Information
- Author Services
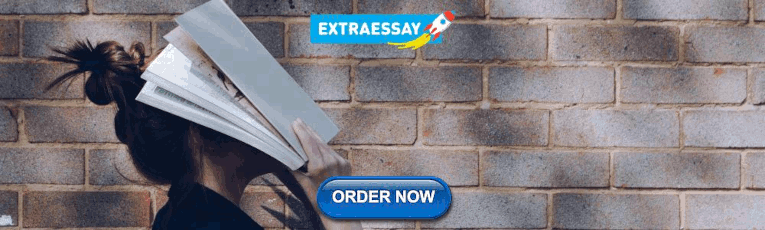
Initiatives
You are accessing a machine-readable page. In order to be human-readable, please install an RSS reader.
All articles published by MDPI are made immediately available worldwide under an open access license. No special permission is required to reuse all or part of the article published by MDPI, including figures and tables. For articles published under an open access Creative Common CC BY license, any part of the article may be reused without permission provided that the original article is clearly cited. For more information, please refer to https://www.mdpi.com/openaccess .
Feature papers represent the most advanced research with significant potential for high impact in the field. A Feature Paper should be a substantial original Article that involves several techniques or approaches, provides an outlook for future research directions and describes possible research applications.
Feature papers are submitted upon individual invitation or recommendation by the scientific editors and must receive positive feedback from the reviewers.
Editor’s Choice articles are based on recommendations by the scientific editors of MDPI journals from around the world. Editors select a small number of articles recently published in the journal that they believe will be particularly interesting to readers, or important in the respective research area. The aim is to provide a snapshot of some of the most exciting work published in the various research areas of the journal.
Original Submission Date Received: .
- Active Journals
- Find a Journal
- Proceedings Series
- For Authors
- For Reviewers
- For Editors
- For Librarians
- For Publishers
- For Societies
- For Conference Organizers
- Open Access Policy
- Institutional Open Access Program
- Special Issues Guidelines
- Editorial Process
- Research and Publication Ethics
- Article Processing Charges
- Testimonials
- Preprints.org
- SciProfiles
- Encyclopedia
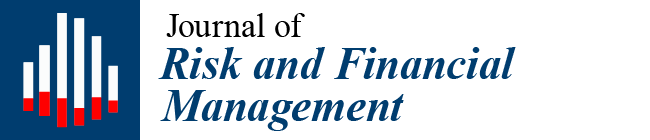
Article Menu
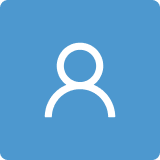
- Subscribe SciFeed
- Recommended Articles
- Google Scholar
- on Google Scholar
- Table of Contents
Find support for a specific problem in the support section of our website.
Please let us know what you think of our products and services.
Visit our dedicated information section to learn more about MDPI.
JSmol Viewer
How many stocks are sufficient for equity portfolio diversification a review of the literature.

1. Introduction
2. data and methods, 3. results of bibliometric analysis, 4. risk diversification thematic review, 4.1. measuring risk diversification, 4.2. number of stocks required for risk diversification, 4.3. optimal portfolio size across different capital markets, 4.4. impact of crises on risk diversification, 5. discussion, 6. conclusions, author contributions, institutional review board statement, informed consent statement, data availability statement, conflicts of interest.
- Aboura, Sofiane, and Julien Chevallier. 2017. A new weighting-scheme for equity indexes. International Review of Financial Analysis 54: 159–75. [ Google Scholar ] [ CrossRef ]
- Ahmed, Awais, Rizwan Ali, Abdullsh Ejaz, and Ishfaq Ahmad. 2018. Sectoral integration and investment diversification opportunities: Evidence from Colombo Stock Exchange. Entrepreneurship and Sustainability Issues 5: 514–27. [ Google Scholar ] [ CrossRef ] [ Green Version ]
- Ahuja, Aakash. 2015. Portfolio Diversification in the Karachi Stock Exchange. Pakistan Journal of Engineering, Technology & Science 1: 37–44. [ Google Scholar ] [ CrossRef ] [ Green Version ]
- Aigner, Philipp, Georg Beyschlag, Tim Friederich, Markus Kalepky, and Rudi Zagst. 2012. Modeling and managing portfolios including listed private equity. Computers & Operations Research 39: 753–64. [ Google Scholar ] [ CrossRef ]
- Alekneviciene, Vilija, Egle Alekneviciute, and Rasa Rinkeviciene. 2012. Portfolio Size and Diversification Effect in Lithuanian Stock Exchange Market. Engineering Economics 23: 338–47. [ Google Scholar ] [ CrossRef ] [ Green Version ]
- Alexeev, Vitali, and Mardi Dungey. 2015. Equity portfolio diversification with high frequency data. Quantitative Finance 15: 1205–15. [ Google Scholar ] [ CrossRef ] [ Green Version ]
- Alexeev, Vitali, and Francis Tapon. 2012. Equity Portfolio Diversification: How Many Stocks are Enough? Evidence from Five Developed Markets. SSRN Electronic Journal . [ Google Scholar ] [ CrossRef ] [ Green Version ]
- Alexeev, Vitali, and Francis Tapon. 2014. How Many Stocks Are Enough for Diversifying Canadian Institutional Portfolios? Hobart: University of Tasmania. [ Google Scholar ]
- Alexeev, Vitali, Giovanni Urga, and Wenying Yao. 2019. Asymmetric jump beta estimation with implications for portfolio risk management. International Review of Economics & Finance 62: 20–40. [ Google Scholar ] [ CrossRef ]
- Alqahtani, Faisal, Nader Trabelsi, Nahla Samargandi, and Syed Jawad Hussain Shahzad. 2020. Tail Dependence and Risk Spillover from the US to GCC Banking Sectors. Mathematics 8: 2055. [ Google Scholar ] [ CrossRef ]
- Anas, Muhammad, Ghulam Mujtaba, Sadaf Nayyar, and Saira Ashfaq. 2020. Time-Frequency Based Dynamics of Decoupling or Integration between Islamic and Conventional Equity Markets. Journal of Risk and Financial Management 13: 156. [ Google Scholar ] [ CrossRef ]
- Ang, Andrew, and Geert Bekaert. 2002. International Asset Allocation With Regime Shifts. The Review of Financial Studies 15: 1137–87. [ Google Scholar ] [ CrossRef ]
- Angelidis, Timotheos, and Nikolaos Tessaromatis. 2010. The efficiency of Greek public pension fund portfolios. Journal of Banking & Finance 34: 2158–67. [ Google Scholar ] [ CrossRef ]
- Arjoon, Vaalmikki, and Chandra Shekhar Bhatnagar. 2017. Dynamic herding analysis in a frontier market. Research in International Business and Finance 42: 496–508. [ Google Scholar ] [ CrossRef ]
- Azmi, Wajahat, Adam Ng, Ginanjar Dewandaru, and Ruslan Nagayev. 2019. Doing well while doing good: The case of Islamic and sustainability equity investing. Borsa Istanbul Review 19: 207–18. [ Google Scholar ] [ CrossRef ]
- Babalos, Vassilios, and Stavros Stavroyiannis. 2017. Modelling correlation dynamics of EMU sovereign debt markets during the recent turmoil. Research in International Business and Finance 42: 1021–29. [ Google Scholar ] [ CrossRef ]
- Bai, Min, Yafeng Qin, and Huiping Zhang. 2021. Stock price crashes in emerging markets. International Review of Economics & Finance 72: 466–82. [ Google Scholar ] [ CrossRef ]
- Bajgrowicz, Pierre, Olivier Scaillet, and Adrien Treccani. 2016. Jumps in High-Frequency Data: Spurious Detections, Dynamics, and News. Management Science 62: 2198–217. [ Google Scholar ] [ CrossRef ] [ Green Version ]
- Baker, Kent, Adri De Ridder, and Annalien De Vries. 2018. Stockholdings of first-time and more experienced investors. Review of Behavioral Finance 10: 146–62. [ Google Scholar ] [ CrossRef ]
- Balcılar, Mehmet, Rıza Demirer, and Shawkat Hammoudeh. 2015. Regional and global spillovers and diversification opportunities in the GCC equity sectors. Emerging Markets Review 24: 160–87. [ Google Scholar ] [ CrossRef ]
- Ban, Gah-Yi, Noureddine El Karoui, and Andrew E. B. Lim. 2016. Machine Learning and Portfolio Optimization. Management Science 64: 1136–54. [ Google Scholar ] [ CrossRef ] [ Green Version ]
- Barber, Brad M., and Terrance Odean. 2000. Too Many Cooks Spoil the Profits: Investment Club Performance. Financial Analysts Journal 56: 17–25. [ Google Scholar ] [ CrossRef ]
- Basu, Anup K., and Jason Huang-Jones. 2015. The performance of diversified emerging market equity funds. Journal of International Financial Markets, Institutions and Money 35: 116–31. [ Google Scholar ] [ CrossRef ] [ Green Version ]
- Batten, Jonathan A., Peter G. Szilagyi, and Niklas F. Wagner. 2015. Should emerging market investors buy commodities? Applied Economics 47: 4228–46. [ Google Scholar ] [ CrossRef ]
- Batten, Jonathan A., Harald Kinateder, Peter G. Szilagyi, and Niklas F. Wagner. 2017. Can stock market investors hedge energy risk? Evidence from Asia. Energy Economics 66: 559–70. [ Google Scholar ] [ CrossRef ]
- Beck, Kristine L., Sieven B. Perfect, and Pamela P. Peterson. 1996. The Role of Alternative Methodology on the Relation Between Portfolio Size and Diversification. The Financial Review 31: 381–406. [ Google Scholar ] [ CrossRef ]
- Bekaert, Gert, Claude B. Erb, Campbell R. Harvey, and Tadas E. Viskanta. 1998. Distributional characteristics of emerging market returns and asset allocation. The Journal of Portfolio Management 24: 102–16. [ Google Scholar ] [ CrossRef ]
- Benjelloun, Hicham. 2010. Evans and Archer—Forty years later. Investment Management and Financial Innovations 7: 8. [ Google Scholar ]
- Berger, Dave, Kuntara Pukthuanthong, and J. Jimmy Yang. 2011. International diversification with frontier markets. Journal of Financial Economics 101: 227–42. [ Google Scholar ] [ CrossRef ]
- Bergmann, Daniel Reed, Jose Roberto Ferreira Savoia, Claudio Felisoni de Angelo, Eduardo Augusto do Rosário Contani, and Fabiana Lopes da Silva. 2018. Portfolio management with tail dependence. Applied Economics 50: 5510–20. [ Google Scholar ] [ CrossRef ]
- Bhatti, M. Ishaq, and Cuong C. Nguyen. 2012. Diversification evidence from international equity markets using extreme values and stochastic copulas. Journal of International Financial Markets, Institutions and Money 22: 622–46. [ Google Scholar ] [ CrossRef ]
- Bradfield, David, and Brian Munro. 2017. The number of stocks required for effective portfolio diversification: The South African case. South African Journal of Accounting Research 31: 44–59. [ Google Scholar ] [ CrossRef ]
- Brands, Simone, and David R. Gallagher. 2005. Portfolio selection, diversification and fund-of-funds: A note. Accounting & Finance 45: 185–97. [ Google Scholar ] [ CrossRef ]
- Branger, Nicole, Katarína Lučivjanská, and Alex Weissensteiner. 2019. Optimal granularity for portfolio choice. Journal of Empirical Finance 50: 125–46. [ Google Scholar ] [ CrossRef ]
- Campbell, John Y., Martin Lettau, Burton G. Malkiel, and Yexiao Xu. 2001. Have Individual Stocks Become More Volatile? An Empirical Exploration of Idiosyncratic Risk. The Journal of Finance 56: 1–43. [ Google Scholar ] [ CrossRef ]
- Cha, Heung-Joo, and Thadavillil Jithendranathan. 2009. Time-varying correlations and optimal allocation in emerging market equities for the US investors. International Journal of Finance & Economics 14: 172–87. [ Google Scholar ] [ CrossRef ] [ Green Version ]
- Chen, Feng, Ole-Kristian Hope, Qingyuan Li, and Xin Wang. 2018. Flight to Quality in International Markets: Investors’ Demand for Financial Reporting Quality during Political Uncertainty Events. Contemporary Accounting Research 35: 117–55. [ Google Scholar ] [ CrossRef ]
- Chen, Ke, Luiz Vitiello, Stuart Hyde, and Ser-Huang Poon. 2018. The reality of stock market jumps diversification. Journal of International Money and Finance 86: 171–88. [ Google Scholar ] [ CrossRef ] [ Green Version ]
- Chiou, Wan-Jiun Paul. 2008. Who benefits more from international diversification? Journal of International Financial Markets, Institutions and Money 18: 466–82. [ Google Scholar ] [ CrossRef ]
- Chong, James, and G. Michael Phillips. 2013. Portfolio Size Revisited. The Journal of Wealth Management 15: 49–60. [ Google Scholar ] [ CrossRef ]
- Conlon, Thomas, John Cotter, and Ramazan Gençay. 2018. Long-run wavelet-based correlation for financial time series. European Journal of Operational Research 271: 676–96. [ Google Scholar ] [ CrossRef ]
- Conover, C. Mitchell, Gerald R. Jensen, and Robert R. Johnson. 2002. Emerging Markets: When Are They Worth It? Financial Analysts Journal 58: 86–95. [ Google Scholar ] [ CrossRef ]
- Corzo, Teresa, Laura Lazcano, Javier Márquez, Laura Gismera, and Sara Lumbreras. 2020. A common risk factor in global credit and equity markets: An exploratory analysis of the subprime and the sovereign-debt crises. Heliyon 6: e03980. [ Google Scholar ] [ CrossRef ] [ PubMed ]
- Dbouk, Wassim, and Lawrence Kryzanowski. 2009. Diversification benefits for bond portfolios. The European Journal of Finance 15: 533–53. [ Google Scholar ] [ CrossRef ]
- De Keyzer, Olivier, and Michiel De Schaepmeester. 2014. How Many Stocks Does an Investor Need to Diversify within Europe? Master’s thesis, University of Ghent, Ghent, Belgium. [ Google Scholar ]
- Delpini, Danilo, Stefano Battiston, Guido Caldarelli, and Massimo Riccaboni. 2019. Systemic risk from investment similarities. PLoS ONE 14: e0217141. [ Google Scholar ] [ CrossRef ] [ PubMed ] [ Green Version ]
- Dimitrios, Kydros, and Oumbailis Vasileios. 2015. A Network Analysis of the Greek Stock Market. Procedia Economics and Finance 33: 340–49. [ Google Scholar ] [ CrossRef ] [ Green Version ]
- Dimitriou, Dimitrios, Dimitris Kenourgios, and Theodore Simos. 2017. Financial crises, exchange rate linkages and uncovered interest parity: Evidence from G7 markets. Economic Modelling 66: 112–20. [ Google Scholar ] [ CrossRef ]
- Diyarbakırlıoğlu, Erkin, and Mehmet H. Satman. 2013. The Maximum Diversification Index. Journal of Asset Management 14: 400–9. [ Google Scholar ] [ CrossRef ]
- Domian, Dale L., David A. Louton, and Marie D. Racine. 2007. Diversification in Portfolios of Individual Stocks: 100 Stocks Are Not Enough. Financial Review 42: 557–70. [ Google Scholar ] [ CrossRef ]
- Dopfel, Frederick E. 2003. Asset Allocation in a Lower Stock-Bond Correlation Environment. The Journal of Portfolio Management 30: 25–38. [ Google Scholar ] [ CrossRef ]
- Dorn, Daniel, and Gur Huberman. 2010. Preferred risk habitat of individual investors. Journal of Financial Economics 97: 155–73. [ Google Scholar ] [ CrossRef ]
- Duarte, Flávio Gabriel, and Leandro Nunes De Castro. 2020. A Framework to Perform Asset Allocation Based on Partitional Clustering. IEEE Access 8: 110775–88. [ Google Scholar ] [ CrossRef ]
- Eom, Cheoljun, Taisei Kaizoji, Giacomo Livan, and Enrico Scalas. 2021. Limitations of portfolio diversification through fat tails of the return Distributions: Some empirical evidence. The North American Journal of Economics and Finance 56: 101358. [ Google Scholar ] [ CrossRef ]
- Evans, John L., and Stephen H. Archer. 1968. Diversification and the Reduction of Dispersion: An Empirical Analysis. The Journal of Finance 23: 761–67. [ Google Scholar ] [ CrossRef ]
- Fang, Kuangnan, Ji Wu, and Cuong Nguyen. 2017. The Risk-Return Trade-Off in a Liberalized Emerging Stock Market: Evidence from Vietnam. Emerging Markets Finance and Trade 53: 746–63. [ Google Scholar ] [ CrossRef ]
- Fazil, Gökgöz, and Altintaş İpek. 2013. Does Idiosyncratic Volatility Matter in the Emerging Markets? Istanbul Stock Exchange Evidence. Economic Research-Ekonomska Istraživanja 26: 133–50. [ Google Scholar ] [ CrossRef ] [ Green Version ]
- Fielitz, Bruce D. 1974. Indirect versus Direct Diversification. Financial Management 3: 54–62. [ Google Scholar ] [ CrossRef ]
- Flavin, Thomas J., and Michael R. Wickens. 2006. Optimal International Asset Allocation with Time-Varying Risk. Scottish Journal of Political Economy 53: 543–64. [ Google Scholar ] [ CrossRef ] [ Green Version ]
- Fong, Tom Pak Wing, Angela Kin Wan Sze, and Edmund Ho Cheung Ho. 2018. Determinants of equity mutual fund flows—Evidence from the fund flow dynamics between Hong Kong and global markets. Journal of International Financial Markets, Institutions and Money 57: 231–47. [ Google Scholar ] [ CrossRef ]
- Fugazza, Carolina, Maela Giofré, and Giovanna Nicodano. 2011. International diversification and industry-related labor income risk. International Review of Economics & Finance 20: 764–83. [ Google Scholar ] [ CrossRef ]
- Gasser, Stephan M., Margarethe Rammerstorfer, and Karl Weinmayer. 2017. Markowitz revisited: Social portfolio engineering. European Journal of Operational Research 258: 1181–90. [ Google Scholar ] [ CrossRef ] [ Green Version ]
- Gilmore, Claire G., and Ginette M. McManus. 2002. International portfolio diversification: US and Central European equity markets. Emerging Markets Review 3: 69–83. [ Google Scholar ] [ CrossRef ]
- Grauer, Robert R., and Frederick C. Shen. 2000. Do constraints improve portfolio performance? Journal of Banking & Finance 24: 1253–74. [ Google Scholar ] [ CrossRef ]
- Guidolin, Massimo, and Allan Timmermann. 2008. International asset allocation under regime switching, skew, and kurtosis preferences. The Review of Financial Studies 21: 889–935. [ Google Scholar ] [ CrossRef ]
- Gupta, G. S., and Ch’ng Huck Khoon. 2001. How many securities make a diversified portfolio: KLSE stocks? Asian Academy of Management Journal 6: 63–79. [ Google Scholar ]
- Habibah, Ume, Ranjeeta Sadhwani, and Pervaiz Memon. 2018. Selecting Optimal Portfolio in Pakistan. Sukkur IBA Journal of Economics and Finance 2: 68–77. [ Google Scholar ] [ CrossRef ]
- Haddad, Michel Ferreira Cardia. 2019. Sphere-sphere intersection for investment portfolio diversification—A new data-driven cluster analysis. MethodsX 6: 1261–78. [ Google Scholar ] [ CrossRef ]
- Hadhri, Sinda. 2021. The nexus, downside risk and asset allocation between oil and Islamic stock markets: A cross-country analysis. Energy Economics 101: 105448. [ Google Scholar ] [ CrossRef ]
- Hadhri, Sinda, and Zied Ftiti. 2019. Asset allocation and investment opportunities in emerging stock markets: Evidence from return asymmetry-based analysis. Journal of International Money and Finance 93: 187–200. [ Google Scholar ] [ CrossRef ]
- Haensly, Paul J. 2020. Risk decomposition, estimation error, and naïve diversification. The North American Journal of Economics and Finance 52: 101146. [ Google Scholar ] [ CrossRef ]
- Halunga, Andreea G., and Christos S. Savva. 2019. Neglecting structural breaks when estimating and valuing dynamic correlations for asset allocation. Econometric Reviews 38: 660–78. [ Google Scholar ] [ CrossRef ]
- Haroon, Omair, Mohsin Ali, Abdullah Khan, Mudeer A. Khattak, and Syed Aun R. Rizvi. 2021. Financial Market Risks during the COVID-19 Pandemic. Emerging Markets Finance and Trade 57: 2407–14. [ Google Scholar ] [ CrossRef ]
- Helberg, Stig, and Snorre Lindset. 2020. Collateral affects return risk: Evidence from the euro bond market. Financial Markets and Portfolio Management 34: 99–128. [ Google Scholar ] [ CrossRef ]
- Herold, Ulf, and Raimond Maurer. 2003. Bayesian Asset Allocation and U.S. Domestic Bias. Financial Analysts Journal 59: 54–65. [ Google Scholar ] [ CrossRef ]
- Horvath, Roman, and Petr Poldauf. 2012. International Stock Market Comovements: What Happened during the Financial Crisis? Global Economy Journal 12: 1850252. [ Google Scholar ] [ CrossRef ] [ Green Version ]
- Hu, Jin-Li, Tzu-Pu Chang, and Ray Yeutien Chou. 2014. Market conditions and the effect of diversification on mutual fund performance: Should funds be more concentrative under crisis? Journal of Productivity Analysis 41: 141–51. [ Google Scholar ] [ CrossRef ]
- Hwang, Inchang, Simon Xu, and Francis In. 2018. Naive versus optimal diversification: Tail risk and performance. European Journal of Operational Research 265: 372–88. [ Google Scholar ] [ CrossRef ]
- Irala, Lokanandha Reddy, and Prakash Patil. 2007. Portfolio Size and Diversification. SCMS Journal of Indian Management 4: 6. [ Google Scholar ]
- Jain, Prayut, and Shashi Jain. 2019. Can Machine Learning-Based Portfolios Outperform Traditional Risk-Based Portfolios? The Need to Account for Covariance Misspecification. Risks 7: 74. [ Google Scholar ] [ CrossRef ] [ Green Version ]
- Kang, Jun-Koo, and Rene´M. Stulz. 1997. Why is there a home bias? An analysis of foreign portfolio equity ownership in Japan. Journal of Financial Economics 46: 3–28. [ Google Scholar ] [ CrossRef ] [ Green Version ]
- Kim, Woohwan, Young Min Kim, Tae-Hwan Kim, and Seungbeom Bang. 2018. Multi-dimensional portfolio risk and its diversification: A note. Global Finance Journal 35: 147–56. [ Google Scholar ] [ CrossRef ]
- Kisaka, Sifunjo E., Joseph Aloise Mbithi, and Hilary Kitur. 2015. Determining the Optimal Portfolio Size on the Nairobi Securities Exchange. Research Journal of Finance and Accounting 6: 215. [ Google Scholar ]
- Kiymaz, Halil, and Koray Simsek. 2017. The performance of US-based emerging market mutual funds. Journal of Capital Markets Studies 1: 58–73. [ Google Scholar ] [ CrossRef ] [ Green Version ]
- Koedijk, Kees G., Alfred M. H. Slager, and Philip A. Stork. 2016. Investing in Systematic Factor Premiums: Investing in Systematic Factor Premiums. European Financial Management 22: 193–234. [ Google Scholar ] [ CrossRef ]
- Kreso, Inda, Amra Kapo, and Lejla Turulja. 2020. Data mining privacy preserving: Research agenda. Wiley Interdisciplinary Reviews: Data Mining and Knowledge Discovery 1: 1–29. [ Google Scholar ] [ CrossRef ]
- Kroencke, Tim A., and Felix Schindler. 2012. International diversification with securitized real estate and the veiling glare from currency risk. Journal of International Money and Finance 31: 1851–66. [ Google Scholar ] [ CrossRef ] [ Green Version ]
- Kryzanowski, Lawrence, and Shishir Singh. 2010. Should Minimum Portfolio Sizes Be Prescribed for Achieving Sufficiently Well-Diversified Equity Portfolios? SSRN Electronic Journal 7: 1–37. [ Google Scholar ]
- Kurtti, Markku. 2020. How Many stocks Make a Diversified Portfolio in a Continuous-time World? Master’s thesis, University of Oulu, Oulu, Finland. [ Google Scholar ]
- Kushnirovich, Nonna. 2016. Immigrant investors in financial markets: Modes of financial behavior. Journal of Business Economics and Management 17: 992–1006. [ Google Scholar ] [ CrossRef ] [ Green Version ]
- Lee, Kuan-Hui. 2011. The world price of liquidity risk. Journal of Financial Economics 99: 136–61. [ Google Scholar ] [ CrossRef ]
- Lee, Darren D., Jacquelyn E. Humphrey, Karen L. Benson, and Jason Y. K. Ahn. 2010. Socially responsible investment fund performance: The impact of screening intensity. Accounting & Finance 50: 351–70. [ Google Scholar ] [ CrossRef ]
- Lee, Yongjae, Woo Chang Kim, and Jang Ho Kim. 2020. Achieving Portfolio Diversification for Individuals with Low Financial Sustainability. Sustainability 12: 7073. [ Google Scholar ] [ CrossRef ]
- Lee, Cheng-Few, Chengru Hu, and Maggie Foley. 2021. Differential risk effect of inside debt, CEO compensation diversification, and firm investment. Review of Quantitative Finance and Accounting 56: 505–43. [ Google Scholar ] [ CrossRef ]
- Levy, Haim, and Moshe Levy. 2021. The cost of diversification over time, and a simple way to improve target-date funds. Journal of Banking & Finance 122: 105995. [ Google Scholar ] [ CrossRef ]
- Li, Hong. 2013. Integration versus segmentation in China’s stock market: An analysis of time-varying beta risks. Journal of International Financial Markets, Institutions and Money 25: 88–105. [ Google Scholar ] [ CrossRef ]
- Li, Mingsheng, and Xin Zhao. 2016. Neighborhood effect on stock price comovement. The North American Journal of Economics and Finance 35: 1–22. [ Google Scholar ] [ CrossRef ]
- Ling, David C., and Andy Naranjo. 2002. Commercial Real Estate Return Performance: A Cross-Country Analysis. The Journal of Real Estate Finance and Economics 24: 119–42. [ Google Scholar ] [ CrossRef ]
- Liu, Edith X. 2016. Portfolio Diversification and International Corporate Bonds. Journal of Financial and Quantitative Analysis 51: 959–83. [ Google Scholar ] [ CrossRef ]
- Lu, Xiaomeng, Jiaojiao Guo, and Li Gan. 2020. International comparison of household asset allocation: Micro-evidence from cross-country comparisons. Emerging Markets Review 43: 100691. [ Google Scholar ] [ CrossRef ]
- Lubatkin, Michael, and Sayan Chatterjee. 1994. Extending Modern Portfolio Theory into the Domain of Corporate Diversification: Does it Apply? Academy of Management Journal 37: 109–36. [ Google Scholar ] [ CrossRef ]
- Magas, I. 2007. The changing benefits of global equity investing: Developed and emerging markets, 1997–2007. Acta Oeconomica 57: 343–62. [ Google Scholar ] [ CrossRef ]
- Markowitz, Harry. 1952. Portfolio Selection. The Journal of Finance 7: 77–91. [ Google Scholar ] [ CrossRef ]
- Martin-Bujack, Karin, Teresa Santamaría, and Isabel Figuerola-Ferretti. 2018. International diversification and global credit risk: A methodology for portfolio building 1. UCJC Business and Society Review 15: 92–136. [ Google Scholar ] [ CrossRef ]
- Meng, Xiangcai, and Chia-Hsing Huang. 2019. The time-frequency co-movement of Asian effective exchange rates: A wavelet approach with daily data. The North American Journal of Economics and Finance 48: 131–48. [ Google Scholar ] [ CrossRef ]
- Mensi, Walid, Shawkat Hammoudeh, Ahmet Sensoy, and Seong-Min Yoon. 2017. Analysing dynamic linkages and hedging strategies between Islamic and conventional sector equity indexes. Applied Economics 49: 2456–79. [ Google Scholar ] [ CrossRef ]
- Mensi, Walid, Mobeen Ur Rehman, Debasish Maitra, Khamis Hamed Al-Yahyaee, and Ahmet Sensoy. 2020. Does bitcoin co-move and share risk with Sukuk and world and regional Islamic stock markets? Evidence using a time-frequency approach. Research in International Business and Finance 53: 101230. [ Google Scholar ] [ CrossRef ]
- Milcheva, Stanimira, and Bing Zhu. 2018. Asset pricing, spatial linkages and contagion in real estate stocks. Journal of Property Research 35: 271–95. [ Google Scholar ] [ CrossRef ]
- Miralles-Marcelo, José Luis, María del Mar Miralles-Quirós, and José Luis Miralles-Quirós. 2012. Asset pricing with idiosyncratic risk: The Spanish case. International Review of Economics & Finance 21: 261–71. [ Google Scholar ] [ CrossRef ]
- Miralles-Quirós, José Luis, María Mar Miralles-Quirós, and José Manuel Nogueira. 2019. Diversification benefits of using exchange-traded funds in compliance to the sustainable development goals. Business Strategy and the Environment 28: 244–55. [ Google Scholar ] [ CrossRef ] [ Green Version ]
- Mitton, Todd, and Keith Vorkink. 2007. Equilibrium Underdiversification and the Preference for Skewness. The Review of Financial Studies 20: 1255–88. [ Google Scholar ] [ CrossRef ]
- Mokni, Khaled, and Faysal Mansouri. 2017. Conditional dependence between international stock markets: A long memory GARCH-copula model approach. Journal of Multinational Financial Management 42–43: 116–31. [ Google Scholar ] [ CrossRef ]
- Murthy, Jogalapuram. 2018. The construction of optimal portfolio using sharpe’s single index model-an empirical study on nifty metal index. JIMS8M: The Journal of Indian Management & Strategy 23: 4. [ Google Scholar ] [ CrossRef ]
- Ngene, Geoffrey, Jordin A. Post, and Ann N. Mungai. 2018. Volatility and shock interactions and risk management implications: Evidence from the U.S. and frontier markets. Emerging Markets Review 37: 181–98. [ Google Scholar ] [ CrossRef ]
- Nguyen, Tung Dao, and Pana Elisabeta. 2016. Financial integration and diversification benefits: China and ASEAN4 countries. Managerial Finance 42: 496–514. [ Google Scholar ] [ CrossRef ]
- Norsiman, Nadia, Noor Yakob, and Carl Mcgowan. 2019. The Effect of Portfolio Diversification for the Bursa Malaysia. Accounting and Finance Research 8: 76. [ Google Scholar ] [ CrossRef ]
- O’Neal, Edward S. 1997. How Many Mutual Funds Constitute a Diversified Mutual Fund Portfolio? Financial Analysts Journal 53: 37–46. [ Google Scholar ] [ CrossRef ]
- Oyenubi, Adeola. 2019. Diversification Measures and the Optimal Number of Stocks in a Portfolio: An Information Theoretic Explanation. Computational Economics 54: 1443–71. [ Google Scholar ] [ CrossRef ] [ Green Version ]
- Pástor, Ľuboš, Robert F. Stambaugh, and Lucian A. Taylor. 2020. Fund tradeoffs. Journal of Financial Economics 138: 614–34. [ Google Scholar ] [ CrossRef ]
- Pizzutilo, Fabio. 2017. Measuring the under-diversification of socially responsible investments. Applied Economics Letters 24: 1005–18. [ Google Scholar ] [ CrossRef ]
- Platanakis, Emmanouil, Charles Sutcliffe, and Xiaoxia Ye. 2021. Horses for courses: Mean-variance for asset allocation and 1/N for stock selection. European Journal of Operational Research 288: 302–17. [ Google Scholar ] [ CrossRef ]
- Rahiminezhad Galankashi, Masoud, Farimah Mokhatab Rafiei, and Maryam Ghezelbash. 2020. Portfolio selection: A fuzzy-ANP approach. Financial Innovation 6: 17. [ Google Scholar ] [ CrossRef ]
- Raju, Rajan, and Sobhesh Kumar Agarwalla. 2021. Equity Portfolio Diversification: How Many Stocks Are Enough? Evidence from India. SSRN Electronic Journal . [ Google Scholar ] [ CrossRef ]
- Rajwani, Shegorika, and Dilip Kumar. 2016. Asymmetric Dynamic Conditional Correlation Approach to Financial Contagion: A Study of Asian Markets. Global Business Review 17: 1339–56. [ Google Scholar ] [ CrossRef ]
- Rehman, Mobeen Ur, Syed Jawad Hussain Shahzad, Nasir Ahmad, and Xuan Vinh Vo. 2021. Dependence among metals and mining companies of the US and Europe during normal and crises periods. Resources Policy 73: 102199. [ Google Scholar ] [ CrossRef ]
- Rizvi, Syed Aun R., and Shaista Arshad. 2018. Understanding time-varying systematic risks in Islamic and conventional sectoral indices. Economic Modelling 70: 561–70. [ Google Scholar ] [ CrossRef ]
- Sandoval, Leonidas, and Italo De Paula Franca. 2012. Correlation of financial markets in times of crisis. Physica A: Statistical Mechanics and Its Applications 391: 187–208. [ Google Scholar ] [ CrossRef ] [ Green Version ]
- Sclip, Alex, Alberto Dreassi, Stefano Miani, and Andrea Paltrinieri. 2016. Dynamic correlations and volatility linkages between stocks and sukuk: Evidence from international markets. Review of Financial Economics 31: 34–44. [ Google Scholar ] [ CrossRef ] [ Green Version ]
- Sharma, Prateek, and Vipul. 2018. Improving portfolio diversification: Identifying the right baskets for putting your eggs. Managerial and Decision Economics 39: 698–711. [ Google Scholar ] [ CrossRef ]
- Shawky, Hany A., and David M. Smith. 2005. Optimal Number of Stock Holdings in Mutual Fund Portfolios Based on Market Performance. Financial Review 40: 481–95. [ Google Scholar ] [ CrossRef ]
- Smimou, Kamal. 2014. International portfolio choice and political instability risk: A multi-objective approach. European Journal of Operational Research 234: 546–60. [ Google Scholar ] [ CrossRef ]
- Solnik, Bruno H. 1974. Why Not Diversify Internationally Rather Than Domestically? Financial Analysts Journal 30: 48–54. [ Google Scholar ] [ CrossRef ]
- Statman, Meir. 1987. How Many Stocks Make a Diversified Portfolio? Journal of Financial and Quantitative Analysis 22: 353–63. [ Google Scholar ] [ CrossRef ]
- Statman, Meir. 2002. How Much Diversification Is Enough? Working Paper. Santa Clara: Leavey School of Business, Santa Clara University. [ Google Scholar ] [ CrossRef ] [ Green Version ]
- Statman, Meir. 2004. The Diversification Puzzle. Financial Analysts Journal 60: 44–53. [ Google Scholar ] [ CrossRef ] [ Green Version ]
- Stotz, Andrew, and Wei Lu. 2014. Eight Stocks are Enough in China. SSRN Electronic Journal . [ Google Scholar ] [ CrossRef ]
- Susmel, Raul. 2001. Extreme observations and diversification in Latin American emerging equity markets. Journal of International Money and Finance 20: 971–86. [ Google Scholar ] [ CrossRef ] [ Green Version ]
- Syriopoulos, Theodore, Beljid Makram, and Adel Boubaker. 2015. Stock market volatility spillovers and portfolio hedging: BRICS and the financial crisis. International Review of Financial Analysis 39: 7–18. [ Google Scholar ] [ CrossRef ]
- Tai, Chu-Sheng. 2018. International diversification during financial crises. Managerial Finance 44: 1434–45. [ Google Scholar ] [ CrossRef ]
- Tang, Gordon Y. N. 2004. How efficient is naive portfolio diversification? an educational note. Omega 32: 155–60. [ Google Scholar ] [ CrossRef ]
- Tang, Xuan, Xing Gao, Qiuping Zhou, and Jian Ma. 2020. The BSS-FM Estimation of International Assets Allocation for China Mainland Investors. Emerging Markets Finance and Trade 56: 1224–36. [ Google Scholar ] [ CrossRef ]
- Topaloglou, Nikolas, Hercules Vladimirou, and Stavros A. Zenios. 2002. CVaR models with selective hedging for international asset allocation. Journal of Banking & Finance 26: 1535–61. [ Google Scholar ] [ CrossRef ]
- Tripathi, Vanita, and Varun Bhandari. 2015. Socially responsible stocks: A boon for investors in India. Journal of Advances in Management Research 12: 209–25. [ Google Scholar ] [ CrossRef ]
- Wander, Brett H., and Ron D’Vari. 2003. The Limitations of Standard Deviation as a Measure of Bond Portfolio Risk. The Journal of Wealth Management 6: 35–38. [ Google Scholar ] [ CrossRef ]
- Wang, Kehluh, Yi-Hsuan Chen, and Szu-Wei Huang. 2011. The dynamic dependence between the Chinese market and other international stock markets: A time-varying copula approach. International Review of Economics & Finance 20: 654–64. [ Google Scholar ] [ CrossRef ]
- Warken, Peter, and Christian Hille. 2018. A risk-based approach to construct multi asset portfolio solutions. The Journal of Investment Strategies 7: 33–51. [ Google Scholar ] [ CrossRef ]
- Wilson, Bonnie. 2004. Diversification of risk and growth. Macroeconomic Dynamics 8: 335–61. [ Google Scholar ] [ CrossRef ]
- Wu, Chih-Chiang, and Shin-Shun Liang. 2011. The economic value of range-based covariance between stock and bond returns with dynamic copulas. Journal of Empirical Finance 18: 711–27. [ Google Scholar ] [ CrossRef ]
- Yilmaz, Tolgahan, and Sema Dube. 2014. Asset allocation and stock selection: Evidence from static and dynamic strategies in Turkish markets. Iktisat Isletme ve Finans 29: 73–94. [ Google Scholar ] [ CrossRef ]
- Zaremba, Adam. 2018. Country Risk and Expected Returns Across Global Equity Markets. Czech Journal of Economics and Finance 68: 374–98. [ Google Scholar ] [ CrossRef ]
- Zhou, Yuan. 2014. Number of Stocks in Portfolio and Risk Reduction. Master’s thesis, University of California, Los Angeles, CA, USA. [ Google Scholar ]
- Zhu, Hui-Ming, Rong Li, and Sufang Li. 2014. Modelling dynamic dependence between crude oil prices and Asia-Pacific stock market returns. International Review of Economics & Finance 29: 208–23. [ Google Scholar ] [ CrossRef ]
Click here to enlarge figure
Author(s) and Year | Research Focus | Market(s) and the Observed Period | Method(s) | Research Findings | Number of Stocks or Other Information |
---|---|---|---|---|---|
( ) | To study the rate at which return fluctuations decrease for randomly selected portfolios as a function of the number of securities in the portfolio. | U.S. 1958–1967 | Equally Weighted Portfolio, Standard Deviation | The results also cast doubt on whether it is economically justified to increase portfolio size beyond 10 or more securities and suggest that both analysts and private investors need to incorporate some form of marginal analysis into their portfolio selection model. | 8–10 stocks |
( ) | Analyze how much to invest in each security to ensure that the return on the investment is not significantly affected by commission charges. | U.S. 1964–1968 | Mean Absolute Deviation (MAD), Equally Weighted Portfolio | Direct investment in a randomly diversified portfolio of common stocks is preferable because the return on the random stock portfolio is, on average, higher than that achievable with mutual funds. | 8 stocks |
( ) | To show that substantial risk reduction benefits can be achieved by portfolio diversification into foreign securities as well as domestic common stocks. | U.S., UK, Germany, France, Switzerland, Italy, Belgium and Netherland 1966–1971 | Standard Deviation | Foreign capital markets differ significantly from the U.S. market. Because European investors often find that their domestic markets do not offer the variety of investment opportunities that Americans enjoy, international diversification is relatively more attractive to them. | 10–15 stocks |
( ) | To show that no fewer than 30 stocks are required for a well-diversified portfolio and to compare this result with the levels of diversification observed in studies of individual investors’ portfolios. | U.S. 1979–1984 | Standard Deviation | The benefits of diversification for stock portfolios are exhausted when the number of stocks reaches 10 or 15. | 30 for borrowing investors and 40 for the lending investors |
( ) | To investigate the development of alternative methods that reduce the impact of repeated replications on test results. | U.S. 1982–1991 | Variance, Correlations, Kruskal-Wallis test, Chi-square test | Researchers studying the issue of portfolio size and portfolio variance may be misled by replication-sensitive tests. | 14–20 securities |
( ) | To examine the impact of holding various numbers of mutual funds on the expected variability of investors’ terminal wealth. | U.S. 1976–1994 | Standard Deviation, Semi-variance, Terminal Wealth Standard Deviation | Two out of three downside risk measures are also substantially reduced by including multiple funds in a portfolio. | 16–18 FoF |
( ) | To investigate the size of a well-diversified portfolio in Malaysia and determine the size of the diversified portfolio for each of the lending and borrowing investors. | Malaysia 1988–1997 | Standard Deviation, T-test | A portfolio of 30 securities results in a well-diversified portfolio for borrowing investors, and one of 50 securities for lending investors. | 27 securities |
( ) | To analyze the performance of a randomly selected sample of 166 investment clubs. | U.S. 1991–1997 | CAPM, Jensen’s Alpha, Fama–French Three-Factor Model | The average club invested in high beta common stocks, small-cap growth stocks, and turned over 65% of its portfolio annually; 60% of clubs underperformed the index. | 7–8 stocks |
( ) | To analyze the optimal number of stocks needed to achieve maximum diversification effects and compare the benefits and costs of diversification using mean-variance and behavioral portfolio theory. | U.S. 1926–2001 | Mean-variance Portfolio Theory, Behavioral Portfolio Theory | The behavior of investors is better described with behavioral portfolio theory; the benefits and costs of diversification under the rules of mean-variance portfolio theory are different from those under the rules of behavioral portfolio theory. | More than 120 stocks |
( ) | To analytically examine the efficiency of naive diversification from an educational point of view. | International 1991–2002 | Variance | Given an infinite population of stocks, a portfolio size of 20 is required to eliminate 95% of the diversifiable risk on average; adding 80 stocks is required to eliminate an additional 4% of the diversifiable risk on average. | 20 stocks |
( ) | To investigate whether the number of stocks required to reduce unsystematic risk has changed over time. | U.S. 1926–2001 | Standard Deviation, Mean-variance Portfolio Theory, Behavioral Portfolio Theory | The diversification puzzle can be solved within the framework of behavioral portfolio theory. | More than 300 stocks |
( ) | Attention to FoF portfolio configuration for Australian investors, examining FoF performance and risk characteristics within traditional asset classes. | Australia 1989–1999 | Standard Deviation, Terminal Wealth Standard Deviation, Sharpe Ratio, Skewness, Kurtosis | As the number of funds in an FoF portfolio increases, performance improves in a mean-variance setting; adding funds to the FoF structure leads to deterioration in FoF portfolio skewness and kurtosis. | 6 FoF |
( ) | Investigate the relationship between the risk-adjusted return and the number of stocks. | U.S. 1992–2000 | Correlation, Ordinary Least Squares Regression (OLS) | Changes in the number of stocks are closely related to the number of new investments and redemptions, but not to changes in fund size due to market returns. | 40–120 stocks |
( ) | To compare results of random diversification with portfolios diversified by industry group. | U.S. 1985–2004 | Shortfall Risk, Ending Wealth, F-test, Industry Diversification | For small portfolios, a small reduction in risk can be achieved by diversifying across industries, but a larger reduction is achieved by simply increasing the number of stocks. | More than 100 stocks |
( ) | To analyze the optimal number of stocks required to achieve maximum diversification effect in the Indian market. | India 1999–2005 | Standard Deviation | A very high degree of diversification is possible in India; a portfolio size of 10–15 stocks is considered appropriate as the risk reduction is marginal thereafter. | 10–15 stocks |
( ) | Evaluate diversification benefits and optimal bond portfolio sizes (PS) for investment opportunity (IO) sets differentiated by issuer type, credit ratings, and term-to-maturity. | U.S. 1985–1997 | Correlations, Skewness, Kurtosis | Minimum PSs vary not only by issuer type, term-to-maturity, and bond rating but also by the metric used to measure the marginal benefits of further diversification. | 25–40 bonds |
( ) | To find out whether minimum portfolio sizes should be prescribed to achieve sufficiently well-diversified equity portfolios. | Canada 1975–2003 | Correlations, Mean Derived Dispersion, Mean Realized Dispersion, Normalized Portfolio Variance, Skewness, Kurtosis | Minimum sizes for a fixed investment opportunity set differ both within and across categories of metrics used to measure diversification benefits. | Depends upon different factors |
( ) | Examine the reduction in time series risk, as measured by the standard deviation of the time series, and cross-sectional risk, as measured by the standard deviation of terminal wealth. | U.S. 1980–2000 | Equally Weighted and Market Weighted Portfolios, Standard Deviation, Terminal Wealth Standard Deviation | Regardless of how risk is measured or how a portfolio is constructed, a randomly selected portfolio of about 40 to 50 stocks can be considered well diversified. | 40–50 stocks |
( ) | Analyze whether the theory of risk reduction through portfolio diversification applies to the Karachi Stock Exchange. | Pakistan 2007–2009 | Standard Deviation | The theory of portfolio diversification applies to Karachi Stock Exchange: a 52.25% reduction in risk was achieved. | 10 stocks |
( ) | Evaluate the diversification opportunities when portfolios consist of differentially weighted stocks and compare the diversification effect of naive and differentially weighted stock portfolios. | Lithuania 2009–2010 | Standard Deviation, HHI index | A greater diversification effect is obtained in naive portfolios. | 22 stocks for equally-weighted portfolios and 25 stocks for differently-weighted portfolios |
( ) | Estimating confidence bands around two central measures to provide portfolio size recommendations that achieve the most diversification benefits 90% of the time, rather than on average. | U.S., UK, Japan, Canada and Australia 1975–2011 | Standard Deviation, Terminal Wealth Standard Deviation, MAD, ES, LMP, Skewness, Kurtosis | The correlation structures in the five markets change in times of financial market crisis, and these changes differ in times of market-wide crises and industry-specific meltdowns. | Influenced by different factors |
( ) | Analyze whether the MDI might prove a useful tool for practitioners seeking to improve portfolio diversification within a smaller and therefore more manageable subset of assets. | U.S. 2006–2011 | Portfolio Diversification Index (PDI), Maximum Diversification Index (MDI) | The MDI can be efficiently implemented to narrow down a large set of investable assets by eliminating those issues that do not improve the diversification characteristics of the underlying portfolio pool. | 90–99 stocks |
( ) | Study the effect of the number of holdings in a portfolio on the properties of the portfolio. | U.S. 2003–2010 | Standard Deviation, Downside Risk, Sharpe Ratio, Beta, Correlations | Massive portfolios are not necessary to achieve diversified portfolios. | 31 stocks on average |
( ) | Analyze the specific relationship between portfolio size and risk reduction. | U.S. 2008–2013 | Standard Deviation, Regression, T-test, F-test | There is a strong relationship between portfolio size and risk, and this relationship could be captured by a decreasing asymptotic function. The results of the analysis using modern stock data are consistent with the result of the analysis using securities data from the 1950s and 1960s. | 10 stocks |
( ) | Determine the number of stocks in a portfolio required to minimize diversifiable risk for Canadian institutional investors using various risk measures, including those that take into account black swan events. | Canada 1975–2011 | Heavy-Tailed Risk, Expected Shortfall, Time Series Standard Deviation, Terminal Wealth Standard Deviation | The recommended number of stocks is influenced by market conditions as well as the average correlations between stocks in the Canadian market. | More than 50 stocks |
( ) | To determine the optimal number of stocks that an active fund manager should hold in a portfolio of stocks in Asia and a portfolio of stocks in the domestic Chinese stock market. | China, India, Korea, Taiwan, Hong-Kong, Malaysia, Singapore, Thailand, Indonesia, Philippines 2003–2013 | Standard Deviation, Risk-Adjusted Return Measure | Adding additional stocks put more downward pressure on returns than in Asia, and market risk in China was almost twice as high as in Asia. | 10 (Asia) and 8 stocks (China) |
( ) | To analyze how the use of higher frequency data affects the recommendations for the number of shares required to reduce risk to a given level. | U.S. 2003–2011 | Unconditional Correlation, Standard Deviation | Investors may not need to hold as large portfolios as lower frequency risk measures suggest, especially during financial crises. During the crisis, the correlation between the best-performing stocks increased more than that between the worst-performing stocks. | 7 (10) stocks |
( ) | Analysis of the impact of the GFC on the optimal number of stocks, and of whether this number differs between better-performing countries and PIIGS or between different sectors. | Europe 2000–2014 | Standard Deviation | For five better-performing countries, the optimal required number of stocks in a portfolio was higher than for the PIIGS countries; the number of stocks is significantly affected by the financial crisis. | Depends on the observed period, sector, as well as on the economic development of the country itself |
( ) | Investigating the optimal portfolio size for an investor at Nairobi Securities Exchange. | Kenya 2009–2013 | Mean-variance Optimization Model, Standard Deviation | Portfolio risk decreased as the number of securities in the portfolio increased, but beyond the optimal portfolio size, risk began to increase again. | 18–22 stocks |
( ) | To analyze the number of stocks that should be held in concentrated markets in South Africa. | South Africa 2002–2014 | Standard Deviation, 4 Different Weighting Schemes | Equally-weighted portfolios require far fewer stocks for effective risk reduction because of the concentrated stock environment in South Africa. | 15–19 for equally-weighted portfolios and 33–60 for SWIX-weighting scheme |
( ) | To explain why the optimal number of stocks in a portfolio is hard to find, the relationship between portfolio diversification and concentration studied using a genetic algorithm. | U.S. 2005–2013 | Portfolio Diversification Index (PDI), HHI index, Variance | For a given universe, there is a set of Pareto-optimal portfolios with a different number of stocks that simultaneously maximizes diversification and minimizes concentration. | 30–40 stocks |
( ) | Determine the number of stocks required to form a well-diversified portfolio in Pakistan, as each investor has limited funds to invest in securities. | Pakistan 2009–2015 | Variance, Correlation, Kurtosis, Skewness | The data are normally distributed and the average return on most securities is positive. | 20 stocks |
( ) | The study focuses on finding an optimal portfolio using Sharpe’s single index model. | India 2012–2016 | Sharpe Single Index Model, Beta, Variance | Only two stocks, namely Vedanta and Tata Steel, are included in the Optimal Portfolio constructed in this study, suggesting a maximum investment of 86% in Vedanta and 14 % in Tata Steel. | 14 stocks |
( ) | Two different bases are used to determine the significant number of stocks required for well-diversified portfolio risk. | Malaysia 2010–2014 | Standard Deviation, Covariance, Correlation | Data frequency affects the number of stocks in a portfolio required to achieve optimal diversification. | 45 stocks (daily basis) 35 stocks (weekly basis) |
( ) | Evaluate the impact of extreme market shifts on equity portfolios and analyze the difference between negative and positive responses to market jumps with implications for portfolio risk management. | U.S. 2003–2017 | Beta, Regression (OLS) | The number of holdings required to stabilize portfolio sensitivity to negative jumps is higher than for positive jumps, and the asymmetry is more pronounced for more extreme events. | 35 (if the asymmetry is ignored) or 54 stocks |
( ) | To examine the factors that determine diversification effects in a real continuous time, as opposed to a thoroughly studied theoretical single period timespan. | U.S. 1973–2018 | Risk Premium Ratio, Gross Compound Excess Wealth Ratio, Shannon Limit, Kelly Criterion, Sharpe Ratio, Variance | These factors are the number of stocks in the benchmark, the Sharpe ratio and variance of the benchmark, the idiosyncratic variance of an average stock, the investment proportion, and time. | 20, 40, or 200 stocks for a short-term risk-neutral investor |
( ) | Investigate different approaches for dividing total portfolio risk into a diversifiable and a non-diversifiable component. | U.S. 2007–2016 | Naive Diversification, Portfolio Risk Decomposition, OLS, Variance | Simulation analysis shows that diversifiable risk cannot be easily diversified away. Regardless of the model used, the dispersion of the cross-sectional distributions of diversifiable risk is not negligible for portfolio sizes up to and including 300 stocks. | 300 stocks |
( ) | To investigate whether it is theoretically possible to construct fully risk-diversified portfolios, even for small numbers of not-so-wealthy individuals. | U.S., UK, Germany, France, Canada and Japan 2008–2019 | Mean-variance Optimization model | Constructing fully risk-diversified portfolios requires more money when the required rate of return is lower; diversification is most expensive in the United Kingdom. | $10,000–$100,000 |
( ) | To examine how many stocks make up a well-diversified portfolio of Indian stocks and to propose a practical heuristic that investors can use to evaluate the number of stocks in their portfolios. | India 2014–2020 | Standard Deviation | The actual number of stocks would depend on the investor’s preferences in terms of risk-reduction preferences, the desired confidence level to meet his objectives, and the weighting scheme used for portfolio construction. | 40–50 stocks |
MDPI stays neutral with regard to jurisdictional claims in published maps and institutional affiliations. |
Share and Cite
Zaimovic, A.; Omanovic, A.; Arnaut-Berilo, A. How Many Stocks Are Sufficient for Equity Portfolio Diversification? A Review of the Literature. J. Risk Financial Manag. 2021 , 14 , 551. https://doi.org/10.3390/jrfm14110551
Zaimovic A, Omanovic A, Arnaut-Berilo A. How Many Stocks Are Sufficient for Equity Portfolio Diversification? A Review of the Literature. Journal of Risk and Financial Management . 2021; 14(11):551. https://doi.org/10.3390/jrfm14110551
Zaimovic, Azra, Adna Omanovic, and Almira Arnaut-Berilo. 2021. "How Many Stocks Are Sufficient for Equity Portfolio Diversification? A Review of the Literature" Journal of Risk and Financial Management 14, no. 11: 551. https://doi.org/10.3390/jrfm14110551
Article Metrics
Article access statistics, further information, mdpi initiatives, follow mdpi.
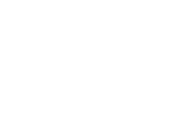
Subscribe to receive issue release notifications and newsletters from MDPI journals
Measuring the Benefits of Diversification Across Portfolios
24 Pages Posted: 29 Mar 2021 Last revised: 5 Aug 2021
Dilip B. Madan
University of Maryland - Robert H. Smith School of Business
Morgan Stanley
Date Written: March 26, 2021
A portfolio diversification index is defined as the ratio of an equivalent number of independent assets to the number of assets. The equivalence is based on either attaining the same diversification benefit or spread reduction. The diversification benefit is the difference in value of a value maximizing portfolio and the maximum value of the components. The spread reduction is the percentage reduction attained by a spread minimizing portfolio relative to the smallest spread for the components. Asset values, bid and ask, are given by conservative valuation operators from the theory of two price economies. The diversification indices fall with the number of assets in the portfolio and they are explained by a measure of concentration applied to normalized eigenvalues of the correlation matrix along with the average level of correlation. A time series of the indices constructed on the basis of the S&P 500 index and the nine sector ETF's reveals a collapse during the final crisis with no recovery until 2016, peaking in February 2020 and a COVID crash in March of 2020. Furthermore factor diversification possibilities are richer than those found in equity indices. Diversification benefits across global indices are not as strong as diversification across an equal number of domestic assets, but they rise substantially for longer horizons of up to three years.
Keywords: Bilateral Gamma, Acceptable Risks, Distorted Expectations.
JEL Classification: G10, G11, G12.
Suggested Citation: Suggested Citation
Dilip B. Madan (Contact Author)
University of maryland - robert h. smith school of business ( email ).
College Park, MD 20742-1815 United States 301-405-2127 (Phone) 301-314-9157 (Fax)
Morgan Stanley ( email )
1585 Broadway New York, NY 10036 United States
Do you have a job opening that you would like to promote on SSRN?
Paper statistics, related ejournals, stochastic models ejournal.
Subscribe to this fee journal for more curated articles on this topic
Econometric Modeling: Capital Markets - Portfolio Theory eJournal
Econometric modeling: capital markets - risk ejournal, investing ejournal.
Academia.edu no longer supports Internet Explorer.
To browse Academia.edu and the wider internet faster and more securely, please take a few seconds to upgrade your browser .
- We're Hiring!
- Help Center
Portfolio Diversification
- Most Cited Papers
- Most Downloaded Papers
- Newest Papers
- Last »
- Equity Markets Integration Follow Following
- Balkans Region Follow Following
- International Stock Markets Follow Following
- Finace Follow Following
- Portfolio Optimization Follow Following
- Optimization (Mathematical Programming) Follow Following
- Risk Management and Insurance Follow Following
- Study on pricing behavior in Indian equity market Follow Following
- Emerging Markets Follow Following
- International Finance Follow Following
Enter the email address you signed up with and we'll email you a reset link.
- Academia.edu Journals
- We're Hiring!
- Help Center
- Find new research papers in:
- Health Sciences
- Earth Sciences
- Cognitive Science
- Mathematics
- Computer Science
- Academia ©2024
Portfolio selection: from under-diversification to concentration
- Published: 12 September 2022
- Volume 64 , pages 1539–1557, ( 2023 )
Cite this article
- Jiawen Xu 1 ,
- Yixuan Li 2 , 3 ,
- Kai Liu 3 , 4 &
- Tao Chen ORCID: orcid.org/0000-0003-2987-6711 2 , 3 , 5
572 Accesses
Explore all metrics
The two opposing investment strategies, diversification and concentration, have often been directly compared. While there is much less dispute regarding Markowitz’s approach as the benchmark for diversification, the precise meaning of concentration in portfolio selection remains unclear. This paper offers a novel definition of concentration, along with an extreme value theory-based estimator for its implementation. When overlaying the performances derived from diversification (in Markowitz’s sense) and concentration (in our definition), we find an implied risk threshold, at which the two polar investment strategies reconcile—diversification has a higher expected return in situations where risk is below the threshold, while concentration becomes the preferred strategy when the risk exceeds the threshold. Different from the conventional concave shape, the estimated frontier resembles the shape of a seagull, which is piecewise concave. Further, taking the equity premium puzzle as an example, we demonstrate how the family of frontiers nested inbetween the estimated curves can provide new perspectives for research involving market portfolios.
This is a preview of subscription content, log in via an institution to check access.
Access this article
Subscribe and save.
- Get 10 units per month
- Download Article/Chapter or eBook
- 1 Unit = 1 Article or 1 Chapter
- Cancel anytime
Price includes VAT (Russian Federation)
Instant access to the full article PDF.
Rent this article via DeepDyve
Institutional subscriptions
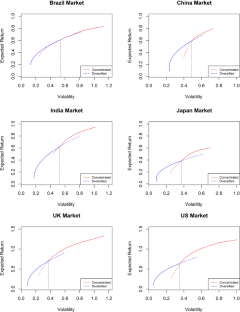
Similar content being viewed by others
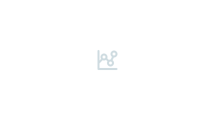
Diversification and portfolio theory: a review
Portfolio Optimization Using Modified Herfindahl Constraint
Harry markowitz, the cowles commission, and portfolio theory.
A concise way of saying the estimated efficient frontier implied by Markowitz’s mean-variance optimization method.
The Brazil stock pool includes all stocks currently listed at the B3 Stock Exchange. The China stock pool includes all stocks currently listed at the Shanghai and the Shenzhen Stock Exchanges. The India stock pool includes all stocks currently listed at the Bombay Stock Exchange and the National Stock Exchange of India. The Japanese stock pool includes all stocks currently listed at the Tokyo Stock Exchange. The US stock pool includes all stocks currently listed at the New York Stock Exchange and the NASDAQ Stock Market. The UK stock pool includes all stocks currently listed at the London Stock Exchange.
The method to estimate the concentrated market frontier is nonparametric, which is generally known for boundary bias. This is the reason we follow the common practice in nonparametric estimation by trimming the observations close to the boundaries. To ensure comparability, both frontiers are estimated based on the same trimmed data set.
Andersen TG, Bollerslev T (1998) Answering the skeptics: Yes, standard volatility models do provide accurate forecasts. Int Econ Rev 885–905
Bali TG, Peng L (2006) Is there a risk-return trade-off? Evidence from high-frequency data, Journal of Applied Econometrics 21:1169–1198
Google Scholar
Bird R, Pellizzari P, Yeung D, Woolley P, et al (2012) The strategic implementation of an investment process in a funds management firm. Technical report
Blume ME, Friend I (1975) The asset structure of individual portfolios and some implications for utility functions. The Journal of Finance 30:585–603
Article Google Scholar
Brands S, Brown SJ, Gallagher DR (2005) Portfolio concentration and investment manager performance. International Review of Finance 5:149–174
Buffett W (1994) Letter to shareholders, Berkshire Hathaway Annual Report
Buser SA (1977) Mean-variance portfolio selection with either a singular or nonsingular variance-covariance matrix. Journal of Financial and Quantitative Analysis 12:347–361
Campbell JY (2018) Financial Decisions and Market - A Course in Asset Pricing. Princeton
Campbell R, Huisman R, Koedijk K (2001) Optimal portfolio selection in a Value-at-Risk framework. Journal of Banking & Finance 25:1789–1804
Caselli F, Ventura J (2000) A representative consumer theory of distribution. The American Economic Review 90:909–926
Chen T, Yang F (2020) Think outside the envelop—efficiency bound estimation through extreme value theory, Working Paper
Choi N, Fedenia M, Skiba H, Sokolyk T (2017) Portfolio concentration and performance of institutional investors worldwide. Journal of Financial Economics 123:189–208
Daniels MJ, Kass RE (1999) Nonconjugate Bayesian estimation of covariance matrices and its use in hierarchical models. Journal of the American Statistical Association 94:1254–1263
Dekkers AL, Einmahl JH, De Haan L et al (1989) A moment estimator for the index of an extreme-value distribution. The Annals of Statistics 17:1833–1855
Dietrich, D., L. Haan, and J. Hüsler (2002): Testing extreme value conditions, Extremes, 5, 71–85.
Ekholm A, Maury B (2014) Portfolio concentration and firm performance. Journal of Financial and Quantitative Analysis 49:903–931
Evans JL, Archer SH (1968) Diversification and the reduction of dispersion: An empirical analysis. J Finance 23:761–767
Fang C-R, You S-Y (2014) “The impact of oil price shocks on the large emerging countries’ stock prices: Evidence from China. India and Russia”, International Review of Economics 29:330–338.
Feibel BJ (2003) Investment performance measurement, Vol 116, Wiley
Fisher L, Lorie JH (1970) Some studies of variability of returns on investments in common stocks. The Journal of Business 43:99–134
Floros C (2005) Price linkages between the US, Japan and UK stock markets. Financial Markets and Portfolio Management 19:169–178
Gay Jr RD et al (2008) Effect of macroeconomic variables on stock market returns for four emerging economies: Brazil, Russia, India, and China. Int Business Econ Res J (IBER), 7
Ghysels E, Santa-Clara P, Valkanov R (2005) There is a risk-return trade-off after all. Journal of Financial Economics 76:509–548
Goetzmann WN, Kumar A (2008) Equity portfolio diversification. Review of Finance 12:433–463
Goetzmann WN, Li L, Rouwenhorst KG et al (2005) Long-term global market correlations. The Journal of Business 78:1–38
Goldman E, Sun Z, Zhou X (2016) The effect of management design on the portfolio concentration and performance of mutual funds. Financial Analysts Journal 72:49–61
Hamao Y, Masulis RW, Ng V (1990) Correlations in price changes and volatility across international stock markets. The Review of Financial Studies 3:281–307
Ivković Z, Sialm C, Weisbenner S (2008) Portfolio concentration and the performance of individual investors. Journal of Financial and Quantitative Analysis 43:613–655
Jenkinson AF (1955) The frequency distribution of the annual maximum (or minimum) values of meteorological elements. Quarterly Journal of the Royal Meteorological Society 81:158–171
Jorion P (2010) Financial risk manager handbook. Wiley
Kacperczyk M, Sialm C, Zheng L (2005) On the industry concentration of actively managed equity mutual funds. The Journal of Finance 60:1983–2011
Kent, J. T. (1983): Information gain and a general measure of correlation, Biometrika, 70, 163–173.
Keynes JM, Moggridge DE, Johnson ES et al (1983) The collected writings of John Maynard Keynes, vol. XII, Macmillan, London
Ledoit O, Wolf M (2003) Improved estimation of the covariance matrix of stock returns with an application to portfolio selection. Journal of Empirical Finance 10:603–621
Ledoit O, Wolf M (2004) Honey, I shrunk the sample covariance matrix, The. J Portfolio Manag 30:110–119
Ledoit O, Wolf M (2012) Nonlinear shrinkage estimation of large-dimensional covariance matrices. The Annals of Statistics 40:1024–1060
Ledoit O, Wolf M (2017) Nonlinear shrinkage of the covariance matrix for portfolio selection: Markowitz meets Goldilocks. The Review of Financial Studies 30:4349–4388
Leonard T, Hsu JS et al (1992) Bayesian inference for a covariance matrix. The Annals of Statistics 20:1669–1696
Liu EX (2016) Portfolio diversification and international corporate bonds. Journal of Financial and Quantitative Analysis 51:959–983
Liu K (2017) Effective dimensionality control in quantitative finance and insurance, PhD thesis, University of Waterloo
Loeb GM (2007) Battle for investment survival, Vol 36, Wiley
Longin F (2016) Extreme events in finance: a handbook of extreme value theory and its applications. Wiley
Longin FM (2000) From Value-at-Risk to stress testing: The extreme value approach. Journal of Banking & Finance 24:1097–1130
Madaleno M, Pinho C (2012) International stock market indices comovements: A new look. International Journal of Finance & Economics 17:89–102
Markowitz H (1952) Portfolio selection, The. Journal of Finance 7:77–91
McNeil AJ, Frey R (2000) Estimation of tail-related risk measures for heteroscedastic financial time series: An extreme value approach. Journal of Empirical Finance 7:271–300
Mehra R (2008) Handbook of the equity risk premium. Elsevier
Mehra R, Prescott EC (1985) The equity premium: A puzzle. Journal of Monetary Economics 15:145–161
Merton R (1972) An analytic derivation of the efficient portfolio frontier. Journal of Financial and Quantitative Analysis 7:1851–1872
Mises RV (1954) La distribution de la plus grande de n valeurs. American Mathematical Society, Providence. RI. II:271–294
Modigliani F, Leah M (1997) Risk-adjusted performance. Journal of Portfolio Management 23:45–54
Oyenubi A (2019) Diversification measures and the optimal number of stocks in a portfolio: An information theoretic explanation. Computational Economics 54:1443–1471
Pappas D, Kiriakopoulos K, Kaimakamis G (2010) Optimal portfolio selection with singular covariance matrix. International Mathematical Forum 5:2305–2318
Pownall RA, Koedijk KG (1999) Capturing downside risk in financial markets: The case of the Asian Crisis. J Int Money Finance 18:853–870
Rocco M (2014) Extreme value theory in finance: A survey. Journal of Economic Surveys 28:82–108
Saranya K, Prasanna PK (2014) Portfolio selection and optimization with higher moments: evidence from the Indian stock market. Asia Pac FinanMarkets 21:133–149
Sharkasi A, Crane M, Ruskin HJ, Matos JA (2006) The reaction of stock markets to crashes and events: A comparison study between emerging and mature markets using wavelet transforms. Physica A: Statistical Mechanics and its Applications 368:511–521
Statman M (1987) How many stocks make a diversified portfolio? Journal of Financial and Quantitative Analysis 22:353–363
Yang R, Berger JO (1994) Estimation of a covariance matrix using the reference prior. Ann Statist 1195–1211
Yeung D, Pellizzari P, Bird R, Abidin S, et al (2012) Diversification versus concentration... and the Winner is? Technical report
Download references
Acknowledgements
The corresponding author would like to thank Francisco Gonzalez and Tony Wirjanto for multiple insightful discussions of this topic.
Author information
Authors and affiliations.
School of Economics, Shanghai University of Finance and Economics, Shanghai, China
Department of Economics, University of Waterloo, Waterloo, ON, Canada
Yixuan Li & Tao Chen
Big Data Research Lab, University of Waterloo, Waterloo, ON, Canada
Yixuan Li, Kai Liu & Tao Chen
School of Mathematical and Computational Sciences, University of Prince Edward Island, Charlottetown, PEI, Canada
Senior Research Fellowship of Labor and Worklife Program, Harvard University, Cambridge, MA, USA
You can also search for this author in PubMed Google Scholar
Contributions
Not applicable
Corresponding author
Correspondence to Tao Chen .
Ethics declarations
The authors did not receive support from any organization for the submitted work.
Conflict of interest
The authors have no relevant financial or nonfinancial interests to disclose.
Ethics approval
Not applicable.
Consent to participate
Consent for publication, availability of data and materials.
The data that support the findings of this study are available from the corresponding author upon request.
Code availability
The code that support the findings of this study are available from the corresponding author upon reasonable request.
Additional information
Publisher's note.
Springer Nature remains neutral with regard to jurisdictional claims in published maps and institutional affiliations.
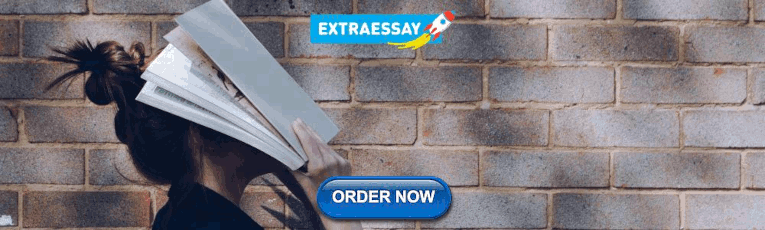
Appendix A: Derivations of the statistical methods
1.1 a.1: the mean-variance optimization.
This section provides the general setup of Markowitz’s mean-variance optimization. Let \(\varvec{E}\) be the vector of expected asset returns in the stock pool, \(\varvec{\mathrm {V}}\) be the covariance matrix of the returns, and \(\varvec{w}\) be the vector of weights indicating the fraction of portfolio wealth held in each asset. Assuming that short sales are permitted, the constrained minimization problem is as follows:
where \(\mu \) denotes the target expected return of the portfolio, and \(\varvec{1}\) denotes a vector of ones. The analytical solution to this problem is derived following Merton ( 1972 ), which we will not expand on here.
1.2 A.2: The DR method
We elaborate on the DR method first introduced by Liu ( 2017 ), which effectively reduces the number of stocks, while still preserves the variance in the market. Suppose that there are N assets with asset prices \(S^{(1)},S^{(2)},...,S^{(N)}\) in the market. Based on the multivariate Black-Scholes model, the asset price processes \(\left\{ S_t^{(h)}\right\} \) for \(h = 1,2,...,N\) solves the stochastic differential equation
where \(B_t^{(1)},B_t^{(2)},...,B_t^{(N)}\) follow the independent standard Brownian motions, \(r_t\) is the short rate of interest, and \([\sigma _{hl}]\) is the matrix capturing the correlation among the assets. Then, the solution to Equation ( 1 ) is
Let \(t_0=0\) , \(t_1 = \Delta ,...,t_m=m\Delta \) be the time steps with equal space \(\Delta \) , and suppose that the continuous forward rate is constant within each period. We denote \(f_j\) as the annualized continuous forward rate for period \(\left( t_{j-1},t_j\right) \) such that
Then, we have
For \(j=1,2,...,m\) , let \(A_j^{(h)}\) be the accumulation factor of the \(h^{th}\) index for the period \((t_{j-1},t_j)\) , that is,
Combining Equation ( 2 ) to ( 4 ), we get
By the property of Brownian motion, we know that \(Z_1^{(l)},Z_2^{(l)},...,Z_m^{(l)}\) are independent random variables with a standard normal distribution. From Equation ( 5 ), we derive the continuous return for the period \((t_{j-1},t_j)\)
The mean and covariance matrix of the returns are given by
Let \(\Sigma \) be the covariance matrix of the annualized continuous returns of the N stocks and
be the Cholesky decomposition of \(\Sigma \) such that
where \({\mathbf {A}}^\intercal \) is the transpose of \({\mathbf {A}}\) . Then, the variance contribution, also known as the explained variance (e.g., Kent 1983 ), of the first \(N_{DR}\) assets with the highest Sharpe ratios can be defined as
where \(A_i\) is the \(i^{th}\) column of \({\mathbf {A}}\) . In this paper, the reduced dimensionality \(N_{DR}\) is the minimum number of assets needed to reach the \(95\%\) explained variance, and the dimensionality reduction is achieved when \(N_{DR}<<N\) .
1.3 A.3: The EVT method
In this section, we discuss the EVT method in further details. We take one stock market, say the China market, as an example and exclude all non-positive returns since our concern is the right-tail return. Let \(X_{1},X_{2},...,X_{n}\) denote the observations of returns in one group, say G1. We consider these n returns as i . i . d . observations from some distribution function F . Let \(X_{1,n} \le X_{2,n} \le ... \le X_{n,n}\) be the associated order returns, so that \(X_{n,n}\) denotes the maximum return in G1. Then, according to Mises ( 1954 ) and Jenkinson ( 1955 ), if the maximum \(X_{n,n}\) , suitably centered and scaled, converges to a non-degenerate random variable, then there exist sequences \(\{a_n\}\) \((a_n > 0)\) and \({b_n}\) \((b_n \in {\mathbb {R}})\) such that
for some \(\gamma \in {\mathbb {R}}\) , with x such that \(1+\gamma x > 0\) . That is, F is in the domain of attraction of some extreme value distribution function \(G_\gamma \) and \(\gamma \) is the extreme-value index. By taking logarithms, Equation ( 6 ) can be written as
where \(q \in {\mathbb {R}}^+\) and \(a_q\) and \(b_q\) are defined by interpolation. We take \(b_q = U(q)\) with
where \(-1\) denotes the left-continuous inverse.
We then estimate \(\gamma \) , \(a_q\) and \(b_q\) as follows. Let, for \(1 \le k < n\) ,
We use the moment estimators for \(\gamma \in {\mathbb {R}}\) introduced by Dekkers et al. ( 1989 ):
Specifically, we first test that \(\gamma \) exists for all groups according to Dietrich et al. ( 2002 ). Next, we plot \(\hat{\gamma }\) as a function of k , which is the number of upper order statistics used for estimation minus 1. Then, we determine the first stable region in k of the estimate from the moment estimator plot. Namely, we try to identify a set of consecutive values of k where the estimated values do not fluctuate much, so that the procedure is insensitive to the choice of k in such a region. For the moment estimator in G1 for the China market, as illustrated in Fig. 9 , such a stable region runs from around \(k = 30\) to \(k = 200\) .
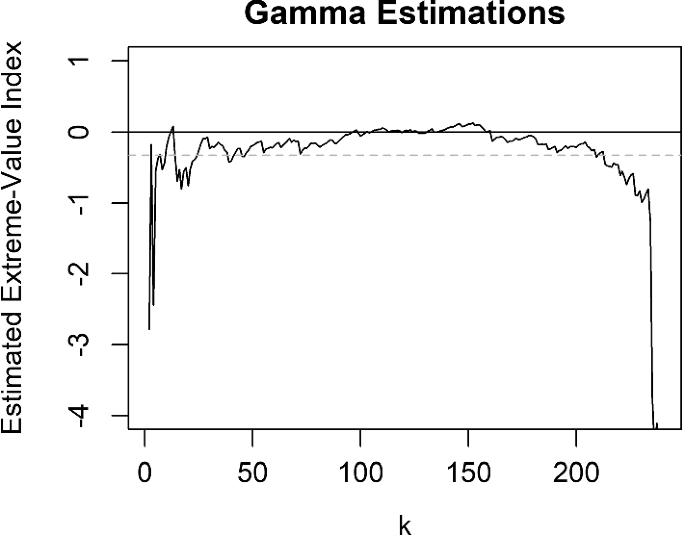
Moment estimator versus k for G1 of the China Market
Next, we define the following estimators for \(a_n/k\) and \(b_n/k\) :
Then, our goal is to estimate the right endpoint
of the distribution function F , that is, the ultimate return of G1 based on the observed returns. When estimating the endpoint, we assume that \(\gamma < 0\) . Next, it can be shown that Equation ( 6 ) is equivalent to
As t gets large, we can write
Because \(\gamma <0\) this yields, for large x and setting \(q=n/k\) ,
Therefore, \(x^*\) can be estimated as
where \(\hat{\gamma } <0\) , and \(\hat{x}^*:=\infty \) otherwise. The endpoint estimate of G1 for the China is shown in Fig. 10 , and the selected estimate is the dotted horizontal line.
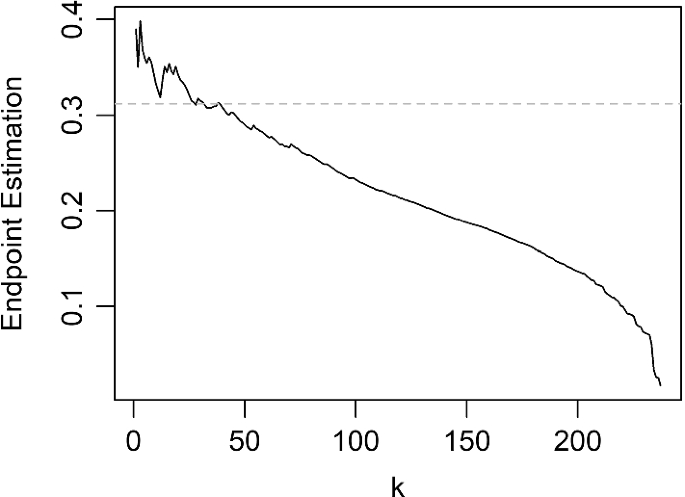
Endpoint estimators versus k for G1 of the China Market
Rights and permissions
Springer Nature or its licensor holds exclusive rights to this article under a publishing agreement with the author(s) or other rightsholder(s); author self-archiving of the accepted manuscript version of this article is solely governed by the terms of such publishing agreement and applicable law.
Reprints and permissions
About this article
Xu, J., Li, Y., Liu, K. et al. Portfolio selection: from under-diversification to concentration. Empir Econ 64 , 1539–1557 (2023). https://doi.org/10.1007/s00181-022-02300-x
Download citation
Received : 24 August 2021
Accepted : 18 August 2022
Published : 12 September 2022
Issue Date : April 2023
DOI : https://doi.org/10.1007/s00181-022-02300-x
Share this article
Anyone you share the following link with will be able to read this content:
Sorry, a shareable link is not currently available for this article.
Provided by the Springer Nature SharedIt content-sharing initiative
- Diversification and concentration
- Efficient frontier
- Market portfolio
- Extreme value theory
- Dimensional reduction
JEL Classification
- Find a journal
- Publish with us
- Track your research
- Open access
- Published: 16 June 2023
Portfolio diversification benefits before and during the times of COVID-19: evidence from USA
- Eman F. Attia ORCID: orcid.org/0000-0002-2006-0823 1 , 2 ,
- Sharihan Mohamed Aly 1 ,
- Ahmed said ElRawas 3 &
- Ebtehal Orabi Awad 4
Future Business Journal volume 9 , Article number: 26 ( 2023 ) Cite this article
3478 Accesses
1 Citations
Metrics details
This paper investigates the portfolio diversification benefits for Islamic and conventional investors in the USA with its major trading partners (United Kingdom, Canada, China, Japan, Malaysia, and Turkey) before and during the COVID-19 crisis period. Using daily data from 2007 to 2020, we employ three relevant time-varying and timescale-dependent techniques, the continuous wavelet transform (CWT) analysis, the wavelet multiple correlation (WMC), and the wavelet multiple cross-correlation (WMCC). The findings suggest that conventional and Islamic US investors who invest with major trading partners may reap large diversification benefits for very short investment horizons (4–8), except for Sharia Malaysia index. However, they may not reap benefits for investment horizons of 8–16 and longer, except for China. In addition, COVID-19 crisis caused a poor diversification opportunity for US investors regardless of the regime they follow (conventional or Islamic). Moreover, the American industrial market depicts a state of impending perfect market integration. Finally, the UK and Canada seem to be the potential market leaders in different wavelet scales. These findings yield important policy implications.
Introduction
The international financial sector has been struck by a new event that has amply affected the portfolio investment business. The spread of the COVID-19 pandemic has generated to a real economic slump in the world. Faced with this perilous situation, most governments have imposed quarantines, containment measures, closures of restaurants, factories, educational institutions, stores, travel bans, deferral of dues and taxes, resilience of banks to contain the virus. This has undeniably blocked most of the commercial activities of the affected countries. The COVID-19 pandemic also caused a real significantly deteriorates of international financial markets. Specifically, it caused high volatility in the US stock market compared to other epidemics of contagious diseases, including notably the Spanish flu. [ 1 , 2 ].
The COVID-19 pandemic has caused significant disruptions in various industries, leading to market volatility and significant losses for investors. As a result, many investors are reevaluating their investment strategies and seeking ways to mitigate risk. One strategy for mitigating risk is portfolio diversification, which involves investing in a variety of assets to reduce the impact of market fluctuations on the overall portfolio. However, the effectiveness of portfolio diversification during the COVID-19 pandemic remains unclear. Therefore, investigating the impact of the pandemic on portfolio diversification and its effectiveness in mitigating risk is a crucial area of research. The paper can contribute to the literature by examining the impact of COVID-19 on portfolio diversification strategies and the effectiveness of various diversification techniques in reducing the impact of the pandemic on investment portfolios. The study can also provide insights into the behavior of different asset classes during the pandemic, enabling investors to make informed decisions on their investment strategies.
Specifically, we analyze the benefits of portfolio diversification before and during the COVID-19 period. Portfolio optimization issues have been at the center of a theoretical and empirical debate in international financial markets since the 1950s. This debate is based on the implementation of an optimal portfolio strategy, which requires the trade-off between maximizing investment returns and minimizing investment risk [ 3 ]. Several factors have contributed significantly to the dramatic evolution of international stock markets. International investors should be vigilant to the increasing integration that is happening between the different stock markets of the world. They are then required to have diversified securities or a portfolio, thus supporting risk reduction [ 4 ]. The excessive interconnectedness between stock markets is caused by the presence of high correlations between stock markets in portfolio diversification theories. Therefore, this is likely to prevent investors from taking advantage of diversification benefits. As a result, maximizing portfolio diversification benefits requires the presence of low correlations in price behavior. However, successful diversification strategies require more than investors and portfolio managers considering the interdependence of international stock markets. It requires the consideration of different investment horizons throughout the investment period. Indeed, the variation in market returns is not exclusively viewed over time. It is also perceived in terms of time scales attached to different investment horizons [ 5 ].
According to the portfolio management literature, conventional finance aims at optimizing the risk-return trade-off of an investment. However, in Islamic finance, profitability is not seen as the only and primary objective of Islamic principles. In classical Islamic jurisprudence, based on the principles of the Sharia , financial transactions are aimed at improving global economic efficiency, while requiring strict screening of commercial and financial activities [ 6 ]. Footnote 1 Several empirical studies have shown that Islamic stock indices, in some emerging countries are less risky and partially protected against financial crashes, thus constituting a viable alternative source, for investors who wish to benefit from the diversification advantages (see among others, [ 7 , 8 , 9 , 10 ].
Our contribution in this paper focuses on two key areas. Firstly, we analyze the effectiveness of portfolio diversification for US investors (Islamic and conventional) and their trading partners during the COVID-19 pandemic using the continuous wavelet transform (CWT) analysis to specify the degree of correlation with both time and frequency changing. Specifically, we examine the impact of the pandemic on various asset classes and assess the effectiveness of diversification strategies in mitigating risk. Secondly, we introduce the use of wavelet multiple correlation (WMC) to measure the degree of integration between different asset classes, providing a more comprehensive analysis of the relationships between assets. Additionally, we use wavelet multiple cross-correlation (WMCC) to identify the market leader among different asset classes, enabling investors to make informed decisions on their investment strategies. Overall, our contribution offers new insights into the effectiveness of portfolio diversification during the COVID-19 pandemic and provides a more robust analysis of the relationships between different asset classes, enabling investors to make more informed decisions. To do so, we use the Dow Jones conventional and Islamic indices as a proxy for the US stock index. Our results show that conventional and Islamic US investors who invest with major trading partners may reap large diversification benefits for very short investment horizons (4–8), except for Sharia Malaysia index. However, they may not reap benefits for investment horizons of 8–16 and longer, except for China. Specifically, COVID-19 crisis caused a poor diversification opportunity for US investors regardless of the regime they follow (conventional or Islamic). Moreover, the American industrial market illustrates an expected state of full market integration. Finally, the UK and Canada seem to be the potential market leaders in different wavelet scales.
In sum up, previous studies have provided important information on the portfolio diversification issue. Therefore, our paper aims to answer three main questions: first, does geographic diversification benefit US investors? Second, how does the COVID-19 crisis affect portfolio diversification opportunities for US investors? Third, how integrated is the US stock market and who is the US industrial market leader?
The rest of this document is structured as follows. Section " Literature review " is devoted to the presentation of the literature review. Section " Methodology " discusses the research methodology. The empirical results are presented in Sect. " Data and empirical results ". Section " Conclusions and implications " concludes with some remarks and recommendations.
Literature review
Theoretically, the portfolio diversification issue is often explained by two main theories: (i) modern portfolio theory and (ii) efficient market hypothesis. Under modern portfolio theory, each security carries its own risk. A portfolio composed of different securities with low or negative correlation between them has a lower risk than the weighted average of the risks of the different securities. The main result of modern portfolio theory is that the risk weight of a portfolio should be lower than the average of the risk weights of the securities in it. This situation is likely to generate optimal diversification [ 3 ]. Under efficient market hypothesis, the perfect market transmits precise signals for a better allocation of resources. This is likely to help investors make their investment decisions, while based on the transparency and availability of information on securities prices [ 11 ].
The connection between international stock market linkages has been the subject of several empirical studies, largely confirming these theories. In addition, these studies have used different estimation methods. Therefore, this paper highlights a brief overview of this subject by referring to some previous studies. More specifically, we classify these studies according to the econometric techniques most frequently used in the literature. The ARDL model and the co-integration method have been extensively used by several researchers to examine the correlation between stock returns at the international and regional level (e.g., [ 12 , 13 , 14 , 15 , 16 , 17 ]. However, these different econometric techniques have not taken into consideration the time-varying correlations. They also ignored the different investment horizons over time.
This is likely to keep the literature on the existing relationship between stock markets very limited. To overcome these shortcomings, some empirical studies have recently been published on this topic, taking into account time-varying correlations and scale-dependent correlations. To do so, they have used more sophisticated econometric methods. First, several studies employed the Dynamic Conditional Correlation models (DCC-MGARCH) in order to detect the presence of time-varying correlations, i.e., whether there are correlations between different periods in the stock markets (e.g., [ 7 , 8 , 10 , 18 , 19 , 20 ]. Second, some empirical applied the CWT to jointly examine co-movements and lead-lag effects between two stock markets in the time and frequency domain, i.e., to assess the time variation and scale variation in co-movements between two stock markets (e.g., [ 7 , 9 , 21 , 22 , 23 , 24 ]. More specifically, other papers used wavelet-based quantile-on-quantile approach [ 25 , 26 ], and spillover index [ 25 , 26 ]. Third, other several studies employed the MODWT approach to provide insights on the analyzed markets volatility in the time–frequency domains (e.g., [ 7 , 27 , 28 ]. Finally, other studies employed the WMC and WMCC to analysis the integration between stock markets and to specify the leader of the market (e.g., [ 22 , 29 , 30 ].
Methodology
In this paper, we employ three econometric methods to examine the portfolio diversification benefits for Islamic and conventional investors in the USA with its major trading partners. The first method is the continuous wavelet transform (CWT) analysis. This approach has been applied by several studies in finance and economics research. Footnote 2 The CWT traces the original time series. It transforms it from a function with a single variable separated in time into a function with two different variables. These two variables include time and frequency. In addition, the CWT method allows the correlations of the series to be plotted in a two-dimensional diagram that helps to identify and interpret patterns or hidden information. The correlation between two CWTs is referred to as wavelet coherence. These plots specify the degree of correlation between two variables as a function of time and frequency. Daubechies [ 39 ] proposes the least length-asymmetric wavelet filter (denoted LA (8)). This filter consists of eight nonzero coefficients. The filter of length L = 8 is considered as a moderate length. The length filter is adequate for dealing with the characteristics of time series data [ 5 , 40 ]. In addition, the wavelet coefficient generated by LA (8) is smoother than that of other filters such as the Haar wavelet filter [ 40 ]. According to Rua & Nunes [ 41 ], the continuous wavelet transform is expressed as follows:
where u reflects the position of the wavelet in the time domain, and s represents the position in the frequency domain. From this information on time and frequency can be obtained together. This is achieved by transforming the original time series into a function of u and s in the wavelet transform. Footnote 3
The coherence of the wavelets is illustrated by a contour plot, where the horizontal axis indicates time, while the vertical axis corresponds to the frequency component (converted into units of time of days). By assessing the degree of co-movements, the coherence changes from blue (low coherence) to red (high coherence). The black area in the coherence graphs is the one that is statistically significant at the 5% significance level. It is estimated from a Monte Carlo simulation. Therefore, cross-wavelet coherence can investigate the different characteristics of the correlation between the index returns in the time–frequency domain. In addition, the dotted arrows indicate the phase difference in the wavelets. This is likely to provide information about the lead/lag relationship in time–frequency space.
The left ( \(\to\) )/right ( \(\leftarrow\) ) arrows indicate that the two-time series under consideration are in-phase and anti-phase, respectively. In-phase and anti-phase phenomena represent positive and negative co-movements, respectively. The upward ( \(\uparrow\) ), right-upward ( \(\nearrow\) ) and left downward ( \(\swarrow\) ) arrows represent the first time series leads the second one. Similarly, the downward ( \(\downarrow\) ), right-downward ( \(\searrow\) ) and left-upward ( \(\nwarrow\) ) arrows indicate the first time-series tends to follow the lead of the second series (e.g., [ 22 , 42 ].
The second and third techniques are the Wavelet Multiple Correlation and Wavelet Multiple Cross-Correlation. Fernández-Macho [ 29 ] developed these techniques to overcome a few of the limitations of pairwise and cross-correlations. For simplicity, the WMC is calculated as:
where \(P_{j}^{ - 1}\) is the correlation matrix of the wavelet coefficients ( \(W_{{{\text{jt}}}} = \left( {w_{{1{\text{jt}}}} , \cdots ,w_{{{\text{njt}}}} } \right)\) ). They coefficients are defined for the respective scales \(\lambda_{j}\) for a multivariate stochastic process \(X_{t} = \left( {x_{1t} , \cdots ,x_{{{\text{nt}}}} } \right)\) to each of the \(x_{it}\) ( \(i = 1 \cdots n\) ) process. Footnote 4 In theory, the WMC may be defined as:
where the wavelet variances \(\left( {{\text{Var}}\left( {w_{{{\text{ijt}}}} } \right)} \right)\) and co-variances \(\left( {{\text{Cov}}\left( {w_{{{\text{ijt}}}} } \right)} \right)\) are examined by: \({\text{Var}}\left( {W_{{{\text{ijt}}}} } \right) = \overline{\delta }_{j}^{2} = 1/T\;_{j} \sum\nolimits_{{t = :j^{ - 1} }}^{T - 1} {W_{{{\text{ijt}}}}^{2} }\) ; \({\text{Var}}\left( {\hat{W}_{{{\text{ijt}}}} } \right) = \overline{\xi }_{j}^{2} = 1/T\;_{j} \sum\nolimits_{{t = :j^{ - 1} }}^{T - 1} {\hat{W}_{{{\text{ijt}}}}^{2} }\) ; \({\text{Cov}}\left( {W_{{{\text{ijt}}}} ,\;\hat{W}_{{{\text{ijt}}}} } \right) = \hat{\gamma }_{j}^{2} = 1/T\;_{j} \sum\nolimits_{{t = :j^{ - 1} }}^{T - 1} {W_{{{\text{ijt}}}} ,\;\hat{W}_{{{\text{ijt}}}} }\) [ 5 ].
Following Fernández-Macho [ 29 ], the WMCC is calculated as:
The methodology of CWT, WMC, and WMCC is particularly useful for analyzing signals or time series data that have non-stationary properties, meaning their statistical properties vary over time. The CWT allows for the decomposition of a signal into different frequency components, while retaining the time information. This allows for the identification of local patterns and variations in the signal that may not be apparent when looking at the signal. The CWT can also be used to filter out certain frequency components or noise from the signal, making it a valuable tool in signal processing [ 41 , 42 ] and [ 22 ]. WMC measures the correlation between multiple time series, considering the non-stationary properties of the data. This is achieved by decomposing each time series using CWT, and then computing the correlation coefficients between the corresponding components at each scale. WMC can be used to identify common patterns or relationships between multiple time series, even if they have different time scales or underlying trends. Hence, as early mentioned, it is used to measure the degree of integration between different asset classes [ 29 ]. WMCC is an extension of WMC that measures the cross-correlation between multiple time series, again considering the non-stationary properties of the data. This is achieved by computing the wavelet coherence between each pair of time series, which measures the degree to which they share common frequency components. WMCC can be used to identify causal relationships between multiple time series, even if there are lags or delays between them. Thus, as early mentioned, it employed to identify the market leader among different asset classes [ 29 ].
Data and empirical results
In this study, the Dow Jones conventional and Islamic indices returns are used as a proxy for the US stock index returns which is the principal Conventional and Islamic benchmark indices of the US stock exchange. In addition, we use the principal trading partners of United States which are from United Kingdom, Canada, China, Japan, Turkey, and Malaysia. The choice of this index, as mentioned above, depends mainly on the largest single-point drop in history for the Dow Jones, which fell 2,997.10 on March 16, 2020. The list of the indexes and their respective tickers are in Table 1 .
All the data comes from Thomson-Reuters Datastream database. These indexes are transformed to market returns by computing the natural logarithmic differences of the daily closing prices ( \({\text{ln}}(p_{t} ) - {\text{ln}}(p_{t - 1} )\) ). The closing prices are collected during the period from 26 th November 2007 to 19th Mars 2020, covering 3195 trading days. There are several reasons why this particular time period may have been chosen. First, it is possible that the researchers chose the sample period of 26th November 2007 to 19th March 2020 in order to capture two significant crises that had a major impact on financial markets: the global financial crisis of 2008 and the COVID-19 pandemic. The global financial crisis of 2008 was a significant event that had a major impact on financial markets around the world. It began in late 2007 and continued through 2008 and beyond. By selecting a sample period that includes the period leading up to the financial crisis, as well as the crisis itself and its aftermath, the researchers may be able to capture the full impact of the crisis on financial markets. Similarly, the COVID-19 pandemic, which began in early 2020, had a significant impact on financial markets. By including data from the beginning of the pandemic through March 2020, the researchers may be able to capture the initial impact of the pandemic on financial markets. Comparing the two crises could also be a reason for selecting this time period. The researchers may be interested in analyzing how the two crises differ in terms of their impact on financial markets, or in identifying similarities or patterns between the two crises. The plots are presented in Fig. 1 .
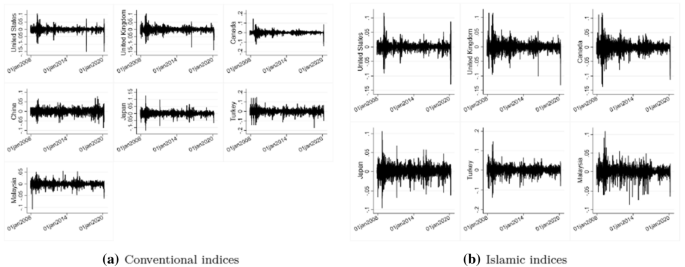
Source Author’s own computation
Returns series plot of conventional and Islamic Dow Jones indices (DJUS, DJUK, DJCA, DJCH, DJJA, DJTUR, and DJMA & DJIUS, DJIUK, DJICA, DJICH, DJIJA, DJITUR, and DJIMA).
Table 2 provides a summary of the descriptive statistics for all the indices used in this study. According to this table, the increase in standard deviation value indicates the existence of increased volatility of returns for the conventional Dow Jones US index. However, this volatility remains the lowest for US Islamic returns. Moreover, with the exception of the Dow Jones Conventional Canada returns, the presence of skewness was detected for all other returns. This asymmetry proves the existence of high risks. Moreover, the results of the normality test show that the kurtosis values are greater than three are. This translates into the fact that the returns of the conventional and Islamic indices are not normally distributed, also showing the existence of high risks. Similarly, referring to the results of the Jarque–Bera test, it can be noted that the distribution is not normal, as all returns of the conventional and Islamic indices are significant. This shows the severity of the variability and risk of the returns of the conventional and Islamic indices, which are significant.
Estimation using the CWT analysis
The results obtained from the CWT analysis, based on various investment horizons, will be discussed in this section. Before performing this approach, it was first necessary to test the linearity hypothesis. Figure 2 plots the linear correlation coefficients. These coefficients show a low degree of correlation at the level of the whole sample. However, it should be noted, that linear correlations may not satisfactorily account for nonlinear relationships [ 22 , 43 ]
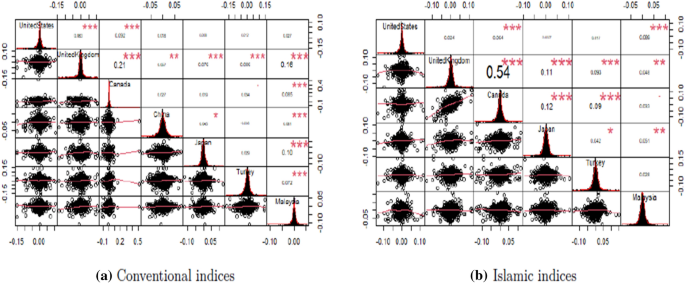
Scatter plots, histograms, and linear correlations of conventional and Islamic Dow Jones indices. Note : ***, **, * represents those linear correlations are significant at 1% 5%, and 10% levels, respectively.
To remedy this problem, we use the BDS test on the residuals of the AR(1) process for the returns of the conventional and Islamic DJ indices [ 22 , 44 ]. The null hypothesis of this test is independent and identical distribution (i.i.d). Table 3 presents the BDS test results, indicating that the null hypothesis could not be accepted. This result indicates the risk of unreliability of linear correlation results and the need to perform wavelet-based correlation.
To perform the CWT analysis, we apply the wavelet coherence method mentioned in the above section. Figures 3 and 4 illustrate the results of the CWT analysis and the phase difference between the returns of the US Dow Jones indices (conventional and Islamic) and their counterparts from scale 1 (one day) to scale 5 (1024 days). The horizontal axis indicates time in terms of the number of days of trading, while the vertical axis denotes the investment horizon. For our study, the conventional and Islamic Dow Jones indices in the US are the first series in all wavelet coherence diagrams. The R language was used to perform all kinds of calculations. In this framework, our analysis is based on four main scales, namely very short investment horizons (2–4 and 4–8), short investment horizons (8–16, 16–32 and 32–64), medium investment horizons (64–128 and 128–256) and longer investment horizons (256–512 and 512–1024).
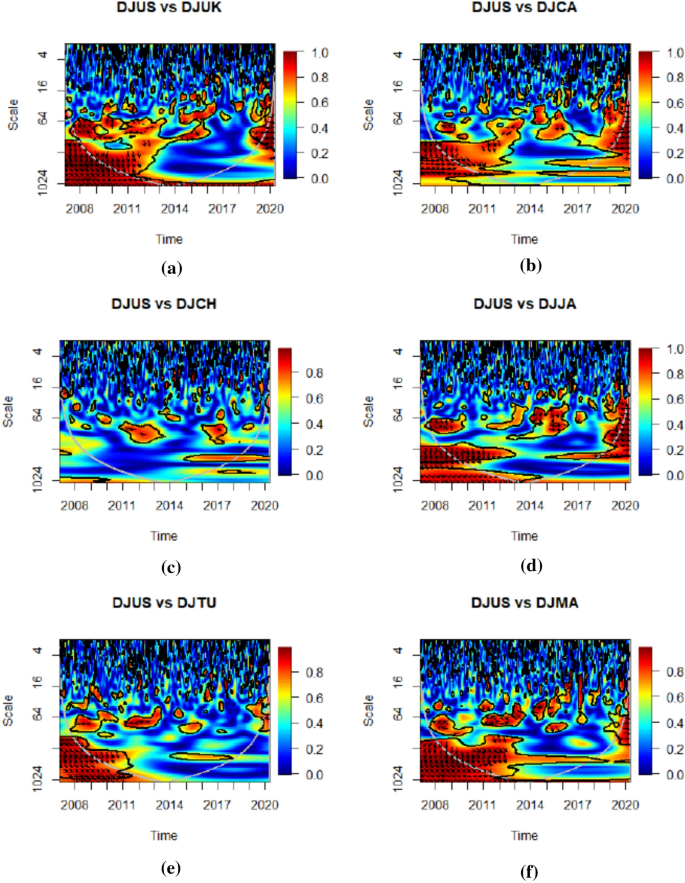
Continuous wavelet transform-Conventional indices returns. Note : Wavelet coherence maps. Note: The red (blue) colors present the region with high (low) coherency. The coherence power color bar indicates the power of coherence coefficients. The black contour presents the 5% significance level estimated from Monte Carlo Simulations.
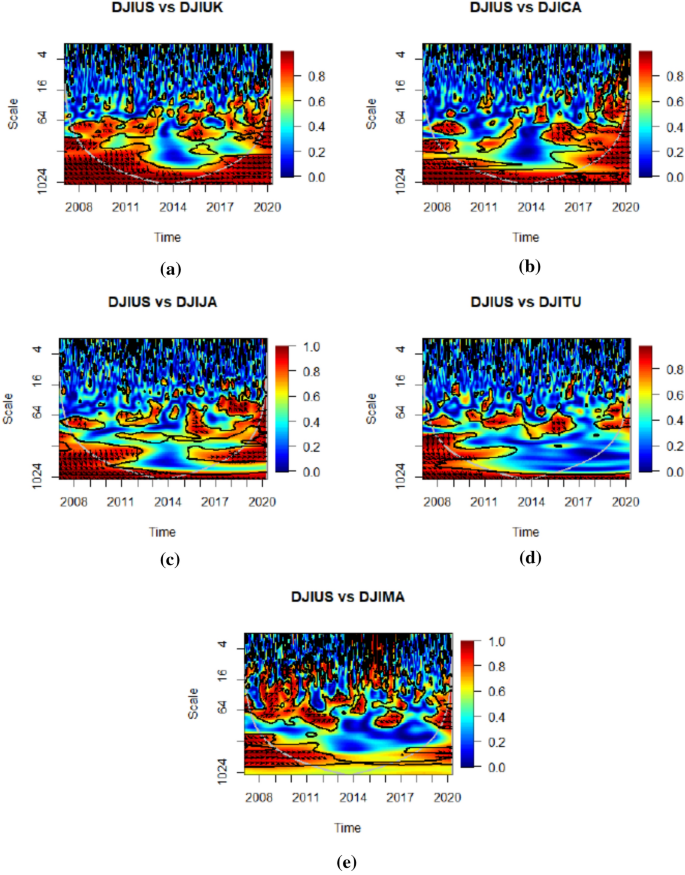
Source : Author’s own computation
Continuous wavelet transform-Islamic indices returns. Note : Wavelet coherence maps. The red (blue) colors present the region with high (low) coherency. The coherence power color bar indicates the power of coherence coefficients. The black contour presents the 5% significance level estimated from Monte Carlo Simulations.
For the very short investment holding periods (2–4 days and 4–8 days), we note that the correlations are low between all the returns in the Dow Jones U.S indices (conventional and Islamic) and the major trading partners throughout the study period, except for the Islamic Malaysia index. Overall, these low correlations are detected through the coherence diagram which represents the regions in blue and light blue color. Thus, these low correlations generate a low risk, which in turn encourages US investors to invest in the short term. From these results, geographic diversification is a good strategy for US investors in the very short term.
However, we remark that the correlations between the returns of the conventional and Islamic Dow Jones Indices are highly elevated for the short (8–16, 16–32, and 32–64) and medium investment horizons (64–128 and 128–256), which lead a low diversification opportunity. Specifically, these high correlations are mainly a result of the onset and propagation of several financial crises, going through the 2008 financial crisis to the COVID-19 crisis. The financial crisis revealed a large crash in the world stock markets that occurred in October 2008. Specifically, the findings show a high correlation between the returns of US indices (conventional an Islamic) and the returns of United Kingdom indices (conventional an Islamic). This high correlation is also due to the Eurozone sovereign crisis of 2011–2012. In addition, the stock market crash in China, which began on June 12, 2015, caused a high correlation between the return of the Dow Jones index of China and the USA. Moreover, the findings also suggest a high correlation between the returns of the Dow Jones Indices of Turkey and the USA during the period of the Turkish lira crisis of 2018. Finally, we thus find a high correlation between all the returns of the conventional and Islamic Dow Jones Indices of the United States and trading partners during the COVID-19 period. Certainly, this high correlation could reduce the investment opportunities for conventional and Islamic US investors.
Turning to the longer investment horizons (256–512 and 512–1024), we observe a strong correlation between all US conventional and Islamic Dow Jones index returns and the returns of major trading partner indices, especially in 2008 at the time of the financial crisis and the COVID-19 crisis. This high correlation indicates poor diversification opportunities for US investors regardless of the regime they follow (conventional or Islamic). In summary, we explain all these circumstances in more detail in Sect. “ Discussions ”.
Estimation applying the WMC and WMCC techniques
The CWT results show higher coherences in the returns of the conventional and Islamic US DJ indices. These higher coherences lead us to analysis the integration of the US stock market. Specifically, we investigate the extent to which the US stock market (conventional and Islamic) is integrated. Based on the financial literature, it appears that a highly integrated market is similar to a common investment portfolio. In other words, the advantage of shifting investments from one economy to another is indifferent [ 22 , 29 , 36 ]. Similar to this literature, we apply wavelet multiple correlation analysis to measure the market integration. Table 4 reports the result of WMC.
The WMC results show low correlation coefficients (0.364 for the conventional market and 0.178 for the Islamic market) at wavelet scale 1 (2–4 days) that increase smoothly to 0.998 at the longest horizon of 128–256 days (for both conventional and Islamic US markets). Thus, the findings show that US markets (as measured by the Dow Jones index) are tending toward an optimal level of integration when there are only limited benefits from diversification among their constituent economies. In addition, Fig. 5 plots the data pictorial representation.
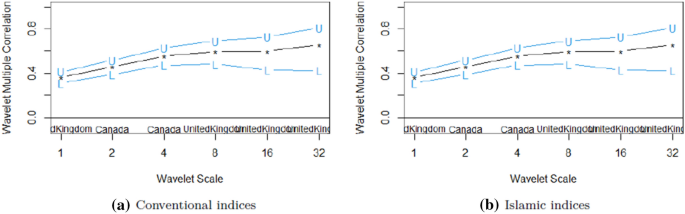
Wavelet multiple correlation for Dow Jones returns. Note : The blue dashes represent the upper and lower bounds of the 95% confidence interval.
Figure 6 reports WMCC results for different wavelet levels applying the Daubechies less asymmetric filter of length eight. Similar to Polanco-Martínez & Fernández-Macho [ 45 ] and Das et al. [ 22 ], the cross-correlations are plotted up to one-month lag. Furthermore, the location of the highest correlation can be seen at the longest wavelet scale of 32 for a lag of up to 10 days. Thus, this method allows the identification of potential market leaders in the US. Following Polanco-Martínez & Fernández-Macho [ 45 ], the right-hand side of the wavelet scale mappings presents the series that optimizes the cross-correlations with respect to the linear combination of the other considered time series. The results then show that the UK appears to be the eventual market leader at wavelet scales 2, 16 and 32. However, at wavelet scales 1, 4 and 8, Canada appears to be the market leader.
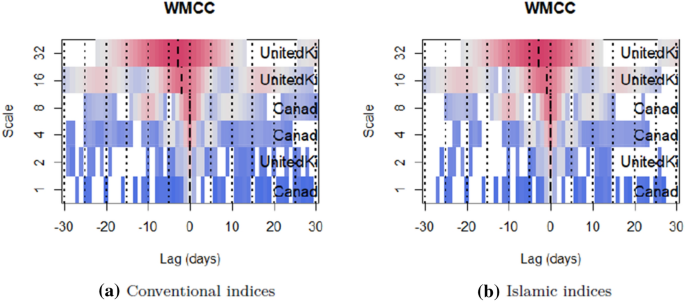
Wavelet multiple cross-correlation for Dow Jones indices (conventional and Islamic) returns. Note : The white parts show the parts where the 95% interval is equal to zero. The long vertical dashed lines show the area with the most significant correlation between the wavelets. The color band on the right presents the strength of the correlation. For each wavelet correlation, the coefficients are within the 95% confidence interval.
Discussions
Based on the results obtained by the different techniques, we find the presence of several circumstances that could explain the change in the level (having direct effects) of the correlations between the returns of the conventional and Islamic Dow Jones indexes of the United States and the main trading partners. Moreover, the results show a strong correlation between the returns of DJUS indices (conventional and Islamic) and the returns of DJCA indices (conventional and Islamic). These results prove that the Canadian stock index is a risky investment for U.S. investors. This could be attributed mainly to the great increase in trade activity between these two countries when comparing to other trading partners. According to Trade (2019), Canada remains the main trading partner of the United States, as bilateral trade between the two countries recorded 612.4 billion in December 2019.
Analyzing the correlation between the returns of the US indices (DJUS and DJIUS) and those of Turkey (DJTUR and DJITUR), we find overall a low correlation, except for the period of the financial crises especially the Turkish lira crisis of 2018. In fact, this period was marked by a massive increase in inflation rates (with an annual inflation rate of around 13% for 2018) and a cut in key interest rates implemented by the Turkish regulatory authority. This situation is mainly the result of trade threats between Turkey and the USA. In this case, the Turkish lira loses almost half of its value on August 13, 2018, from 3.5 lira to 1 dollar to 7.5 lira to 1 dollar. This decrease generated therefore to a strong association between the returns of the indices of two countries.
Relating to the medium investment horizons (64–128 and 128–256), correlations were high between the US and the Asian trading partner (Malaysia and Japan). These results are consistent with those found by Rahim & Masih [ 7 ]. The authors concluded that the markets in Malaysia and Japan were evolving toward greater integration. This was clearly observed after the 1997 financial crisis. Specifically, there is a relatively strong correlation between the returns of the US indices (DJUS, DJIUS) and those of Malaysia (DJMA and DJIMA). Therefore, if traditional US investors decided to invest in Malaysia, diversification benefits could be generated when investing at shorter time periods than 8–16 days. However, US Islamic investors do not benefit from diversification if they invest in Malaysia during all investment horizons.
The low correlation between the returns of the U.S. (DJUS and DJIUS) and China (DJCH and DJICH) indices that is observed during for almost all investment horizons is explained by commercial relationship. Indeed, China is considered the largest trading partner in terms of imports for the USA with 452 . 2 billion. This shows an increase in trade activity between the two countries. However, the market crash in China, which began on June 12, 2015, by the ricochet of the US stock markets, leads to a high correlation between the returns of these indices. This period was marked by a drop in the value of stocks on the Shanghai stock exchange (about a the one-third) for a month. In this case, the ability of listed companies to access financing has been reduced, thus hindering their growth. This crash created a financial bubble that began in November 2014. Moreover, in 2018, the increase in customs taxes, resulting from trade disputes, disrupted the financial markets of these countries. Therefore, if US investors were to invest in China, they could realize the benefits of diversification when they do not do so during the average time horizons (64–128 and 128–256). Finally, the COVID-19 crisis did not lead to a significant correlation between the returns of the two countries' indices.
Turning to the WMCC findings, the basic reason for Canada’s emergence as a market leader in the USA is obvious in the North American Free Trade Agreement (NAFTA). Canada and the US trade more than 1 million dollars in goods and services every minute of every day of the year, totaling an estimated 718.4 billion dollars in 2019 (USTR, 2019). Following this agreement, Canada has restructured certain industrial sectors to better integrate its economy with that of the USA In addition, the main reason for United Kingdom’s emergence as a market leader in the USA could be attributed to the spatial relationship between the two countries. Although the UK was the seventh largest trading partner of the USA in 2019 [ 46 ], WMCC results suggest that it is the US industrial market leader. These findings could encourage the two countries to conclude a free trade agreement.
Based on these findings, a more important question deserves to be asked: why has the spread of COVID-19 provoked such perverse repercussions on the stock markets since the end of February 2020? To answer on this question, we focus on recent studies with different explanations. According to Baker et al. [ 2 ], the current pandemic has serious public health and economic implications. In addition, these authors linked this spread of COVID-19 to several factors such as the severity of the pandemic and the non-negligible increase in mortality rates. In addition, the period of COVID-19 is accompanied by a huge technological development compared to that of the Spanish flu. Therefore, this technological development has been a major factor in the disclosure of reliable information about the COVID-19 pandemic [ 2 ]. The most important conclusion reached by the authors is that the effect of the COVID-19 pandemic on the stock markets turns out to be more concentrated in time. This effect is also able to generate a daily jump trigger and high stock market volatility compared to the evolution of the Spanish flu over the last century [ 2 ]. A more extensive explanation focuses on the attitude and policy responses to the COVID-19 outbreak situation. Baldwin [ 47 ] suggested that the crisis period and the confinement policies of COVID-19 generated a direct and huge reduction in the flow of labor to firms. These policies then led to a dramatic reduction in the production of goods and services. In addition, other factors led to the disruption of financial markets, the most reactive of which was the voluntary adoption of social distancing practices.
Conclusions and implications
Conclusions.
This paper investigates the portfolio diversification benefits for investors in the USA with their main commercial partners, both Islamic and conventional (United Kingdom, Canada, China, Japan, Malaysia, and Turkey) before and during the COVID-19 crisis period. To do so, we collected daily time series closing price data for the Dow Jones conventional and Islamic indices returns from November 26, 2007, to March 19, 2020. Similar to previous studies, we employ three econometric methods: CWT analysis WMC, and WMCC. The results of the CWT analysis focused on four main scales namely very short investment horizons (2–4 and 4–8), short investment horizons (8–16, 16–32 and 32–64), medium investment horizons (64–128 and 128–256) and longer investment horizons (256–512 and 512–1024). Regarding the first scale results (2–4 days and 4–8 days), these proved that the correlations are generally low between all the returns of the US Dow Jones Indices (conventional and Islamic) and trading partners during the full period, except for the Islamic Malaysia index. Turning to the second (8–16, 16–32 and 32–64) and third scales results (64–128 and 128–256), these showed that the correlations between the returns of the US Dow Jones Indices (conventional and Islamic) and the major trading partners are highly significant. Finally, except for China, the fourth scale results (256–512 and 512–1024) indicated high correlation between the returns of US Dow Jones Indices (conventional and Islamic) and major trading partners, specifically during the financial and Covid-19 crises. These findings indicate poor diversification opportunities for US investors regardless of the regime they apply (conventional or Islamic).
In addition, the WMC results indicate that the US markets (as measured by the Dow Jones index) are trending toward a point of optimal integration. Finally, the WMCC findings suggest that the UK appears to be the eventual market leader at the 2, 16 and 32 wavelet scales. However, at wavelet time scales 1, 4 and 8, Canada appears to be the market leader.
Certainly, serval conclusions can be drawn from the case study on portfolio diversification benefits in the USA. First, the case study could explore in more depth the specific benefits of portfolio diversification, such as risk reduction, improved returns, and better asset allocation. The study could also discuss how these benefits apply to different types of investors, such as institutional investors, individual investors, and high-net-worth individuals. Second, the study could analyze how different economic conditions affect the benefits of portfolio diversification. For example, during a recession, diversification may provide a greater degree of risk reduction than during a boom period. The study could discuss the benefits of international diversification, including the potential to reduce overall portfolio risk by investing in a broader range of markets and asset classes. It could also examine the challenges and risks associated with international diversification, such as currency fluctuations and political instability. Third, the study could provide practical advice for investors who want to diversify their portfolios. This could include information on how to determine an appropriate asset allocation, how to select diversified investments, and how to monitor and adjust the portfolio over time. Finally, the study could also discuss the limitations of portfolio diversification, such as the fact that diversification does not eliminate all investment risk, and the potential for correlation among different asset classes during times of market stress. Overall, the case study on portfolio diversification benefits in the USA provides a valuable starting point for further discussion and analysis. By exploring the various aspects of portfolio diversification, investors can gain a better understanding of the potential benefits and risks and make more informed decisions about how to structure their investment portfolios.
Practical implications
In terms of policy implications, it is essential to highlight that geographic diversification is an appropriate policy for US investors in very short investment horizons (2–4 and 4–8). However, during the COVID-19 period, this policy is not an appropriate investment for US investors if they are investing in the horizons of 16–32 days and longer. This is because the integration of financial markets could generate risks (a risk of exchange rate volatility, an increase in the rate of inflation, etc.), leading to global shocks. However, during the COVID-19 crisis, conventional US investors investing with Chinese partners may reap large diversification benefits for medium and longer investment horizons. In sum up, American investors and portfolio managers must adopt a sectoral diversification rather than geographic diversification in times of COVID-19 because the pandemic operates in waves. In addition, to take benefit of portfolio diversification, US investors should not invest only in stocks (e.g., include other financial assets) and reassess their investment horizons. Therefore, policymakers need to consider these implications.
Portfolio diversification is a risk management strategy that involves investing in a variety of assets to reduce the overall risk of the portfolio. Empirical analyses have shown that diversification can offer several practical implications and economic benefits, especially during times of economic uncertainty and market volatility. The financial crisis of 2008 highlighted the importance of diversification in investment portfolios. During this period, many investors suffered significant losses due to the high concentration of investments in a few asset classes. However, those who had diversified portfolios with investments in different asset classes, such as bonds and real estate, were better positioned to weather the storm. Similarly, the COVID-19 pandemic has demonstrated the importance of diversification during times of unexpected economic shocks. The pandemic led to significant market volatility, causing many asset classes to suffer losses. However, those with diversified portfolios were able to mitigate their losses by having investments in sectors that were less affected by the pandemic, such as technology and healthcare. Moreover, empirical analyses have shown that portfolio diversification can provide economic benefits such as higher returns and lower volatility. A well-diversified portfolio can provide exposure to a range of assets with different risk and return characteristics, which can lead to better risk-adjusted returns. In addition, events such as changes in interest rates and inflation can also have implications for portfolio diversification. For example, in a high inflation environment, investors may benefit from having exposure to commodities and real estate, which can act as a hedge against inflation. Overall, portfolio diversification is an important strategy for investors to manage risk and maximize returns. By diversifying across different asset classes and sectors, investors can reduce the impact of market volatility and position themselves to take advantage of economic opportunities.
Limits and future research
Finally, boosted by the announcement of a future vaccine on November 8, 2020, the Dow Jones closed sharply up 2.95% at 29,157.97 points. In this case, our study indicates avenues for future research that could examine portfolio diversification benefits after the vaccination period.
Availability of data and materials
We have used secondary sources to complete our study. No new data are used or produced in this study.
Restrictions, imposed then by Islamic law, such as the prohibition of riba and gharar , subrogation of bank loans by investments in real assets (i.e., Sukuk, Murabaha, Ijara…etc.) and interest charged with profit and loss sharing among stakeholders, are put in place in order to improve economic efficiency.
See, e.g., Vacha & Barunik [ 31 ], Madaleno & Pinho [ 32 ], Abdullah et al. [ 33 ], Najeeb et al. [ 34 ], Dewandaru et al. [ 35 ], Tiwari et al. [ 36 ],Yang et al. [ 37 ], Sakti et al. [ 38 ], Das et al. [ 22 ].
For more details of the Wavelet approach specification, see, among others, Rahim & Masih [ 7 ], Das et al. [ 22 ].
For more details in the specification of this techniques, see e.g., Fernández-Macho [ 29 ], Das et al. [ 22 ].
Albulescu CT (2021) COVID-19 and the United States financial markets’ volatility. Financ Res Lett 38:101699
Article Google Scholar
Baker SR, Bloom N, Davis SJ, Kost KJ, Sammon MC, & Viratyosin T (2020) The unprecedented stock market impact of COVID-19. National Bureau of Economic Research
Markowitz H (1952) Portfolio selection. J Financ 7(1):77–91
Google Scholar
Khan TA (2011) Cointegration of international stock markets: an investigation of diversification opportunities. Undergrad Econ Rev 8(1):7
Gençay R, Selçuk F, Whitcher BJ (2001) An introduction to wavelets and other filtering methods in finance and economics. Elsevier
El-Gamal MA (2006) Islamic finance. Law, economics, and practice. Cambridge University Press, Cambridge
Book Google Scholar
Rahim AM, Masih M (2016) Portfolio diversification benefits of Islamic investors with their major trading partners: evidence from Malaysia based on MGARCH-DCC and wavelet approaches. Econ Model 54:425–438
Raza N, Ali S, Shahzad SJH, Rehman MU, Salman A (2019) Can alternative hedging assets add value to Islamic-conventional portfolio mix: evidence from MGARCH models. Resour Policy 61:210–230
Rizvi SAR, Arshad S, Alam N (2015) Crises and contagion in Asia Pacific—Islamic v/s conventional markets. Pac Basin Financ J 34:315–326
Trabelsi N, Naifar N (2017) Are Islamic stock indexes exposed to systemic risk? Multivariate GARCH estimation of CoVaR. Res Int Bus Financ 42:727–744
Fama EF (1970) Efficient market hypothesis: a review of theory and empirical work. J Financ 25(2):28–30
Arshanapalli B, Doukas J (1993) International stock market linkages: evidence from the pre-and post-October 1987 period. J Bank Financ 17(1):193–208
Bley J, Chen KH (2006) Gulf cooperation council (GCC) stock markets: the dawn of a new era. Glob Financ J 17(1):75–91
Majdoub J, Mansour W, Jouini J (2016) Market integration between conventional and Islamic stock prices. North Am J Econ Financ 37:436–457
Majid M, Kassim SH (2010) Potential diversification benefits across global Islamic equity markets. J Econ Coop Dev 31(4):103–126
Marashdeh H (2005) Stock market integration in the MENA region: an application of the ARDL bounds testing approach
Wu Y, Jing W, Liu J, Ma Q, Yuan J, Wang Y, Du M, Liu M (2020) Effects of temperature and humidity on the daily new cases and new deaths of COVID-19 in 166 countries. Sci Total Environ 729:139051
Bugan MF, Cevik EI, Dibooglu S (2021) Emerging market portfolios and Islamic financial markets: diversification benefits and safe havens. Borsa Istanbul Rev 22:77–91
Celık S (2012) The more contagion effect on emerging markets: the evidence of DCC-GARCH model. Econ Model 29(5):1946–1959
Saiti B, Bacha OI, Masih M (2014) The diversification benefits from Islamic investment during the financial turmoil: the case for the US-based equity investors. Borsa Istanb Rev 14(4):196–211
Aloui C, Hkiri B (2014) Co-movements of GCC emerging stock markets: new evidence from wavelet coherence analysis. Econ Model 36:421–431
Das D, Kannadhasan M, Al-Yahyaee KH, Yoon S-M (2018) A wavelet analysis of co-movements in Asian gold markets. Physica A 492:192–206
Mariana CD, Ekaputra IA, Husodo ZA (2021) Are bitcoin and ethereum safe-havens for stocks during the COVID-19 pandemic? Financ Res Lett 38:101798
Sun Q, Xu W (2018) Wavelet analysis of the co-movement and lead–lag effect among multi-markets. Physica A 512:489–499
Su C-W, Rizvi SKA, Naqvi B, Mirza N, Umar M (2022) COVID19: a blessing in disguise for European stock markets? Financ Res Lett 49:103135
Su C-W, Yuan X, Umar M, Chang T (2022) Is presidential popularity a threat or encouragement for investors? Econ Res Ekonomska Istraživanja. https://doi.org/10.1080/1331677X.2022.2129409
Reboredo JC, Rivera-Castro MA (2013) A wavelet decomposition approach to crude oil price and exchange rate dependence. Econ Model 32:42–57
Reboredo JC, Rivera-Castro MA (2014) Wavelet-based evidence of the impact of oil prices on stock returns. Int Rev Econ Financ 29:145–176
Fernández-Macho J (2012) Wavelet multiple correlation and cross-correlation: a multiscale analysis of Eurozone stock markets. Physica A 391(4):1097–1104
Kumah SP, Odei-Mensah J (2021) Are cryptocurrencies and African stock markets integrated? Q Rev Econ Financ 81:330–341
Vacha L, Barunik J (2012) Co-movement of energy commodities revisited: evidence from wavelet coherence analysis. Energy Econ 34(1):241–247
Madaleno M, Pinho C (2012) International stock market indices comovements: a new look. Int J Financ Econ 17(1):89–102
Abdullah AM, Saiti B, Masih M (2016) The impact of crude oil price on Islamic stock indices of Southeast Asian countries: evidence from MGARCH-DCC and wavelet approaches. Borsa Istanbul Rev 16(4):219–232
Najeeb SF, Bacha O, Masih M (2015) Does heterogeneity in investment horizons affect portfolio diversification? Some insights using M-GARCH-DCC and wavelet correlation analysis. Emerg Mark Financ Trade 51(1):188–208
Dewandaru G, Masih R, Masih AMM (2016) Contagion and interdependence across Asia-Pacific equity markets: an analysis based on multi-horizon discrete and continuous wavelet transformations. Int Rev Econ Financ 43:363–377
Tiwari AK, Mutascu MI, Albulescu CT (2016) Continuous wavelets transform and rolling correlation of European stock markets. Int Rev Econ Financ 42:237–256
Yang L, Cai XJ, Hamori S (2017) Does the crude oil price influence the exchange rates of oil-importing and oil-exporting countries differently? A wavelet coherence analysis. Int Rev Econ Financ 49:536–547
Sakti MRP, Masih M, Saiti B, Tareq MA (2018) Unveiling the diversification benefits of Islamic equities and commodities: evidence from multivariate-GARCH and continuous wavelet analysis. Manag Financ 44(6):830–850
Daubechies I (1992) Ten lectures on wavelets, Vol. 61 of CBMS-NSF regional conference series in applied mathematics, SIAM: Philadelphia
In F, Kim S (2013) An introduction to wavelet theory in finance: a wavelet multiscale approach. World Scientific, Singapore
Rua A, Nunes LC (2009) International comovement of stock market returns: a wavelet analysis. J Empir Financ 16(4):632–639
Jiang Z-Q, Yang Y-H, Wang G-J, Zhou W-X (2017) Joint multifractal analysis based on wavelet leaders. Front Phys 12(6):128907
Andrieş AM, Ihnatov I, Tiwari AK (2016) Comovement of exchange rates: a wavelet analysis. Emerg Mark Financ Trade 52(3):574–588
Broock WA, Scheinkman JA, Dechert WD, LeBaron B (1996) A test for independence based on the correlation dimension. Economet Rev 15(3):197–235
Polanco-Martínez JM, Fernández-Macho FJ (2014) Package W2CWM2C: description, features, and applications. Comput Sci Eng 16(6):68–78
Commerce (2019a) U.S. Department of Commerce. In U.S. Department of Commerce
Baldwin R (2020) The supply side matters: Guns versus butter, COVID-style. VOX CEPR Policy Portal, 22
Download references
Acknowledgements
We thank the editor and the anonymous reviewers for their constructive comments.
The authors received no specific funding.
Author information
Authors and affiliations.
Department of Accounting, College of Management and Technology, Arab Academy for Science, Technology and Maritime Transport, Giza, Egypt
Eman F. Attia & Sharihan Mohamed Aly
Finance Department, College of Management and Technology, Arab Academy for Science, Technology and Maritime Transport, Giza, Egypt
Eman F. Attia
Finance Department, College of Management and Technology, Arab Academy for Science, Technology and Maritime, Giza, Egypt
Ahmed said ElRawas
Accounting Department, Sadat Academy for Management Sciences, Cairo, Egypt
Ebtehal Orabi Awad
You can also search for this author in PubMed Google Scholar
Contributions
EA contributed in the conceptualization, validation, the design of the work, and writing the original draft. AR supervised project and provided his valuable comments to develop the quality of research. SM reviewed and edited the writing of the manuscript. EOA contributed in the acquisition, analysis, or interpretation of data; the using of statistical analysis in the work, writing the original draft. All authors have read and approved the manuscript. All authors read and approved the final manuscript.
Corresponding author
Correspondence to Eman F. Attia .
Ethics declarations
Ethics approval and consent to participate.
Not applicable.
Consent for publication
Competing interests.
The authors declare that they have no conflict of interest.
Additional information
Publisher's note.
Springer Nature remains neutral with regard to jurisdictional claims in published maps and institutional affiliations.
Rights and permissions
Open Access This article is licensed under a Creative Commons Attribution 4.0 International License, which permits use, sharing, adaptation, distribution and reproduction in any medium or format, as long as you give appropriate credit to the original author(s) and the source, provide a link to the Creative Commons licence, and indicate if changes were made. The images or other third party material in this article are included in the article's Creative Commons licence, unless indicated otherwise in a credit line to the material. If material is not included in the article's Creative Commons licence and your intended use is not permitted by statutory regulation or exceeds the permitted use, you will need to obtain permission directly from the copyright holder. To view a copy of this licence, visit http://creativecommons.org/licenses/by/4.0/ .
Reprints and permissions
About this article
Cite this article.
Attia, E.F., Aly, S.M., ElRawas, A.s. et al. Portfolio diversification benefits before and during the times of COVID-19: evidence from USA. Futur Bus J 9 , 26 (2023). https://doi.org/10.1186/s43093-023-00205-4
Download citation
Received : 21 December 2022
Accepted : 31 March 2023
Published : 16 June 2023
DOI : https://doi.org/10.1186/s43093-023-00205-4
Share this article
Anyone you share the following link with will be able to read this content:
Sorry, a shareable link is not currently available for this article.
Provided by the Springer Nature SharedIt content-sharing initiative
- Diversification benefits
- Trading partners
- Wavelet analysis
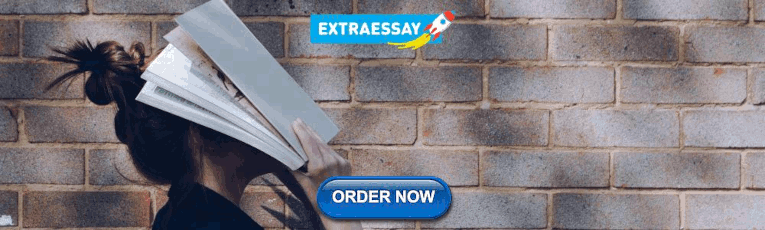
IMAGES
VIDEO
COMMENTS
Diversification is one of the major components of investment decision-making under risk or uncertainty. However, paradoxically, as the 2007-2009 financial crisis revealed, the concept remains misunderstood. Our goal in writing this paper is to correct this issue by reviewing the concept in portfolio theory. The core of our review focuses on the following diversification principles: law of ...
The remainder of the paper is organized as follows: ... Portfolio diversification strategies research stream focuses on examining the diversification strategies for the stock-bond markets along with other alternative assets (e.g., Dbouk and Kryzanowski, 2009). Examining the most studied diversification strategies, novel contributions to the ...
1. INTRODUCTION. 1.1 It's often said that diversi cation is the only 'free lunch' available to investors; meaning that a properly diversi ed portfolio reduces total risk without necessarily ...
This paper gathers the various approaches on portfolio diversification throughout history, placing a higher emphasis on recent developments. The goal of this paper is not to provide an exhaustive list of diversification strategies, but rather to highlight the most commonly used ones, provide the motivation behind each approach, and show how ...
By Rustam Ibragimov2 3. nomics, Harvard UniversityABSTRACTThis paper focuses on the study of portfolio diversi ̄cation and value at r. sk analysis under heavy-tailedness. We use a notion of diversi ̄cation based on majorization theory. that will be explained in the text. The paper shows that the stylized fact that portfolio diversi ̄cation ...
In this paper we examine the portfolios of more than 40,000 equity investment accounts from a large discount brokerage during a six y ear period (1991-96) in recent U.S. capital market history . Using
A research in the Journal of Business found 90% of specific risk can be removed by just holding 16 stocks . A paper published by the Omega Journal shows that 20 stocks can eliminate 95% of the diversifiable risk in a portfolio . But the portfolio requires additional 80 stocks to remove another 4% of specific risk. ... This shows the ability of ...
A new framework for portfolio diversification is introduced which goes beyond the classical mean-variance approach and portfolio allocation strategies such as risk parity. It is based on a novel concept called portfolio dimensionality that connects diversification to the non-Gaussianity of portfolio returns and can typically be defined in terms of the ratio of risk measures which are ...
Using extensive and comprehensive databases to select a subset of research papers, we aim to critically analyze previous empirical studies to identify certain patterns in determining the optimal number of stocks in well-diversified portfolios in different markets, and to compare how the optimal number of stocks has changed over different periods and how it has been affected by market turmoil ...
Choosing the number of stocks to hold in a portfolio can significantly affect its risk. We use daily observations for traded equity returns in the US, UK, Japan, Canada and Australia from 1975 to 2011 to simulate portfolios and calculate several measures of risk, including heavy tailed.
Abstract. Investment diversification is a widely accepted investment strategy, aimed at reducing investment uncertainty, while simultaneously keeping the expected return on investment unaltered ...
The diversification benefit is the difference in value of a value maximizing portfolio and the maximum value of the components. The spread reduction is the percentage reduction attained by a spread minimizing portfolio relative to the smallest spread for the components. Asset values, bid and ask, are given by conservative valuation operators ...
The Value of Diversification . Diversification is the only free lunch in finance . ... a Research Associate at the National Bureau of Economic Research (NBER), a Fellow of the TIAA-CREF Institute, and a member of the Asset Allocation Advisory Board to Norges Bank ... company on the value of a well diversified portfolio is trivially small, while ...
This paper analyses whether Sukuk can be used as alternative asset classes in conventional portfolios, and whether that can lead to diversification benefits and reduce volatility risks. One of the motivations of this paper and its research questions is to understand the practical uses of regionalised financial instruments like Sukuk and suggest ...
The two opposing investment strategies, diversification and concentration, have often been directly compared. While there is much less dispute regarding Markowitz's approach as the benchmark for diversification, the precise meaning of concentration in portfolio selection remains unclear. This paper offers a novel definition of concentration, along with an extreme value theory-based estimator ...
Academia Journal of Educational Research 10(1): 019-030, January 2020 DOI: 10.15413/ajer.2021.0146 ISSN 2315-7704 ©2022 Academia Publishing Research Paper Domestic international portfolio diversification gains in the case of US investors Accepted 27th December, 2021 ABSTRACT
Achieving Portfolio Diversification for Individuals with Low Financial Sustainability. August 2020. Sustainability 12 (17):7073. DOI: 10.3390/su12177073. License. CC BY 4.0. Authors: Yongjae Lee ...
This paper investigates the portfolio diversification benefits for Islamic and conventional investors in the USA with its major trading partners (United Kingdom, Canada, China, Japan, Malaysia, and Turkey) before and during the COVID-19 crisis period. Using daily data from 2007 to 2020, we employ three relevant time-varying and timescale-dependent techniques, the continuous wavelet transform ...
The research in this paper has considered the level of integration between BRICS markets to help identify where the most benefits from portfolio diversification can be achieved. While this is one factor that needs to be considered when making investment decisions, another key factor is to consider the macro-economic factors and other residual ...
T ypes, modes, and levels of diversi cation are the most complicated yet intertwined. processes in the strategic management literature for optimization of rm. performance. e antecedents and ...
not imply the superiority of diversification must be rejected both as a hypothesis and as a maxim. * This paper is based on work done by the author while at the Cowles Commission for Research in Economics and with the financial assistance of the Social Science Research Council. It will be reprinted as Cowles Commission Paper, New Series, No. 60. 1.
monitoring of va rious assets within a portfolio to optimize returns depending on an investor's. investing goals, time horizon, and risk tolerance. During its early phases of development ...
Abstract. Diversification is a strategic option that investors use to optimize their portfolio. Diversification is investing in many assets for the purpose of minimizing risk or maximizing return ...