dependencies in the source data, unstable to outliers and omissions in the data | Ease of implementation and interpretation of results | More research is needed | [ ] | No information available |
Method | Scope of Application | Forecasting Error | Identified Problems | Advantages of the Method | The Possibility of Using the Model to Solve Problems of Medium-Term Forecasting (i.e., How It Will Behave for Long Intervals) | Main Publications | Initial Dataset | |
---|---|---|---|---|---|---|---|---|
% | Estimation (+/−) | |||||||
Perceptron | Regional Dispatch Management | Weekly average 1.82 1.46 | + | The need to retrain the network to account for the seasonality of data. Retraining the network. | The possibility of obtaining a reliable forecast in conditions of incompleteness of the initial data, a small complexity of the method. | More research is needed. | [ , ] | Hourly load values for the days preceding the predicted ones (24 values) and for the days a week ago (24 values). Initial network training: load data from two weeks ago. Accounting for the type of day (working, weekend, or holiday). |
Fuzzy neural network | Regional Dispatch Management | Mean for a week 1.18 1.34 | + | The complexity of the method, the need for an expert decision when choosing the necessary rules. The need for experimental selection of parameters. | The ability to add new rules. | It can be used for medium-term forecasting (for a month in advance) with a decrease in the accuracy of forecasting and, consequently, the need to re-evaluate the parameters of the model. | [ , ] | Hourly load values for the days preceding the predicted ones (24 values) and for the days a week ago (24 values). Initial network training: load data from two weeks ago. Accounting for the type of day (working, weekend, or holiday). Consideration of meteorological factors. |
Hybrid model: multidimensional singular spectral analysis and fuzzy neural network | Regional Dispatch Management | 1.3 | + | The greater complexity of the method compared to the isolated use of a fuzzy neural network | The possibility of decomposing a number of power consumption into additive components: trend, seasonal (harmonic), and noise, which contributes to a better selection of signs supplied to the input of the neural network. | It can be used for medium-term forecasting (a month in advance) with a decrease in the accuracy of forecasting and, consequently, the need to re-evaluate the parameters of the model. | [ ] | Daily schedules of electrical loads, meteorological factors (temperature, natural light) |
One-dimensional Convolutional Neural Network (CNN) | Sewage treatment plants | <5% (630 kWh standard error of the forecast) | + | The accuracy of the forecast can be higher if one adds data on the level of water pollution. However, there is a delay in obtaining the initial data: data on water level pollution is obtained through laboratory means, so the forecast of electricity consumption with these factors can be obtained only after a day. | Shorter forecast execution time and better generalizing ability of the method compared to the perceptron. | Additional research is needed. | [ ] | Daily data on energy consumption. Data characterizing the volume of water flow at the entrance to treatment facilities and meteorological factors (25 factors, including temperature, humidity, wind speed, etc.). Open data of the wholesale electricity market and the total planned consumption capacity for the second price zone (zone Siberia). |
Holt–Winters exponential smoothing model using a moving average to identify trends when smoothing graphs | Enterprises of the mineral resource complex located on the territory of Siberia | 1.08 | + | The complexity of the method. The complexity of the selection of model parameters. | Taking into account the trend and seasonality contributes to obtaining an accurate forecast. The model gives more accurate prediction results than the neural network model. | [ , ] | ||
Recurrent neural network LSTM | Power systems of large federal districts of the Russian Federation | 2.1 | + | The complexity of the selection of model parameters. The difficulty of interpreting the forecast | Fast execution of the forecast. The presence of a memorization mechanism. Taking into account the nonlinearity of the source data. Lower susceptibility to outliers compared to classical methods (moving average, linear regression). | [ ] | Data on the power consumption of federal districts for 13 years, meteorological data, information about the day of the week according to the production calendar, and features of industrial production in the relevant federal district (statistical information). Data for 3 days preceding the forecast period are sufficient for forecasting. | |
Linear regression | Power systems of large federal districts of the Russian Federation | 3.3 | + | Susceptibility to seasonal fluctuations. The need to retrain the model when using new data. | Easy to implement | [ ] |
Method | Scope of Application | Forecasting Error | Identified Problems | Advantages of the Method | The Possibility of Using the Model to Solve Short-Term/Long-Term Forecasting Problems (i.e., How It Will Behave for Sort Intervals) | Main Publications | Initial Dataset | Performance (Forecast Execution Time, s) | |
---|---|---|---|---|---|---|---|---|---|
% | Estimation (+/−) | ||||||||
Perceptron | Large residential buildings in 6 districts of China | 13.29 (average for 6 buildings) | + | Network overfitting. The complexity of the method implementation. The complexity of obtaining the source data. | The principal component method and multiple regression analysis are used to analyze factors, which increases the accuracy of the model. The Levenberg–Marquardt algorithm was chosen as the neural network learning algorithm. There is an analysis of the application of the method by season: the best forecast in winter (error 4.51%), the worst in winter (8.82%). | The model is applicable to solving hourly forecasting problems. | [ , ] | Load on the power grid, social factors (employment): twelve input data: month, day, hour, minute, outdoor air temperature, outdoor air humidity, outdoor air pressure, wind speed, wind direction, visibility, number of people under the age of fifteen (for example, zero, one, two, three and etc.), and current power consumption | No information available |
CatBoost Gradient Boosting | A small industrial enterprise | 7.95% | + | The model does not take into account the data of the technological process. | The performance of the model. Automated selection of hyperparameters. Resistance to emissions. | The model can be used for short-term forecasting. Additional studies are needed to evaluate the application in the long term. | [ ] | Monthly power consumption for 5 years by divisions of the enterprise with a division into technical needs and lighting. Monthly average weather data (humidity, wind speed, temperature, dew point temperature). | 50 |
Autoregression of the integrated moving average ARIMA | A small industrial enterprise | 15.92 | − | Insufficient level of model accuracy due to the complexity of the selection of model parameters. | A well-developed mathematical apparatus. Ease of implementation. | More research is needed. | [ ] | Monthly power consumption for 5 years by divisions of the enterprise with a division into technical needs and lighting. Monthly average weather data (humidity, wind speed, temperature, dew point temperature). | 70 |
Method | Scope of Application | Forecasting Error | Identified Problems | Advantages of the Method | The Possibility of Using the Model to Solve Problems of Medium-Term Forecasting | Main Publications | Initial Dataset | Performance (Forecast Execution Time, s) | |
---|---|---|---|---|---|---|---|---|---|
% | Estimation (+/−) | ||||||||
A method based on the values of rank norms | Socioeconomic systems of the regions of the Russian Federation | 4.87 | + | The complexity of the mathematical apparatus. The need for competencies in the field of rank analysis of technocenoses. | Obtaining a reliable forecast without the need to take into account factors affecting power consumption. | The model can be applied for medium-term forecasting. | [ ] | Monthly data on regional electricity consumption Russia for eleven years from 2009 to 2019 | No information available |
Deep Neural Network with Fuzzy Wavelets | Urban Buildings | − | + | The complexity of the mathematical apparatus. The complexity of evaluating the results of forecasting. | A small number of rules and less complexity of the model compared to a fully connected neural network. | The model can be applied for medium-term forecasting. | [ ] | Electricity consumption in Tehran, Mashhad, Ahvaz, and Urmia from 2010 to 2021 | 50 |
Delphi | The Arctic Energy System | − | + | Subjectivity of expert opinions. The complexity of evaluating the results of forecasting. | There are three reasonable scenarios of development: positive, negative, and neutral. Taking into account many risks when developing scenarios. Analysis of consumers by energy consumption level. | More research is needed. | [ ] | Expert assessments | No information available |
Gray Model | China’s Industries | − | + | The complexity of evaluating the results of forecasting. | A small amount of the initial sample is necessary for forecasting. Consideration of socio-economic development scenarios. | Not applicable | [ ] | Data on the level of production, urbanization and socioeconomic development for 2011–2020 | No information available |
Nonlinear autoregressive exogenous neural network | Regional municipalities | 4.2 | + | The complexity of selecting hyperparameters of the model | Prediction accuracy. The possibility of using the method for other prediction intervals. The ability to scale the model taking into account new factors. | It is possible to build a forecast for the short and medium term. | [ ] | The dataset includes hourly load demand data for nine years for Bruce County, Ontario, Canada combined with climate information (temperature and wind speed) for 2010–2018 (forecast made for 2019). | 960 |
MDPI stays neutral with regard to jurisdictional claims in published maps and institutional affiliations. |
Share and Cite
Klyuev, R.V.; Morgoev, I.D.; Morgoeva, A.D.; Gavrina, O.A.; Martyushev, N.V.; Efremenkov, E.A.; Mengxu, Q. Methods of Forecasting Electric Energy Consumption: A Literature Review. Energies 2022 , 15 , 8919. https://doi.org/10.3390/en15238919
Klyuev RV, Morgoev ID, Morgoeva AD, Gavrina OA, Martyushev NV, Efremenkov EA, Mengxu Q. Methods of Forecasting Electric Energy Consumption: A Literature Review. Energies . 2022; 15(23):8919. https://doi.org/10.3390/en15238919
Klyuev, Roman V., Irbek D. Morgoev, Angelika D. Morgoeva, Oksana A. Gavrina, Nikita V. Martyushev, Egor A. Efremenkov, and Qi Mengxu. 2022. "Methods of Forecasting Electric Energy Consumption: A Literature Review" Energies 15, no. 23: 8919. https://doi.org/10.3390/en15238919
Article Metrics
Article access statistics, further information, mdpi initiatives, follow mdpi.
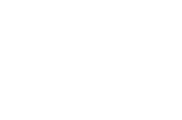
Subscribe to receive issue release notifications and newsletters from MDPI journals
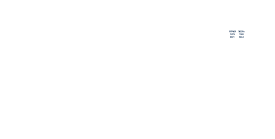
Energy Production and Consumption
Explore data on how energy production and use varies across the world..
The availability of energy has transformed the course of humanity over the last few centuries. Not only have new sources of energy been unlocked — first fossil fuels, followed by diversification to nuclear, hydropower, and now other renewable technologies — but also in the quantity we can produce and consume.
This article focuses on the quantity of energy we consume — looking at total energy and electricity consumption; how countries compare when we look at this per person; and how energy consumption is changing over time.
In our pages on the Energy Mix and Electricity Mix , we look in more detail at what sources provide this energy.
Global energy consumption
How much energy does the world consume.
The energy system has transformed dramatically since the Industrial Revolution. We see this transformation of the global energy supply in the interactive chart shown here. It graphs global energy consumption from 1800 onwards.
It is based on historical estimates of primary energy consumption from Vaclav Smil, combined with updated figures from BP's Statistical Review of World Energy . 1
Note that this data presents primary energy consumption via the “substitution method”. The substitution method — in comparison to the direct method — attempts to correct for the inefficiencies (energy wasted as heat during combustion) in fossil fuel and biomass conversion. It does this by correcting nuclear and modern renewable technologies to their “primary input equivalents” if the same quantity of energy was produced from fossil fuels.
How is global energy consumption changing year-to-year?
Demand for energy is growing across many countries in the world, as people get richer and populations increase.
If this increased demand is not offset by improvements in energy efficiency elsewhere, then our global energy consumption will continue to grow year-on-year. Growing energy consumption makes the challenge of transitioning our energy systems away from fossil fuels towards low-carbon sources of energy more difficult: new low-carbon energy has to meet this additional demand and try to displace existing fossil fuels in the energy mix.
This interactive chart shows how global energy consumption has been changing from year to year. The change is given as a percentage of consumption in the previous year.
We see that global energy consumption has increased nearly every year for more than half a century. The exceptions to this are in the early 1980s, and 2009 following the financial crisis.
Global energy consumption continues to grow, but it does seem to be slowing — averaging around 1% to 2% per year.
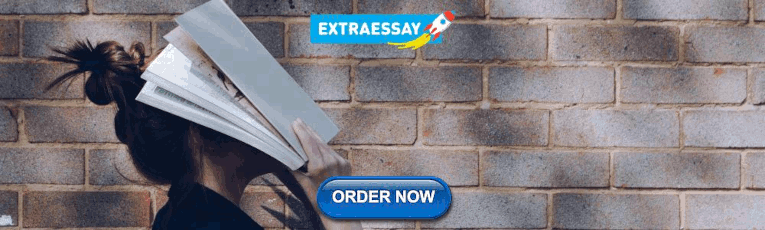
Primary energy consumption
Total energy consumption.
How much energy do countries across the world consume?
This interactive chart shows primary energy consumption country-by-country. It is the sum of total energy consumption, including electricity, transport, and heating. We look at electricity consumption individually later in this article.
Note, again, that this is based on primary energy via the substitution method: this means nuclear and renewable energy technologies have been converted into their “primary input equivalents” if they had the same levels of inefficiency as fossil fuel conversion.
Per capita: where do people consume the most energy ?
When we look at total energy consumption, differences across countries often reflect differences in population size: countries with lots of people inevitably consume more energy than tiny countries.
How do countries compare when we look at energy consumption per person ?
This interactive chart shows per capita energy consumption. We see vast differences across the world.
The largest energy consumers include Iceland, Norway, Canada, the United States, and wealthy nations in the Middle East such as Oman, Saudi Arabia, and Qatar. The average person in these countries consumes as much as 100 times more than those in some of the poorest countries.
In fact, the true differences between the richest and poorest might be even greater. We do not have high-quality data on energy consumption for many of the world's poorest countries. This is because they often use very few commercially traded energy sources (such as coal, oil, gas, or grid electricity) and instead rely on traditional biomass — crop residues, wood, and other organic matter that is difficult to quantify. This means we often lack good data on energy consumption for the world's poorest.
Where is energy consumption growing or falling?
Year-on-year change in primary energy consumption.
Globally, primary energy consumption has increased nearly every year for at least half a century. But this is not the case everywhere in the world.
Energy consumption is rising in many countries where incomes are rising quickly and the population is growing. But in many countries — particularly richer countries trying to improve energy efficiency — energy consumption is actually falling.
This interactive chart shows the annual growth rate of energy consumption. Positive values indicate a country's energy consumption was higher than the previous year. Negative values indicate its energy consumption was lower than the previous year.
Electricity generation
Total electricity generation: how much electricity does each country generate.
We previously looked at total energy consumption. This is the sum of energy used for electricity, transport, and heating.
Although the terms “electricity” and “energy” are often used interchangeably, it's important to understand that electricity is just one component of total energy consumption.
Let's take a look at electricity data. This interactive chart shows the amount of electricity generated by a country each year.
Per capita: which countries generate the most electricity ?
Just as with total energy, comparisons of levels of electricity generation often reflect population size. It tells us nothing about how much electricity the average person in a given country consumes relative to another.
This interactive chart shows per capita electricity generation per person. Again we see vast differences in electricity per person across the world. The largest producers — Iceland, Norway, Sweden, and Canada — generate hundreds of times as much electricity as the smallest.
In many of the poorest countries in the world, people consume very little electricity, which is estimated to be lower than 100 kilowatt-hours per person in some places.
Energy production and consumption by source
This page focuses on total energy and electricity consumption, without digging into the details of where this energy comes from, and how sources are changing over time.
In our pages on the Energy Mix and Electricity Mix , we look at full breakdowns of the energy system; how much of our energy comes from fossil fuels versus low-carbon sources; and whether we're making progress on decarbonization.
Vaclav Smil (2017). Energy Transitions: Global and National Perspectives .
Cite this work
Our articles and data visualizations rely on work from many different people and organizations. When citing this article, please also cite the underlying data sources. This article can be cited as:
BibTeX citation
Reuse this work freely
All visualizations, data, and code produced by Our World in Data are completely open access under the Creative Commons BY license . You have the permission to use, distribute, and reproduce these in any medium, provided the source and authors are credited.
The data produced by third parties and made available by Our World in Data is subject to the license terms from the original third-party authors. We will always indicate the original source of the data in our documentation, so you should always check the license of any such third-party data before use and redistribution.
All of our charts can be embedded in any site.
Our World in Data is free and accessible for everyone.
Help us do this work by making a donation.
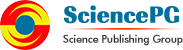
Journals By Subject
- Proceedings
Information

A Comprehensive Study of Electricity Consumption of People in Each Region in the Philippines
Renz Chester Rosales Gumaru
Math Department, Arellano University, Pasay, Philippines
Renren Banta
Math Department, Alabang High School, Alabang, Philippines
Add to Mendeley

Electricity is one of the most important source of energy in our everyday lives. We use it every day in different situations and circumstances. However, it is also the most used and most expensive energy that we always use. This study will determine which region in the Philippines consumed the most and least electricity. It will also study the relationship between the different distributor of electricity and electricity consumption. This study will help the society in solving the problem of dealing will high cost of electricity. Knowing which regions are the most and least consuming in terms of electricity will surely help the government identify why that particular regions consume a lot, or consume less. This study will also determine if there are changes every month in the most and least consuming electricity regions. The researchers also explore different and various problems and factors contributing to the consumption of electricity of each region in the Philippines. And the researchers also seek if there is a significant difference in the consumption of electricity between regions in the National Capital Region, and I n province. This study will surely help and contribute a lot to a developing country like the Philippines, especially the country is known for consumption of too much electricity.
Published in | ( ) |
DOI | |
Page(s) | 45-48 |
Creative Commons |
This is an Open Access article, distributed under the terms of the Creative Commons Attribution 4.0 International License ( ), which permits unrestricted use, distribution and reproduction in any medium or format, provided the original work is properly cited. |
Copyright | Copyright © The Author(s), 2019. Published by Science Publishing Group |
Electricity, Energy, Philippines, Source, Power
[1] | Yu Tui Chen, The Factors affecting the Electricity Consumption and the Consumption Characteristics in the Residential Sector, (2017). |
[2] | Björg Sigurjónsdóttir, Monitoring and Reducing the Consumption of Home Electric Appliances, (2013). |
[3] | Berk. Kevin, Modelling and Forecasting Electricity Demand, (2012). |
[4] | NTRC Tax Research Journal Volume XXIII, A Review of Electrical Energy Management Techniques and Consumer Side, (2011). |
[5] | Mohamed Tariq Khan, Philippines: household electricity consumption for capita, (2015). |
[6] | Eddie Seva See (2010), Electric Energy Utilization on the Household of Albay province, (2012). |
[7] | JORDEENE B. LAGARE, PH Power Consumption up nearly 4% Cost Last Year, (2018). |
[8] | https://psa.gov.ph/tags/energy-consumption), Energy Consumption in the Philippines, (2011). |
[9] | Jane Moraleda, Energy consumption in the Philippines, (2015). |
[10] | https://www.indexmundi.com/philippines/electricity_consumption.html, Philippines Electricity Consumption Energy, (2016). |
[11] | https://pids.gov.ph/press-releases/325, weather, gender, affect household enebry Consumption, (2013). |
[12] | Maureen Anne Araneta1, Cost- effective Programming of electric Demand in U. P Diliman (2017). |
[13] | Uy, Frances Irene, Energy Pricing in the Philippines and its effect in Economic Growth, (2016). |
[14] | Connie Bayudan-Dacuycuy Lawrence B. Dacuycuy, Urban and Rural Household’s energy use in the Philippines, (2018). |
[15] | Antonio A. Ver, Electricity most used Household Power Source, (2019). |
[16] | KRISTYN NIKA M. LAZO, Philippines: Energy sector Assessment, (2013). |
Renz Chester Rosales Gumaru, Renren Banta. (2019). A Comprehensive Study of Electricity Consumption of People in Each Region in the Philippines. American Journal of Mechanics and Applications , 7 (3), 45-48. https://doi.org/10.11648/j.ajma.20190703.12

Renz Chester Rosales Gumaru; Renren Banta. A Comprehensive Study of Electricity Consumption of People in Each Region in the Philippines. Am. J. Mech. Appl. 2019 , 7 (3), 45-48. doi: 10.11648/j.ajma.20190703.12
Renz Chester Rosales Gumaru, Renren Banta. A Comprehensive Study of Electricity Consumption of People in Each Region in the Philippines. Am J Mech Appl . 2019;7(3):45-48. doi: 10.11648/j.ajma.20190703.12
Cite This Article
- Author Information
Verification Code/
The captcha is required.
Captcha is not valid.

Verification Code
Science Publishing Group (SciencePG) is an Open Access publisher, with more than 300 online, peer-reviewed journals covering a wide range of academic disciplines.
Learn More About SciencePG
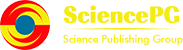
- Special Issues
- AcademicEvents
- ScholarProfiles
- For Authors
- For Reviewers
- For Editors
- For Conference Organizers
- For Librarians
- Article Processing Charges
- Special Issues Guidelines
- Editorial Process
- Peer Review at SciencePG
- Open Access
- Ethical Guidelines
Important Link
- Manuscript Submission
- Propose a Special Issue
- Join the Editorial Board
- Become a Reviewer
- Bibliography
- More Referencing guides Blog Automated transliteration Relevant bibliographies by topics
- Automated transliteration
- Relevant bibliographies by topics
- Referencing guides
MS Thesis Defense: Scott Maurer
"identifying savings in residential electricity consumption through data-driven analysis".
Optional ZOOM LINK
Abstract : Climate change and rising electricity demand have driven the need for energy efficiency in buildings. Single-family residential buildings present significant opportunities for improvement, but current strategies for identifying savings are labor and capital-intensive. Developing a residential version of virtual energy audit tools like EDIFES offers a cheaper, non-intrusive alternative.
This study used data from 25 all-electric homes in Florida to refine algorithms from EDIFES, originally designed for commercial buildings. The analysis utilized one year of 15-minute interval electricity consumption data, along with square footage, number of floors, and location. These markers include heating and cooling type, setpoint setback, HVAC load, pool pump detection, refrigeration load, and effective Rvalue. The heating and cooling type marker uses linear or segmented models based on daily consumption and outdoor temperatures. The type of heating and cooling systems were correctly identified in all but one building. A 1°C shift in setpoints resulted in an average 10% annual reduction in electricity consumption. The setpoint marker also estimated HVAC load with an 11% mean error, with 40 of 50 cases having errors under 20%. Pool pump markers, designed for constant-speed pumps, used kernel density to calculate their size and detected their presence with 100% accuracy. Validation with submeter data showed a 4.2% average error in load prediction. Reducing pump schedules to five hours per day led to a 21% reduction in pump load while replacing pumps with variable-speed models resulted in a 23% reduction in whole-home consumption. The refrigeration load marker identified cyclical loads, mainly from the compressor, with a 15% error. However, expected savings are minimal due to the small size of the refrigeration load. Effective R-value was calculated using consumption and temperature data. If R-values were below ASHRAE 90.2 standards, insulation improvements were simulated to quantify potential savings.
Surprisingly, few buildings had lower-than-desired R-values, indicating a need for further study to refine the assumed parameters. The largest and most cost-effective savings came from pool pump replacements and setpoint setbacks. Replacing pumps requires a modest investment but offers significant savings with a quick payback. Setpoint setbacks involve no capital cost and provide immediate savings.
Thesis Committee : Alexis Abramson (Chair), Roger French, Charles Sullivan, Vikrant Vaze
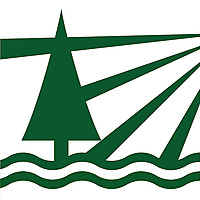
Climate tech startup Mantel to use molten salt to capture factory flue CO2 with $30 million funding from Shell, VCs
- Medium Text
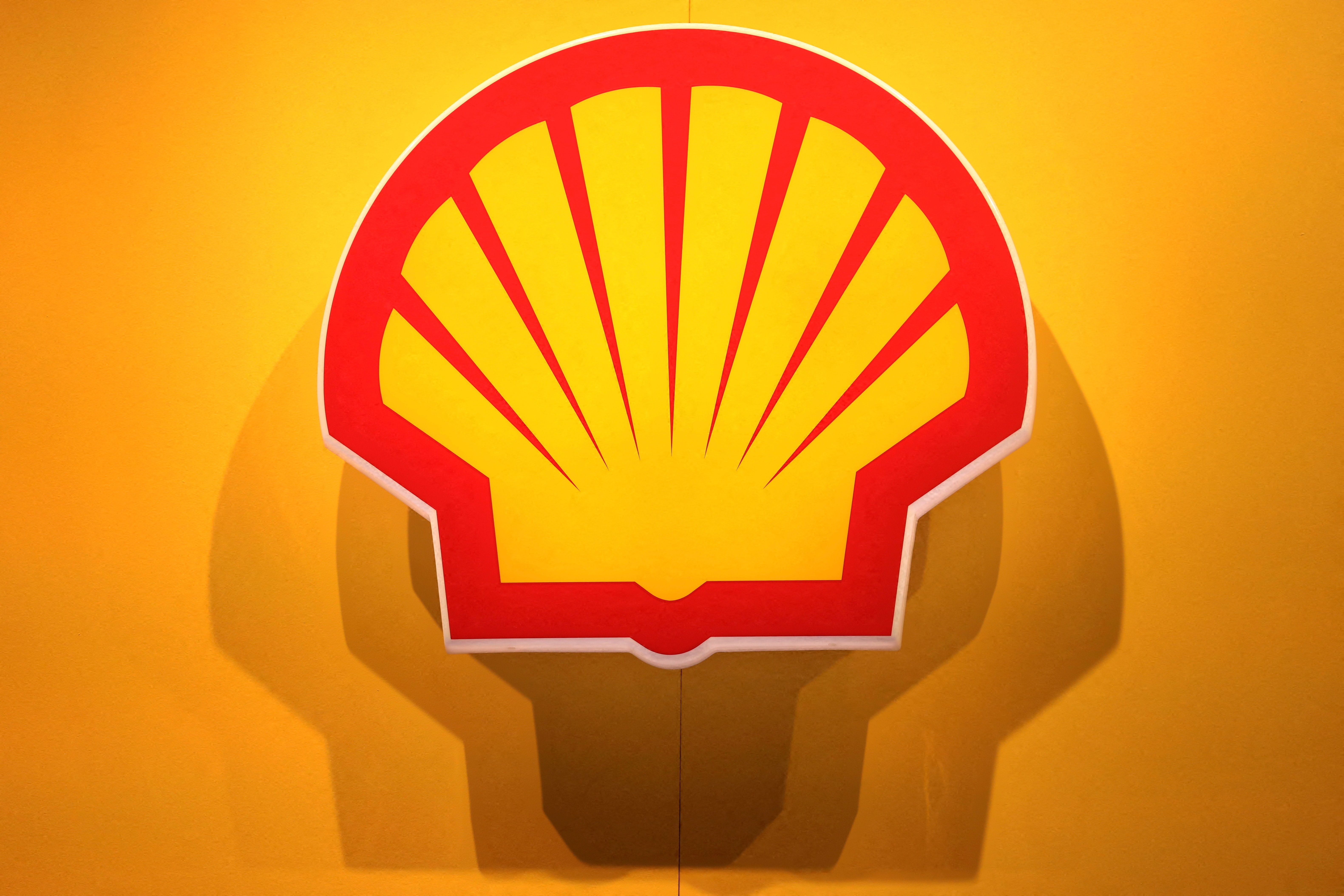
- Series A raise of $30 mln to fund demonstration project
- Technology can capture emissions inside kilns, boilers, furnaces
- Potential for 'step change' in lowering costs - Shell Ventures
- Technology has yet to be tested at scale
Sign up here.
Reporting by Peter Henderson in San Francisco and Simon Jessop in London; Editing by Jamie Freed
Our Standards: The Thomson Reuters Trust Principles. , opens new tab

Thomson Reuters
Simon leads a team tracking how the financial system and companies more broadly are responding to the challenges posed by climate change, nature loss and other environmental, social and governance (ESG) issues including diversity and inclusion.
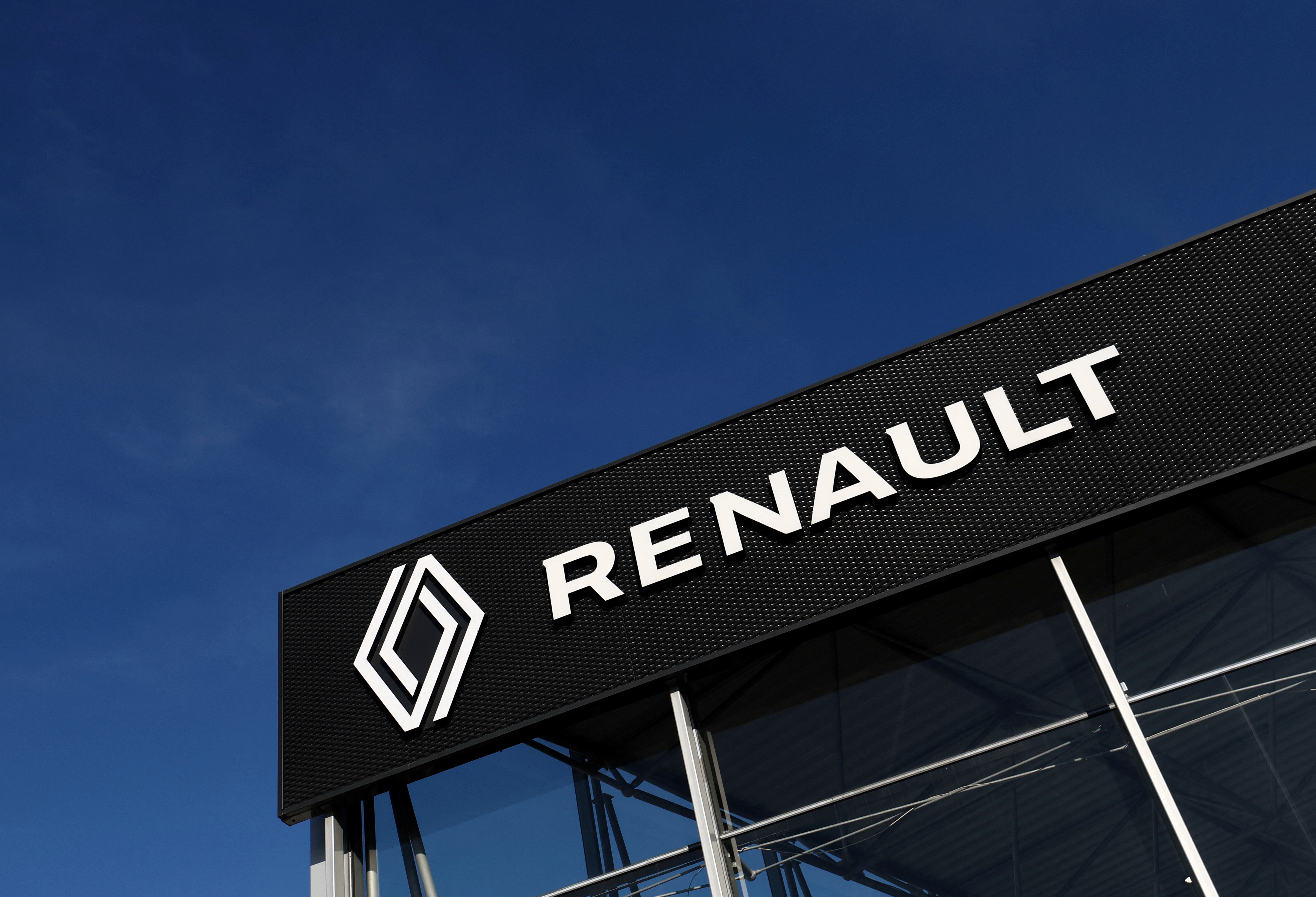
Sustainability
Super typhoon yagi makes landfall in vietnam after casualties in china.
Asia's most powerful storm this year made landfall in northern Vietnam on Saturday, the meteorological agency said, killing at least two people after tearing through China's island of Hainan, where it reportedly killed another two and injured dozens more.
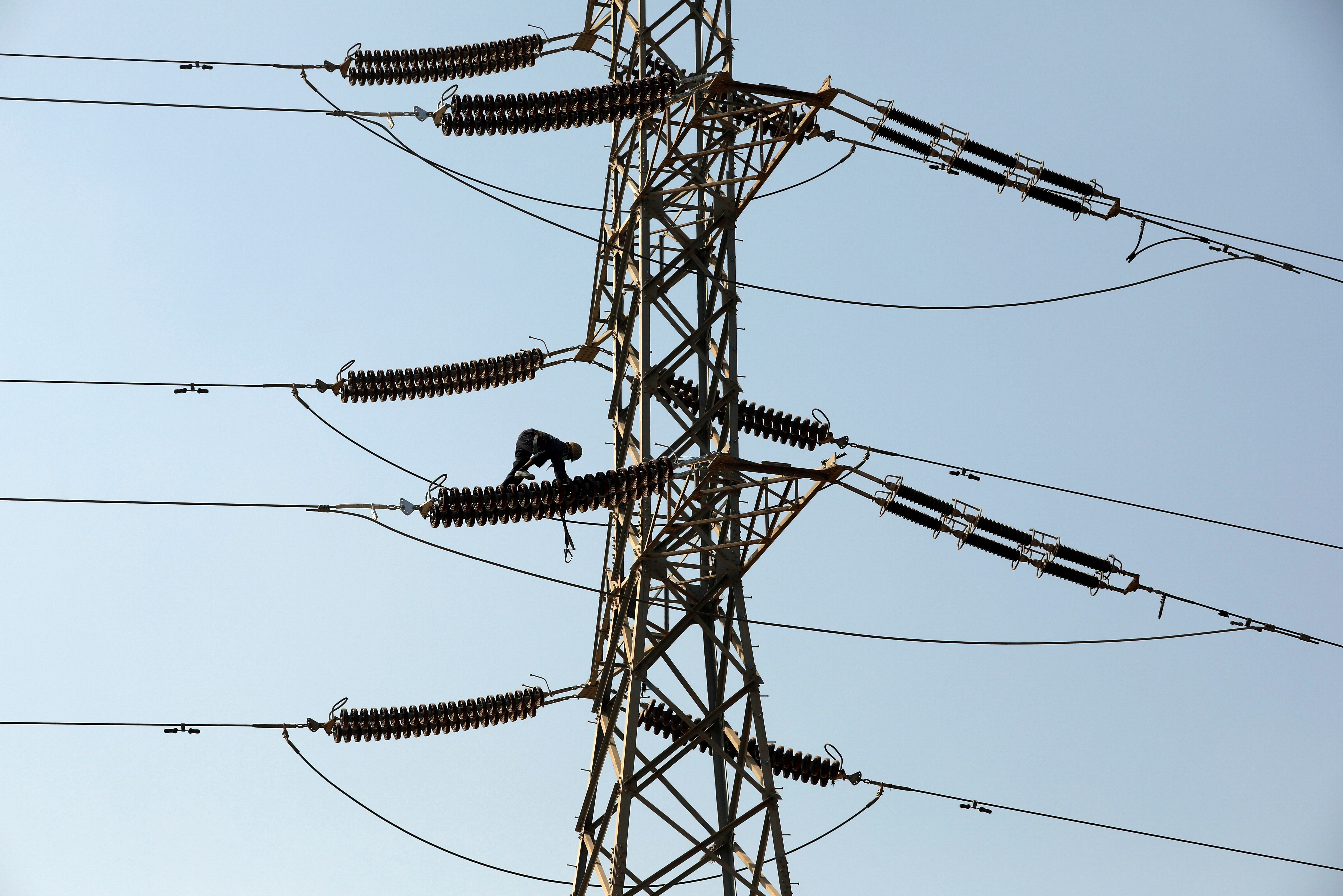
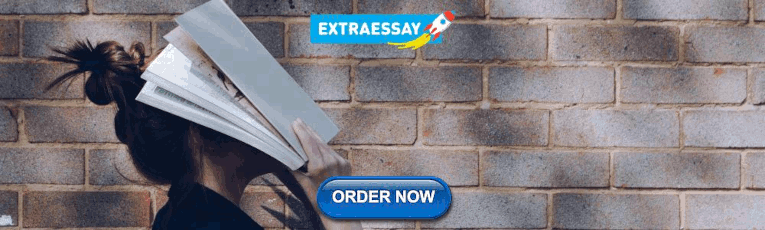
IMAGES
VIDEO
COMMENTS
The results show that electricity demand can be modeled using machine learning algorithms, deploying renewable energy, planning for high/low load days, and reducing wastage from polluting on reserve standby generation, detecting abnormalities in consumption trends, and quantifying energy and cost-saving measures.
1.1. Household energy consumption background. Environmental issues and social crises induce a shortage of energy all over the world. The coronavirus (COVID-19) pandemic from 2019 to 2022, in particular, resulted in a dramatic change in people's lives and activities around the world, including energy use behaviors.
On the average household spent $547.89and $661.05 on motor gasoline quarterly (or $2191.56 and $2644.2 annually) in 2006 and 2008 respectively, suggesting an increase of 20.65%. The average gasoline consumption per household was 218.99 and 214.44 gallon per quarter in 2006 and 2008 decreasing at the rate of 2.10%.
In PH, electricity consumption climbed to 94,370-gigawatt hours (GWh) in 2017, the Department of Energy (DoE) announced (Lagare 2018, in Rosales Gumaru and Banta 2019). Although statistics related ...
Data description on Google search was all content on 'Energy consumption and economic growth, Mendley general search made on 21st July, 2021 on energy consumption and economic growth gave results of 11,872 of which 8722 were from journals, 1374 from conference proceedings, 567 from book section, 557 from generic and 214 from thesis, while ...
generation etc. Out of these, buildings are the highest single contributor to world energy consumption and greenhouse gas emissions [2]. Therefore, building energy consumption in the industrial sector is a major contributor to global energy consumption. The international energy agency (IEA) has laid out a 2DS pathway for global climate change
Household electricity consumption behaviour: a meta-analysis and experimental approaches Penelope Buckley To cite this version: Penelope Buckley. Household electricity consumption behaviour: a meta-analysis and experimen-tal approaches. Economics and Finance. Université Grenoble Alpes, 2019. English. �NNT: 2019GREAE001�. �tel-02281733�
However, energy consumption and the resulting enhanced greenhouse gas emissions are thought to have led to a series of natural disasters. Since ... objective of this thesis is to identify the determinant factors of renewable and non-renewable energy consumption in 29 OECD countries. It also analyses and compares
Though as energy consumption per capita increases, the relationship stagnates at an HDI value of approximately 0.8 based on the principle of diminishing returns (ibid). It is noted that this study was conducted on total energy consumption per capita and does not delineate between fossil fuel energy consumption and renewable energy consumption.
Analysis of Correlations between Energy Consumption, Structural Specifications and Climate-Induced Variables to increase Energy Efficiency in Households and Buildings through a Prediction Model Christoffer Stene Rasmussen EOM-3901 Master's thesis in Energy, Climate and Environment June 2021
The regions in the province consume less electricity than those regions in commercial areas. 2. There is a significant difference between the electricity consumption between each region of the Philippines. 3. All regions use electricity but differs on the number of consuming the product due to the fact that they have different necessities.
This study revisits the economic growth-energy consumption thesis by investigating the impact of economic, social, and political globalization on the economic growth-energy consumption nexus in a panel of 23 emerging economies for the period 1970-2015. The results that emanate from an instrumental variable generalized method of moment ...
Energy transformation and sustainability is a global challenge, for many developing countries, facing energy-related issues like wide demand-supply consumption pattern by shifting or reducing ...
Balancing the production and consumption of electricity is an urgent task. Its implementation largely depends on the means and methods of planning electricity production. Forecasting is one of the planning tools since the availability of an accurate forecast is a mechanism for increasing the validity of management decisions. This study provides an overview of the methods used to predict ...
This interactive chart shows per capita energy consumption. We see vast differences across the world. The largest energy consumers include Iceland, Norway, Canada, the United States, and wealthy nations in the Middle East such as Oman, Saudi Arabia, and Qatar. The average person in these countries consumes as much as 100 times more than those ...
Electricity consumption in Malaysia over 10 years period (Suruhanjaya Tenaga Malaysia, 2019) ... Books, journal, thesis, online literature or online source. Gaining more knowledge of information based on the scope of research can be applied to help the analysis of the research more effective and get view from several angles.
The aim of this thesis is to briefly introduce the reader to the problem of development of the electricity consumption, to show the possibilities of its prediction, and provide an example of electricity consumption analysis, which may serve as a basis for long-term forecast.
Electricity is one of the most important source of energy in our everyday lives. We use it every day in different situations and circumstances. However, it is also the most used and most expensive energy that we always use. This study will determine which region in the Philippines consumed the most and least electricity. It will also study the relationship between the different distributor of ...
Energy Consumption Theory. Babak Vosooghzadeh. October 28, 2021. Overview. Diverse energy resources have to be developed [1 -2] which are environmentally-safe, and energy consumption. has to ...
(a.i) it increases output by increasing consumption of all the inputs, i.e., intermediate goods (I), including electricity (ECI), as well as capital (K) and labor (L) in the production process.This is the case when industry follows the production input growth path (see Jorgenson et al., 2014, Saari, 2006 and Garrigosa and Grifell Tatjé (1992) for further discussion).
Hence, the autonomous energy consumption scheduling of residential loads and electric vehicles (EVs) is necessary for the users to benefit from the DSM programs. In this thesis, we propose different algorithms to schedule the energy consumption of residential loads and EVs, and provide ancillary services to the electrical grid.
This paper focuses on the interactions of economic growth with both petroleum consumption and electricity consumption. 2.0. Energy consumption and the Nigerian economy. Images of energy-powered industrial revolutions around the globe emphasize the role of energy on economic growth.
This work is focused on the energy consumption of the server, which belongs to the data center's IT infrastructure. Understanding how the energy is consumed among the components of the server is essential to define an energy prediction model. This thesis presents a system-level power model of the server, which includes the power consumption
The heating and cooling type marker uses linear or segmented models based on daily consumption and outdoor temperatures. The type of heating and cooling systems were correctly identified in all but one building. A 1°C shift in setpoints resulted in an average 10% annual reduction in electricity consumption.
Boston — Massachusetts and Rhode Island announced today the selection of 2,878 megawatts (MW) of offshore wind in the first coordinated procurement of its kind. As a part of the procurement, Massachusetts selected 2,678 MW in total from three projects. This selection is New England's and Massachusetts' largest offshore wind selection to date, representing nearly 20 percent of Massachusetts ...
Item 1 of 2 The logo of British multinational oil and gas company Shell is displayed during the LNG 2023 energy trade show in Vancouver, British Columbia, Canada, July 12, 2023.