
An official website of the United States government
The .gov means it’s official. Federal government websites often end in .gov or .mil. Before sharing sensitive information, make sure you’re on a federal government site.
The site is secure. The https:// ensures that you are connecting to the official website and that any information you provide is encrypted and transmitted securely.
- Publications
- Account settings
- My Bibliography
- Collections
- Citation manager
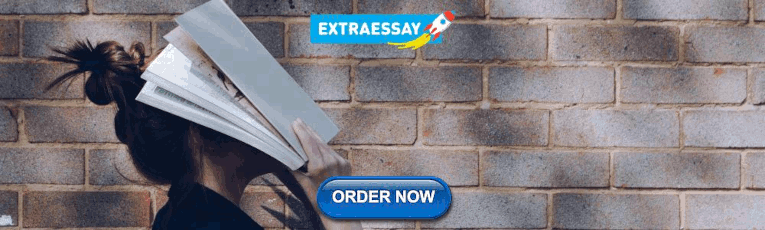
Save citation to file
Email citation, add to collections.
- Create a new collection
- Add to an existing collection
Add to My Bibliography
Your saved search, create a file for external citation management software, your rss feed.
- Search in PubMed
- Search in NLM Catalog
- Add to Search
Targeting Inflammation in Acne: Current Treatments and Future Prospects
Affiliations.
- 1 Department of Dermatology, Keck School of Medicine, University of Southern California, 830 S Flower St Ste 100, Los Angeles, CA, 90017, USA.
- 2 Department of Dermatology, Keck School of Medicine, University of Southern California, 830 S Flower St Ste 100, Los Angeles, CA, 90017, USA. [email protected].
- PMID: 37328614
- PMCID: PMC10460329
- DOI: 10.1007/s40257-023-00789-1
Acne is a common, chronic inflammatory condition affecting millions of people worldwide, with significant negative impact on quality of life and mental health. Acne is characterized by comedones, inflammatory papules, pustules, and nodulocystic lesions, with long-lasting sequelae including scarring and dyspigmentation, the latter of which is more common in skin of color. The four main pillars of acne pathophysiology include alteration of sebum production and concentration, hyperkeratinization of the follicular unit, Cutibacterium acnes strains, and an inflammatory immune response. Newer research has provided greater insight into these pathophysiologic categories. This greater understanding of acne pathogenesis has led to numerous new and emerging treatment modalities. These modalities include combinations of existing treatments, repurposing of existing agents historically used for other conditions, new topical treatments, novel antibiotics, topical and oral probiotics, and various procedural devices. This article will provide an overview of emerging treatments of acne and their link to our current and improved understanding of acne pathogenesis.
© 2023. The Author(s).
PubMed Disclaimer
Conflict of interest statement
Dr. Elbuluk has served as a consultant to Galderma. Dr. Cruz and Dr. Vecerek have no conflicts of interest.
Similar articles
- Acne. A review of optimum treatment. Sykes NL Jr, Webster GF. Sykes NL Jr, et al. Drugs. 1994 Jul;48(1):59-70. doi: 10.2165/00003495-199448010-00006. Drugs. 1994. PMID: 7525195 Review.
- Rational therapy for acne vulgaris: an update on topical treatment. Leyden JJ, Shalita AR. Leyden JJ, et al. J Am Acad Dermatol. 1986 Oct;15(4 Pt 2):907-15. doi: 10.1016/s0190-9622(86)70250-8. J Am Acad Dermatol. 1986. PMID: 2945846
- Common and alternate oral antibiotic therapies for acne vulgaris: a review. Amin K, Riddle CC, Aires DJ, Schweiger ES. Amin K, et al. J Drugs Dermatol. 2007 Sep;6(9):873-80. J Drugs Dermatol. 2007. PMID: 17941358 Review.
- Topical acne treatments in Europe and the issue of antimicrobial resistance. Leccia MT, Auffret N, Poli F, Claudel JP, Corvec S, Dreno B. Leccia MT, et al. J Eur Acad Dermatol Venereol. 2015 Aug;29(8):1485-92. doi: 10.1111/jdv.12989. Epub 2015 Feb 10. J Eur Acad Dermatol Venereol. 2015. PMID: 25677763 Review.
- Diagnosis and treatment of acne. Titus S, Hodge J. Titus S, et al. Am Fam Physician. 2012 Oct 15;86(8):734-40. Am Fam Physician. 2012. PMID: 23062156 Review.
- Fibromyalgia and Skin Disorders: A Systematic Review. D'Onghia M, Ciaffi J, Calabrese L, Tognetti L, Cinotti E, Rubegni P, Frediani B, Ursini F. D'Onghia M, et al. J Clin Med. 2024 Jul 27;13(15):4404. doi: 10.3390/jcm13154404. J Clin Med. 2024. PMID: 39124671 Free PMC article. Review.
- Exploring Acne Treatments: From Pathophysiological Mechanisms to Emerging Therapies. Kim HJ, Kim YH. Kim HJ, et al. Int J Mol Sci. 2024 May 13;25(10):5302. doi: 10.3390/ijms25105302. Int J Mol Sci. 2024. PMID: 38791344 Free PMC article. Review.
- Illuminating microflora: shedding light on the potential of blue light to modulate the cutaneous microbiome. Serrage HJ, O' Neill CA, Uzunbajakava NE. Serrage HJ, et al. Front Cell Infect Microbiol. 2024 Apr 10;14:1307374. doi: 10.3389/fcimb.2024.1307374. eCollection 2024. Front Cell Infect Microbiol. 2024. PMID: 38660491 Free PMC article. Review.
- Recent Trends in the Management of Acne Vulgaris: A Review Focusing on Clinical Studies in the Last Decade. Jaiswal S, Jawade S, Madke B, Gupta S. Jaiswal S, et al. Cureus. 2024 Mar 20;16(3):e56596. doi: 10.7759/cureus.56596. eCollection 2024 Mar. Cureus. 2024. PMID: 38646359 Free PMC article. Review.
- Recent advances in the treatment of acne using radiofrequency techniques. Zhang X, Zhou M, Liu Y, Zeng R. Zhang X, et al. Lasers Med Sci. 2024 Mar 19;39(1):92. doi: 10.1007/s10103-024-04039-6. Lasers Med Sci. 2024. PMID: 38499897 Review.
- Heng AHS, Chew FT. Systematic review of the epidemiology of acne vulgaris. Sci Rep. 2020;10(1):5754. doi: 10.1038/s41598-020-62715-3. - DOI - PMC - PubMed
- Knutsen-Larson S, Dawson AL, Dunnick CA, Dellavalle RP. Acne vulgaris: pathogenesis, treatment, and needs assessment. Dermatol Clin. 2012;30(1):99–106. doi: 10.1016/j.det.2011.09.001. - DOI - PubMed
- Vos T, Flaxman AD, Naghavi M, et al. Years lived with disability (YLDs) for 1160 sequelae of 289 diseases and injuries 1990–2010: a systematic analysis for the Global Burden of Disease Study 2010. The Lancet. 2012;380(9859):2163–2196. doi: 10.1016/S0140-6736(12)61729-2. - DOI - PMC - PubMed
- Bickers DR, Lim HW, Margolis D, et al. The burden of skin diseases: 2004. J Am Acad Dermatol. 2006;55(3):490–500. doi: 10.1016/j.jaad.2006.05.048. - DOI - PubMed
- Stathakis V, Kilkenny M, Marks R. Descriptive epidemiology of acne vulgaris in the community. Australas J Dermatol. 1997;38(3):115–123. doi: 10.1111/j.1440-0960.1997.tb01126.x. - DOI - PubMed
- Search in MeSH
Related information
Linkout - more resources, full text sources.
- Europe PubMed Central
- PubMed Central
- MedlinePlus Health Information
- Citation Manager
NCBI Literature Resources
MeSH PMC Bookshelf Disclaimer
The PubMed wordmark and PubMed logo are registered trademarks of the U.S. Department of Health and Human Services (HHS). Unauthorized use of these marks is strictly prohibited.
Log in using your username and password
- Search More Search for this keyword Advanced search
- Latest content
- For authors
- Browse by collection
- BMJ Journals
You are here
- Volume 11, Issue 2
- Views and experiences of people with acne vulgaris and healthcare professionals about treatments: systematic review and thematic synthesis of qualitative research
- Article Text
- Article info
- Citation Tools
- Rapid Responses
- Article metrics

- http://orcid.org/0000-0002-8574-2569 Athena Ip 1 , 2 ,
- http://orcid.org/0000-0001-9341-6133 Ingrid Muller 1 ,
- http://orcid.org/0000-0001-7984-8351 Adam W A Geraghty 1 ,
- Duncan Platt 1 ,
- http://orcid.org/0000-0003-3664-1873 Paul Little 1 ,
- Miriam Santer 1
- 1 School of Primary Care, Population Sciences and Medical Education, Faculty of Medicine , University of Southampton , Southampton , UK
- 2 Faculty of Health and Medical Sciences, School of Health Sciences , University of Surrey , Guildford , Surrey , UK
- Correspondence to Athena Ip; a.ip{at}soton.ac.uk
Objectives The objective of this study was to systematically review and synthesise qualitative papers exploring views and experiences of acne and its treatments among people with acne, their carers and healthcare professionals (HCPs).
Design Systematic review and synthesis of qualitative papers.
Methods Papers were identified through Medline, EMBASE, PubMed, PsychINFO and CINAHL on 05 November 2019, forward and backward citation searching, Google Scholar and contacting authors. Inclusion criteria were studies reporting qualitative data and analysis, studies carried out among people with acne, their carers or HCPs and studies comprising different skin conditions, including acne. The title and abstracts of papers were independently screened by three researchers. Appraisal was carried out using the adapted Critical Appraisal Skills Programme tool. Thematic synthesis was used to synthesise findings.
Results A total of 20 papers were included from six countries. Papers explored; experiences living with acne, psychosocial impact of acne, views on causation of acne, perceptions of acne treatments, ambivalence and ambiguity in young people’s experience of acne and HCPs’ attitudes towards acne management. Findings suggest that people often viewed acne as short-term and that this had implications for acne management, particularly long-term treatment adherence. People often felt that the substantial impact of acne was not recognised by others, or that their condition was ‘trivialised’ by HCPs. The sense of a lack of control over acne and control over treatment was linked to both psychological impact and treatment adherence. Concerns and uncertainty over acne treatments were influenced by variable advice and information from others.
Conclusions People need support with understanding the long-term management of acne, building control over acne and its treatments, acknowledging the impact and appropriate information to reduce the barriers to effective treatment use.
PROSPERO registration number CRD42016050525.
- qualitative research
- dermatology
This is an open access article distributed in accordance with the Creative Commons Attribution Non Commercial (CC BY-NC 4.0) license, which permits others to distribute, remix, adapt, build upon this work non-commercially, and license their derivative works on different terms, provided the original work is properly cited, appropriate credit is given, any changes made indicated, and the use is non-commercial. See: http://creativecommons.org/licenses/by-nc/4.0/ .
https://doi.org/10.1136/bmjopen-2020-041794
Statistics from Altmetric.com
Request permissions.
If you wish to reuse any or all of this article please use the link below which will take you to the Copyright Clearance Center’s RightsLink service. You will be able to get a quick price and instant permission to reuse the content in many different ways.
Strengths and limitations of this study
This synthesis of qualitative studies provides a broader understanding around perceptions of acne and acne treatments than any single study, which can inform barriers and facilitators to treatment adherence.
The search strategy was comprehensive and used the Information Specialists’ Sub-Group search filter resource to ensure that all relevant terms were covered.
The methods were robust, including three researchers screening all papers identified from database searches, independent quality appraisal of publications and a team approach to developing codes, themes and model to best reflect the data.
The review was limited by gaps in the evidence base, which helps highlight future areas for further qualitative research.
Introduction
Acne vulgaris is a common skin condition worldwide. 1 It can have a substantial impact on quality of life both physically and psychologically. 2 Treatments for mild to moderate acne are topical preparations including topical retinoids or adapalene, topical antibiotics, combination topicals and azelaic acid. 3 If these are not effective, oral antibiotics are prescribed or, in women, combined oral contraception or cocyprindiol. More severe acne is treated with oral isotretinoin. 3 4
Quantitative research has found that adherence to acne treatments is poor. 5 This is primarily the case for topical treatments for reasons including side effects, young age and forgetfulness. 6 While quantitative research is useful for determining the prevalence and common reasons given for non-adherence, qualitative research is essential for understanding people’s views and perceptions around treatments and more fully understand barriers and facilitators to treatment adherence.
By synthesising qualitative research on acne, we can generate new understandings that go beyond the primary studies. 7 This is useful for informing future research and for developing interventions to support people in effectively managing their condition.
The aim of this systematic review was to identify and synthesise qualitative papers exploring views and experiences of acne and its treatments among people with acne, their carers and healthcare professionals (HCPs).
The Enhancing transparency in reporting the synthesis of qualitative research statement was used to facilitate appropriate reporting for this synthesis of qualitative studies. 8
Search strategy
Five databases were searched on 05 November 2019 using a comprehensive search strategy: Medline (1946–2019), EMBASE (1974–2019), PubMed (1996–2019), PsychINFO (1806–2019) and CINAHL (1981–2019). Databases were chosen to ensure that literature on nursing, social science, psychology and medicine were searched as comprehensively as possible. Other resources included backward and forward citation searching using Google Scholar, contacting authors of included papers regarding other articles or when full texts were unavailable.
The search strategy was developed through discussions with coauthors and a medical librarian at the University of Southampton. Searching for qualitative literature can be difficult and that is why we included a librarian and used the Information Specialists’ Sub-Group search filter resource to ensure that all relevant terms related to acne and qualitative research were covered (see online supplemental material A for the list of search terms). We defined qualitative as papers presenting qualitative method of data collection and analysis as well as presenting qualitative data (quotes). There were no date or language restrictions.
Supplemental material
Eligible papers reported on studies that used qualitative methods of data collection and analysis, presented qualitative data either standalone or distinct part of a mixed-methods study, included people with acne, HCPs treating acne or carers/parents of children with acne and studies that considered more than one skin condition that included acne.
Selection process
Three independent researchers screened the title and abstracts of the papers (AI, DP and IM). AI conducted the full-text screening of eligible papers and any uncertainties were discussed with coauthors.
Quality appraisal
An adapted version of the Critical Appraisal Skills Programme tool was used to provide an indication of strengths and weaknesses of the qualitative papers. 9 All papers were included regardless of quality. Papers were appraised by AI, and other members of the research team (AWAG, MS and IM) independently appraised a third of papers each. Disagreements in quality assessment were resolved through discussion.
Data extraction
Study characteristics extracted from each paper included: author(s), country, year of publication, focus, participants, skin conditions, data collection, methodology, analysis and key themes presented by the author. The papers were repeatedly read by AI to ensure that all quotes and relevant text under the ‘results’ or ‘findings’ were extracted onto NVivo V.11 software to manage and code the data. 10
Synthesis of findings
A thematic synthesis was carried out involving three stages. 7 First AI carried out line-by-line coding of relevant text (quotes or authors’ descriptions). Next, the free codes were organised to develop descriptive themes across studies. A coding manual was produced to facilitate the systematic coding of the data. The themes identified were deliberated with IM, MS, AWAG and PL and any discrepancies were discussed until the agreement was reached. The third stage involved ‘going beyond’ the data to develop analytical themes that generate additional understanding from synthesising original studies. Analytical themes were produced through team discussions and a model was developed showing the interrelationship between themes and their association with treatment initiation (decision to start treatment) and adherence.
Patient and public involvement
No patients were involved in carrying out this systematic review. Following publication, results will be disseminated through lay summary and social media.
The database search identified 2931 records and seven papers were found through other resources (2519 after removing duplicates). After eligibility screening, 20 papers were included in the synthesis ( figure 1 ).
- Download figure
- Open in new tab
- Download powerpoint
PRISMA flow diagram of search strategy and included papers.
Study characteristics
The included studies were relatively heterogeneous, primarily exploring the following topics: experiences living with acne, psychosocial impact of acne, complementary and alternative medicines (CAM), sexual life and acne, patients’ relationships with their doctors, views on causation of acne, perceptions of acne treatments, ambivalence and ambiguity in young people’s experience of acne and HCPs’ attitudes towards acne management. Methods of data collection included face-to-face, video or telephone interviews, written interviews online and searching online discussion forums. Studies were carried out in India, US, UK, Australia, Italy and Germany ( table 1 ).
- View inline
Study characteristics of papers included in the synthesis
Quality appraisal results
The overall quality of the papers varied with longer articles providing more information for the checklist. Many of the studies did not explore reflexivity of the researcher in terms of their disciplinary knowledge and epistemological position. In addition, many of the papers did not include participant characteristics when presenting quotes. Some papers did not explicitly state the qualitative approach or a recognised approach to analysis. One paper reported findings from a commercial trial that could result in bias and therefore conclusions from this study should be drawn with caution. 11
Synthesis of results
Four overarching analytical themes were further developed from descriptive themes generated in the line-by-line coding: (1) People with acne tended to view their condition as short-term, (2) impact of acne not recognised by HCPs, others or self, (3) people wanted to have a sense of control over acne treatments and acne and (4) a range of barriers to acne treatments and strategies to help cope with acne. Figure 2 presents how the analytical and descriptive themes influence people’s initiation and adherence to acne treatment. Table 2 presents a checklist of the studies that reported on each analytical theme. Example quotes or authors’ description of quotes are presented in table 3 . General practitioners’ (GPs) views and perceptions are summarised separately as only one paper reported on this.
Model presenting the interrelationships between themes and how they influence treatment initiation and adherence. CAM, complementary and alternative medicine.
Analytical and descriptive themes with study reference
Analytical and descriptive themes with representative quotes or authors' descriptions
Acne is viewed as short-term
People with acne often seemed to view their condition as short-term and not requiring long-term treatment. Study participants commonly seemed to have little initial concern over their acne as they expected to ‘grow out of it’ due to the perception that their acne was caused by puberty or other underlying causes/triggers. Studies showed how people expected treatment to cure their acne as opposed to control it, suggesting that they did not view their condition as requiring long-term management, with implications for initiating and adhering to treatment ( table 3 ).
Identifying potential causes or triggers of acne
A common perception across studies was of viewing acne as a ‘normal’ part of adolescence. However, most participants seemed to have followed a more chronic course with some experiencing acne as an adult, which led to frustration and confusion. 12–17 People looked for other possible causes including hygiene and diet with the hope of ‘curing’ their acne. Hygiene concerns with regards to acne were related to dirty occupations, pollution, sweat, makeup and inadequate washing. 13 18 Dietary considerations around acne included foods such as chocolate, soft drinks, fast foods, coffee, yeast and alcohol. 13 15 Genetics and stress were less commonly mentioned by study participants. 13
Expectation that treatment will cure acne not control it
People expected medical treatments to ‘cure’ their acne, often feeling disappointed when this was not met. Participants described treatment as ‘keeping their acne at bay’, being only partially effective or not working at all. 14 19 This appeared to have implications for acne management, with disappointment leading to stopping treatment early or opting for alternative treatments in the absence of ‘instant’ results.
Impact of acne not recognised
People across studies experienced substantial impact because of their acne and were frustrated when they felt that this was not recognised by HCPs, friends and family. Physical, psychological and social impact were common and often led to problems with forming new relationships as well as maintaining current ones. Perceptions of blame from others and self-blame were apparent in the data, sometimes relating to the myths and misconceptions around acne causation. The perceived trivialisation by HCPs and work colleagues was common across the data and appeared to have implications for acne management including consulting behaviours.
Perceived physical impact
Physical impact was commonly discussed across studies and consisted of physical appearance, itching, quality of sleep, burning, scaring, redness and pain. 11 12 15 16 18 20 21
Perceived psychological impact
Study participants described the psychological impact of acne as feeling embarrassed, self-conscious, angry about the perceived cause of their acne, low self-esteem, suicidal, changes in personality and feeling ostracised from society due to the image of ‘perfect skin’ portrayed by the media. 11 12 15–19 21–23
Perceived social impact
The social impact of acne was commonly reported across studies. People engaged in avoidance behaviours had a negative effect on relationships due to feeling self-conscious about their appearance 11 15 16 18 and a lack of confidence and worry about how they would be perceived. 12 16 17 20 21 23 Bullying and teasing appeared to increase psychological impact. 15–18 21 22 24 In terms of education and employment, participants reported missing school, feeling distracted, 16 experiencing interpersonal difficulties (insensitive work colleagues and the public) and feeling self-conscious. 15 16 21
Perceived blame
A number of studies reported on feelings of self-blame and blame inflicted by others. 12 15 16 18 25 Family members were sometimes perceived to blame participants if they had not ‘grown out of it’ as expected. When participants perceived their acne to be caused by diet or hygiene, this sometimes led to self-blame as these were within their control.
Perceived trivialisation by HCPs, others and self
Participants in several studies perceived acne to be ‘trivialised’ by HCPs, for instance, leaving consultations feeling as though they were not listened to, feeling as though prescriptions were given without a second thought or feeling as though their condition was not taken seriously due to waiting for a referral to see a dermatologist. 15 16 19 26 Participants also perceived trivialisation of acne among work colleagues, for instance, ignorance about acne and the need for appointments with HCPs, or around work absence. 21 There was an element of ‘self-trivialisation’ as participants in some studies described feeling reluctant to take on the ’sick role’ mainly due to the stigma associated with acne, believing that their condition was a cosmetic issue rather than a medical one. 12 As a result, people may try alternative treatments for their acne to avoid consulting the HCP.
Perceived control over acne treatments and acne
Across studies, there were two aspects of control: people’s perceived control over acne and their control over treatment. 13 18 27 Their perceived control over treatment referred to people’s beliefs in their chosen treatment rather than the control being in someone else’s hands (HCP). For example, people in the studies opted for CAM and behavioural strategies, which they felt would alleviate the psychological impact of acne. Three studies reported on people’s perceived control over their acne including feelings of powerlessness when treatments were perceived as ineffective. 11 16 17 One study (reporting findings from a commercial trial) found when people perceived increased control over their acne, this improved satisfaction with acne symptoms and alleviated the impact, regardless of acne improvement using a topical. 11 Having control over treatment or acne appeared to help alleviate the psychological impact and improve adherence.
Barriers to acne treatments and use of coping strategies
Across studies, a key barrier to use of acne treatments was concern and uncertainty regarding their effectiveness, exacerbated by variable advice and support people received from others. Studies highlighted coping strategies discussed by participants, including concealment/compensation (as described below) and making comparisons, which some participants found useful in the short-term. Participants in many studies reported a preference for using CAM and behavioural strategies to address their acne. This could be viewed as a barrier to engaging with effective acne treatment or be perceived as a mechanism for coping through seeking control over the condition.
Concerns about perceived adverse effects and effectiveness of acne treatments
Concerns around topical treatments for acne included side effects (bleaching, irritation), strength of medication, speed of onset of action, what constituted appropriate application, storage, understanding different topicals and, as mentioned above, uncertainty around their effectiveness. 14 16 28 One study found effective use of topicals increased control over acne and reduced the psychological impact, although they did not explore perceptions of treatment ineffectiveness. 11 Two studies highlighted how patients viewed oral isotretinoin as an effective treatment, although they expressed concerns around the treatment’s side effects. 19 29 Perceived effectiveness of oral antibiotics varied as participants either found them effective, ineffective or partially effective where they worked temporarily. Barriers included delayed onset of action, perceived strength of treatment and adverse effects. 14 19 27 One study highlighted barriers such as cost of treatment and understanding processes used by health insurance companies. 28 This study was carried out in USA and therefore, the barriers may not be relevant to the UK population.
Desire to use CAM and behavioural strategies
Some studies explored CAM and behavioural strategies for treating acne. CAM treatments included oils, citrus washes, aloe vera, tablets and vitamins. Participants reported a preference for CAM over medical treatments due to ‘natural’ ingredients and fewer adverse effects. 27–29 Other reasons included sense of internal control and accessibility. 18 Behavioural strategies included dietary manipulation, face washing and sun and sea exposure (less commonly mentioned). The belief that hygiene caused or exacerbated acne led participants to excessively wash or pick their acne to resolve the issue. 13 17–19 25 Dietary manipulation included avoiding foods deemed unhealthy and increasing water intake. 13 18 19
Receiving variable advice and support about treatments and next steps
Support from family members was appreciated and included encouragement to consult HCPs, suggestions about which products to try 25 and some felt that humour about the condition or about their treatment (isotretinoin) from friends or family could make them feel less uncomfortable. 17 21 Participants felt that support from friends with acne were useful as they were able to relate to their situation 16 and recommendations from friends such as products to try were often seen as useful particularly for male participants as some female participants found the advice unsolicited. 21 25 Advice from online discussion forums was felt to be variable and often consisted of treatment recommendations or suggestions about consulting and navigating health services. 19
Concealment/compensation to cope with acne
Strategies to cope with acne included concealment to take attention away from their acne such as changing clothing and hairstyles. 11 16 17 Applying makeup helped some participants cope emotionally, but for others, this emphasised their spots or wore off quickly and some viewed makeup as a cause of acne. 12 16 People reported compensating for their acne by doing activities including martial arts 18 or losing weight. 25
Comparisons to earlier self and others
Strategies including making comparisons to others or their earlier self were seen as a double-edged sword, whereby participants either felt better about their acne or felt worse, further exacerbating the psychological impact. 12 17 19 25 Participants made comparisons to other health conditions to validate the negative impact of acne or to feel grateful that things were not worse. 12
Key differences between GPs and patients’ views and experiences
One study highlighted GP’s acknowledgement of the psychological impact as well as motivation to escalate severe cases for referrals. 30 Research suggests that people’s own assessment of acne severity differs from clinical assessments that may explain the contrasting views compared with people with acne in other studies, where they felt HCPs did not always take acne seriously. 31–33 The current study also found that GPs were uncertain about topical treatment effectiveness, which they posited may be related to patients’ treatment adherence. 30
This systematic review and synthesis of qualitative research highlighted four analytical themes that influence treatment initiation and adherence. People often viewed acne as a short-term condition resulting in implications for self-management, particularly challenges to long-term treatment adherence. The impact of acne was substantial for participants in these studies and they were often frustrated when they perceived others to trivialise their condition. The importance of perceived control was highlighted, including the wish to feel in control of acne and the wish to control treatment. Having control over either one appeared to help alleviate the psychological impact and improve adherence. People had common concerns around treatments that were further influenced by variable advice.
Strengths and weaknesses
To our knowledge, this is the first systematic review and synthesis of qualitative papers on acne. It provides a comprehensive overview of people’s views and experiences of acne and its treatments. We are confident that all relevant papers were included as three independent researchers were involved with screening the title and abstracts of papers. However, there is the possibility that we may have missed some studies because of our definition of qualitative and our inclusion/exclusion criteria whereby papers needed to present qualitative data, qualitative methods of data collection and analysis and provide a sufficient amount of information about the qualitative aspect if it was part of a wider study (eg, questionnaire development paper).
A potential weakness was the limited original research available as many of the included papers (eight) were from the same author. However, although these papers used the same sample, they focused on different research questions and looked at a breadth of peoples’ experiences. We found areas that were underrepresented including HCPs’ experiences treating acne, studies outside of UK and Australia and men with acne. The review was also restricted by the strengths and weaknesses present in the original papers.
Comparison with other studies
The findings are consistent with a review on the impact of eczema, psoriasis and epidermolysis bullosa, which found that people with chronic skin conditions experience negative social interactions. 34
A review of qualitative studies on adherence to medicines found that people were reluctant to take medicines partly because of concerns over its use including adverse effects and perceived effectiveness. 35 They also highlight how people wish to take control over their own treatment. 35 These findings are consistent with those in this current study, which goes further by suggesting that an increased feeling of control was felt to alleviate the psychological impact and improve adherence.
Studies exploring other skin conditions (vitiligo, psoriasis and eczema) including a paper from this current synthesis have also found that patients feel their HCP trivialises their skin condition. 26 36–39 Through synthesising the studies, we have also highlighted the role of self-trivialisation in influencing people’s consulting behaviours.
A quantitative systematic review of treatment adherence in acne found similar barriers around treatment adherence including adverse effects and delayed onset of action resulting in low adherence. 6 Our qualitative synthesis explores this further, suggesting that treatment adherence is influenced by the variable advice received, desire to use CAM and behavioural strategies and perception around the causes of acne, particularly perception that it is a short-term condition.
This synthesis suggests the need for further research exploring HCPs’ views and experiences with people with acne as certain areas (eg, perceived trivialisation, treatment choice, acne as a short-term condition and the psychological impact of acne) could be better addressed from both sides. The findings highlight the importance of communicating the long-term management of acne and the importance of control over acne or control over treatment. Further research around providing support for people with acne is needed, with emphasis on the need for mitigating psychological impact. Finally, people need reliable information about acne treatments including how to use them appropriately, time taken until onset of action and how to manage side effects to help them to effectively manage the condition.
- Williams HC , et al
- Williams HC ,
- Dellavalle RP ,
- Gollnick HP ,
- Bettoli V ,
- Lambert J , et al
- Zaenglein AL ,
- Schlosser BJ , et al
- Thiboutot D ,
- Gollnick H , et al
- Crandell I ,
- Davis SA , et al
- Flemming K ,
- McInnes E , et al
- Smith H , et al
- Skaggs RL ,
- Huang KE , et al
- Heading G , et al
- Geraghty AWA , et al
- Fabbrocini G ,
- Cacciapuoti S ,
- Monfrecola G
- Murray CD ,
- Chandler D ,
- Lown M , et al
- Pruthi GK ,
- Heading G ,
- Adams J , et al
- Heading GS , et al
- Ryskina KL ,
- Goldberg E ,
- Lott B , et al
- Zureigat M ,
- Hammond A , et al
- In S-I , et al
- Standage M ,
- Chen Wee Aw D ,
- Lee SH , et al
- Thompson AR
- Britten N ,
- Morgan M , et al
- Porter JR ,
- Lerner A , et al
- Burgess H ,
- Yardley L , et al
Supplementary materials
Supplementary data.
This web only file has been produced by the BMJ Publishing Group from an electronic file supplied by the author(s) and has not been edited for content.
- Data supplement 1
Twitter @IngridMuller7
Contributors Conception, design and planning of the study were by AI, IM, AWAG, MS and PL as this was part of AI’s PhD. Data curation, formal analysis and writing the original draft were by AI. AI screened all title and abstracts supported by IM and DP who carried out double screening of these articles. Full-text articles were screened by AI and any uncertainties were discussed with the team. AI carried out the quality appraisal on all papers and MS, AWAG and IM independently appraised a third each of these. All authors were involved with reviewing and editing the manuscript.
Funding This study is funded by the National Institute for Health Research (NIHR) School for Primary Care Research PhD Studentship for AI. The views expressed are those of the authors and not necessarily those of the NIHR or the Department of Health and Social Care.
Competing interests None declared.
Patient consent for publication Not required.
Provenance and peer review Not commissioned; externally peer reviewed.
Data availability statement All data relevant to the study are included in the article or uploaded as supplemental information.
Supplemental material This content has been supplied by the author(s). It has not been vetted by BMJ Publishing Group Limited (BMJ) and may not have been peer-reviewed. Any opinions or recommendations discussed are solely those of the author(s) and are not endorsed by BMJ. BMJ disclaims all liability and responsibility arising from any reliance placed on the content. Where the content includes any translated material, BMJ does not warrant the accuracy and reliability of the translations (including but not limited to local regulations, clinical guidelines, terminology, drug names and drug dosages), and is not responsible for any error and/or omissions arising from translation and adaptation or otherwise.
Read the full text or download the PDF:
REVIEW article
A review of advancement on influencing factors of acne: an emphasis on environment characteristics.
- 1 The Center for Modern Chinese City Studies, East China Normal University, Shanghai, China
- 2 School of Urban and Regional Science, East China Normal University, Shanghai, China
- 3 Department of Dermatology and Venereology, First Affiliated Hospital of Kunming Medical University, Kunming, China
- 4 Department of Dermatology, Third People's Hospital of Hangzhou, Hangzhou, China
Background: Acne vulgaris is known as a commonly-seen skin disease with a considerable impact on the quality of life. At present, there have been a growing number of epidemiological, medical, demographic and sociological researches focusing on various influencing factors in the occurrence of acne. Nevertheless, the correlation between environmental factors and acne has yet to be fully investigated.
Objective: To assess the impacts of individual, natural and social environmental factors on acne and to construct a framework for the potential impact of built environment on acne.
Methods: A thorough review was conducted into the published social demographical, epidemiological, and environmental studies on acne through PubMed, Google Scholar and Web of Science, with reference made to the relevant literature.
Results: The influencing factors in acne are classed into four major categories. The first one includes individual socio-economic and biological factors, for example, gender, age, economic level, heredity, obesity, skin type, menstrual cycle (for females), diet, smoking, cosmetics products, electronic products, sleep quality and psychological factors. The second one includes such natural environmental factors as temperature, humidity, sun exposure, air pollution and chloracne. The third one relates to social environment, including social network and social media. The last one includes built environmental factors, for example, population density, food stores, green spaces, as well as other built environment characteristics for transport. Acne can be affected negatively by family history, overweight, obesity, oily or mixed skin, irregular menstrual cycles, sugary food, greasy food, dairy products, smoking, the improper use of cosmetics, the long-term use of electronics, the poor quality of sleep, stress, high temperature, sun exposure, air pollution, mineral oils and halogenated hydrocarbons. Apart from that, there are also potential links between built environment and acne.
Conclusions: It is necessary to determine the correlation between the built environment and acne based on the understanding of the impact of traditional factors (sociology of population and environment) on acne gained by multidisciplinary research teams. Moreover, more empirical studies are required to reveal the specific relationship between built environment and acne.
Introduction
Chinese cities face many health challenges posed by rapidly changing urban environments (e.g., air pollution, water pollution, zoning and mix use of land, reduction of vegetation coverage and growing population density) and lifestyles (e.g., lacking physical activity, unbalanced diets, tobacco and alcohol use), especially non-communicable chronic diseases, such as cardiovascular disease, cancer, respiratory diseases, diabetes and mental illness, which have replaced infectious diseases as major contributors to the overall disease burden ( 1 ). Many skin diseases are also non-communicable chronic diseases, especially acne that mainly occurs on the face, which is easily affected by external factors.
Acne is a chronic inflammatory skin disease involving the sebaceous glands. Four major pathogenesis are involved in the development of androgen-induced increased sebum hyperproduction, altered follicular keratinization, inflammation and Propionibacterium acnes (P. acne) ( 2 , 3 ). It is also affected by environmental pollution, social environment, changes of dietary structure and lifestyle, for example, worsening air pollution, the intake of sweets, staying up late, social network and social media. Thus, the prevalence of acne increases year by year. According to a systematic analysis for the Global Burden of Disease Study, in 2010, the prevalence of acne among all the population in the world was 9.38%, ranking the eighth in the world ( 4 ). From 2006 to 2016, the prevalence of acne increased by 5.1% ( 5 ). In the meantime, in the US, the median cost per person per 7 months for acne treatments approved by the US Food and Drug Administration was $350–3,806 1 ( 6 ). Because of its high prevalence and recurrence, acne patients have suffered from the corresponding economic burden. In addition, although acne is not a life-threatening disease, it damages the appearance, which might leave scars on patients if not treated in time. Moreover, for young men and women, discosmetic dermatosis can easily lead to inferiority, even affecting the employment and marriage of patients. According to the study in China, 30.8% acne patients reported that acne had a negative impact on their quality of life ( 7 ). Several studies showed that people with acne had lower self-confidence, the difficulty of making friends, challenges of going to school, and the trouble of finding a job ( 8 , 9 ). Moreover, acne patients have a higher propensity of underlying mental disorders, including anxiety, depression and suicide ( 10 , 11 ). The prevalence of acne can not only impact the cost of drug treatment, but also the psychological disorders associated with acne and quality of life.
Built environment is defined to include all buildings, spaces, and products that are built or modified by humans. There is growing evidence that the built environment affects health in different ways and mechanisms ( 12 ), especially chronic diseases such as obesity ( 13 ), mental health ( 14 ), cardiovascular disease ( 15 ), and respiratory health ( 16 ). Acne is a chronic disease in which both environmental and genetic factors interact ( 17 ). Therefore, it might also be affected by the built environment. However, previous acne epidemiological studies mainly focus on individual factors (such as family history, diet, lifestyle, occupation, and psychological factors) and other natural and social environmental factors (such as air pollution and social network) ( 18 , 19 ), there is very limited research that examines whether a relationship exists between the built environment and acne. In order to fill up this gap, this article will first do a comprehensive review on the basis of the previous studies of sociology of population, epidemiology, and environmental factors, and further build a framework for the potential impact of built environment on acne for the future research.
Search Strategies and Selection of Studies
We searched all publications included in the electronic databases of PubMed, Google Scholar and Web of Science (from 2000–present). The search stratagem used the term “acne vulgaris (or acne),” with the following combinations: epidemiology, prevalence, Propionibacterium acnes (or P. Acnes), sociology of population, gender, age, hormones, diet, sweets, milk, dairy, greasy, dairy products, spicy, chocolate, glycaemic index, smoking, tobacco, cosmetics, electronic productors, overweight, obesity, mental health, mental disorder, stress, economic, skin type, menstrual cycle, exposure, climate, environment, temperature, humidity, sun, pollution, chloracne, social environment, social network, social media, and built environment. Furthermore, in order to avoid missing relevant literature, we also reviewed the reference lists of the identified papers and manually searched for additional publications. Next, we evaluated the title and abstract of each article based on the inclusion criteria. The full text review was then conducted to determine whether the article met all criteria. Inclusion criteria included: (1) Being written in English, (2) Epidemiological studies of acne. Exclusion criteria included: (1) respondents with systemic disorders (such as cardiovascular, respiratory, urinary, reproductive and endocrine diseases, etc.) 2 . (2) studies that did not focus on acne. After searching the literature, there were few relevant studies on built environment and acne, only one study about built environment and skin cancer was found. Research found that the occurrence of acne was strongly attributed to the exposure of skin in the natural environment, the obesity and psychological issues, which could be affected by the built environment factors. In order to establish an indirect relationship between built environment and acne, the search stratagem also used the term “built environment,” with the following combinations: obesity, overweight, mental health, anxiety, depression and suicide. Inclusion criteria included: (1) Research written in English, (2) original articles. Exclusion criteria included: studies that did not have a significant focus on built environment and obesity, mental health. We initially selected 158 studies based on the titles and abstracts. After reading the full texts, a total of 80 articles met all the criteria and were included in the review. All the 80 studies identified were quantitative ( Figure 1 ). Sample size ranged from 50 to 2472004.
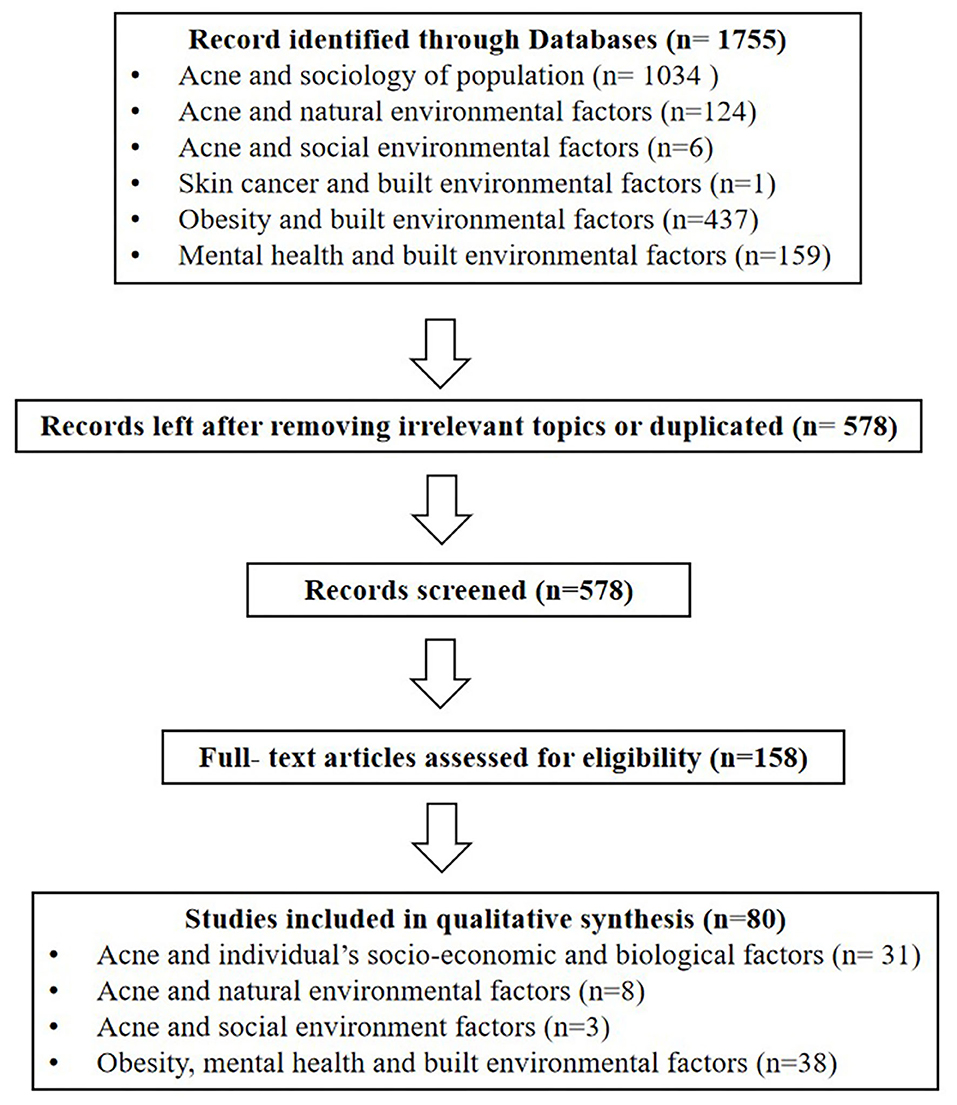
Figure 1 . Illustrates the study inclusion and exclusion process.
The factors that affect acne are classified into four main categories: individual's socio-economic and biological factors, natural environmental factors, social environmental factors and built environmental factors.
Individual's Socio-Economic and Biological Factors
With the rapid urbanization process, there are large-scale migrating and aging populations, changes in dietary structure and lifestyle, and social inequality, leading to a high incidence of chronic diseases ( 20 ). As one of the highly recurrent chronic diseases, acne to a large extent is also affected by the relevant demographic and sociological factors, including demographic characteristics, physiological factors, lifestyle and psychological factors ( Figure 2 , Table 1 in Appendix ).
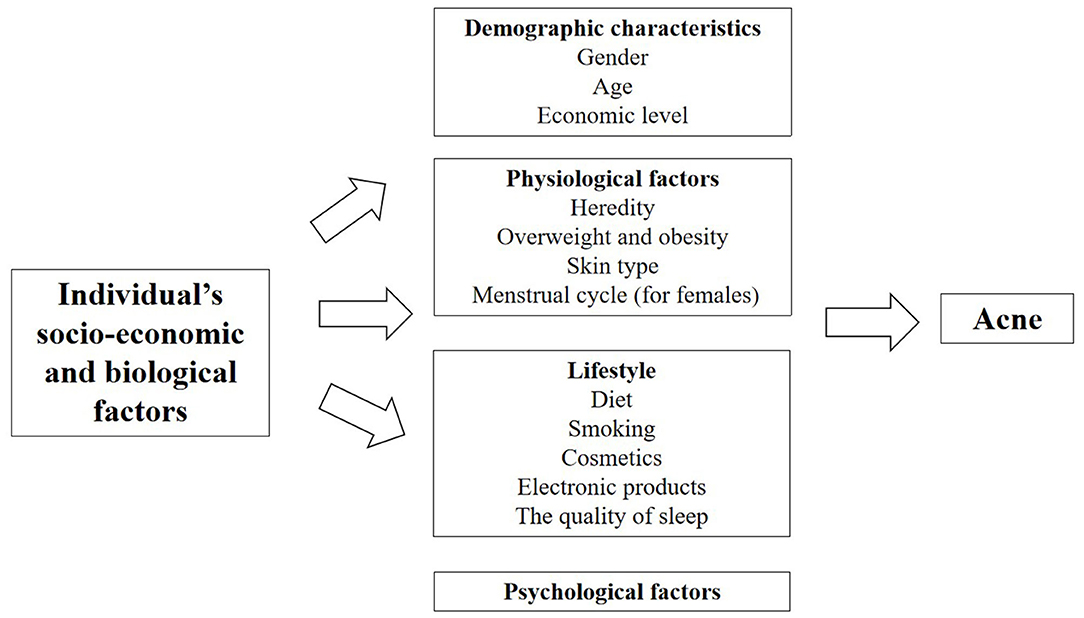
Figure 2 . Factors of individual's socio-economic and biological factors affecting acne.
There are differences in endocrine levels between the genders, resulting in differences in the prevalence of acne. The epidemiological survey of acne among undergraduates in the North East China showed that the total prevalence of acne among adolescents was 51.30% (52.74% in males, 49.65% in females) ( 21 ). The overall prevalence of acne among European aged 15–24 years was 57.8% (58.28% in males, 56.97% in females) ( 22 ). An epidemiology in Singapore showed there were more males than females suffering from adolescence acne (61.3 vs. 38.8%) and more females suffering from post-adolescence acne (69.0 vs. 31.0%) ( 23 ). The above studies indicated that more males than females suffered from acne during adolescence and more females than males suffered from acne during post-adolescence.
The epidemiology of acne continues to evolve with changes in hormone levels that vary with age. An Italian study of pediatric outpatients aged 9–14 found that 34.3% patients had acne, with the lowest prevalence rate of 6% at age 9, and the incidence of acne increased to 36.3% after the age of 13 ( 24 ). From the prevalence of acne among Chinese adolescents, we found increased age was related to higher prevalence and severity of acne vulgaris: 15.6, 44.9, and 70.4% for 10, 13, and 16 years old ( 25 ). The European study showed the prevalence of acne was highest at the age of 15–17 and decreased with age ( 22 ). These studies confirmed that acne was more common during adolescence.
Economic Level
According to family income and regional characteristics, urban residents can be divided into poverty, low-middle income, middle-high income and wealthy groups ( 26 ). There were differences in the medical services enjoyed by patients at different economic levels, which might affect the prevalence of acne. According to the Canadian study, only 17% of low-income people earning <$ 20,000 referred a dermatologist, while 24% of high-income people earning more than $ 80,000 consulted a dermatologist ( 27 ). Furthermore, there are differences in the prevalence of acne between urban and rural areas. Dreno et al. found acne patients were more likely to live in urban areas with higher socio-economic status ( 28 ).
In clinical work, children of acne patients tend to suffer from acne. Heredity plays a dominant role in the occurrence of acne, especially in severe acne with nodules, cysts and scars. A study of twin models in the UK found that 81% acne variants were caused by genetics and family history, proving that acne have a significant genetic effect ( 17 ). An Italian study found that moderate to severe acne is closely related to family history of first-degree relatives ( 29 ). An epidemiological study in Iran also showed that the severity of risk of acne increased with the number of family members with acne history, especially a mother with acne history had the greatest impact on acne severity of next generation ( 30 ). Studies in China ( 21 ) and Europe ( 22 ) have found similar results. Other studies demonstrated that the family history of acne was associated with early onset of acne, more skin lesions, and difficult treatment ( 31 ). He et al. conducted on a cohort study in the Han population and found that identify two new susceptibility loci at 11p11.2 (damage-specific DNA binding protein 2) and 1q24.2 (selectin L) that are involved in androgen metabolism, inflammation processes and scar formation in severe acne ( 32 ).
Overweight and Obesity
Obesity has become a global public health crisis. In China, 46% of adults and 15% of children are obese or overweight. There is a significant relationship between the growing prevalence of obesity and chronic diseases ( 33 ). Increased secretion of the insulin-like growth factors−1 (IGF-1) in the body ( 34 , 35 ) and insulin resistance are possible mechanisms by which obesity affects the occurrence of acne ( 36 ). In insulin resistance, decreased sensitivity leads to increased insulin release, which in turn leads to increased production of IGF-1 ( 34 ). There were studies demonstrated that overweight and obesity (Body Mass Index, BMI ≥ 25 kg/m 2 ) were positively correlated with an increased risk of acne ( 37 – 39 ). However, a study in Taiwan, China, indicated that BMI was negatively associated with the number of acne lesions from moderate to severe post-adolescent acne among Taiwanese women aged between 25 and 45 years ( 40 ). Recently a nationwide study of 600,404 adolescents indicated overweight and obesity were inversely associated with acne in a dose-dependent manner. In this case, the proportion of adolescents with acne decreased gradually from the underweight to the severely obese group (males, from 19.9 to 13.9%; females, from 16.9 to 11.3%) ( 41 ). However, the study had a limitation about the missing information on potential confounders and acne severity. Therefore, the correlation between obesity and acne should be further explored by controlling other influencing factors.
Increased sebum production is key factor with interrelated mechanisms, previous study found the sebum level of face was more in population with acne than without acne ( 42 , 43 ). Excessive sebum secretion is characterized by oily or mixed skin. In addition, Choi indicated the casual sebum level was positively correlated with the number of acne lesions ( 44 ). The epidemiology found oily skin and mixed type skin were risk factors to the acne ( 21 , 25 ).
Menstrual Cycle (for Females)
Acne in women is frequently associated with hormonal derangement, including hyperandrogenism. Shrestha et al. showed hormonal alteration in females with adult acne had significant association with irregular menstruation ( 45 ). Stoll et al. found 44% of women with acne aggravated in premenstrual period ( 46 ). Ghodsi et al. also reported the premenstrual phase was recognized as risk factors for moderate to severe acne ( 30 ). In addition, Wei et al. indicated dysmenorrhea was a risk factor to the acne suffers ( 21 ). Therefore, dermatologists should consider hormonal alterations in acne patients with irregular menstruation.
The relationship between diet and acne has been a hot topic in the research of acne epidemiology. At present, many studies have confirmed that high sugar diet and dairy products are risk factors for acne ( 47 ). Increased sugar intake (≥100 g/d), frequent intake (≥7 times per week) of soft drinks (such as carbonated sodas, sweetened tea drinks and fruit-flavored drinks), and daily consuming dark chocolate were significantly positively associated with acne ( 30 , 48 – 52 ). High glycemic load diet can lead to the rise in blood glucose in the body, therefore, islets secrete large amounts of insulin to lower blood glucose, and elevated insulin levels lead to increased secretion of insulin-like growth factors-1 (IGF-1), IGF-1 can increase androgen levels, promote sebum secretion, and promote hyperkeratosis of hair follicle sebaceous glands to affect lipid excretion, thereby inducing or aggravating the occurrence of acne ( 53 – 56 ). And there were studies about a positive association between the incidence of acne and the intake of whole milk and skim milk ( 57 , 58 ). Milk can also increase the level of IGF-1, which can lead to acne ( 47 , 59 – 61 ). In addition, acne can be caused by greasy, fatty foods ( 62 , 63 ), due to the fact that the release of free fatty acids by triglycerides under the action of P. acnes could promote the development of acne ( 64 ). However, it is controversial whether spicy food affects acne. The epidemiological survey of college students in North East China showed that spicy food was a risk factor for acne ( 21 ). But other studies have shown that spicy food was not related to the duration or severity of acne ( 30 , 65 ). Since the two studies did not subdivide the types of spicy foods, the relationship between spicy food and acne needs to be further explored.
The relationship between acne and smoking remains controversial. The previous studies found that the prevalence of acne was significantly higher in active smokers than ex-smokers or those who had never smoked ( 7 , 66 ). And the study have also indicated that in contrast to non-smoking group, smokers had significantly higher levels of inflammatory cytokines ( 67 ). However, other studies found that people who smoked regularly showed a significantly lower prevalence of severe acne than non-smokers ( 22 , 68 , 69 ). Therefore, the potential influence and mechanisms between acne and smoking need to be further studied.
An improper use of cosmetics may cause the recurrence of acne, the study indicated there is a significant positive correlation between frequent exposure to cosmetics and the severity of acne in adolescent women ( 70 ). Studies of Latin America and the Iberian Peninsula have shown consistent results ( 71 ). Chinese studies also found cosmetic make-up use was a risk factor of acne ( 25 ). The reason was because improper skin care practices (such as essential oils or too oily substrates, makeup, excessive cleansing of the skin and soaps with pH 8.0) can modify skin barrier function and skin sebum areas, especially the microbiome balance, thereby activating innate immunity to trigger inflammation ( 72 ).
Electronic Products
Visible light emitted by electronic products is a risk factor for acne. Taheri et al. found exposure to short-wavelength visible light emitted from smartphones and tablets could increase the proliferation of Staphylococcus aureus, which could give a rise to an increase incidence of acne ( 73 ). Dreno et al. showed people who exposed to screens and tablets before falling asleep were more likely to have acne ( 28 ). However, using the computer for <2 h a day was considered a protective factor for acne ( 21 ).
The Quality of Sleep
Good sleep is essential to good health, poor sleepers [Pittsburg Sleep Quality Index (PSQI) > 5, sleep duration ≤ 5 h] had significantly higher levels of trans epidermal water loss (TEWL) than good sleepers (PSQI ≤ 5, sleep duration 7–9 h). After tape stripping 3 for 72 h, people with good sleep quality had 30% greater barrier recovery than people with poor sleep. After 24 h of exposure to ultraviolet light, erythema recovery in good sleepers was significantly better ( 74 ). When the skin barrier is damaged, the skin's defense system against external stimuli is weakened, which can further lead to skin diseases, especially acne ( 75 ). Dreno et al. indicated significantly more individuals with acne than without reported lacking sleep ( 28 ). The Chinese study indicate that, sleep duration <8 h per day is a risk factor for acne ( 21 ). Surveys in South Korea ( 76 ) and Japan ( 77 ) have consistent results.
Psychological Factors
With the continuous social and economic changes in the contemporary society, the widening of income gap and the increasing stress, the prevalence of mental disorders in China is as high as 9.3% ( 78 ). Psychological factors induce the release of neuropeptides and hormones that activate cells to participate in the acne issue ( 79 ). The study showed that psychological stress and depression were main risk factors for being acne among college students in the North East China ( 21 ). Dreno et al. indicated individuals with acne suffered from significantly higher stress levels than in acne-free individuals ( 28 ). Epidemiological surveys in Japan ( 77 ), India ( 80 ), and South Korea ( 76 ) all found that stress was an aggravating factor for the cause of acne.
Natural Environmental Factors
The skin is an important organ that is directly exposed to the external environment. It is also the first barrier against the influence of environmental factors. It protects various tissues and organs in the body from physical, chemical and biological harmful factors. Skin participates in the balance adjustment of the whole body and realize the unification with the external environment. In 2018, Dreno et al. studied the effects of environmental exposure on acne and found that with the changing natural and environmental factors, the response and the susceptibility of body skins to natural environment will accordingly change to a different extent ( 18 ). As a consequence of negative impacts on the skin functions, it will increase the occurrence and facilitate the development of acne on people exposed to unfriendly environment ( Figure 3 , Table 2 in Appendix ).
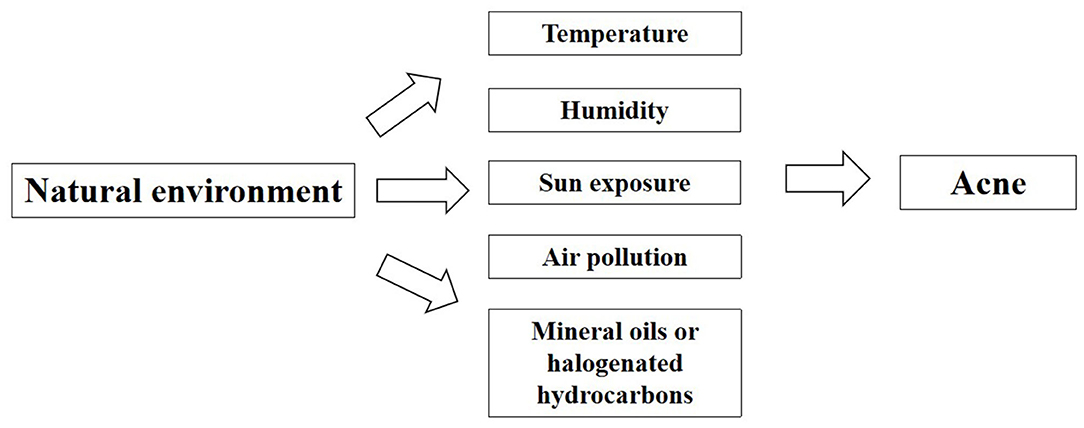
Figure 3 . Factors of natural environment affecting acne.
Temperature and Humidity
Differences in temperature and humidity in different seasons and regions may lead to different prevalence rates of acne. An Indian study found 82 (47.95%) out of 171 acne patients reported seasonal variations in the severity level of acne. It was statistically significant that there were more aggravated acne issues for acne patients in summer (average temperature 32.2°C, average humidity 49.8%) as compared to rainy (average temperature 31.0°C, average humidity 68.5%) and winter season (average temperature 15.1°C, average humidity 79.7%) ( 81 ). The study showed that hot weather was risk factors for acne. However, Dreno et al. found there was no significant difference in prevalence of people with or without acne living in temperate or cold regions. Conversely, acne occurrance was significantly more frequent in hot or humid regions ( 28 ). Williams et al. indicated sebum excretion rate varied with local temperature, that is, sebum excretion rate increased by 10% for every 1°C increase in temperature ( 82 ). A recent study also showed hot environments cause more production of sebum secretion, especially on the forehead ( 83 ). Increased sebum excretion might cause acne to worsening. According to a systematic review and meta-analysis, the prevalence rates of acne in the southern China was higher than that of the northern China, because the southern part is more humid and warmer than the north ( 84 ). A study also showed that the higher the altitude, the lower the prevalence of acne, which may be related to higher altitudes and lower temperature and humidity ( 85 ). The above studies indicated that hot weather might aggravate acne, but further quantitative studies are needed on the relationship between humidity and acne.
Sun Exposure
Sun exposure played a significant role in the incidence of acne. A survey of acne patients in India showed that 26.4% of them developed skin lesions after exposure to sunlight and seasonal variation was observed in 44.5% patients exacerbated, because of increased amount of sunshine exposure in summer months ( 80 ). Dreno et al. found acne was significantly more frequent in individuals with moderate or intensive sun exposure due to their work or daily activities ( 28 ). Lee et al. showed ultraviolet B irradiation increased the expression of inflammatory cytokines in cultured sebocytes ( 86 ).
Air Pollution
Air pollution is the most challenging environmental problem for Chinese cities. According to 2016 report on the state of environment in China, only 84 (25%) of the 338 cities have achieved qualified air quality standards for the living of human beings. Over the past decades, people had become more and more concerned about the living condition of urban environment and the health risks related to the increasing and serious air pollution such asPM 2.5 and PM 10 . Especially, the relevant negative effects of air pollution on the skins have been the key attention of dermatologists and general physicians ( 87 ). Clinical studies reported that air pollutants had a deleterious effect on the skin by increasing oxidative stress, leading to severe change of the normal functions of lipids, deoxyribonucleic acid and/or proteins in the human skin ( 88 ). Two clinical studies comparing subjects in the highly polluted areas to ones in the less polluted areas in Shanghai and Mexico discovered that skin quality declined with chronic exposure to ambient air pollution ( 87 , 89 ). A study in Beijing also indicated that increased concentrations of ambient PM 2.5 , PM10, and NO 2 were positively correlated with numbers of outpatient visits of acne vulgaris over the past 2 years, which further provides an indirect evidence for a link between acne vulgaris and air pollution ( 90 ).
Mineral Oils or Halogenated Hydrocarbons
Chloracne is also known as occupational acne, it is a special type of acne caused by exposure to mineral oils or certain halogenated hydrocarbons in production labor ( 91 ). The increase of cysts in number is a signal of aggravation of chloracne ( 92 ). Dreno et al. found the vast majority of people with acne were significantly more exposed to tar, solvent emanation and crude oil or oil emanation than people without acne ( 28 ). Therefore, an effective way to prevent chloracne is to avoid the contact with halogenated hydrocarbons.
Social Environmental Factors
In addition to natural environment, social environment plays a critical role in the health, behavioral norm and social adaptation of the population as a whole ( Figure 4 , Table 3 in Appendix ).

Figure 4 . Factors of social environment affecting acne.
Social Network
Social network refers to the relatively stable relationship system established by the interaction between different individuals comprising the society, with individuals embedded in the thick webs of social relationships and interactions ( 93 ). Recently, there has been much emphasis on the role that social network plays in our physical health, mental health, social behavior and social adaptation ( 94 ). According to Cohen-Cole et al. found a friend's acne problems increased an individual's odds of having acne problems ( 95 ), which is potentially associated with the similar and interactional living environment, lifestyle and diet among friends. In addition, social networks may have an indirect impact on the occurrence of acne. In recent studies, it has indicated that obesity could spread through various social relationships, which means that the chance of a person developing obesity increases if his or her friend, sibling or spouse becomes obese, suggesting that people embedded in social networks are subject to the influence from the evident appearance and behaviors of those around them ( 96 , 97 ). Moreover, there are a growing number of studies demonstrating that social networks could exert impacts on the psychology and behavior, such as depression, anxiety, smoking, drinking and aggression ( 98 – 100 ). In previous studies, obesity, anxiety, depression, and smoking have been identified as the risk factors for acne ( 21 , 28 , 37 , 66 ). Therefore, it is possible that social networks have impacts on obesity, anxiety, depression, and smoking among peers, thus impacting on acne indirectly. In this regard, if more studies can be conducted to determine the mechanism of social network behind the occurrence and development of acne, the effective means of behavior intervention can be developed in the future.
Social Media
With the rapid advancement of novel technology, social media has made it convenient for patients to communicate their skin diseases, share treatment and skin care, and even get access to the education on their illness. As revealed by Yousaf et al., 45% of the patients resorted to social media for expert advice on acne treatment (54% of women vs. 31% of men), which evidences the influence of social media on acne treatment. Nevertheless, merely 31% of the participants turning to social media made the changes fully compliant with the American Academy of Dermatology (AAD) clinical guidelines ( 101 ). According to Borba et al., the videos of acne education that viewers seek online are clearly inaccurate and poor in quality ( 102 ). The incorrect or irregular treatment suggested on social media may contribute to the aggravation of acne. Therefore, the dermatologist appointment on social media is expected to provide the right information to help educate patients.
Built Environmental Factors
The built environment is human-made or modified surroundings, such as buildings, land use (e.g., layout of communities, transportation systems, infrastructures), or green space ( 12 ). Research has indicated that built environments and health issues are inextricably linked, because exposure factors affects body condition of human beings ( 103 ). Patterns of land development, transportation infrastructure, and building location and design—the built environment could affect the natural environment by replacing natural areas and changing functions and services of ecosystem, which are closely related to the exposure of human beings in the environment.
At present, a growing number of studies have focused on the impact of built environment on health (Table 4 in Appendix ), especially those chronic diseases such as obesity ( 13 ), cardiovascular disease ( 15 ) and mental health ( 14 ). Studies indicated that obesity was positively associated with population density and the availability of fast-food outlets from the people's residence ( 104 – 108 ). Moreover, other studies also found the incidence of cardiovascular disease was significantly higher with more fast-food outlets than areas with no fast-food outlets ( 15 , 109 ). On the contrary, compared with cities with less green space, cities with larger or medium green areas had a lower risk of suicide ( 14 , 110 ). Green plants affect people's psychological function, making them less susceptible to stressful life events, that is, alleviating stress and supporting their reflection on life ( 111 ). In addition, the diversity of resources, ease of access, mobility, personal safety, and street connectivity were closely associated with the higher mental well-being scores among the neighborhoods ( 112 ). Active transport, including walking, cycling and the use of public transport, delivered health benefits in reducing type 2 diabetes and the mortality due to various causes ( 113 ).
All these studies indicated that the built environment played an important role in the incidence of chronic diseases. In the existing research, it has confirmed that built environment exerted some indirect impacts on the health of individuals. Population density, fast food outlets, green spaces and public transport accessibility are exemplified as shown in Figure 5 . With regard to population density, the potential mechanism lying behind the correlation between residential density and overweight may be associated with sedentary behaviors, as indicated by Xu et al. who demonstrated that the participants in higher-density areas spent more time in sedentary behaviors than those in lower-density areas ( 114 ). In terms of fast food, on the one hand, fast food outlets jeopardize the health through high-density fast food restaurants, increasing the chances of eating unhealthy food, frequent fast-food consumption further leads to low nutritional value, excessive sodium intake, increased saturated fat intake, which is linked to cardiovascular disorders, obesity and other metabolic diseases ( 115 – 118 ). While green spaces promote health through four general pathways ( 119 , 120 ). The first pathway is reducing harm (e.g., reducing exposure to air pollution, noise and heat). With increasing outdoor levels of certain greenspace indicators, indoor levels of PM2.5 and noise annoyance are reduced ( 121 , 122 ). The second pathway is restoring capacities (e.g., attention restoration and physiological stress recovery). Viewing plants and other natural environmental features can evoke positive emotions very quickly, thereby shielding negative thoughts and emotions, improving or turning off stress responses ( 111 ). The third pathway is building capacities (e.g., encouraging physical activity). Green spaces may provide a safe, accessible and attractive environment for physical activity ( 123 ). The fourth way is to promote social cohesion. Green spaces provide an environment for contact with neighbors, which may increase social cohesion within the community ( 124 ). In respect of other built environment characteristics for transport, there is evidence that people using public transport are four times more likely to reach the recommended amount of physical activity than ordinary motorists, which is equivalent to an additional 33 min of walking per day ( 125 ), moreover, active travel, particularly walking and cycling, has been recommended because of the health benefits associated with increased physical activity ( 126 ). Increased physical activity is associated with lower body weight ( 127 ).
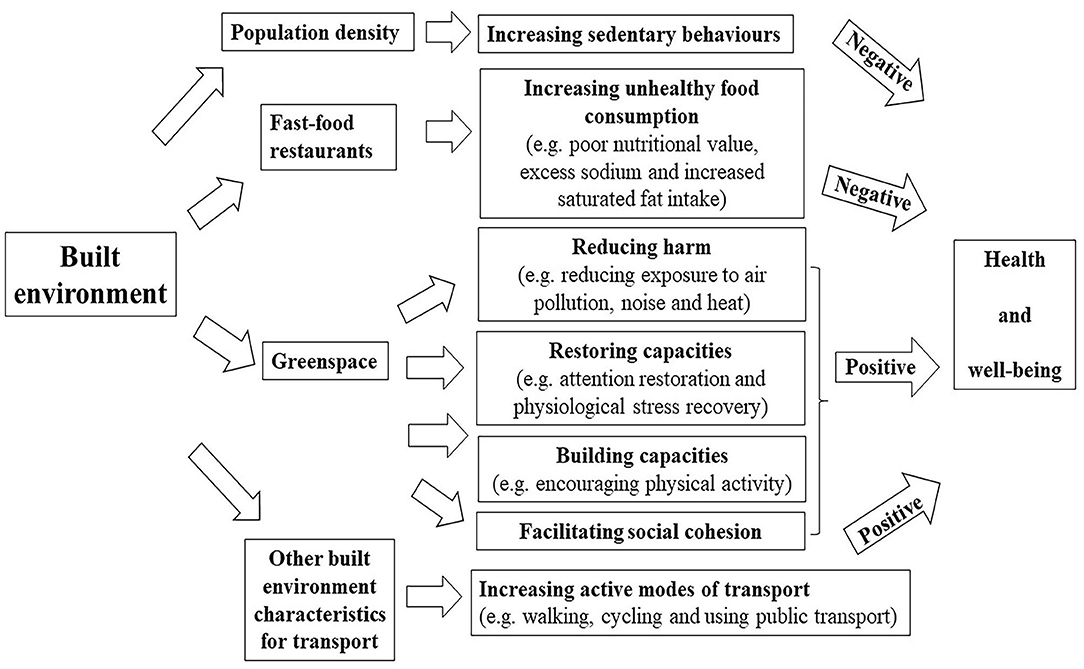
Figure 5 . Pathways linking built environment (fast food restaurant and greenspace) to health outcomes (negative: there is a negative effect; positive: there is a positive effect).
Skin is one of the main interfaces between human body and external environment and is one of the main barriers to prevent pathogens to invade human body. The main function of the skin is to act as a physical barrier to protect our bodies from potential attack by foreign organisms, toxins, or any other external physical, chemical, or organic factors ( 128 ). The built environment may affect the skin through the following mechanisms. Firstly, high population density environment, as a psychosocial stress, induced the impairment of barrier function and water retention property concomitant with decline of ceramide and pyrrolidone carboxylic acid in the stratum corneum ( 129 ). Secondly, Yamane et al. suggested high-fat diet reduces the levels of type I tropocollagen and hyaluronan in the skin by inhibiting the effects of transforming growth factor (TGF)-β1, IGF-I and adiponectin, and these effects are harmful for skin function ( 130 ). In addition, Meeran et al. showed high-fat diet might increase susceptibility to inflammation-related skin diseases, including the risk of skin cancer ( 131 ). So frequent fast-food consumption with high-fat may have a negative impact on the skin. Thirdly, there is increasing evidence that air pollution (e.g., PM 2.5 , PM 10 , NO 2 , SO 2 ) exerts negative effects on the human skin, it may activate cell metabolism and inflammation ( 132 ). Moreover, it has been reported that PM is associated with increased risks of skin diseases, especially skin aging ( 133 ), acne ( 87 ), atopic dermatitis or eczema ( 134 ). Through the above analysis on mechanism, greenspace can reduce exposure to air pollution. Accordingly, the reduction of pollutants is a protective factor for the skin, and the occurrence of skin diseases may also be reduced. Fourthly, psychosocial stress has a negative impact on skin disease by activating the expression of inflammatory cytokines or compromising both permeability barrier homeostasis and stratum corneum integrity ( 135 , 136 ). Thus, greenspace may protect the skin by reducing psychological stress. Lastly, the study have found high physical activity group showed a positive outcome with respect to wrinkles compared to low and middle physical activity group ( 137 ). Therefore, we can guess that greenspace, better public transport accessibility and active travel (e.g., walking and cycling) may be beneficial for reducing wrinkles by providing more space for increasing physical activity.
Acne is a common and chronic inflammatory skin disease, Dreno et al. confirm that internal and external exposome factors had a significant impact on acne ( 28 ). Thus, the built environment is closely related to our lives and may also have a potential impact on acne.
Building the Indirect Relationship Between the Built Environment and Acne
There is still a lack of scientific research on whether the built environment is related to the occurrence of acne. To bridge this knowledge gap, we will explore the indirect relationship between the built environment and acne, and provide a scientific basis for future epidemiological investigations ( Figure 6 ).
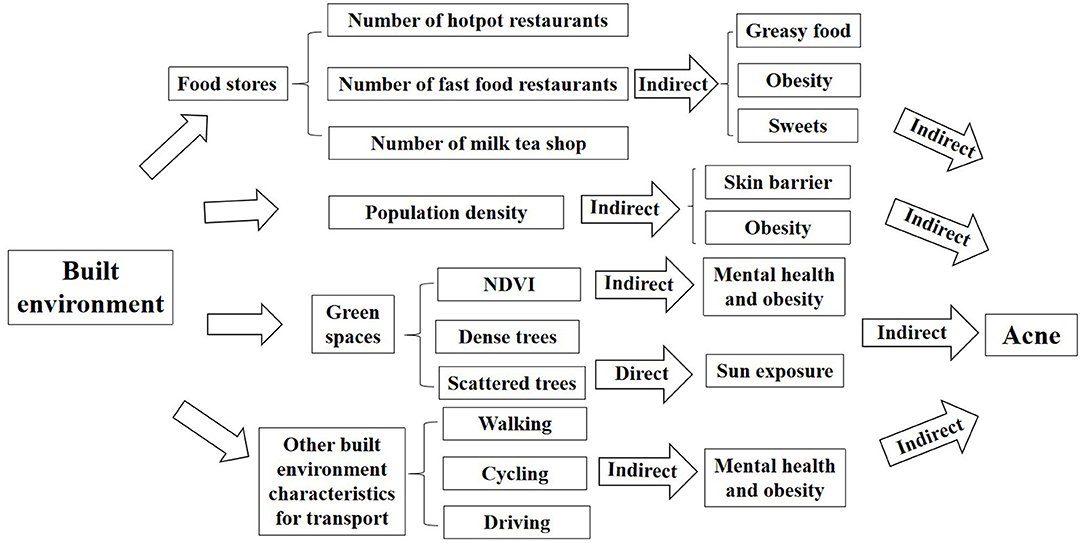
Figure 6 . The framework of impacts of built environment on acne (indirect: there is an indirect effect; direct: there is a direct effect).
Population Density
Population density refers to the average number of people living on land per unit area. According to the study of Tsinghua–Lancet commission on healthy cities in China, between 1978 and 2015, China experienced the largest population migration from rural areas to cities in human history. The urbanization rate increased from 17.9 to 56.1%, and the urban population also increased from 170 to 771 million ( 1 ). An epidemiological survey in Asia has found an increase in the prevalence of diseases in areas with high population density ( 138 ). Xu et al. indicated residential density was positively associated with being overweight among urban Chinese adolescents ( 114 ). High density can increase obesity, which may lead to acne. In addition, an animal study found that high population density in mice damaged skin barrier function and TEWL ( 129 ). When the skin barrier was damaged, the skin defense against external exposure was weakened, which could lead to acne ( 75 ). However, whether population density affects acne is unknown, the correlation between population density and acne needs further study.
Food Stores
On the one hand, in summary of the factors affecting acne, we found that greasy food, spicy food, dairy products, and sweets are risk factors for acne. So hotpot restaurants and milk tea shops around residents' residences may indirectly affect acne, because a higher number of hotpot restaurants and milk tea shops around the location people work and live, it may be more likely to provide greasy food, spicy food, dairy products, and sweets to people, which could largely increase the possibility of people being acne. On the other hand, obesity is a risk factor for acne, which implies that types and numbers of restaurants around the residential and work location of people could be related to their obesity. A number of studies in the US ( 139 ), New York ( 105 ), Utah ( 106 ), the UK ( 13 , 140 ), Porto ( 104 ), New Orleans ( 141 ), and China ( 142 ) showed higher fast food restaurant density was significantly associated with higher obesity rates among students. Therefore, an increased number of fast food restaurants near the address may increase the risk of obesity, which may indirectly affect the occurrence of acne. The number of fast food restaurants near the address is one of the measurement indexes of built environment, but whether it affects acne, needs empirical studies to verify.
Green Spaces
Green spaces encourage people to spend more outdoor time for sports, entertainment and social activities. It could have unexpected but important consequences for health in countries with very high levels of ultraviolet (UV) radiation, because sun exposure is one of the risk factors for acne ( 28 ). A study in Australia showed that compared to people with 0–20% green space, those with 80% green space had a 9% higher chance for skin cancer. Because people who live near green spaces have higher exposure to outdoor environments and the incidence of skin cancer increases accordingly ( 143 ). Different types of green spaces may affect acne, for instance dense trees could reduce UV radiation and therefore protect skin by providing shade, while scattered trees are less protective of skin because they cannot block UV radiation ( 144 ). Moreover, there is growing evidence that natural outdoor environments, such as green spaces (i.e., grass, forests, or parks) was increasingly shown to promote mental health. A study in the Netherlands found cities with a large proportion of green space (>85%) or a moderate proportion of green space (>25% to ≤85%) had a lower suicide risk than cities with less green space (≤25%) ( 14 ). Epidemiology research have confirmed that depression and stress are important factors of acne, which means that green space may indirectly affect the occurrence of acne by affecting mental health. According to a study in South Africa, each participant was assigned a value for a green living space, which was obtained from a normalized difference vegetation index (NDVI) generated by a satellite based on the global positioning system (GPS) coordinates of their household location ( 110 ). Liu et al. suggested sufficient green infrastructure at the neighborhood scale could protect against depression and promote mental well-being in Chinese urban settings ( 145 – 150 ). Furthermore, green space may be associated with decreased risk of excess weight/obesity ( 151 – 153 ). Therefore, an increase in the proportion of green space near the living and working places may encourage people to participate in more outdoor activities so that decrease the risk of stress, depression and obesity, which may indirectly decrease the occurrence of acne. Green space is one of the measurement indexes of built environment, but its effect on skin, especially acne, needs to be verified by empirical studies.
Other Built Environment Characteristics for Transport
Land-use diversity and street connectivity can influence the choice over transport mode, which in turn affects health ( 154 ). In previous studies, it has been demonstrated that a reduction to the distances to public transport could reduce motorization, which means a modal shift from private motorized vehicles to walking, cycling, and public transport can help improve physical health for all urban residents, for example, reducing obesity, diabetes, cardiovascular disease and respiratory diseases ( 155 – 157 ). Then, the characteristics of built environment for transport may also have impacts on acne indirectly. On the one hand, improving accessibility to public transport will improve population health by promoting the engagement in physical activities ( 158 ). A European study was conducted to demonstrate that BMI dropped when people started or increased cycling, but increased when car was used, suggesting the health benefits were created by active mobility ( 159 ). Those preferring walking or cycling exhibited a lower BMI over time than those using cars on a long-term basis ( 160 ). Additionally, Liao et al. found out that Taiwanese adults mainly reliant on public transport for travel showed a higher likelihood of engagement in transport-related physical activity and a lower level of risk of developing obesity than those who traveled by walking, cycling, or private vehicles ( 161 ). On the other hand, different transport modes had different impacts on mental health. Cycling and walking were linked to the positive self-perception about health ( 162 ). However, the commute by car has been associated with high stress and lower mental well-being ( 163 , 164 ). In general, people walking or cycling as a frequent means of commute may have lower BMI and better mental state, which may contribute indirectly to reducing the occurrence of acne, regarding that obesity, psychological stress and depression are the risk factors for acne. Thus, reducing obesity and improving mental health may help reduce the incidence of acne. While the commute by car might produce the opposite result. However, the correlation between the characteristics of built environment for transport and acne may also be influenced by other potential factors, for example, walking without the protection against solar radiation may increase sun exposure, which will aggravate acne as well. Therefore, further research is required to confirm the potential relationship between them.
Strengths and Limitations
The core merit of our paper lies in a thorough review of the relevant socio-economic, biological and environmental factors that could impact on acne as well as of the underlying mechanisms. Based on that, the direct and indirect relationships were established between built environmental factors (population density, food stores, social network, transport mode, land use) and acne by reviewing the effects of built environment on health, thus providing a theoretical basis for a further research on the effective means of behavior intervention.
It is essential to acknowledge the weaknesses of the current study, that is, there remain few studies focusing on the impact of built environment on acne. In this situation, attempt was made in our study to determine the indirect relationship between built environment and acne by exploring its impact on obesity and psychology. It was possible to miss some information about the potential confounders impacts on acne.
Conclusions
Acne refers to a polygenic genetic disorder affected by the interaction between genetic and environmental factors. In this study, it was concluded that acne mostly occurs during adolescence, with age and gender playing a significant role in its occurrence. Besides, the prevalence of acne showed a decreasing trend with age. Males outnumbered females in terms of adolescence acne while it was the opposite in terms of post-adolescence acne. Moreover, acne can be affected negatively by such influencing factors such as family history, overweight, obesity, oily and mixed skin, irregular menstrual cycles, sweet food, greasy food, dairy products, smoking, the improper use of cosmetics, the long-term use of electronics, the poor quality of sleep and stress. In addition, environmental factors play a crucial role, along with various natural environmental factors, including temperature, sun exposure, air pollution, mineral oils and halogenated hydrocarbons, serve as risk factors for acne. Moreover, a further qualitative research is required to figure out the impact of humidity on acne. Lastly, social networks and social media can affect acne as well.
However, the impact of built environment on acne has yet to be reported in previous studies. Thus, an attempt was made in this study to determine the indirect relationships between built environment and acne regarding the impacts of built environment on the risk factors for acne. To cure such a chronic disease, it is necessary to understand the indirect relationship between the built environment and acne by gaining understanding as to the impact of traditional factors on the pathogenesis of acne.
In the future, the study conducted from the perspectives of medicine, sociology of population and geography will be required, and more empirical studies are required to reveal the specific relationship between built environment and acne. The potential built environmental factors for acne ought to be analyzed by collecting the data on demographic characteristics, physiological factors, lifestyle, psychological factors, as well as population density, food stores, green space, climate, pollution status, and so on in relation to the residence of patients with acne, in combination with traditional research factors. In doing so, the impact of built environmental factors on acne can be fully understood to provide specific guidance on reducing the prevalence of acne.
Author Contributions
JY, HY, and LH were equally responsible for writing, editing, and literature review. All authors were involved in manuscript preparation, approved the final version, and agreed to be accountable for all aspects of the work.
This work was supported by the Strategic Priority Research Program (A) of Chinese Academy of Sciences (XDA19040402), Program for Innovative Research Team, the Ministry of Education of China (IRT17-R49), Major science and technology projects in Yunnan Province China (2018ZF005), Science and Technology Leadership Talent Project in Yunnan China (2017HA010), and Clinical Medical Center in Yunnan Province China (ZX2019-03-02).
Conflict of Interest
The authors declare that the research was conducted in the absence of any commercial or financial relationships that could be construed as a potential conflict of interest.
Supplementary Material
The Supplementary Material for this article can be found online at: https://www.frontiersin.org/articles/10.3389/fpubh.2020.00450/full#supplementary-material
1. ^ Spironolactone is $350, oral antibiotics are $501, topical antibiotics are $920, topical retinoids are $1,805, topical combination antibiotics are $2,282, topical combination antibiotics and retinoids are $3,770, the median cost for isotretinoin in males and females are $3,227 and $3,806 respectively.
2. ^ If the topic of studies was not only associated with acne but also other diseases, there might exist confounding effects issues in between.
3. ^ Applying tape to disrupt the skin barrier.
1. Yang J, Siri JG, Remais JV, Cheng Q, Zhang H, Chan KKY, et al. The Tsinghua-Lancet Commission on Healthy Cities in China: unlocking the power of cities for a healthy China. Lancet. (2018) 391:2140–84. doi: 10.1016/S0140-6736(18)30486-0
PubMed Abstract | CrossRef Full Text | Google Scholar
2. Williams HC, Dellavalle RP, Garner S. Acne vulgaris. Lancet. (2012) 379:361–72. doi: 10.1016/S0140-6736(11)60321-8
3. Dreno B. What is new in the pathophysiology of acne, an overview. J Eur Acad Dermatol Venereol. (2017) 31 (Suppl. 5):8–12. doi: 10.1111/jdv.14374
4. Vos T, Flaxman AD, Naghavi M, Lozano R, Michaud C, Ezzati M, et al. Years lived with disability (YLDs) for 1160 sequelae of 289 diseases and injuries 1990-2010: a systematic analysis for the Global Burden of Disease Study 2010. Lancet. (2012) 380:2163–96. doi: 10.1016/S0140-6736(12)61729-2
5. GBD 2016 DALYs and HALE Collaborators. Global, regional, and national disability-adjusted life-years (DALYs) for 333 diseases and injuries and healthy life expectancy (HALE) for 195 countries and territories, 1990-2016: a systematic analysis for the Global Burden of Disease Study 2016. Lancet. (2017) 390:1260–344. doi: 10.1016/S0140-6736(17)32130-X
6. Tassavor M, Payette MJ. Estimated cost efficacy of U.S. Food and Drug Administration-approved treatments for acne. Dermatol Ther. (2019) 32:e12765. doi: 10.1111/dth.12765
7. Shen Y, Wang T, Zhou C, Wang X, Ding X, Tian S, et al. Prevalence of acne vulgaris in Chinese adolescents and adults: a community-based study of 17,345 subjects in six cities. Acta Derm Venereol. (2012) 92:40–4. doi: 10.2340/00015555-1164
8. Ritvo E, Del Rosso JQ, Stillman MA, La Riche C. Psychosocial judgements and perceptions of adolescents with acne vulgaris: a blinded, controlled comparison of adult and peer evaluations. Biopsychosoc Med Biopsychosoc Med. (2011) 5:11. doi: 10.1186/1751-0759-5-11
9. Dreno B, Bordet C, Seite S, Taieb C. Acne relapses: impact on quality of life and productivity. J Eur Acad Dermatol Venereol. (2019) 33:937–43. doi: 10.1111/jdv.15419
10. Halvorsen JA, Stern RS, Dalgard F, Thoresen M, Bjertness E, Lien L. Suicidal ideation, mental health problems, and social impairment are increased in adolescents with acne: a population-based study. J Invest Dermatol. (2011) 131:363–70. doi: 10.1038/jid.2010.264
11. Lukaviciute L, Navickas P, Navickas A, Grigaitiene J, Ganceviciene R, Zouboulis CC. Quality of life, anxiety prevalence, depression symptomatology and suicidal ideation among acne patients in Lithuania. J Eur Acad Dermatol Venereol. (2017) 31:1900–6. doi: 10.1111/jdv.14477
12. Rao M, Prasad S, Adshead F, Tissera H. The built environment and health. Lancet. (2007) 370:1111–3. doi: 10.1016/S0140-6736(07)61260-4
13. Mason KE PN, Cummins S. Associations between fast food and physical activity environments and adiposity in mid-life: cross-sectional, observational evidence from UK Biobank. Lancet Public Health. (2018) 3:e24–33. doi: 10.1016/S2468-2667(17)30212-8
14. Helbich M, de Beurs D, Kwan M-P, O'Connor RC, Groenewegen PP. Natural environments and suicide mortality in the Netherlands: a cross-sectional, ecological study. Lancet Planet Health. (2018) 2:e134–9. doi: 10.1016/S2542-5196(18)30033-0
15. Poelman M, Strak M, Schmitz O, Hoek G, Karssenberg D, Helbich M, et al. Relations between the residential fast-food environment and the individual risk of cardiovascular diseases in The Netherlands: A nationwide follow-up study. Eur J Prev Cardiol. (2018) 25:1397–405. doi: 10.1177/2047487318769458
16. Wang L, Sun W, Zhou K, Zhang M, Bao P. Spatial analysis of built environment risk for respiratory health and its implication for urban planning: a case study of Shanghai. Int J Environ Res Public Health. (2019) 16:1455. doi: 10.3390/ijerph16081455
17. Bataille V, Snieder H, MacGregor AJ, Spector TD. The influence of genetics and environmental factors in the pathogenesis of acne: a twin study of acne in women. J Invest Dermatol. (2002) 119:1317–22. doi: 10.1046/j.1523-1747.2002.19621.x
18. Dréno B, Bettoli V, Araviiskaia E, Sanchez Viera M, Bouloc A. The influence of exposome on acne. J Eur Acad Dermatol Venereol. (2018) 32:812–9. doi: 10.1111/jdv.14820
19. Tan JK, Bhate K. A global perspective on the epidemiology of acne. Br J Dermatol. (2015) 172 (Suppl. 1):3–12. doi: 10.1111/bjd.13462
20. Li X, Wang C, Zhang G, Xiao L, Dixon J. Urbanisation and human health in China: spatial features and a systemic perspective. Environ Sci Pollut Res Int. (2012) 19:1375–84. doi: 10.1007/s11356-011-0718-7
21. Wei B, Pang Y, Zhu H, Qu L, Xiao T, Wei HC, et al. The epidemiology of adolescent acne in North East China. J Eur Acad Dermatol Venereol. (2010) 24:953–7. doi: 10.1111/j.1468-3083.2010.03590.x
22. Wolkenstein P, Machovcova A, Szepietowski JC, Tennstedt D, Veraldi S, Delarue A. Acne prevalence and associations with lifestyle: a cross-sectional online survey of adolescents/young adults in 7 European countries. J Eur Acad Dermatol Venereol. (2018) 32:298–306. doi: 10.1111/jdv.14475
23. Han XD, Oon HH, Goh CL. Epidemiology of post-adolescence acne and adolescence acne in Singapore: a 10-year retrospective and comparative study. J Eur Acad Dermatol Venereol. (2016) 30:1790–3. doi: 10.1111/jdv.13743
24. Napolitano M, Ruggiero G, Monfrecola G, Megna M. Acne prevalence in 9 to 14-year-old old patients attending pediatric ambulatory clinics in Italy. Int J Dermatol. (2018) 57:1320–3. doi: 10.1111/ijd.14138
25. Wu TQ, Mei SQ, Zhang JX, Gong LF. Prevalence and risk factors of facial acne vulgaris among Chinese adolescents. Int J Adolesc Med Health. (2007) 19:407–12. doi: 10.1515/IJAMH.2007.19.4.407
26. Anderson GFA, Pittau MG, Zelli R. A new approach to measuring and studying the characteristics of class membership: examining poverty, inequality and polarization in urban China. J Econom. (2015) 191:348–59. doi: 10.1016/j.jeconom.2015.12.006
CrossRef Full Text | Google Scholar
27. Haider A, Mamdani M, Shaw JC, Alter DA, Shear NH. Socioeconomic status influences care of patients with acne in Ontario, Canada. J Am Acad Dermatol. (2006) 54:331–5. doi: 10.1016/j.jaad.2005.03.029
28. Dreno B, Shourick J, Kerob D, Bouloc A, Taïeb C. The role of exposome in acne: results from an international patient survey. J Eur Acad Dermatol Venereol. (2019) 34:1057–64. doi: 10.1111/jdv.16119
29. Di Landro A, Cazzaniga S, Parazzini F, Ingordo V, Cusano F, Atzori L, et al. Family history, body mass index, selected dietary factors, menstrual history, and risk of moderate to severe acne in adolescents and young adults. J Am Acad Dermato. (2012) 67:1129–35. doi: 10.1016/j.jaad.2012.02.018
30. Ghodsi SZ, Orawa H, Zouboulis CC. Prevalence, severity, and severity risk factors of acne in high school pupils: a community-based study. J Invest Dermatol. (2009) 129:2136–41. doi: 10.1038/jid.2009.47
31. Ballanger F, Baudry P, N'Guyen JM, Khammari A, Dréno B. Heredity: a prognostic factor for acne. Dermatology. (2006) 212:145–9. doi: 10.1159/000090655
32. He L, Wu WJ, Yang JK, Cheng H, Zuo XB, Lai W, et al. Two new susceptibility loci 1q24.2 and 11p11.2 confer risk to severe acne. Nat Commun. (2014) 5:2870. doi: 10.1038/ncomms3870
33. Wang Y, Xue H, Sun M, Zhu X, Zhao L, Yang Y. Prevention and control of obesity in China. Lancet Glob Health . (2019) 7:e1166–7. doi: 10.1016/S2214-109X(19)30276-1
34. Clatici VG, Voicu C, Voaides C, Roseanu A, Icriverzi M, Jurcoane S. Diseases of civilization - cancer, diabetes, obesity and acne - the implication of milk, IGF-1 and mTORC1. Maedica. (2018) 13:273–81. doi: 10.26574/maedica.2018.13.4.273
35. Kumari R, Thappa DM. Role of insulin resistance and diet in acne. Indian J Dermatol Venereol Leprol. (2013) 79:291–9. doi: 10.4103/0378-6323.110753
36. Chen W, Obermayer-Pietsch B, Hong JB, Melnik BC, Yamasaki O, Dessinioti C, et al. Acne-associated syndromes: models for better understanding of acne pathogenesis. J Eur Acad Dermatol Venereol. (2011) 25:637–46. doi: 10.1111/j.1468-3083.2010.03937.x
37. Halvorsen JA, Vleugels RA, Bjertness E, Lien L. A population-based study of acne and body mass index in adolescents. Arch Dermatol. (2012) 148:131–2. doi: 10.1001/archderm.148.1.131
38. Cesko E, Korber A, Dissemond J. Smoking and obesity are associated factors in acne inversa: results of a retrospective investigation in 100 patients. Eur J Dermatol. (2009) 19:490–3. doi: 10.1684/ejd.2009.0710
39. Karciauskiene J, Valiukeviciene S, Gollnick H, Stang A. The prevalence and risk factors of adolescent acne among schoolchildren in Lithuania: a cross-sectional study. J Eur Acad Dermatol Venereol. (2014) 28:733–40. doi: 10.1111/jdv.12160
40. Lu PH, Hsu CH. Body mass index is negatively associated with acne lesion counts in Taiwanese women with post-adolescent acne. J Eur Acad Dermatol Venereol. (2015) 29:2046–50. doi: 10.1111/jdv.12754
41. Snast I, Dalal A, Twig G, Astman N, Kedem R, Levin D, et al. Acne and obesity: a nationwide study of 600,404 adolescents. J Am Acad Dermatol. (2019) 81:723–9. doi: 10.1016/j.jaad.2019.04.009
42. Kim MK, Choi SY, Byun HJ, Huh CH, Park KC, Patel RA. Comparison of sebum secretion, skin type, pH in humans with and without acne. Arch Dermatol Res. (2006) 298:113–9. doi: 10.1007/s00403-006-0666-0
43. Pappas A, Johnsen S, Liu JC, Eisinger M. Sebum analysis of individuals with and without acne. Dermatoendocrinol. (2009) 1:157–61. doi: 10.4161/derm.1.3.8473
44. Choi CW, Choi JW, Park KC, Youn SW. Facial sebum affects the development of acne, especially the distribution of inflammatory acne. J Eur Acad Dermatol Venereol. (2013) 27:301–6. doi: 10.1111/j.1468-3083.2011.04384.x
45. Shrestha S. Correlation of hormonal profile and lipid levels with female adult acne in a tertiary care center of Nepal. J Nepal Health Res Counc. (2018) 16:222–7. doi: 10.3126/jnhrc.v16i2.20315
46. Stoll S, Shalita AR, Webster GF, Kaplan R, Danesh S, Penstein A. The effect of the menstrual cycle on acne. J Am Acad Dermatol. (2001) 45:957–60. doi: 10.1067/mjd.2001.117382
47. Melnik BC, Schmitz G. Role of insulin, insulin-like growth factor-1, hyperglycaemic food and milk consumption in the pathogenesis of acne vulgaris. Exp Dermatol. (2009) 18:833–41. doi: 10.1111/j.1600-0625.2009.00924.x
48. Burris J, Rietkerk W, Woolf K. Relationships of self-reported dietary factors and perceived acne severity in a cohort of New York young adults. J Acad Nutr Diet. (2014) 114:384–92. doi: 10.1016/j.jand.2013.11.010
49. Huang X, Zhang J, Li J, Zhao S, Xiao Y, Huang Y, et al. Daily intake of soft drinks and moderate-to-severe acne vulgaris in chinese adolescents. J Pediatr. (2019) 204:256–62. doi: 10.1016/j.jpeds.2018.08.034
50. Vongraviopap S, Asawanonda P. Dark chocolate exacerbates acne. Int J Dermatol . (2016) 55:587–91. doi: 10.1111/ijd.13188
51. Delost GR, Delost ME, Lloyd J. The impact of chocolate consumption on acne vulgaris in college students: a randomized crossover study. J Am Acad Dermatol. (2016) 75:220–2. doi: 10.1016/j.jaad.2016.02.1159
52. Aksu AE, Metintas S, Saracoglu ZN, Gurel G, Sabuncu I, Arikan I, et al. Acne: prevalence and relationship with dietary habits in Eskisehir, Turkey. J Eur Acad Dermatol Venereol. (2012) 26:1503–9. doi: 10.1111/j.1468-3083.2011.04329.x
53. Smith RN, Mann NJ, Braue A, Makelainen H, Varigos GA. The effect of a high-protein, low glycemic-load diet versus a conventional, high glycemic-load diet on biochemical parameters associated with acne vulgaris: a randomized, investigator-masked, controlled trial. J Am Acad Dermatol. (2007) 57:247–56. doi: 10.1016/j.jaad.2007.01.046
54. Çerman AA, Aktaş E, Altunay IK, Arici JE, Tulunay A, Ozturk FY. Dietary glycemic factors, insulin resistance, and adiponectin levels in acne vulgaris. J Am Acad Dermatol. (2016) 75:155–62. doi: 10.1016/j.jaad.2016.02.1220
55. Melnik BC. Diet in acne: further evidence for the role of nutrient signalling in acne pathogenesis. Acta Derm Venereol. (2012) 92:228–31. doi: 10.2340/00015555-1358
56. Smith RN, Mann NJ, Braue A, Mäkeläinen H, Varigos GA. A low-glycemic-load diet improves symptoms in acne vulgaris patients: a randomized controlled trial. Am J Clin Nutr. (2007) 86:107–15. doi: 10.1093/ajcn/86.1.107
57. Adebamowo CA, Spiegelman D, Danby FW, Frazier AL, Willett WC, Holmes MD. High school dietary dairy intake and teenage acne. J Am Acad Dermatol. (2005) 52:207–14. doi: 10.1016/j.jaad.2004.08.007
58. Aalemi AK, Anwar I, Chen H. Dairy consumption and acne: a case control study in Kabul, Afghanistan. Clin Cosmet Investig Dermatol. (2019) 12:481–7. doi: 10.2147/CCID.S195191
59. Melnik BC. Evidence for acne-promoting effects of milk and other insulinotropic dairy products. Nestle Nutr Workshop Ser Pediatr Program . (2011) 67:131–45. doi: 10.1159/000325580
60. Melnik B. Milk consumption: aggravating factor of acne and promoter of chronic diseases of Western societies. J Dtsch Dermatol Ges. (2009) 7:364–70. doi: 10.1111/j.1610-0387.2009.07019.x
61. Rahaman SMA, De D, Handa S, Pal A, Sachdeva N, Ghosh T, et al. Association of insulin-like growth factor (IGF)-1 gene polymorphisms with plasma levels of IGF-1 and acne severity. J Am Acad Dermatol. (2016) 75:768–73. doi: 10.1016/j.jaad.2016.05.019
62. Nguyen QG, Markus R, Katta R. Diet and acne: an exploratory survey study of patient beliefs. Dermatol Pract Concept. (2016) 6:21–7. doi: 10.5826/dpc.0602a05
63. Abo El-Fetoh NM, Alenezi NG, Alshamari NG, Alenezi OG. Epidemiology of acne vulgaris in adolescent male students in Arar, Kingdom of Saudi Arabia. J Egypt Public Health Assoc. (2016) 91:144–9. doi: 10.1097/01.EPX.0000492401.39250.62
64. Akaza N, Akamatsu H, Numata S, Matsusue M, Mashima Y, Miyawaki M, et al. Fatty acid compositions of triglycerides and free fatty acids in sebum depend on amount of triglycerides, and do not differ in presence or absence of acne vulgaris. J Dermatol. (2014) 41:1069–76. doi: 10.1111/1346-8138.12699
65. El Darouti MA, Zeid OA, Abdel Halim DM, Hegazy RA, Kadry D, Shehab DI, et al. Salty and spicy food; are they involved in the pathogenesis of acne vulgaris? A case controlled study. J Cosmet Dermatol. (2016) 15:145–9. doi: 10.1111/jocd.12200
66. Schäfer T, Nienhaus A, Vieluf D, Berger J, Ring J. Epidemiology of acne in the general population: the risk of smoking. Br J Dermatol. (2001) 145:100–4. doi: 10.1046/j.1365-2133.2001.04290.x
67. Yang YS, Lim HK, Hong KK, Shin MK, Lee JW, Lee SW, et al. Cigarette smoke-induced interleukin-1 alpha may be involved in the pathogenesis of adult acne. Ann Dermatol. (2014) 26:11–6. doi: 10.5021/ad.2014.26.1.11
68. Klaz I, Kochba I, Shohat T, Zarka S, Brenner S. Severe acne vulgaris and tobacco smoking in young men. J Invest Dermatol. (2006) 126:1749–52. doi: 10.1038/sj.jid.5700326
69. Wolkenstein P, Misery L, Amici J-M, Maghia R, Branchoux S, Cazeau C, et al. Smoking and dietary factors associated with moderate-to-severe acne in French adolescents and young adults: results of a survey using a representative sample. Dermatology. (2015) 230:34–9. doi: 10.1159/000366195
70. Perera MPN, Peiris W, Pathmanathan D, Mallawaarachchi S, Karunathilake IM. Relationship between acne vulgaris and cosmetic usage in Sri Lankan urban adolescent females. J Cosmet Dermatol. (2018) 17:431–6. doi: 10.1111/jocd.12431
71. Kaminsky A, Florez-White M, Bagatin E, Arias M. Large prospective study on adult acne in Latin America and the Iberian Peninsula: risk factors, demographics, and clinical characteristics. Int J Dermatol. (2019) 58:1277–82. doi: 10.1111/ijd.14441
72. Levin J. The relationship of proper skin cleansing to pathophysiology, clinical benefits, and the concomitant use of prescription topical therapies in patients with acne vulgaris. Dermatol Clin. (2016) 34:133–45. doi: 10.1016/j.det.2015.11.001
73. Taheri M, Darabyan M, Izadbakhsh E, Nouri F, Haghani M, Mortazavi SAR, et al. Exposure to visible light emitted from smartphones and tablets increases the proliferation of Staphylococcus aureus : can this be linked to acne? J Biomed Phys Eng. (2017) 7:163–8. doi: 10.22086/jbpe.v7i2%20Jun.651
74. Oyetakin-White P, Suggs A, Koo B, Matsui MS, Yarosh D, Cooper KD, et al. Does poor sleep quality affect skin ageing? Clin Exp Dermatol. (2015) 40:17–22. doi: 10.1111/ced.12455
75. Fabbrocini G, Rossi AB, Thouvenin MD, Peraud C, Mengeaud V, Bacquey A, et al. Fragility of epidermis: acne and post-procedure lesional skin. J Eur Acad Dermatol Venereol. (2017) 31 (Suppl. 6):3–18. doi: 10.1111/jdv.14410
76. Suh DH, Kim BY, Min SU, Lee DH, Yoon MY, Kim NI, et al. A multicenter epidemiological study of acne vulgaris in Korea. Int J Dermatol. (2011) 50:673–81. doi: 10.1111/j.1365-4632.2010.04726.x
77. Kubota Y, Shirahige Y, Nakai K, Katsuura J, Moriue T, Yoneda K. Community-based epidemiological study of psychosocial effects of acne in Japanese adolescents. J Dermatol. (2010) 37:617–22. doi: 10.1111/j.1346-8138.2010.00855.x
78. Huang Y, Wang Y, Wang H, Liu Z, Yu X, Yan J, et al. Prevalence of mental disorders in China: a cross-sectional epidemiological study. Lancet Psychiatry. (2019) 6:211–24. doi: 10.1016/S2215-0366(18)30511-X
79. Ganceviciene R, Böhm M, Fimmel S, Zouboulis CC. The role of neuropeptides in the multifactorial pathogenesis of acne vulgaris. Dermatoendocrinol. (2009) 1:170–6. doi: 10.4161/derm.1.3.8496
80. George RM, Sridharan R. Factors aggravating or precipitating acne in indian adults: a hospital-based study of 110 cases. Indian J Dermatol. (2018) 63:328–31. doi: 10.4103/ijd.IJD_565_17
81. Narang I, Sardana K, Bajpai R, Garg VK. Seasonal aggravation of acne in summers and the effect of temperature and humidity in a study in a tropical setting. J Cosmet Dermatol. (2019) 18:1098–104. doi: 10.1111/jocd.12777
82. Williams M, Cunliffe WJ, Williamson B, Forster RA, Cotterill JA, Edwards JC, et al. The effect of local temperature changes on sebum excretion rate and forehead surface lipid composition. Br J Dermatol. (1973) 88:257–62. doi: 10.1111/j.1365-2133.1973.tb07544.x
83. Kim S, Park JW, Yeon Y, Han JY, Kim E. Influence of exposure to summer environments on skin properties. J Eur Acad Dermatol Venereol. (2019) 33:2192–6. doi: 10.1111/jdv.15745
84. Li D, Chen Q, Liu Y; Liu T, Tang W; Li S. The prevalence of acne in Mainland China: a systematic review and meta-analysis. BMJ Open. (2017) 7:e015354. doi: 10.1136/bmjopen-2016-015354
85. Robeva R, Assyov Y, Tomova A, Kumanov P. Acne vulgaris is associated with intensive pubertal development and altitude of residence–a cross-sectional population-based study on 6,200 boys. Eur J Pediatr. (2013) 172:465–71. doi: 10.1007/s00431-012-1907-1
86. Lee WJ, Park KH, Sohn MY, Lee WC, Lee SJ, Kim DW. Ultraviolet B irradiation increases the expression of inflammatory cytokines in cultured sebocytes. J Dermatol. (2013) 40:993–7. doi: 10.1111/1346-8138.12330
87. Lefebvre MA, Pham DM, Boussouira B, Bernard D, Camus C; Nguyen QL. Evaluation of the impact of urban pollution on the quality of skin: a multicentre study in Mexico. Int J Cosmet Sci. (2015) 37:329–38. doi: 10.1111/ics.12203
88. Krutmann J, Moyal D, Liu W, Kandahari S, Lee GS, Nopadon N, et al. Pollution and acne: is there a link? Clin Cosmet Investig Dermatol. (2017) 10:199–204. doi: 10.2147/CCID.S131323
89. Lefebvre MA, Pham DM, Boussouira B, Qiu H, Ye C, Long, et al. Consequences of urban pollution upon skin status. A controlled study in Shanghai area. Int J Cosmet Sci. (2016) 38:217–23. doi: 10.1111/ics.12270
90. Liu W, Pan X, Vierkotter A, Guo Q, Wang X, Wang Q, et al. A time-series study of the effect of air pollution on outpatient visits for acne vulgaris in Beijing. Skin Pharmacol Physiol. (2018) 31:107–13. doi: 10.1159/000484482
91. Kokelj F. Occupational acne. Clin Dermatol. (1992) 10:213–7. doi: 10.1016/0738-081X(92)90108-B
92. Ju Q, Zouboulis CC, Xia L. Environmental pollution and acne: chloracne. Dermatoendocrinol. (2009) 1:125–8. doi: 10.4161/derm.1.3.7862
93. Borgatti SP, Mehra A, Brass DJ, Labianca G. Network analysis in the social sciences. Science. (2009) 323:892–5. doi: 10.1126/science.1165821
94. Cohen S. Social relationships and health. Am Psychol. (2004) 59:676–84. doi: 10.1037/0003-066X.59.8.676
95. Cohen-Cole E, Fletcher JM. Detecting implausible social network effects in acne, height, and headaches: longitudinal analysis. BMJ. (2008) 337:a2533. doi: 10.1136/bmj.a2533
96. Christakis NA, Fowler JH. The spread of obesity in a large social network over 32 years. N Engl J Med. (2007) 357:370–9. doi: 10.1056/NEJMsa066082
97. Valente TW, Fujimoto K, Chou CP, Spruijt-Metz D. Adolescent affiliations and adiposity: a social network analysis of friendships and obesity. J Adolesc Health. (2009) 45:202–4. doi: 10.1016/j.jadohealth.2009.01.007
98. Mercken L, Snijders TA, Steglich C, de Vries H. Dynamics of adolescent friendship networks and smoking behavior: social network analyses in six European countries. Soc Sci Med. (2009) 69:1506–14. doi: 10.1016/j.socscimed.2009.08.003
99. Van Zalk MH, Kerr M, Branje SJ, Stattin H, Meeus WH. It takes three: selection, influence, and de-selection processes of depression in adolescent friendship networks. Dev Psychol. (2010) 46:927–38. doi: 10.1037/a0019661
100. Van Zalk N, Van Zalk M, Kerr M, Stattin H. Social anxiety as a basis for friendship selection and socialization in adolescents' social networks. J Pers. (2011) 79:499–525. doi: 10.1111/j.1467-6494.2011.00682.x
101. Yousaf A, Hagen R, Delaney E, Davis S, Zinn Z. The influence of social media on acne treatment: a cross-sectional survey. Pediatr Dermatol. (2020) 37:301–4. doi: 10.1111/pde.14091
102. Borba AJ, Young PM, Read C, Armstrong AW. Engaging but inaccurate: a cross-sectional analysis of acne videos on social media from non-health care sources. J Am Acad Dermatol. (2019) 83:610–2. doi: 10.1016/j.jaad.2019.08.035
103. Bird EL, Ige JO, Pilkington P, Pinto A, Petrokofsky C, Burgess-Allen J. Built and natural environment planning principles for promoting health: an umbrella review. BMC Public Health . (2018) 18:930. doi: 10.1186/s12889-018-5870-2
104. Ribeiro AI, Santos AC, Vieira VM, Barros H. Hotspots of childhood obesity in a large metropolitan area: does neighbourhood social and built environment play a part? Int J Epidemiol. (2019) 49:934–43. doi: 10.1093/ije/dyz205
105. Dwicaksono A, Brissette I, Birkhead GS, Bozlak CT, Martin EG. Evaluating the contribution of the built environment on obesity among New York State Students. Health Educ Behav. (2018) 45:480–91. doi: 10.1177/1090198117742440
106. Xu Y, Wen M, Wang F. Multilevel built environment features and individual odds of overweight and obesity in Utah. Appl Geogr. (2015) 60:197–203. doi: 10.1016/j.apgeog.2014.10.006
107. Yang Y, Jiang Y, Xu Y, Mzayek F, Levy M. A cross-sectional study of the influence of neighborhood environment on childhood overweight and obesity: variation by age, gender, and environment characteristics. Prev Med. (2018) 108:23–28. doi: 10.1016/j.ypmed.2017.12.021
108. Wasserman JA, Suminski R, Xi J, Mayfield C, Glaros A, Magie R. A multi-level analysis showing associations between school neighborhood and child body mass index. Int J Obes. (2014) 38:912–8. doi: 10.1038/ijo.2014.64
109. Malambo P, Kengne AP, De Villiers A, Lambert EV, Puoane T. Built environment, selected risk factors and major cardiovascular disease outcomes: a systematic review. PLoS ONE. (2016) 11:e0166846. doi: 10.1371/journal.pone.0166846
110. Tomita A, Vandormael AM, Cuadros D, Di Minin E, Heikinheimo V, Tanser F, et al. Green environment and incident depression in South Africa: a geospatial analysis and mental health implications in a resource-limited setting. Lancet Planet Health. (2017) 1:e152–62. doi: 10.1016/S2542-5196(17)30063-3
111. Hartig T, Mitchell R, de Vries S, Frumkin H. Nature and health. Annu Rev Public Health. (2014) 35:207–28. doi: 10.1146/annurev-publhealth-032013-182443
112. Gao M, Ahern J, Koshland CP. Perceived built environment and health-related quality of life in four types of neighborhoods in Xi'an, China. Health Place. (2016) 39:110–5. doi: 10.1016/j.healthplace.2016.03.008
113. Saunders LE, Green JM, Petticrew MP, Steinbach R, Roberts H. What are the health benefits of active travel? A systematic review of trials and cohort studies. PLoS ONE. (2013) 8:e69912. doi: 10.1371/journal.pone.0069912
114. Xu F, Li J, Liang Y, Wang Z, Hong X, Ware RS, et al. Residential density and adolescent overweight in a rapidly urbanising region of Mainland China. J Epidemiol Community Health. (2010) 64:1017–21. doi: 10.1136/jech.2009.094169
115. Saluja T, Davies A, Oldmeadow C, Boyle A. Impact of fast food outlet density on incidence of myocardial infarction in the hunter region. Intern Med J. (2020) 28. doi: 10.1111/imj.14745
116. Burgoine T, Forouhi NG, Griffin SJ, Brage S, Wareham NJ, Monsivais P. Does neighborhood fast-food outlet exposure amplify inequalities in diet and obesity? A cross-sectional study. Am J Clin Nutr. (2016) 103:1540–7. doi: 10.3945/ajcn.115.128132
117. Burgoine T, Sarkar C, Webster CJ, Monsivais P. Examining the interaction of fast-food outlet exposure and income on diet and obesity: evidence from 51,361 UK Biobank participants. Int J Behav Nutr Phys Act. (2018) 15:71. doi: 10.1186/s12966-018-0713-1
118. Cutumisu N, Traoré I, Paquette MC, Cazale L, Camirand H, Lalonde B, et al. Association between junk food consumption and fast-food outlet access near school among Quebec secondary-school children: findings from the Quebec Health Survey of High School Students (QHSHSS) 2010-11. Public Health Nutr . (2017) 20:927–37. doi: 10.1017/S136898001600286X
119. Markevych I, Schoierer J, Hartig T, Chudnovsky A, Hystad P, Dzhambov AM, et al. Exploring pathways linking greenspace to health: theoretical and methodological guidance. Environ Res. (2017) 158:301–17. doi: 10.1016/j.envres.2017.06.028
120. Dzhambov A, Hartig T, Markevych I, Tilov B, Dimitrova D. Urban residential greenspace and mental health in youth: different approaches to testing multiple pathways yield different conclusions. Environ Res. (2018) 160:47–59. doi: 10.1016/j.envres.2017.09.015
121. Mueller W, Steinle S, Pärkkä J, Parmes E, Liedes H, et al. Urban greenspace and the indoor environment: pathways to health via indoor particulate matter, noise, and road noise annoyance. Environ Res . (2020) 180:108850. doi: 10.1016/j.envres.2019.108850
122. Klompmaker JO, Janssen NAH, Bloemsma LD, Gehring U, Wijga AH, van den Brink C. Residential surrounding green, air pollution, traffic noise and self-perceived general health. Environ Res. (2019) 179(Pt A):108751. doi: 10.1016/j.envres.2019.108751
123. Almanza E, Jerrett M, Dunton G, Seto E, Pentz MA. A study of community design, greenness, and physical activity in children using satellite, GPS and accelerometer data. Health Place. (2012) 18:46–54. doi: 10.1016/j.healthplace.2011.09.003
124. Jennings V, Bamkole O. The relationship between social cohesion and urban green space: an avenue for health promotion. Int J Environ Res Public Health. (2019) 16:452. doi: 10.3390/ijerph16030452
125. Rissel C, Curac N, Greenaway M, Bauman A. Physical activity associated with public transport use–a review and modelling of potential benefits. Int J Environ Res Public Health. (2012) 9:2454–78. doi: 10.3390/ijerph9072454
126. Sener IN, Lee RJ, Elgart Z. Potential health implications and health cost reductions of transit-induced physical activity. J Transp Health. (2016) 3:133–40. doi: 10.1016/j.jth.2016.02.002
127. Faulkner GE, Buliung RN, Flora PK, Fusco C. Active school transport, physical activity levels and body weight of children and youth: a systematic review. Prev Med. (2009) 48:3–8. doi: 10.1016/j.ypmed.2008.10.017
128. Madison KC. Barrier function of the skin: “la raison d'être” of the epidermis. J Invest Dermatol. (2003) 121:231–41. doi: 10.1046/j.1523-1747.2003.12359.x
129. Aioi A, Okuda M, Matsui M, Tonogaito H, Hamada K. Effect of high population density environment on skin barrier function in mice. J Dermatol Sci . (2001) 25:189–97. doi: 10.1016/S0923-1811(00)00133-X
130. Yamane T, Kobayashi-Hattori K, Oishi Y, Takita T. High-fat diet reduces levels of type I tropocollagen and hyaluronan in rat skin. Mol Nutr Food Res. (2010) 54 (Suppl. 1):S53–61. doi: 10.1002/mnfr.201000022
131. Meeran SM, Singh T, Nagy TR, Katiyar SK. High-fat diet exacerbates inflammation and cell survival signals in the skin of ultraviolet B-irradiated C57BL/6 mice. Toxicol Appl Pharmacol. (2009) 241:303–10. doi: 10.1016/j.taap.2009.09.003
132. Araviiskaia E, Berardesca E, Bieber T, Gontijo G, Sanchez Viera M, Marrot L, et al. The impact of airborne pollution on skin. J Eur Acad Dermatol Venereol. (2019) 33:1496–505. doi: 10.1111/jdv.15583
133. Schikowski T, Hüls A. Air pollution and skin aging. Curr Environ Health Rep. (2020) 7:58–64. doi: 10.1007/s40572-020-00262-9
134. Nakhjirgan P, Mahmoodi M, Kashani H, Firooz A, Nabizadeh R, Kermani M, et al. Air pollution and exacerbation of skin itching and sleep disturbance in Iranian atopic dermatitis patients. J Environ Health Sci Eng. (2019) 17:811–6. doi: 10.1007/s40201-019-00397-4
135. Hunter HJA, Momen SE, Kleyn CE. The impact of psychosocial stress on healthy skin. Clin Exp Dermatol. (2015) 40:540–6. doi: 10.1111/ced.12582
136. Choi EH, Brown BE, Crumrine D, Chang S, Man MQ, Elias PM, et al. Mechanisms by which psychologic stress alters cutaneous permeability barrier homeostasis and stratum corneum integrity. J Invest Dermatol. (2005) 124:587–95. doi: 10.1111/j.0022-202X.2005.23589.x
137. Lee JK, Y; So WY. Differences in skin health outcomes according to physical activity level among Korean Female College Students. Iran J Public Health. (2019) 48:1924–6 doi: 10.18502/ijph.v48i10.3504
138. Ng SC, Kaplan GG, Tang W, Banerjee R, Adigopula B, Underwood FE, et al. Population density and risk of inflammatory bowel disease: a prospective population-based study in 13 countries or regions in Asia-Pacific. Am J Gastroenterol. (2019) 114:107–15. doi: 10.1038/s41395-018-0233-2
139. Xu Y, Wang F. Built environment and obesity by urbanicity in the U.S. Health Place. (2015) 34:19–29. doi: 10.1016/j.healthplace.2015.03.010
140. Howard Wilsher S, Harrison F, Yamoah F, Fearne A, Jones A. The relationship between unhealthy food sales, socio-economic deprivation and childhood weight status: results of a cross-sectional study in England. Int J Behav Nutr Phys Act. (2016) 13:21. doi: 10.1186/s12966-016-0345-2
141. Bodor JN, Rice JC, Farley TA, Swalm CM, Rose D. The association between obesity and Urban food environments. J Urban Health. (2010) 87:771–81. doi: 10.1007/s11524-010-9460-6
142. Lin L. Leisure-time physical activity, objective urban neighborhood built environment, and overweight and obesity of Chinese school-age children. J Transport Health. (2018) 10:322–33. doi: 10.1016/j.jth.2018.05.001
143. Astell-Burt T, Feng X, Kolt GS. Neighbourhood green space and the odds of having skin cancer: multilevel evidence of survey data from 267072 Australians. J Epidemiol Community Health. (2014) 68:370–4. doi: 10.1136/jech-2013-203043
144. Stewart I D, Oke T R. Local climate zones for urban temperature studies. Bull Am Meteorol Soc. (2012) 93:1879–900. doi: 10.1175/BAMS-D-11-00019.1
145. Liu Y, Wang R, Grekousis G, Liu Y, Yuan Y, Li Z. Neighbourhood greenness and mental wellbeing in Guangzhou, China: what are the pathways? Landsc Urban Plann. (2019) 190:103602. doi: 10.1016/j.landurbplan.2019.103602
146. Liu Y, Wang R, Xiao Y, Huang B, Chen H, Li Z. Exploring the linkage between greenness exposure and depression among Chinese people: mediating roles of physical activity, stress and social cohesion and moderating role of urbanicity. Health Place. (2019) 58:102168. doi: 10.1016/j.healthplace.2019.102168
147. Wang R, Helbich M, Yao Y, Zhang J, Liu P, Yuan Y, et al. Urban greenery and mental wellbeing in adults: cross-sectional mediation analyses on multiple pathways across different greenery measures. Environ Res. (2019) 176:108535. doi: 10.1016/j.envres.2019.108535
148. Helbich M, Yao Y, Liu Y, Zhang J, Liu P, Wang R. Using deep learning to examine street view green and blue spaces and their associations with geriatric depression in Beijing, China. Environ Int. (2019) 126:107–17. doi: 10.1016/j.envint.2019.02.013
149. Huang B, Liu Y, Feng Z, Pearce JR, Wang R, Zhang Y, et al. Residential exposure to natural outdoor environments and general health among older adults in Shanghai, China. Int J Equity Health. (2019) 18:178. doi: 10.1186/s12939-019-1081-4
150. Wang R, Yang B, Yao Y, Bloom MS, Feng Z, Yuan Y, et al. Residential greenness, air pollution and psychological well-being among urban residents in Guangzhou, China. Sci Total Environ. (2020) 711:134843. doi: 10.1016/j.scitotenv.2019.134843
151. O'Callaghan-Gordo C, Espinosa A, Valentin A, Tonne C, Perez-Gomez B, Castano-Vinyals G, et al. Green spaces, excess weight and obesity in Spain. Int J Hyg Environ Health. (2020) 223:45–55. doi: 10.1016/j.ijheh.2019.10.007
152. Lovasi GS, Schwartz-Soicher O, Quinn JW, Berger DK, Neckerman KM, Jaslow R, et al. Neighborhood safety and green space as predictors of obesity among preschool children from low-income families in New York City. Prev Med. (2013) 57:189–93. doi: 10.1016/j.ypmed.2013.05.012
153. Huang WZ, Yang BY, Yu HY, Bloom MS, Markevych I, Heinrich J, et al. Association between community greenness and obesity in urban-dwelling Chinese adults. Sci Total Environ. (2020) 702:135040. doi: 10.1016/j.scitotenv.2019.135040
154. McClure RJ, Adriazola-Steil C, Mulvihill C, Salmon P, Bonnington CP, Stevenson M. Simulating the dynamic effect of land use and transport policies on the health of populations. Am J Public Health. (2015) 105 (Suppl. 2):S223–9. doi: 10.2105/AJPH.2014.302303
155. Stevenson M, Thompson J, de Sá TH, Ewing R, Mohan D, McClure R, et al. Land use, transport, and population health: estimating the health benefits of compact cities. Lancet. (2016) 388:2925–35. doi: 10.1016/S0140-6736(16)30067-8
156. Frank LD, Andresen MA, Schmid TL. Obesity relationships with community design, physical activity, and time spent in cars. Am J Prev Med. (2004) 27:87–96. doi: 10.1016/j.amepre.2004.04.011
157. Mobley LR, Root ED, Finkelstein EA, Khavjou O, Farris RP, Will JC. Environment, obesity, and cardiovascular disease risk in low-income women. Am J Prev Med. (2006) 30:327–32. doi: 10.1016/j.amepre.2005.12.001
158. Brown V, Barr A, Scheurer J, Magnus A, Zapata-Diomedi B, Bentley R. Better transport accessibility, better health: a health economic impact assessment study for Melbourne, Australia. Int J Behav Nutr Phys Act. (2019) 16:89. doi: 10.1186/s12966-019-0853-y
159. Dons E, Rojas-Rueda D, Anaya-Boig E, Avila-Palencia I, Brand C, Cole-Hunter T. Transport mode choice and body mass index: cross-sectional and longitudinal evidence from a European-wide study. Environ Int. (2018) 119:109–16. doi: 10.1016/j.envint.2018.06.023
160. Turrell G, Hewitt BA, Rachele JN, Giles-Corti B, Brown WJ. Prospective trends in body mass index by main transport mode, 2007–2013. J Transp Health. (2018) 8:183–92. doi: 10.1016/j.jth.2017.12.004
161. Liao Y, Tsai H-H, Wang H-S, Lin C-P, Wu M-C, Chen J-F. Travel mode, transportation-related physical activity, and risk of overweight in Taiwanese adults. J Transp Healt. (2016) 3:220–5. doi: 10.1016/j.jth.2016.02.012
162. Avila-Palencia I, Int Panis L, Dons E, Gaupp-Berghausen M, Raser E, Götschi T, et al. The effects of transport mode use on self-perceived health, mental health, and social contact measures: a cross-sectional and longitudinal study. Environ Int. (2018) 120:199–206. doi: 10.1016/j.envint.2018.08.002
163. Mattisson K, Håkansson C, Jakobsson K. Relationships between commuting and social capital among men and women in Southern Sweden. Environ Behav. (2015) 47:734–53. doi: 10.1177/0013916514529969
164. Martin A, Goryakin Y, Suhrcke M. Does active commuting improve psychological wellbeing? Longitudinal evidence from eighteen waves of the British Household Panel Survey. Prev Med. (2014) 69:296–303. doi: 10.1016/j.ypmed.2014.08.023
Keywords: acne, sociology of population, natural environment, social environment, built environment, green spaces
Citation: Yang J, Yang H, Xu A and He L (2020) A Review of Advancement on Influencing Factors of Acne: An Emphasis on Environment Characteristics. Front. Public Health 8:450. doi: 10.3389/fpubh.2020.00450
Received: 11 April 2020; Accepted: 21 July 2020; Published: 17 September 2020.
Reviewed by:
Copyright © 2020 Yang, Yang, Xu and He. This is an open-access article distributed under the terms of the Creative Commons Attribution License (CC BY) . The use, distribution or reproduction in other forums is permitted, provided the original author(s) and the copyright owner(s) are credited and that the original publication in this journal is cited, in accordance with accepted academic practice. No use, distribution or reproduction is permitted which does not comply with these terms.
*Correspondence: Haoran Yang, haoranyang0119@126.com
Disclaimer: All claims expressed in this article are solely those of the authors and do not necessarily represent those of their affiliated organizations, or those of the publisher, the editors and the reviewers. Any product that may be evaluated in this article or claim that may be made by its manufacturer is not guaranteed or endorsed by the publisher.
Information
- Author Services
Initiatives
You are accessing a machine-readable page. In order to be human-readable, please install an RSS reader.
All articles published by MDPI are made immediately available worldwide under an open access license. No special permission is required to reuse all or part of the article published by MDPI, including figures and tables. For articles published under an open access Creative Common CC BY license, any part of the article may be reused without permission provided that the original article is clearly cited. For more information, please refer to https://www.mdpi.com/openaccess .
Feature papers represent the most advanced research with significant potential for high impact in the field. A Feature Paper should be a substantial original Article that involves several techniques or approaches, provides an outlook for future research directions and describes possible research applications.
Feature papers are submitted upon individual invitation or recommendation by the scientific editors and must receive positive feedback from the reviewers.
Editor’s Choice articles are based on recommendations by the scientific editors of MDPI journals from around the world. Editors select a small number of articles recently published in the journal that they believe will be particularly interesting to readers, or important in the respective research area. The aim is to provide a snapshot of some of the most exciting work published in the various research areas of the journal.
Original Submission Date Received: .
- Active Journals
- Find a Journal
- Proceedings Series
- For Authors
- For Reviewers
- For Editors
- For Librarians
- For Publishers
- For Societies
- For Conference Organizers
- Open Access Policy
- Institutional Open Access Program
- Special Issues Guidelines
- Editorial Process
- Research and Publication Ethics
- Article Processing Charges
- Testimonials
- Preprints.org
- SciProfiles
- Encyclopedia
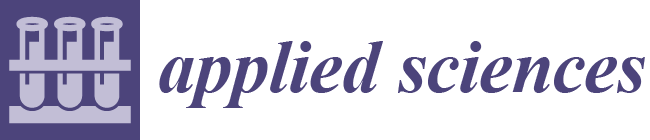
Article Menu

- Subscribe SciFeed
- Recommended Articles
- Google Scholar
- on Google Scholar
- Table of Contents
Find support for a specific problem in the support section of our website.
Please let us know what you think of our products and services.
Visit our dedicated information section to learn more about MDPI.
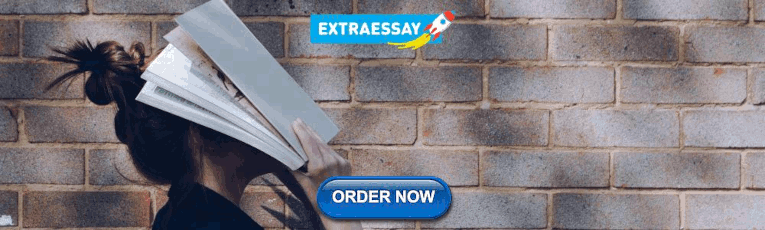
JSmol Viewer
Efficacy, tolerability, and face lipidomic modification of new regimen with cleanser and corrective serum in women with acne-prone skin.
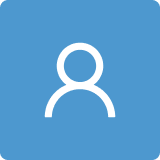
1. Introduction
1.1. adult female acne, 1.2. etiopathogenesis of afa, 1.3. skin barrier alteration in a. vulgaris, 1.4. quantitative and qualitative change in sebum, 1.5. study aim, 2. materials and methods, 2.1. study design, 2.2. statistical analysis, 4. discussion, 5. conclusions, author contributions, institutional review board statement, informed consent statement, data availability statement, conflicts of interest.
- Tanghetti, E.A.; Kawata, A.K.; Daniels, S.R.; Yeomans, K.; Burk, C.T.; Callender, V.D. Understanding the Burden of Adult Female Acne. J. Clin. Aesthetic Dermatol. 2014 , 7 , 22–30. [ Google Scholar ]
- Rocha, M.A.; Bagatin, E. Adult-Onset Acne: Prevalence, Impact, and Management Challenges. Clin. Cosmet. Investig. Dermatol. 2018 , 11 , 59–69. [ Google Scholar ] [ CrossRef ] [ PubMed ]
- Kutlu, Ö.; Karadağ, A.S.; Wollina, U. Adult Acne versus Adolescent Acne: A Narrative Review with a Focus on Epidemiology to Treatment. Bras. Dermatol. 2023 , 98 , 75–83. [ Google Scholar ] [ CrossRef ] [ PubMed ]
- Bhate, K.; Williams, H.C. Epidemiology of Acne Vulgaris. Br. J. Dermatol. 2013 , 168 , 474–485. [ Google Scholar ] [ CrossRef ]
- Collier, C.N.; Harper, J.C.; Cantrell, W.C.; Wang, W.; Foster, K.W.; Elewski, B.E. The Prevalence of Acne in Adults 20 Years and Older. J. Am. Acad. Dermatol. 2008 , 58 , 56–59. [ Google Scholar ] [ CrossRef ] [ PubMed ]
- Tan, A.U.; Schlosser, B.J.; Paller, A.S. A Review of Diagnosis and Treatment of Acne in Adult Female Patients. Int. J. Womens Dermatol. 2018 , 4 , 56–71. [ Google Scholar ] [ CrossRef ]
- Bagatin, E.; de Freitas, T.H.P.; Rivitti-Machado, M.C.; Ribeiro, B.M.; Nunes, S.; da Rocha, M.A.D. Adult Female Acne: A Guide to Clinical Practice. Bras. Dermatol. 2019 , 94 , 62–75. [ Google Scholar ] [ CrossRef ]
- Kamangar, F.; Shinkai, K. Acne in the Adult Female Patient: A Practical Approach. Int. J. Dermatol. 2012 , 51 , 1162–1174. [ Google Scholar ] [ CrossRef ]
- Dréno, B.; Thiboutot, D.; Layton, A.M.; Berson, D.; Perez, M.; Kang, S. Large-scale International Study Enhances Understanding of an Emerging Acne Population: Adult Females. J. Eur. Acad. Dermatol. Venereol. 2015 , 29 , 1096–1106. [ Google Scholar ] [ CrossRef ]
- Kim, H.J.; Kim, Y.H. Exploring Acne Treatments: From Pathophysiological Mechanisms to Emerging Therapies. Int. J. Mol. Sci. 2024 , 25 , 5302. [ Google Scholar ] [ CrossRef ]
- Bhat, Y.; Latief, I.; Hassan, I. Update on Etiopathogenesis and Treatment of Acne. Indian. J. Dermatol. Venereol. Leprol. 2017 , 83 , 298. [ Google Scholar ] [ CrossRef ]
- Zouboulis, C.C.; Picardo, M.; Ju, Q.; Kurokawa, I.; Törőcsik, D.; Bíró, T.; Schneider, M.R. Beyond Acne: Current Aspects of Sebaceous Gland Biology and Function. Rev. Endocr. Metab. Disord. 2016 , 17 , 319–334. [ Google Scholar ] [ CrossRef ] [ PubMed ]
- Ramasamy, S.; Barnard, E.; Dawson, T.L.; Li, H. The Role of the Skin Microbiota in Acne Pathophysiology. Br. J. Dermatol. 2019 , 181 , 691–699. [ Google Scholar ] [ CrossRef ]
- Rocha, M.A.; Bagatin, E. Skin Barrier and Microbiome in Acne. Arch. Dermatol. Res. 2018 , 310 , 181–185. [ Google Scholar ] [ CrossRef ]
- Schachner, L.A.; Alexis, A.F.; Andriessen, A.; Berson, D.; Gold, M.; Goldberg, D.J.; Hu, S.; Keri, J.; Kircik, L.; Woolery-Lloyd, H. Insights into Acne and the Skin Barrier: Optimizing Treatment Regimens with Ceramide-containing Skincare. J. Cosmet. Dermatol. 2023 , 22 , 2902–2909. [ Google Scholar ] [ CrossRef ] [ PubMed ]
- Kurokawa, I.; Layton, A.M.; Ogawa, R. Updated Treatment for Acne: Targeted Therapy Based on Pathogenesis. Dermatol. Ther. 2021 , 11 , 1129–1139. [ Google Scholar ] [ CrossRef ]
- Del Rosso JQ, L.J. The Clinical Relevance of Maintaining the Functional Integrity of the Stratum Corneum in Both Healthy and Disease-Affected Skin. J. Clin. Aesthet. Dermatol. 2011 , 4 , 22–42. [ Google Scholar ]
- Yamamoto, A.; Takenouchi, K.; Ito, M. Impaired Water Barrier Function in Acne Vulgaris. Arch. Dermatol. Res. 1995 , 287 , 214–218. [ Google Scholar ] [ CrossRef ]
- Pappas, A.; Kendall, A.C.; Brownbridge, L.C.; Batchvarova, N.; Nicolaou, A. Seasonal Changes in Epidermal Ceramides Are Linked to Impaired Barrier Function in Acne Patients. Exp. Dermatol. 2018 , 27 , 833–836. [ Google Scholar ] [ CrossRef ] [ PubMed ]
- Ottaviani, M.; Camera, E.; Picardo, M. Lipid Mediators in Acne. Mediat. Inflamm. 2010 , 2010 , 858176. [ Google Scholar ] [ CrossRef ]
- Carmina, E.; Dreno, B.; Lucky, W.A.; Agak, W.G.; Dokras, A.; Kim, J.J.; Lobo, R.A.; Ramezani Tehrani, F.; Dumesic, D. Female Adult Acne and Androgen Excess: A Report from the Multidisciplinary Androgen Excess and PCOS Committee. J. Endocr. Soc. 2022 , 6 , bvac003. [ Google Scholar ] [ CrossRef ]
- McGinley, K.J.; Webster, G.F.; Ruggieri, M.R.; Leyden, J.J. Regional Variations in Density of Cutaneous Propionibacteria: Correlation of Propionibacterium Acnes Populations with Sebaceous Secretion. J. Clin. Microbiol. 1980 , 12 , 672–675. [ Google Scholar ] [ CrossRef ]
- Csak, T.; Ganz, M.; Pespisa, J.; Kodys, K.; Dolganiuc, A.; Szabo, G. Fatty Acid and Endotoxin Activate Inflammasomes in Mouse Hepatocytes That Release Danger Signals to Stimulate Immune Cells. Hepatology 2011 , 54 , 133–144. [ Google Scholar ] [ CrossRef ] [ PubMed ]
- Li, Q.; Fang, H.; Dang, E.; Wang, G. The Role of Ceramides in Skin Homeostasis and Inflammatory Skin Diseases. J. Dermatol. Sci. 2020 , 97 , 2–8. [ Google Scholar ] [ CrossRef ] [ PubMed ]
- Ding, W.; Hu, Y.; Yu, X.; He, C.; Tian, Y. Analysis on the Difference of Skin Surface Lipids during Blue Light Therapy for Acne by Lipidomics. Biomed. Opt. Express 2022 , 13 , 3434. [ Google Scholar ] [ CrossRef ] [ PubMed ]
- Dias da Rocha, M.A.; Saint Aroman, M.; Mengeaud, V.; Carballido, F.; Doat, G.; Coutinho, A.; Bagatin, E. Unveiling the Nuances of Adult Female Acne: A Comprehensive Exploration of Epidemiology, Treatment Modalities, Dermocosmetics, and the Menopausal Influence. Int. J. Womens Health 2024 , 16 , 663–678. [ Google Scholar ] [ CrossRef ]
- Del Rosso, J.Q.; Harper, J.C.; Graber, E.M.; Thiboutot, D.; Silverberg, N.B.; Eichenfield, L.F. Status Report from the American Acne & Rosacea Society on Medical Management of Acne in Adult Women, Part 2: Topical Therapies. Cutis 2015 , 96 , 321–325. [ Google Scholar ]
- Hammill, C.; Vaillancourt, T. Acne and Its Association with Internalizing Problems. Dermatol. Rev. 2023 , 4 , 228–238. [ Google Scholar ] [ CrossRef ]
- Altunay, I.; Özkur, E.; Dalgard, F.; Gieler, U.; Aragones, L.; Lien, L.; Poot, F.; Jemec, G.; Misery, L.; Szabó, C.; et al. Psychosocial Aspects of Adult Acne: Data from 13 European Countries. Acta Derm. Venereol. 2020 , 100 , 5671. [ Google Scholar ] [ CrossRef ]
- Branisteanu, D.; Toader, M.; Porumb, E.; Serban, I.; Pinzariu, A.; Branisteanu, C.; Vicovan, A.; Dimitriu, A.; Fartusnic, I.-A.; Boda, D.; et al. Adult Female Acne: Clinical and Therapeutic Particularities (Review). Exp. Ther. Med. 2021 , 23 , 151. [ Google Scholar ] [ CrossRef ]
Click here to enlarge figure
The statements, opinions and data contained in all publications are solely those of the individual author(s) and contributor(s) and not of MDPI and/or the editor(s). MDPI and/or the editor(s) disclaim responsibility for any injury to people or property resulting from any ideas, methods, instructions or products referred to in the content. |
Share and Cite
Vitale, M.; Gómez-Sánchez, M.J.; Vicente, M.H.; Colombo, F.; Milani, M. Efficacy, Tolerability, and Face Lipidomic Modification of New Regimen with Cleanser and Corrective Serum in Women with Acne-Prone Skin. Appl. Sci. 2024 , 14 , 7799. https://doi.org/10.3390/app14177799
Vitale M, Gómez-Sánchez MJ, Vicente MH, Colombo F, Milani M. Efficacy, Tolerability, and Face Lipidomic Modification of New Regimen with Cleanser and Corrective Serum in Women with Acne-Prone Skin. Applied Sciences . 2024; 14(17):7799. https://doi.org/10.3390/app14177799
Vitale, Maria, María José Gómez-Sánchez, Mencía Hermosa Vicente, Francesca Colombo, and Massimo Milani. 2024. "Efficacy, Tolerability, and Face Lipidomic Modification of New Regimen with Cleanser and Corrective Serum in Women with Acne-Prone Skin" Applied Sciences 14, no. 17: 7799. https://doi.org/10.3390/app14177799
Article Metrics
Article access statistics, further information, mdpi initiatives, follow mdpi.
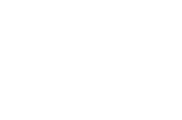
Subscribe to receive issue release notifications and newsletters from MDPI journals
Thank you for visiting nature.com. You are using a browser version with limited support for CSS. To obtain the best experience, we recommend you use a more up to date browser (or turn off compatibility mode in Internet Explorer). In the meantime, to ensure continued support, we are displaying the site without styles and JavaScript.
- View all journals
- Explore content
- About the journal
- Publish with us
- Sign up for alerts
- Open access
- Published: 11 April 2018
An Automatic Diagnosis Method of Facial Acne Vulgaris Based on Convolutional Neural Network
- Xiaolei Shen 1 ,
- Jiachi Zhang 1 ,
- Chenjun Yan 1 &
- Hong Zhou 1
Scientific Reports volume 8 , Article number: 5839 ( 2018 ) Cite this article
18k Accesses
47 Citations
10 Altmetric
Metrics details
- Acne vulgaris
- Imaging techniques
- Machine learning
- Network models
In this paper, we present a new automatic diagnosis method for facial acne vulgaris which is based on convolutional neural networks (CNNs). To overcome the shortcomings of previous methods which were the inability to classify enough types of acne vulgaris. The core of our method is to extract features of images based on CNNs and achieve classification by classifier. A binary-classifier of skin-and-non-skin is used to detect skin area and a seven-classifier is used to achieve the classification task of facial acne vulgaris and healthy skin. In the experiments, we compare the effectiveness of our CNN and the VGG16 neural network which is pre-trained on the ImageNet data set. We use a ROC curve to evaluate the performance of binary-classifier and use a normalized confusion matrix to evaluate the performance of seven-classifier. The results of our experiments show that the pre-trained VGG16 neural network is effective in extracting features from facial acne vulgaris images. And the features are very useful for the follow-up classifiers. Finally, we try applying the classifiers both based on the pre-trained VGG16 neural network to assist doctors in facial acne vulgaris diagnosis.
Similar content being viewed by others
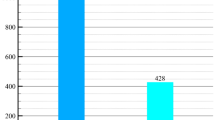
Efficient diagnosis of psoriasis and lichen planus cutaneous diseases using deep learning approach

Prediction of skin disease using a new cytological taxonomy based on cytology and pathology with deep residual learning method
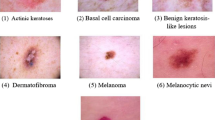
Skin lesion classification of dermoscopic images using machine learning and convolutional neural network
Introduction.
Acne is a chronic skin disease whose characteristics and mechanisms are very complex. Because of its diversity, we cannot find a unified classification method to assist in diagnosis. In this article, we adopt a type of classification consisting of non-inflammatory lesions (open comedones called blackheads and closed comedones called whiteheads), inflammatory lesions (papules and pustules) and relatively more severe types, nodules and cysts 1 . The data sets and the classifier in this article are all designed to detect these six types of acne vulgaris (blackheads, whiteheads, papules, pustules, modules and cysts) mentioned above on human faces. A patient’s face usually suffers from multiple types of acne lesions at the same time. The causes and treatments of different acne lesions are different, so it is necessary to make an accurate and objective diagnosis for the face with acne vulgaris before treatment 2 . The manual observation and counting acne is the traditional diagnosis method. However, the method is not effective enough. It is labor-intensive, time-consuming and subjective, because the diagnosis results depend on expert’s experience and ability. Therefore, a computerized method is very necessary. Many researches have been done on the automatic diagnosis for facial acne with image processing techniques and machine learning theories.
Automatic diagnosis of facial acne needs to detect and classify the region of interest(ROI). The detection of ROI is mainly equal to the detection of facial skin. Previous automatic diagnosis methods also include specific positioning for various acne. Common skin detection models are based on special color spaces to complete, such as RGB, HSV, YCbCr 3 , 4 , 5 , 6 , 7 . Due to mixing of chrominance and luminance data, RGB is not a good choice for skin detection. Although YCbCr avoids this problem, its actual detection effect is still unstable and susceptible to some environmental influences 8 . In past automatic diagnosis methods, specific positioning of the acne area is necessary. Chantharaphaichit et al . proposed a facial acne area detection method based on grayscale and HSV color space 9 . However, with this method, the color, shape and lighting conditions of acne have a great influence on the detection result. Kittigul and Uyyanonvara proposed a different method based on Heat-Mapping and adaptive thresholding 10 . However, the detection results still contain some noise, such as the area of mouth and skin. The above methods are based on special color spaces and thresholds to achieve the detection of acne area. However, the defects of these methods are criticized for their high dependency on threshold values and lack of generalization ability.
Previous automatic diagnosis methods of acne are mostly based on feature extraction to achieve classification of acne. Chang and Liao, and Malik et al . proposed two similar approaches both based on support vector machine and feature extraction to achieve the classification 11 , 12 . Furthermore, Malik et al . classified the severity levels into mild, moderate, severe and very severe. Because of the limitation of classification, these methods can’t achieve the integral analysis with a detailed description.
CNNs have been widely applied to image classification. Sometimes it presents a high recognition ability and gives a better performance than human beings in certain projects, such as the recognition of traffic signs, faces and handwritten digits 13 , 14 , 15 . In recent years, with a lot of research work on the ImageNet data set, the image recognition based on CNN has been continuously improved. In recent studies, CNN with a deeper and wider structure has a large number of parameters. So, the neural network is easy to over fit in the training process. To prevent over fitting, Hinton et al . proposed the “dropout” method and the data augmentation method 16 , 17 , 18 .
In this paper, we propose a novel automatic diagnosis method to overcome the shortcoming of classification categories in the previous methods and achieve a holistic analysis of facial acne vulgaris with a detailed description. Different from the old methods, our method extracts features by CNN instead of manual selection. With the novel method, more and deeper features are extracted to enhance classification accuracy and add more classification types 19 , 20 . The major work in this paper is to achieve the detection of facial skin and the seven-type-classification of facial acne vulgaris by the classifiers with CNN. In the experiment, we compare the validity of extracting features between the CNN model constructed manually by ourselves and the VGG16 model which has been pre-trained on ImageNet. We select the excellent performance model to achieve facial skin area detection and acne seven classification (including 6 types of acne and normal skin) task. On the basis of the classification results, we finally realize the automatic diagnosis and the integral analysis of patients’ faces.
Proposed Methodology
This prospective study was approved by the institution of DOCTOR MIAO NATIONALITY. All study procedures and dataset were performed in accordance with relevant guidelines and regulations. Informed oral consent for the study and publication was obtained from all patients.
The process of the automatic diagnosis method based on CNN is shown in Fig. 1 . There are two main steps: (1) skin detection to locate ROI and (2) seven-type-classification of facial acne vulgaris to achieve the automatic acne diagnosis. The skin detection method is completed by a binary-classifier differentiating skin from non-skin. The binary-classifier with CNN can extract the features from input images and classify them into skin and non-skin to achieve the skin detection. The acne classification method is achieved by a seven-classifier with CNN. The seven-classifier can extract the features from input images by CNN and classify them into one of the seven categories. Then, based on the classification results, integral analysis with a detailed description including acne categories and their respective proportions will be generated.
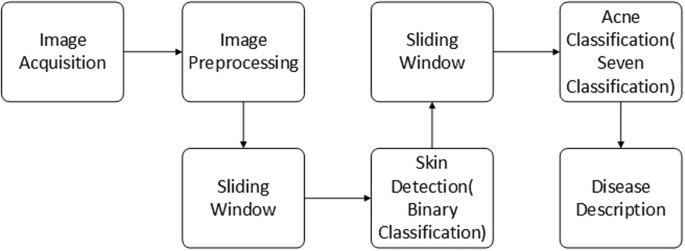
The process of the automatic diagnosis method (sec. 2).
The data sets, data augmentation and data preprocessing
We collect two data sets for construct a binary-classifier and a seven-classifier respectively. 80% of the each data set is used for training, 10% is used for validation and the rest is used for testing. The data set for the binary-classifier consists of 50 × 50 images which are cut from the original 500 × 500 images manually. This data set contains 3000 skin images and 3000 non-skin images. To analyze facial acne vulgaris, skin images include normal skin and diseased skin. A portion of the data set for the binary-classifier models is shown in the Fig. 2 . The data set for the seven-classifier model consists of 50 × 50 images cut from the original 500 × 500 images manually,too. And images augmentation is based on this data set for the seven-classification task. The augmented data set include 6000 blackhead images, 6000 whitehead images, 6000 papule images, 6000 pustule images, 6000 cyst images, 6000 nodule images, 6000 normal skin images. A portion of the data set for the seven-classifier model is shown in the Fig. 3 . Furthermore, we also apply the integrated models on 500 × 500 images to evaluate the practical value. The result is shown in section experiments and results.
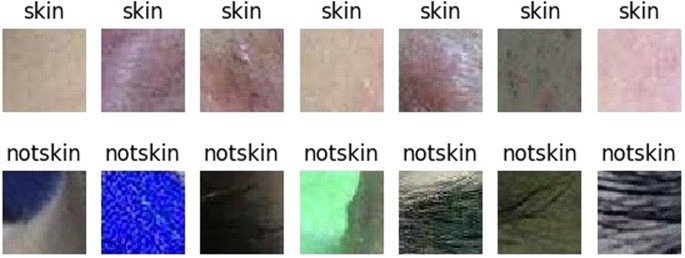
A part of the data set for the training,validation and testing of the binary-classifier (sec. 2.1).

A part of the data set for the training, verification and testing of the seven-classifier (sec. 2.1) Papule (C0), cyst (C1), blackhead (C2), normal skin (C3), pustule (C4), whitehead (C5), nodule (C6).
The original data of seven-classification is so small that it’s very easy to lead the neural network over fitting. We use the simplest and most commonly used method data augmentation to prevent over fitting 17 . In this paper, we used the transformation method of random rotation, shift, shear, scaling and horizontal flip to enlarge our data sets. Transformation can be represented by a transformation matrix. There is a transformation matrix M ,
A and E control the scaling of the image, C and F control the shift of the image, B and D control the shear of the image. Detailedly, the transform matrix of rotation is
In the matrix, h is the height of the picture, w is the width of the picture, θ is the angle of rotation. The transform matrix of shift is
In the matrix, tx is the shift size of height, ty is the shift size of width. The transform matrix of shear is
In the matrix, shear is the transformation intensity of shear, h is the height of the picture, w is the width of the picture. The transform matrix of zoom is
In the matrix, zx and zy control the zoom of the image. Moreover, we set the random horizontal flip image. The examples of transformation are shown in the Fig. 4 .
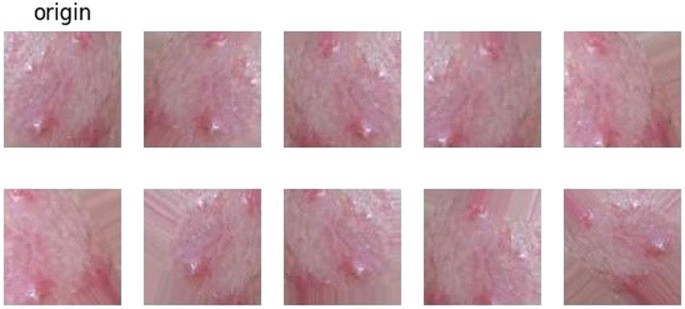
The examples of transformation (sec. 2.1).
Every original image has been transformed several times, but retained its key features. Additionally, the raw pixels of the images are located between 0–255. They already have a certain standard ability. In the experiment, we used a normalization approach to scale the pixel data, which is beneficial for the gradient descent in the training process.
Export the feature vector
CNNs have the ability to extract image features. Normally, a deeper CNN can extract more specific and complex features. However, it cannot ensure better performance of the model if it involves too many convolutional layers. In the transfer learning, VGG16 is a more commonly used CNN model. A VGG16 model without top layers is shown in Table 1 . On the ImageNet and other large data sets VGG16 has an imposing identifying ability, and its effectiveness of the feature extraction has been proved valid on large data sets 21 . In this paper, we use the pre-trained VGG16 network to extract the characteristics from the binary-classification data sets and the seven-classification data sets, and express their features with a 512-dimension feature vector. The advantage of using pre-trained models is obvious. It not only extracts effective features but also reduces the training time. Furthermore, the validity of extraction feature of pre-trained model has been validated. Relatively speaking, to train a capable network for extracting efficient features needs to consume more resources.
In this paper, we also construct a CNN. The network structure is shown in Table 2 . To train a efficient model for extracting features from the seven-classification data set is more difficult than from the binary-classification data set. So, The model constructed by ourselves is trained, validated and tested on the binary-classification data set, which extract the image features alike VGG16. And we use the pre-trained VGG16 model to extract features from seven-classification data set.
Skin detection (binary-classifier) and acne classification (seven-classifier)
The detection of skin area is based on the binary-classifier. Relatively, to build a binary-classifier with high accuracy is not a difficult task, because the random classification has an accuracy of 50%. The feature vector of the image is extracted by a CNN model, and the classifier is used to classify and output the probability of skin or non-skin to achieve the skin detection. Similarly, the classification of acne is based on the feature extraction by the CNN and classification by the seven-classifier, and output the probability of each acne class. The structure of the binary-classifier is shown in Table 3 . Seven-classifier structure is similar to the binary-classifier. The only difference is the 7-dimension output vector at the final dense layer.
After training, the seven-type-classifiers can classify six types of acne and healthy skin. Combined with skin-and-non-skin binary-classifier, this classifier can achieve a facial acne diagnosis. This paper introduces a sliding window method to achieve automatic cropping and traversing of the input facial images then classifier of each small area. Statistics of all classification results complete the overall description of facial acne vulgaris.
In this paper, we adopt some techniques to lower the risk of over fitting. We use the “ReLU” activation function and the “dropout” trick to prevent over fitting 17 . Similarly, the data augmentation and the data normalization have the same effect.
Experiment and Results
Evaluation method.
ROC represents the “Receiver Operating Characteristic” curve, usually used to assess the performance of a binary-classifier. And AUC represents the Area Under ROC. Normally, the classifier’s AUC is larger, the classifier’s performance is better. The straight line connected by point (0,0) and point (1,1) represents a random binary-classifier’s performance 22 , 23 . The Youden’s index is a summation of the ROC, assessing the validity of the diagnostic marker, and selecting the optimal separation threshold 23 , 24 . ROC is very appropriate to evaluate the performance of binary-classifier, but less credible to evaluate multi-classifier. So, in this paper, we do not use the extended form of the ROC to evaluate the performance of seven-classifier, we use the normalized confusion matrix as the method to evaluate the performance of seven-classifier. Normalized confusion matrix is normalized from the confusion matrix. Confusion matrix comprises of the numbers of each categories into which the images of our testing data set are classified, including both correct numbers and incorrect numbers 25 .
Skin detection and the performance of binary-classifier
Skin detection task is completed by a binary-classifier. In the experiments, we compared the performance of the model constructed by ourselves and the model based on pre-trained VGG16. First of all, we scale the data set to range 0–1 through dividing by 255. When training the model constructed by ourselves, we use the binary cross entropy as the loss function. The binary cross entropy loss is
where P ( T = i | X ) is the probability that the model assigns to the label i , and the binary label t ∈ {0,1}. And we use the Adam optimizer whose learning rate is 0.001, beta 1 is 0.9, beta 2 is 0.999 to minimize the loss function. We set the batch size for model’s training is 64, the epochs is 50 and a random seed is 1337 for reproducibility. After 50 epochs, we choose the best model by evaluating the performance of this model on validation data set. When training the model based on pre-trained VGG16, we use the same loss function, optimizer, batch size, epochs and random seed. The difference is that we only need to train the classifier without training the feature extractor. So, its training time is very shorter than the previous model. Similarly, we choose the best model after 50 epochs. What’s more, we tune the feature extractors (the last few convolutional layers) of model based on pre-trained VGG16 with the SGD optimizer whose learning rate is [0.001, 0.01]. However, the performance doesn’t improve obviously. We compare the performance of the two trained models on test data. The test data set hasn’t been seen by the two models. Finally, we will choose the better model to apply into practice. The ROC of the two binary-classifiers are shown in Fig. 5 . The detailed index analysis is shown in Table 4 and the results of skin detection(apply into practice) are shown in Fig. 6 . The AUC of the model based on pre-trained VGG16 is a little bit bigger. But we choose the model based on pre-trained VGG16 by analyzing the data in Table 4 , because it has a good balance between the specificity and the sensitivity. The non-skin areas in the original image including hair, eyes, background, etc. are well detected and covered with black blocks, and the skin areas in the original image are also retained as much as possible. Misjudgments mainly occur when the sliding window contains the junction area of skin area and non-skin. This is the major source of error during detection.
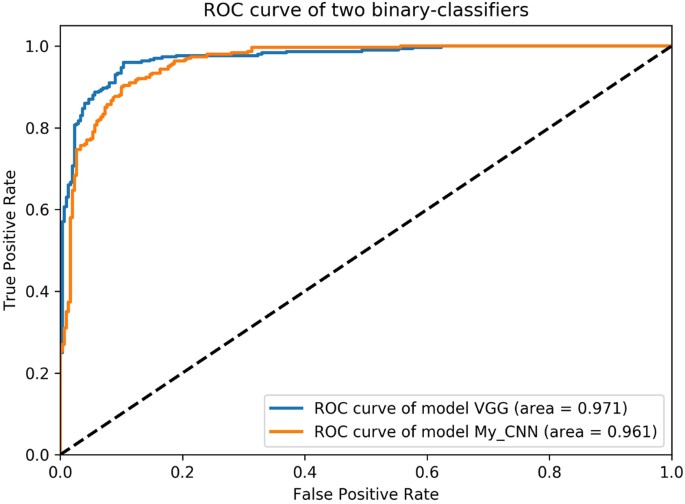
The ROC of two skin-and-non-skin binary-classifier models (sec. 3.2) class 0 represents skin, class 1 represents non-skin. class 0 is regarded as positive example, class 1 is regarded as negative example (epochs = 50). Y-axis is TPR (Sensitivity), X-axis is FPR (1-Specificity).
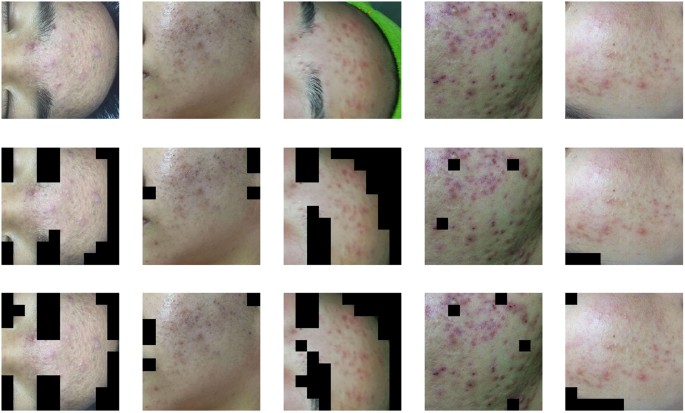
The results of skin detection by the binary-classifier with CNN (sec. 3.2) The first row presents five original images (500 × 500), each of which includes skin areas and non-skin areas. The second row presents five images covered by masks which are built through the binary-classifier based on a manually constructed neural network. The mask is built by 50 × 50 boxes. The third row presents five images covered by masks built through the binary-classifier based on the VGG16 neural network.
Acne classification and the performance of seven-classifier
Because the seven-classification data set is abundant enough after data augmentation, we standardize all the data set with the mean(183.643) and the standard deviation(38.210) of the train data set. When training, we use the categorical cross entropy as the loss function. The categorical cross entropy loss is
where y i is the probability that the model assigns to the label t i , and the seven classification label t ∈ {0, 1, 2, 3, 4, 5, 6}. And we use the same Adam optimizer whose learning rate is 0.001, beta 1 is 0.9, beta 2 is 0.999 to minimize the loss function, the same batch size, epochs and a random seed. After 50 epochs, we choose the best model by evaluating the performance of the model on seven-classification validation data set. What’s more, we also tune the model with SGD whose learning rate is [0.001, 0.01]. But the performance also doesn’t improve obviously,too. In this paper, the normalized confusion matrix is used to evaluate the performance of seven-classifier based on the pre-trained VGG16 model on the testing data set. The normalized confusion matrix is shown in Fig. 7 . The overall description of facial acne symptoms(apply into practice) in this article is shown in Fig. 8 . The overall description of the image predicted by our model and the overall description of the experts’ diagnoses are shown in Table 5 . By analyzing the normalized confusion matrix, although the model has some wrong classification, it can still complete the classification task well. The accuracy of any class is exceed 81%. And by analyzing the Fig. 8 and the Table 5 , it prove the value of the model in practical. In Table 5 , we determine the symptoms based on the predicted probability. In particular, We ignore the probability of the normal skin(c3) class, and choose the large predicted probability to determine the symptoms by model. Compare the result of paper(C) with result of experts, our trained model has the ability to diagnose automatically. What’s more, the model can provide an auxiliary diagnosis function.
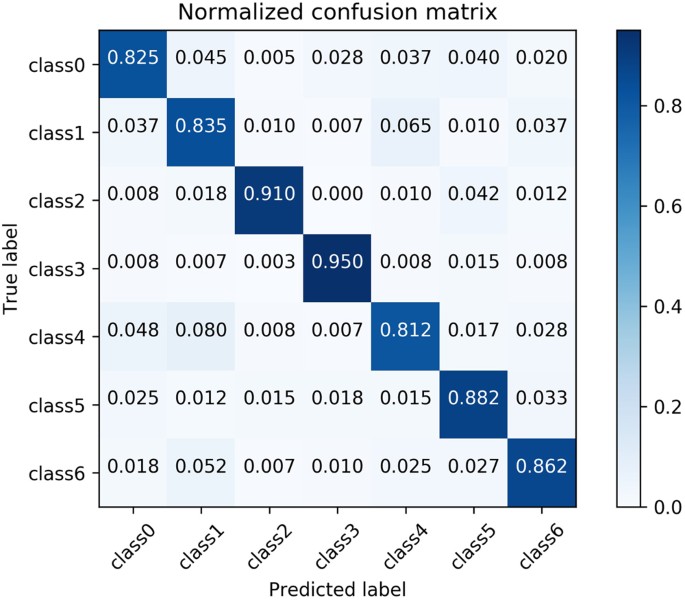
The normalized confusion matrix of the seven-classifier based on VGG16 model (sec. 3.3) Papule (class0), cyst (class1), blackhead (class2), normal skin (class3), pustule (class4), whitehead (class5), nodule (class6). Each column of the matrix represents the instances in a predicted class while each row represents the instances in an actual class.
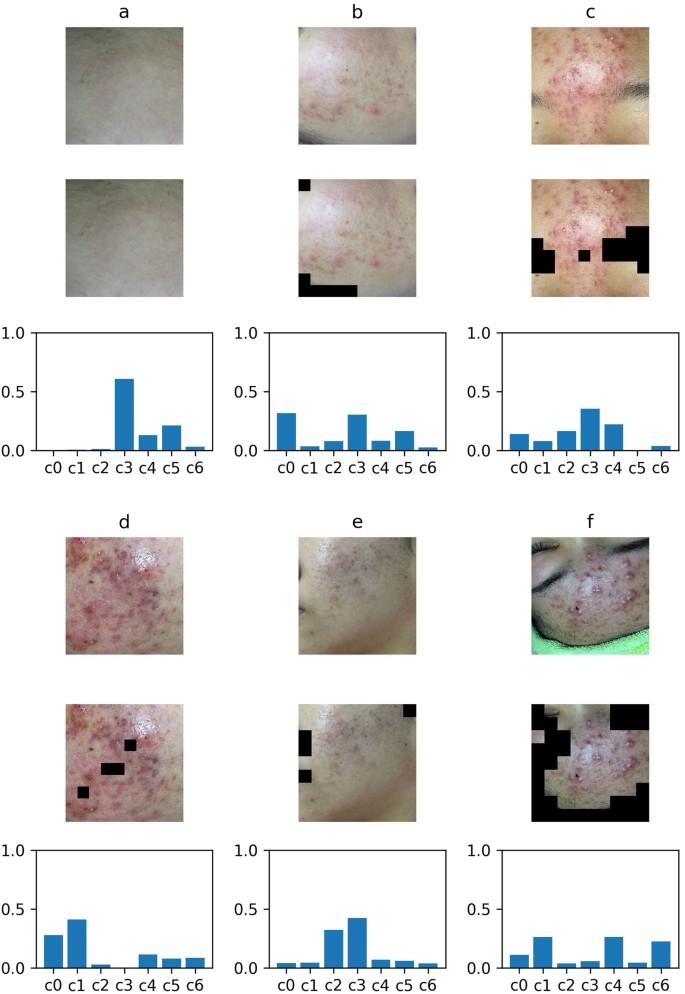
The integral analysis of facial skin area (sec. 3.3) Six images with masks are the input images. The areas with black blocks are non-skin areas, the rest areas are skin areas. The result of integral analysis is shown with the histogram of corresponding proportions. Vertical coordinate of the histogram is the proportion value, abscissa is the type of symptoms, followed by papule (c0), cyst (c1), blackhead (c2), normal skin (c3), pustule (c4), whitehead (c5), nodule (c6).
Compared with traditional skin detection methods based on special color space, the skin detection method in this paper based on skin-and-non-skin binary-classifier is more effective and more robust. Specifically, we find the skin detection method in this paper has a strong anti-interference ability to light conditions and color difference. Therefore, it is more adaptive to many different conditions for the skin detection task. However, it can be seen that there are still some hair areas at junction areas of skin and non-skin that cannot be well detected. The split boundaries in the test images have a clear zigzag shape. It looks bad, but in this experiment, it’s a side effect of an adaptive method, 50 × 50 cutting. And it rarely affects the accuracy of the acne detection in this article. In general, our skin detection method has a better performance in macro aspect, but its micro performance is not satisfying enough.
The seven-type-classifier is the core of the article and is the necessary step to achieve the overall description of facial acne vulgaris. The seven-type-classifier has managed to cut and classify skin areas of the input images. Its practical value is proved through the performance indicators in the Table 5 and Fig. 7 . Compared with past methods, we break the restrictions of classification types. We achieve the seven classification of facial acne vulgaris, realize the automatic diagnosis and give the integral analysis of patients’ face. The most important advancement is the number of the classification categories increased to seven, and propose a more detailed holistic analysis.
Generally speaking, if the acne traits are more obvious, the seven-classifier recognition ability is also stronger on this category. Because this type of acne can be better expressed by the 512-dimensional feature vector. In this article, the detection performances of healthy skin, blackhead, whitehead and nodule are better in the automatic diagnosis task, and the detection results of papule, cysts and pustules are relatively poor. The main problem is the 50 × 50 box cannot contain the whole information of cysts and pustules whose disease area is large. The loss of information leads to the effect decline in detection results.
In this paper, we also made a comparison between different binary-classification models. We find the performance of extracting image features by the network we constructed is a little bit weaker than the pre-trained VGG16 model. In the experiment, the ROC was used to evaluate the performance of the two classifiers based on two different CNN models. The experimental results show that the CNN we constructed gains a little bit smaller AUC on the binary-classification test data set, and the detection result is little poorer in the apply task. In seven-classification task, We consider that the small data set and the high similarity of the augmentation data, we use the pre-trained VGG16 model to complete the seven-classification task. So, we use the VGG16 CNN to successfully achieve the transfer learning on small data sets, and to complete the extraction of effective features in the images. The feature vector is regarded as the input of seven-classifier to complete the seven-classification task.
In the future researches, we are prepared to build a more standardized data set, which is supposed to include more types of symptoms and conditions, to meet more standardized training and testing requirements of CNN and the generalization performance. As the data set grows stronger, we will consider to add more detailed description to the automatic diagnosis such as the severe level or the ages of acne vulgaris. In addition, we will consider the use of multiple pre-trained CNN models to replace the current single VGG16 neural network model, to make full use of each neural network model so that we can extract more effective features from the input images.
Furthermore, macro symptoms can be traced back to gene expression. Recent years, many experimental researches have shown that long non-coding RNAs(lncRNAs) and microRNAs(miRNAs) are closely related to human complex diseases. And they could be considered as potential biomarkers for disease diagnosis, treatment and so on 26 , 27 , 28 . Many computational models have been proposed to predict the potential lncRNAs-disease association and miRNAs-disease association 26 , 27 , 28 , 29 , 30 , 31 . These computational models all follow the basic assumption that similar diseases tend to have associations with functionally similar lncRNAs or miRNAs 26 , 27 , 28 , 29 , 30 , 31 . In the future researches, the similarities between facial acne and the diseases in public databases would be computed with the aforementioned computational models to find the associated lncRNAs and miRNAs. It’s obvious that it will help understand the pathogenesis and treatment of facial acne at lncRNA and miRNA level. However, this is also a huge challenge because the known lncRNA-disease associations and the known miRNA-disease associations are rare 27 , 28 .
Williams, H. C., Dellavalle, R. P. & Garner, S. Acne vulgaris. The Lancet 379 , 361–372 (2012).
Article Google Scholar
Bhate, K. & Williams, H. Epidemiology of acne vulgaris. British Journal of Dermatology 168 , 474–485 (2013).
Article CAS PubMed Google Scholar
Hsu, R.-L., Abdel-Mottaleb, M. & Jain, A. K. Face detection in color images. IEEE transactions on pattern analysis and machine intelligence 24 , 696–706 (2002).
Phung, S. L., Bouzerdoum, A. & Chai, D. A novel skin color model in ycbcr color space and its application to human face detection. In Image Processing . 2002 . Proceedings . 2002 International Conference on , vol. 1, I–I (IEEE, 2002).
Vezhnevets, V., Sazonov, V. & Andreeva, A. A survey on pixel-based skin color detection techniques. In Proc . Graphicon , vol. 3, 85–92 (Moscow, Russia, 2003).
Phung, S. L., Bouzerdoum, A. & Chai, D. Skin segmentation using color pixel classification: analysis and comparison. IEEE transactions on pattern analysis and machine intelligence 27 , 148–154 (2005).
Article PubMed Google Scholar
Jones, M. J. & Rehg, J. M. Statistical color models with application to skin detection. International Journal of Computer Vision 46 , 81–96 (2002).
Article MATH Google Scholar
Kim, I., Shim, J. H. & Yang, J. Face detection. Face Detection Project, EE368, Stanford University 28 , 538 (2003).
Chantharaphaichi, T., Uyyanonvara, B., Sinthanayothin, C. & Nishihara, A. Automatic acne detection for medical treatment. In Information and Communication Technology for Embedded Systems (IC-ICTES ) , 2015 6th International Conference of , 1–6 (IEEE, 2015).
Kittigul, N. & Uyyanonvara, B. Automatic acne detection system for medical treatment progress report. In Information and Communication Technology for Embedded Systems (IC-ICTES ) , 2016 7th International Conference of , 41–44 (IEEE, 2016).
Chang, C.-Y. & Liao, H.-Y. Automatic facial skin defects detection and recognition system. In Genetic and Evolutionary Computing (ICGEC ) , 2011 Fifth International Conference on , 260–263 (IEEE, 2011).
Malik, A. S. et al . Digital assessment of facial acne vulgaris. In Instrumentation and Measurement Technology Conference (I2MTC) Proceedings, 2014 IEEE International, 546–550 (IEEE, 2014).
Ciregan, D., Meier, U. & Schmidhuber, J. Multi-column deep neural networks for image classification. In Computer vision and pattern recognition (CVPR ) , 2012 IEEE conference on , 3642–3649 (IEEE, 2012).
LeCun, Y. et al . Backpropagation applied to handwritten zip code recognition. Neural computation 1 , 541–551 (1989).
Sermanet, P., Chintala, S. & LeCun, Y. Convolutional neural networks applied to house numbers digit classification. In Pattern Recognition (ICPR), 2012 21st International Conference on , 3288–3291 (IEEE, 2012).
Hinton, G. E., Srivastava, N., Krizhevsky, A., Sutskever, I. & Salakhutdinov, R. R. Improving neural networks by preventing co-adaptation of feature detectors. arXiv preprint arXiv:1207 . 0580 (2012).
Krizhevsky, A., Sutskever, I. & Hinton, G. E. Imagenet classification with deep convolutional neural networks. In Advances in neural information processing systems , 1097–1105 (2012).
He, K., Zhang, X., Ren, S. & Sun, J. Delving deep into rectifiers: Surpassing human-level performance on imagenet classification. In Proceedings of the IEEE international conference on computer vision , 1026–1034 (2015).
LeCun, Y., Kavukcuoglu, K. & Farabet, C. Convolutional networks and applications in vision. In Circuits and Systems (ISCAS), Proceedings of 2010 IEEE International Symposium on , 253–256 (IEEE, 2010).
Cireşan, D. C., Meier, U., Masci, J., Gambardella, L. M. & Schmidhuber, J. High-performance neural networks for visual object classification. arXiv preprint arXiv : 1102 . 0183 (2011).
Simonyan, K. & Zisserman, A. Very deep convolutional networks for large-scale image recognition. arXiv preprint arXiv : 1409 . 1556 (2014).
Fawcett, T. An introduction to roc analysis. Pattern recognition letters 27 , 861–874 (2006).
Hajian-Tilaki, K. Receiver operating characteristic (roc) curve analysis for medical diagnostic test evaluation. Caspian journal of internal medicine 4 , 627 (2013).
PubMed PubMed Central Google Scholar
Fluss, R., Faraggi, D. & Reiser, B. Estimation of the youden index and its associated cutoff point. Biometrical journal 47 , 458–472 (2005).
Article MathSciNet PubMed Google Scholar
Pedregosa, F. et al . Scikit-learn: Machine learning in python. Journal of machine learning research 12 , 2825–2830 (2011).
MathSciNet MATH Google Scholar
Chen, X., Yan, C. C., Zhang, X. & You, Z.-H. Long non-coding rnas and complex diseases: from experimental results to computational models. Briefings in bioinformatics 18 , 558–576 (2016).
Google Scholar
Chen, X. & Yan, G.-Y. Novel human lncrna–disease association inference based on lncrna expression profiles. Bioinformatics 29 , 2617–2624 (2013).
Chen, X., Xie, D., Zhao, Q. & You, Z.-H. Micrornas and complex diseases: from experimental results to computational models. Briefings in bioinformatics (2017).
Chen, X., Niu, Y.-W., Wang, G.-H. & Yan, G.-Y. Hamda: Hybrid approach for mirna-disease association prediction. Journal of biomedical informatics 76 , 50–58 (2017).
Chen, X. & Huang, L. Lrsslmda: Laplacian regularized sparse subspace learning for mirna-disease association prediction. PLoS computational biology 13 , e1005912 (2017).
Article ADS PubMed PubMed Central Google Scholar
You, Z.-H. et al . Pbmda: A novel and effective path-based computational model for mirna-disease association prediction. PLoS computational biology 13 , e1005455 (2017).
Article PubMed PubMed Central Google Scholar
Download references
Acknowledgements
Thanks to the institution of DOCTOR MIAO NATIONALITY for the provision of data and the support of diagnostic knowledge. The datasets generated and analyzed during the current study are available from the corresponding author on reasonable request.
Author information
Authors and affiliations.
Key Laboratory for Biomedical Engineering of Ministry of Education, Zhejiang University, HangZhou, 310027, China
Xiaolei Shen, Jiachi Zhang, Chenjun Yan & Hong Zhou
You can also search for this author in PubMed Google Scholar
Contributions
H.Z. conceived the experiments; X.L.S. and J.C.Z. designed and performed the experiments; H.Z., X.L.S. and J.C.Z. analyzed the data and results; C.J.Y., X.L.S. and J.C.Z. wrote the manuscript and prepared all tables and figures; These authors contributed equally to this work.
Corresponding author
Correspondence to Hong Zhou .
Ethics declarations
Competing interests.
The authors declare no competing interests.
Additional information
Publisher's note: Springer Nature remains neutral with regard to jurisdictional claims in published maps and institutional affiliations.
Rights and permissions
Open Access This article is licensed under a Creative Commons Attribution 4.0 International License, which permits use, sharing, adaptation, distribution and reproduction in any medium or format, as long as you give appropriate credit to the original author(s) and the source, provide a link to the Creative Commons license, and indicate if changes were made. The images or other third party material in this article are included in the article’s Creative Commons license, unless indicated otherwise in a credit line to the material. If material is not included in the article’s Creative Commons license and your intended use is not permitted by statutory regulation or exceeds the permitted use, you will need to obtain permission directly from the copyright holder. To view a copy of this license, visit http://creativecommons.org/licenses/by/4.0/ .
Reprints and permissions
About this article
Cite this article.
Shen, X., Zhang, J., Yan, C. et al. An Automatic Diagnosis Method of Facial Acne Vulgaris Based on Convolutional Neural Network. Sci Rep 8 , 5839 (2018). https://doi.org/10.1038/s41598-018-24204-6
Download citation
Received : 06 November 2017
Accepted : 20 March 2018
Published : 11 April 2018
DOI : https://doi.org/10.1038/s41598-018-24204-6
Share this article
Anyone you share the following link with will be able to read this content:
Sorry, a shareable link is not currently available for this article.
Provided by the Springer Nature SharedIt content-sharing initiative
This article is cited by
Dermsegnet: smart iot model for multi-class dermatological lesion diagnosis using adaptive segmentation and improved efficientnetb3.
- Rupali Kiran Shinde
- Md.Biddut Hossain
Applied Intelligence (2024)
Automated Facial Acne Lesion Detecting and Counting Algorithm for Acne Severity Evaluation and Its Utility in Assisting Dermatologists
- Dong Hyo Kim
- Dae Hun Suh
American Journal of Clinical Dermatology (2023)
Identification of facial skin diseases from face phenotypes using FSDNet in uncontrolled environment
- Rola El Saleh
- Samer Chantaf
- Amine Nait-ali
Machine Vision and Applications (2022)
Construction and Evaluation of a Deep Learning Model for Assessing Acne Vulgaris Using Clinical Images
Dermatology and Therapy (2021)
Deep neural network based artificial intelligence assisted diagnosis of bone scintigraphy for cancer bone metastasis
Scientific Reports (2020)
By submitting a comment you agree to abide by our Terms and Community Guidelines . If you find something abusive or that does not comply with our terms or guidelines please flag it as inappropriate.
Quick links
- Explore articles by subject
- Guide to authors
- Editorial policies
Sign up for the Nature Briefing newsletter — what matters in science, free to your inbox daily.

A survey of recent advances in analysis of skin images
- Research Paper
- Published: 25 August 2024
Cite this article
- Pragya Gupta 1 ,
- Jagannath Nirmal 1 &
- Ninad Mehendale 1
48 Accesses
1 Altmetric
Explore all metrics
Skin disorders significantly impact quality of life, necessitating advanced diagnostic tools. Machine Learning (ML) and Deep Learning (DL), particularly Convolutional Neural Networks (CNNs), offer promising solutions. ML aids in image segmentation and lesion classification, while DL captures intricate features for improved accuracy. Challenges include limited annotated data, the ‘black box’ nature of AI decision-making, and seamless integration into clinical workflows. Recent advancements such as development of ML and DL algorithms and availability of publicly available datasets, hold promise for earlier diagnoses and improved outcomes in dermatology. Building ML and DL models robust to variations in image quality, lighting conditions, and patient demographics is hence, paramount to enhance the accuracy and efficiency of skin image analysis in clinical practice. In conclusion, while ML and DL techniques show promise, exploring hybrid approaches combining the strengths of both could lead to more robust diagnostic tools for dermatology, revolutionizing skin disorder diagnosis and treatment.
This is a preview of subscription content, log in via an institution to check access.
Access this article
Subscribe and save.
- Get 10 units per month
- Download Article/Chapter or eBook
- 1 Unit = 1 Article or 1 Chapter
- Cancel anytime
Price includes VAT (Russian Federation)
Instant access to the full article PDF.
Rent this article via DeepDyve
Institutional subscriptions
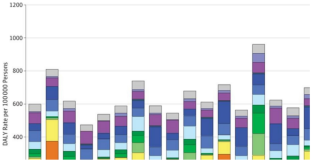
Similar content being viewed by others
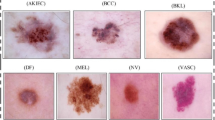
Role of Artificial Intelligence and Deep Learning in Skin Disease Prediction: A Systematic Review and Meta-analysis
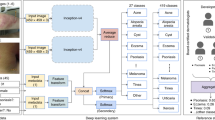
A deep learning system for differential diagnosis of skin diseases
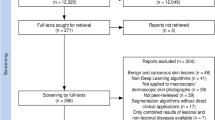
Systematic review of deep learning image analyses for the diagnosis and monitoring of skin disease
Explore related subjects.
- Artificial Intelligence
- Medical Imaging
- Medical Ethics
Data Availability
No datasets were generated or analysed during the current study.
Dermnet NZ. (1996) URL www.dermnetnz.org
ISIC (2016) ISIC Challenge 2016. URL https://challenge.isic-archive.com/data/
ISIC (2017) ISIC Challenge 2017. URL https://challenge.isic-archive.com/data/#2017
ISIC (2018) ISIC Challenge 2018. URL https://challenge.isic-archive.com/data/#2018
ISIC (2019) ISIC Challenge 2019. URL https://challenge.isic-archive.com/data/#2019
ISIC (2020) ISIC Challenge 2020. URL https://challenge.isic-archive.com/data/#2020
Abbas Q, Celebi ME (2019) DermoDeep—a classification of melanoma-nevus skin lesions using multi-feature fusion of visual features and deep neural network. Multimed Tools Appl 78(16):23559–23580
Article Google Scholar
Adegun AA, Viriri S (2020) FCN-based DenseNet framework for automated detection and classification of skin lesions in dermoscopy images. IEEE Access 8:150377–150396
Ahammed M, Mamun MA, Uddin MS (2022) A machine learning approach for skin disease detection and classification using image segmentation. Healthcare Anal. https://doi.org/10.1016/j.health.2022.100122
Ain QU, Al-Sahaf H, Xue B et al (2022) Automatically diagnosing skin cancers from multimodality images using two-stage genetic programming. IEEE Trans Cybern. https://doi.org/10.1109/TCYB.2022.3182474
Akram A, Rashid J, Jaffar MA et al (2023) Segmentation and classification of skin lesions using hybrid deep learning method in the Internet of Medical Things. Skin Res Technol 29(11):e13524
Akram T, Lodhi HMJ, Naqvi SR et al (2020) A multilevel features selection framework for skin lesion classification. Human-centric Comput Info Sci 10:1–26
Google Scholar
Al-Masni MA, Kim DH, Kim TS (2020) Multiple skin lesions diagnostics via integrated deep convolutional networks for segmentation and classification. Comput Methods Progr Biomed 190:105351
Albahar MA (2019) Skin lesion classification using convolutional neural network with novel regularizer. IEEE Access 7:38306–38313. https://doi.org/10.1109/ACCESS.2019.2906241
Arasi MA, El-Horbaty ESM, El-Sayed A (2018) Classification of dermoscopy images using naive bayesian and decision tree techniques. In: 2018 1st Annual international conference on information and sciences (AICIS), pp 7–12
Arnold M, Singh D, Laversanne M et al (2022) Global burden of cutaneous melanoma in 2020 and projections to 2040. JAMA Dermatol 158(5):495–503
Baig AR, Abbas Q, Almakki R et al (2023) Light-Dermo: a lightweight pre-trained convolution neural network for the diagnosis of multiclass skin lesions. Diagnostics. https://doi.org/10.3390/diagnostics13030385
Baig IT, Nguyen QBD, Jahan-Tigh RR et al (2023) Digital photography for the dermatologist. Clin Dermatol 41(1):171–177
Bama S, Velumani R, Prakash NB et al (2021) Automatic segmentation of melanoma using superpixel region growing technique. Mater Today: Proc 45:1726–1732
Bansal N, Sridhar S (2024) HEXA-GAN: skin lesion image inpainting via hexagonal sampling based generative adversarial network. Biomed Signal Process Control 89:105603
Bansal P, Garg R, Soni P (2022) Detection of melanoma in dermoscopic images by integrating features extracted using handcrafted and deep learning models. Comput Ind Eng 168:108060
Barata C, Celebi ME, Marques JS (2014) Improving dermoscopy image classification using color constancy. IEEE J Biomed Health Inform 19(3):1146–1152
Bechelli S, Delhommelle J (2022) Machine learning and deep learning algorithms for skin cancer classification from dermoscopic images. Bioengineering. https://doi.org/10.3390/bioengineering9030097
Cao W, Yuan G, Liu Q et al (2023) ICL-Net: global and local inter-pixel correlations learning network for skin lesion segmentation. IEEE J Biomed Health Inform 27(1):145–156. https://doi.org/10.1109/JBHI.2022.3162342
Chan TF, Vese LA (2001) Active contours without edges. IEEE Trans Image Process 10(2):266–277
Choy SP, Kim BJ, Paolino A et al (2023) Systematic review of deep learning image analyses for the diagnosis and monitoring of skin disease. NPJ Digital Medicine 6(1):180
Christensen RE, Jafferany M (2023) Psychiatric and psychologic aspects of chronic skin diseases. Clin Dermatol 41(1):75–81
Dinnes J, Deeks JJ, Chuchu N et al (2018) Dermoscopy, with and without visual inspection, for diagnosing melanoma in adults. Cochrane Database of Syst Rev. https://doi.org/10.1002/14651858.CD011902.pub2
Escalé-Besa A, Y´elamos O, Vidal-Alaball J, et al (2023) Exploring the potential of artificial intelligence in improving skin lesion diagnosis in primary care. Sci Rep 13(1):4293
Esteva A, Kuprel B, Novoa RA et al (2017) Dermatologist-level classification of skin cancer with deep neural networks. Nature 542(7639):115–118
Flohr C, Hay R (2021) Putting the burden of skin diseases on the global map. Br J Dermatol 184(2):189–190
Garcia-Arroyo JL, Garcia-Zapirain B (2019) Segmentation of skin lesions in dermoscopy images using fuzzy classification of pixels and histogram thresholding. Comput Methods Progr Biomed 168:11–19
Gayatri E, Aarthy SL (2023) Challenges and imperatives of deep learning approaches for detection of melanoma: a review. Int J Image Graph 23(03):2240012
Gessert N, Sentker T, Madesta F et al (2020) Skin lesion classification using CNNs with patch-based attention and diagnosis-guided loss weighting. IEEE Trans Biomed Eng 67(2):495–503. https://doi.org/10.1109/TBME.2019.2915839
Goceri E (2022) Evaluation of denoising techniques to remove speckle and Gaussian noise from dermoscopy images. Comput Biol Med 152:106474
Goyal M, Oakley A, Bansal P et al (2020) Skin lesion segmentation in dermoscopic images with ensemble deep learning methods. IEEE Access 8:4171–4181. https://doi.org/10.1109/ACCESS.2019.2960504
Grignaffini F, Barbuto F, Piazzo L et al (2022) Machine learning approaches for skin cancer classification from dermoscopic images: a systematic review. Algorithms 15(11):438
Gupta C, Gondhi NK, Lehana PK (2019) Analysis and identification of dermatological diseases using Gaussian mixture modeling. IEEE Access 7:99407–99427
Haenssle HA et al (2018) Man against machine: diagnostic performance of a deep learning convolutional neural network for dermoscopic melanoma recognition in comparison to 58 dermatologists. Ann Oncol 29(8):1836–1842. https://doi.org/10.1093/annonc/mdy166
Haenssle HA et al (2020) Man against machine reloaded: performance of a market- approved convolutional neural network in classifying a broad spectrum of skin lesions in comparison with 96 dermatologists working under less artificial conditions. Ann Oncol 31(1):137–143. https://doi.org/10.1016/j.annonc.2019.10.013
Hagerty JR, Stanley RJ, Almubarak HA et al (2019) Deep learning and handcrafted method fusion: higher diagnostic accuracy for melanoma dermoscopy images. IEEE J Biomed Health Info 23(4):1385–1391. https://doi.org/10.1109/JBHI.2019.2891049
Han Q, Wang H, Hou M et al (2023) HWA-SegNet: multi-channel skin lesion image segmentation network with hierarchical analysis and weight adjustment. Comput Biol Med 152:106343
Hanlon KL, Wei G, Braue J et al (2022) Improving dermal level images from reflectance confocal microscopy using wavelet-based transformations and adaptive histogram equalization. Lasers Surg Med 54(3):384–391
Hasan MK, Dahal L, Samarakoon PN et al (2020) DSNet: automatic dermoscopic skin lesion segmentation. Comput Biol Med. https://doi.org/10.1016/j.compbiomed.2020.103738
Hasan MK, Ahamad MA, Yap CH et al (2023) A survey, review, and future trends of skin lesion segmentation and classification. Comput Biol Med 155:106624
Hauser K, Kurz A, Haggenmu¨ller S et al (2022) Explainable artificial intelligence in skin cancer recognition: a systematic review. Eur J Cancer 167:54–69
Hosny KM, Kassem MA, Foaud MM (2019) Classification of skin lesions using transfer learning and augmentation with Alex-net. PLoS ONE. https://doi.org/10.1371/journal.pone.0217293
Hu K, Liu S, Zhang Y et al (2020) Automatic segmentation of dermoscopy images using saliency combined with adaptive thresholding based on wavelet transform. Multimed Tools Appl 79:14625–14642
Jahanifar M, Tajeddin NZ, Asl BM et al (2018) Supervised saliency map driven segmentation of lesions in dermoscopic images. IEEE J Biomed Health Inform 23(2):509–518
Jasil SG, Ulagamuthalvi V (2021) Deep learning architecture using transfer learning for classification of skin lesions. J Am Intell Hum Comput. https://doi.org/10.1007/s12652-021-03062-7
Javed R, Rahim MSM, Saba T et al (2020) A comparative study of features selection for skin lesion detection from dermoscopic images. Netw Mod Anal Health Info Bioinform 9:1–13
Joseph S, Olugbara OO (2022) Preprocessing effects on performance of skin lesion saliency segmentation. Diagnostics 12(2):344
Kadampur MA, Riyaee SA (2020) Skin cancer detection: applying a deep learning based model driven architecture in the cloud for classifying dermal cell images. Inform Med Unlock. https://doi.org/10.1016/j.imu.2019.100282
Karimkhani C, Dellavalle RP, Coffeng LE et al (2017) Global skin disease morbidity and mortality: an update from the global burden of disease study 2013. JAMA Dermatol 153(5):406–412
Karthik R, Vaichole TS, Kulkarni SK et al (2022) Eff2Net: an efficient channel attention-based convolutional neural network for skin disease classification. Biomed Signal Process Control 73:103406
Kasmi R, Hagerty J, Young R et al (2023) SharpRazor: automatic removal of hair and ruler marks from dermoscopy images. Skin Res Technol 29(4):e13203
Khan MA, Akram T, Sharif M et al (2019) Construction of saliency map and hybrid set of features for efficient segmentation and classification of skin lesion. Microsc Res Tech 82(6):741–763
Khan MA, Muhammad K, Sharif M et al (2021) Multi-class skin lesion detection and classification via teledermatology. IEEE J Biomed Health Inform 25(12):4267–4275
Khan MA, Sharif M, Akram T et al (2021) Skin lesion segmentation and multiclass classification using deep learning features and improved moth flame optimization. Diagnostics. https://doi.org/10.3390/diagnostics11050811
Khanvilkar D, Bhatt A (2022) Skin cancer detection from RGB images using the LBP and HOG texture feature descriptors with help of machine learning algorithm. In: 2022 International Conference on signal and information processing (IConSIP), pp 1–5
Kinyanjui NM, Odonga T, Cintas C et al. (2020) Fairness of classifiers across skin tones in dermatology. In: International conference on medical image computing and computer-assisted intervention, pp 320–329
Kowsalya N, Kalyani A, Shree TV et al. (2018) Skin-melanoma evaluation with Tsallis’s thresholding and Chan-Vese approach. In: 2018 IEEE International conference on system, computation, automation and networking (ICSCA), pp 1–5
Lee K, Cavalcanti TC, Kim S et al (2023) Multi-task and few-shot learning-based fully automatic deep learning platform for mobile diagnosis of skin diseases. IEEE J Biomed Health Info 27(1):176–187. https://doi.org/10.1109/JBHI.2022.3193685
Leite M, Parreira WD, da Rocha Fernandes AM et al (2022) Image segmentation for human skin detection. Appl Sci. https://doi.org/10.3390/app122312140
Li X, Desrosiers C, Liu X (2023) Deep neural forest for out-of-distribution detection of skin lesion images. IEEE J Biomed Health Inform 27(1):157–165. https://doi.org/10.1109/JBHI.2022.3171582
López-Leyva JA, Guerra-Rosas E, A´lvarez Borrego J (2021) Multi-class diagnosis of skin lesions using the fourier spectral information of images on additive color model by artificial neural network. IEEE Access 9:35207–35216
Mahbod A, Schaefer G, Wang C et al. (2019) Skin lesion classification using hybrid deep neural networks. In: ICASSP 2019–2019 IEEE International conference on acoustics, speech and signal processing (ICASSP), pp 1229–1233
Mahbod A, Schaefer G, Wang C et al (2020) Transfer learning using a multi-scale and multi-network ensemble for skin lesion classification. Comput Methods Progr Biomed. https://doi.org/10.1016/j.cmpb.2020.105475
Mahum R, Aladhadh S (2022) Skin lesion detection using hand-crafted and DL- based features fusion and LSTM. Diagnostics. https://doi.org/10.3390/diagnostics12122974
Maron RC et al (2019) Systematic outperformance of 112 dermatologists in multiclass skin cancer image classification by convolutional neural networks. Eur J Cancer 119:57–65. https://doi.org/10.1016/j.ejca.2019.06.013
Mendonça T, Celebi M, Mendonca T et al (2015) Ph2: a public database for the analysis of dermoscopic images. In: Celebi ME, Mendonca T, Marques JS (eds) Dermoscopy image analysis. CRC Press, Boca Raton, pp 419–439
Mirikharaji Z, Abhishek K, Bissoto A et al (2023) A survey on deep learning for skin lesion segmentation. Med Image Anal 88:102863
Mishra NK, Kaur R, Kasmi R et al (2019) Automatic lesion border selection in dermoscopy images using morphology and color features. Skin Res Technol 25(4):544–552
Moussaoui H, Akkad NE, Benslimane M (2023) A hybrid skin lesions segmentation approach based on image processing methods. Stat, Optim Info Comput 11(1):95–105. https://doi.org/10.19139/soic-2310-5070-1549
Murugan A, Nair SAH, Kumar KS (2019) Detection of skin cancer using SVM, random forest and kNN classifiers. J Med Syst 43:1–9
Namboodiri TS, Jayachandran A (2020) Multi-class skin lesions classification system using probability map based region growing and DCNN. Int J Comput Intell Syst 13(1):77–84
Vao N, Rajasekar V, Arya MS (2023) Skin lesion segmentation and classification using Fcn-Alexnet framework. J Theo Appl Info Technol 101:24
Nancy VAO, Prabhavathy P, Arya MS (2024) Role of artificial intelligence and deep learning in skin disease prediction: a systematic review and meta-analysis. Ann Data Sci. https://doi.org/10.1007/s40745-023-00503-2
Nauta M, Walsh R, Dubowski A et al (2022) Uncovering and correcting shortcut learning in machine learning models for skin cancer diagnosis. Diagnostics. https://doi.org/10.3390/diagnostics12010040
Nida N, Irtaza A, Javed A et al (2019) Melanoma lesion detection and segmentation using deep region based convolutional neural network and fuzzy C-means clustering. Int J Med Inform 124:37–48
Nugroho AK, Wardoyo R, Wibowo ME et al (2024) Image dermoscopy skin lesion classification using deep learning method: systematic literature review. Bull Electr Eng Inform 13(2):1042–1049
Okuboyejo DA, Olugbara OO (2022) Classification of skin lesions using weighted majority voting ensemble deep learning. Algorithms 15(12):443
Öztürk, Özkaya U (2020) Skin lesion segmentation with improved convolutional neural network. J Digit Imaging 33:958–970
Pacheco AG, Krohling RA (2020) The impact of patient clinical information on automated skin cancer detection. Comput Biol Med. https://doi.org/10.1016/j.compbiomed.2019.103545
Pereira PM, Tavora LM, Fonseca-Pinto R et al. (2019) Image segmentation using gradient-based histogram thresholding for skin lesion delineation. In: Conference: 6th international conference on bioimaging, pp 84–91
Rahman I, Islam MK, Chy AN et al. (2022) Fusion of shallow and deep features for classifying skin lesions. In: 2022 25th International conference on computer and information technology (ICCIT), pp 418–423
Rao R (2016) Jaya: a simple and new optimization algorithm for solving constrained and unconstrained optimization problems. Int J Ind Eng Comput 7(1):19–34
Rashid H, Tanveer MA, Khan HA (2019) Skin lesion classification using GAN based data augmentation. In: 2019 41st annual international conference of the IEEE engineering in medicine and biology society (EMBC), pp 916–919
Riaz F, Naeem S, Nawaz R et al (2018) Active contours based segmentation and lesion periphery analysis for characterization of skin lesions in dermoscopy images. IEEE J Biomed Health Inform 23(2):489–500
Rotemberg V, Kurtansky N, Betz-Stablein B et al (2021) A patient-centric dataset of images and metadata for identifying melanomas using clinical context. Sci data 8(1):34
Saba T (2021) Computer vision for microscopic skin cancer diagnosis using handcrafted and non-handcrafted features. Microsc Res Tech 84(6):1272–1283
Saiwaeo S, Mungmai L, Preedalikit W et al. (2022) A comparative study of image enhancement methods for human skin image. In: 2022 Joint International conference on digital arts, media and technology with ECTI Northern section conference on electrical, electronics, computer and telecommunications engineering (ECTI DAMT & NCON), pp 484–488
Sauter D, Lodde G, Nensa F et al (2023) Deep learning in computational dermatopathology of melanoma: a technical systematic literature review. Comput Biol Med 163:107083
Schaefer G, Rajab MI, Celebi ME et al (2011) Colour and contrast enhancement for improved skin lesion segmentation. Comput Med Imaging Graph 35(2):99–104
Seeja RD, Suresh A (2019) Deep learning based skin lesion segmentation and classification of melanoma using support vector machine (SVM). Asian Pacific J Cancer Prev 20(5):1555–1561. https://doi.org/10.31557/APJCP.2019.20.5.1555
Sekhar KSR, Babu TR, Prathibha G et al (2021) Dermoscopic image classification using CNN with Handcrafted features. J King Saud Univ-Sci 33(6):101550
Senan EM, Jadhav ME (2021) Techniques for the detection of skin lesions in PH2 dermoscopy images using local binary pattern (LBP). In: recent trends in image processing and pattern recognition: third international conference, RTIP2R 2020, Aurangabad, India, January 3–4, 2020, Revised Selected Papers, Part II 3, pp 14–25
Sengupta S, Mittal N, Modi M (2019) Segmentation of skin lesion images using fudge factor based techniques. Adv Interdiscipl Eng: Select Proc FLAME 2018:837–846
Sengupta S, Mittal N, Modi M (2020) Improved skin lesions detection using color space and artificial intelligence techniques. J Dermatol Treat 31(5):511–518
Serte S, Demirel H (2019) Gabor wavelet-based deep learning for skin lesion classification. Comput Biol Med. https://doi.org/10.1016/j.compbiomed.2019.103423
Sharafudeen M (2023) Detecting skin lesions fusing handcrafted features in image network ensembles. Multimed Tools Appl 82(2):3155–3175
Sharma AK, Tiwari S, Aggarwal G et al (2022) Dermatologist-level classification of skin cancer using cascaded ensembling of convolutional neural network and handcrafted features based deep neural network. IEEE Access 10:17920–17932
Shetty B, Fernandes R, Rodrigues AP et al (2022) Skin lesion classification of dermoscopic images using machine learning and convolutional neural network. Sci Rep. https://doi.org/10.1038/s41598-022-22644-9
Singh RK, Gorantla R, Allada SGR et al (2022) SkiNet: a deep learning framework for skin lesion diagnosis with uncertainty estimation and explainability. PLoS ONE. https://doi.org/10.1371/journal.pone.0276836
Solatidehkordi Z, Zualkernan I (2022) Survey on recent trends in medical image classification using semi-supervised learning. Appl Sci 12(23):12094
Song L, Lin J, Wang ZJ et al (2020) An End-to-End Multi-Task Deep Learning Frame- work for Skin Lesion Analysis. IEEE J Biomed Health Inform 24(10):2912–2921. https://doi.org/10.1109/JBHI.2020.2973614
Sreedhar B, BE MS, Kumar MS (2020) A comparative study of melanoma skin cancer detection in traditional and current image processing techniques. In: 2020 Fourth international conference on I-SMAC (IoT in social, mobile, analytics and cloud) (I- SMAC), pp 654–658
Su Q, Hamed HNA, Isa MA et al (2024) A GAN-based data augmentation method for imbalanced multi-class skin lesion classification. IEEE Access. https://doi.org/10.1109/ACCESS.2024.3360215
Sun J, Yao K, Huang G et al (2023) Machine learning methods in skin disease recognition: a systematic review. Processes 11(4):1003
Tamoor M, Naseer A, Khan A et al (2023) Skin lesion segmentation using an ensemble of different image processing methods. Diagnostics 13(16):2684
Tang P, Liang Q, Yan X et al (2019) Efficient skin lesion segmentation using separable-Unet with stochastic weight averaging. Comput Methods Progr Biomed 178:289–301. https://doi.org/10.1016/j.cmpb.2019.07.005
Tiwari AK, Mishra MK, Panda AR et al (2024) Survey on computer-aided automated melanoma detection. Comput Methods Biomech Biomed Eng: Imaging Vis 11(7):2300257
Tsallis C (1988) Possible generalization of Boltzmann-Gibbs statistics. J Stat Phys 52:479–487
Article MathSciNet Google Scholar
Tschandl P, Rosendahl C, Kittler H (2018) The HAM10000 dataset, a large collection of multi-source dermatoscopic images of common pigmented skin lesions. Sci data 5(1):1–9
Ünver HM, Ayan E (2019) Skin lesion egmentation in dermoscopic images with combination of YOLO and grabcut algorithm. Diagnostics 9(3):72
Wang J, Wei L, Wang L et al. (2021) Boundary-aware transformers for skin lesion segmentation. In: Medical image computing and computer assisted intervention MICCAI 2021: 24th International conference, Strasbourg, France, September 27- October 1, 2021, Proceedings, Part I 24, pp 206–216
Wang S, Yin Y, Wang D et al (2022) Interpretability-based multimodal convolutional neural networks for skin lesion diagnosis. IEEE Trans Cybern 52(12):12623–12637. https://doi.org/10.1109/TCYB.2021.3069920
Wang X, Jiang X, Ding H et al (2020) Bi-directional dermoscopic feature learning and multi-scale consistent decision fusion for skin lesion segmentation. IEEE Trans Image Process 29:3039–3051. https://doi.org/10.1109/TIP.2019.2955297
Wei L, Ding K, Hu H (2020) Automatic skin cancer detection in dermoscopy images based on ensemble lightweight deep learning network. IEEE Access 8:99633–99647. https://doi.org/10.1109/ACCESS.2020.2997710
Xie Y, Zhang J, Xia Y et al (2020) A mutual bootstrapping model for automated skin lesion segmentation and classification. IEEE Trans Med Imaging 39(7):2482–2493
Yadav R, Bhat A (2024) A systematic literature survey on skin disease detection and classification using machine learning and deep learning. Multimed Tools Appl. https://doi.org/10.1007/s11042-024-18119-w
Yao P, Shen S, Xu M et al (2022) Single model deep learning on imbalanced small datasets for skin lesion classification. IEEE Trans Med Imaging 41(5):1242–1254. https://doi.org/10.1109/TMI.2021.3136682
Yaqoob MM, Alsulami M, Khan MA et al (2023) Symmetry in privacy-based healthcare: a review of skin cancer detection and classification using federated learning. Symmetry 15(7):1369
Yue G, Wei P, Zhou T et al (2022) Toward multicenter skin lesion classification using deep neural network with adaptively weighted balance loss. IEEE Trans Med Imaging 42(1):119–131
Zhang B, Wang Z, Gao J et al (2021) Short-term lesion change detection for melanoma screening with novel siamese neural network. IEEE Trans Med Imaging 40(3):840–851. https://doi.org/10.1109/TMI.2020.3037761
Zhang J, Xie Y, Xia Y et al (2019) Attention residual learning for skin lesion classification. IEEE Trans Med Imaging 38(9):2092–2103
Zhou L, Liang L, Sheng X (2023) GA-Net: ghost convolution adaptive fusion skin lesion segmentation network. Comput Biol Med 164:107273
Download references
No funding.
Author information
Authors and affiliations.
K.J. Somaiya College of Engineering, Somaiya Vidyavihar University, Vidyavihar, Mumbai, India
Pragya Gupta, Jagannath Nirmal & Ninad Mehendale
You can also search for this author in PubMed Google Scholar
Contributions
Conceptualization was done by Pragya Gupta (PG), Jagannath Nirmal (JN), and Ninad Mehendale (NM). The review work was done by PG. All the summarization was performed by PG,JN, and NM. The manuscript draft was prepared by PG, and corrections were made by JN and NM. Data analysis and graphics designing were done by PG.
Corresponding author
Correspondence to Ninad Mehendale .
Ethics declarations
Conflict of interest.
The authors declare no competing interests.
Additional information
Publisher's note.
Springer Nature remains neutral with regard to jurisdictional claims in published maps and institutional affiliations.
Rights and permissions
Springer Nature or its licensor (e.g. a society or other partner) holds exclusive rights to this article under a publishing agreement with the author(s) or other rightsholder(s); author self-archiving of the accepted manuscript version of this article is solely governed by the terms of such publishing agreement and applicable law.
Reprints and permissions
About this article
Gupta, P., Nirmal, J. & Mehendale, N. A survey of recent advances in analysis of skin images. Evol. Intel. (2024). https://doi.org/10.1007/s12065-024-00977-w
Download citation
Received : 22 January 2024
Revised : 19 June 2024
Accepted : 19 August 2024
Published : 25 August 2024
DOI : https://doi.org/10.1007/s12065-024-00977-w
Share this article
Anyone you share the following link with will be able to read this content:
Sorry, a shareable link is not currently available for this article.
Provided by the Springer Nature SharedIt content-sharing initiative
- Skin analysis
- Machine learning
- Image processing
- Deep learning
- Image segmentation
- Feature extraction
- Image classification
- Find a journal
- Publish with us
- Track your research
Acne is a skin condition that occurs when your hair follicles become plugged with oil and dead skin cells. It causes whiteheads, blackheads or pimples. Acne is most common among teenagers, though it affects people of all ages.
Effective acne treatments are available, but acne can be persistent. The pimples and bumps heal slowly, and when one begins to go away, others seem to crop up.
Depending on its severity, acne can cause emotional distress and scar the skin. The earlier you start treatment, the lower your risk of such problems.
Acne signs vary depending on the severity of your condition:
- Whiteheads (closed plugged pores)
- Blackheads (open plugged pores)
- Small red, tender bumps (papules)
- Pimples (pustules), which are papules with pus at their tips
- Large, solid, painful lumps under the skin (nodules)
- Painful, pus-filled lumps under the skin (cystic lesions)
Acne usually appears on the face, forehead, chest, upper back and shoulders.
When to see a doctor
If self-care remedies don't clear your acne, see your primary care doctor. He or she can prescribe stronger medications. If acne persists or is severe, you may want to seek medical treatment from a doctor who specializes in the skin (dermatologist or pediatric dermatologist).
For many women, acne can persist for decades, with flares common a week before menstruation. This type of acne tends to clear up without treatment in women who use contraceptives.
In older adults, a sudden onset of severe acne may signal an underlying disease requiring medical attention.
The Food and Drug Administration (FDA) warns that some popular nonprescription acne lotions, cleansers and other skin products can cause a serious reaction. This type of reaction is quite rare, so don't confuse it with any redness, irritation or itchiness that occurs in areas where you've applied medications or products.
Seek emergency medical help if after using a skin product you experience:
- Difficulty breathing
- Swelling of the eyes, face, lips or tongue
- Tightness of the throat
Four main factors cause acne:
- Excess oil (sebum) production
- Hair follicles clogged by oil and dead skin cells
- Inflammation
Acne typically appears on your face, forehead, chest, upper back and shoulders because these areas of skin have the most oil (sebaceous) glands. Hair follicles are connected to oil glands.
The follicle wall may bulge and produce a whitehead. Or the plug may be open to the surface and darken, causing a blackhead. A blackhead may look like dirt stuck in pores. But actually the pore is congested with bacteria and oil, which turns brown when it's exposed to the air.
Pimples are raised red spots with a white center that develop when blocked hair follicles become inflamed or infected with bacteria. Blockages and inflammation deep inside hair follicles produce cystlike lumps beneath the surface of your skin. Other pores in your skin, which are the openings of the sweat glands, aren't usually involved in acne.
Certain things may trigger or worsen acne:
- Hormonal changes. Androgens are hormones that increase in boys and girls during puberty and cause the sebaceous glands to enlarge and make more sebum. Hormone changes during midlife, particularly in women, can lead to breakouts too.
- Certain medications. Examples include drugs containing corticosteroids, testosterone or lithium.
- Diet. Studies indicate that consuming certain foods — including carbohydrate-rich foods, such as bread, bagels and chips — may worsen acne. Further study is needed to examine whether people with acne would benefit from following specific dietary restrictions.
- Stress. Stress doesn't cause acne, but if you have acne already, stress may make it worse.
These factors have little effect on acne:
- Chocolate and greasy foods. Eating chocolate or greasy food has little to no effect on acne.
- Hygiene. Acne isn't caused by dirty skin. In fact, scrubbing the skin too hard or cleansing with harsh soaps or chemicals irritates the skin and can make acne worse.
- Cosmetics. Cosmetics don't necessarily worsen acne, especially if you use oil-free makeup that doesn't clog pores (noncomedogenics) and remove makeup regularly. Nonoily cosmetics don't interfere with the effectiveness of acne drugs.
Complications
People with darker skin types are more likely than are people with lighter skin to experience these acne complications:
- Scars. Pitted skin (acne scars) and thick scars (keloids) can remain long-term after acne has healed.
- Skin changes. After acne has cleared, the affected skin may be darker (hyperpigmented) or lighter (hypopigmented) than before the condition occurred.
Risk factors
Risk factors for acne include:
- Age. People of all ages can get acne, but it's most common in teenagers.
- Hormonal changes. Such changes are common during puberty or pregnancy.
- Family history. Genetics plays a role in acne. If both of your parents had acne, you're likely to develop it too.
- Greasy or oily substances. You may develop acne where your skin comes into contact with oil or oily lotions and creams.
- Friction or pressure on your skin. This can be caused by items such as telephones, cellphones, helmets, tight collars and backpacks.
Mayo Clinic Footer
Legal conditions and terms.
- Terms and Conditions
- Privacy Policy
- Notice of Privacy Practices
- Notice of Nondiscrimination
- Manage Cookies
Advertising
Mayo Clinic is a nonprofit organization and proceeds from Web advertising help support our mission. Mayo Clinic does not endorse any of the third party products and services advertised.
- Advertising and sponsorship policy
- Advertising and sponsorship opportunities
Reprint Permissions
A single copy of these materials may be reprinted for noncommercial personal use only. "Mayo," "Mayo Clinic," "MayoClinic.org," "Mayo Clinic Healthy Living," and the triple-shield Mayo Clinic logo are trademarks of Mayo Foundation for Medical Education and Research.

An official website of the United States government
The .gov means it’s official. Federal government websites often end in .gov or .mil. Before sharing sensitive information, make sure you’re on a federal government site.
The site is secure. The https:// ensures that you are connecting to the official website and that any information you provide is encrypted and transmitted securely.
- Publications
- Account settings
Preview improvements coming to the PMC website in October 2024. Learn More or Try it out now .
- Advanced Search
- Journal List
- Front Public Health
- PMC10149786
The burden of skin and subcutaneous diseases: findings from the global burden of disease study 2019
Aobuliaximu yakupu.
1 Department of Burn, Ruijin Hospital, Shanghai Jiao Tong University School of Medicine, Shanghai, China
2 Wound Healing Center, Ruijin Hospital, Shanghai Jiao Tong University School of Medicine, Shanghai, China
Rehanguli Aimaier
3 Department of Plastic and Reconstructive Surgery, Shanghai Ninth People's Hospital, Shanghai Jiao Tong University School of Medicine, Shanghai, China
4 Department of Burn and Plastic Surgery, Guangzhou Red Cross Hospital, Jinan University, Guangzhou, China
5 Department of Burn and Plastic Surgery, Affiliated Hospital of Jiangnan University, Wuxi, China
Yaohua Zhao
6 Department of Burn and Plastic Surgery, Jiangyin Hospital Affiliated to Medical College of Southeast University, Jiangyin, China
7 Department of Burns and Plastic Surgery, Shanghai Ninth People's Hospital, Shanghai Jiao Tong University School of Medicine, Shanghai, China
8 Institute of Traumatic Medicine, Shanghai Jiao Tong University School of Medicine, Shanghai, China
Jiaoyun Dong
Shuliang lu, associated data.
Publicly available datasets were analyzed in this study. This data can be found at: The datasets analyzed during the current study are available in the GBD 2019 repository, which is freely available at http://ghdx.healthdata.org/gbd-results-tool .
The small number of existing integrative studies on the global distribution and burden of all types of skin and subcutaneous diseases hinders relevant comparisons.
This study aimed to determine the latest distribution, epidemiological differences, and factors potentially influencing each skin and subcutaneous disease and the policy implications.
Data on the skin and subcutaneous diseases were obtained from the Global Burden of Disease Study 2019. The incidence, disability-adjusted life years (DALYs), and deaths due to skin and subcutaneous diseases in 204 countries and regions from 1990 to 2019 were analyzed and stratified by sex, age, geographical location, and sociodemographic index (SDI). The annual age-standardized rate of change in the incidence was obtained to evaluate temporal trends.
Of 4,859,267,654 (95% uncertainty interval [UI], 4,680,693,440–5,060,498,767) new skin and subcutaneous disease cases that were identified, most were fungal (34.0%) and bacterial (23.0%) skin diseases, which accounted for 98,522 (95% UI 75,116–123,949) deaths. The burden of skin and subcutaneous diseases measured in DALYs was 42,883,695.48 (95%UI, 28,626,691.71-63,438,210.22) in 2019, 5.26% of which were years of life lost, and 94.74% of which were years lived with disability. The highest number of new cases and deaths from skin and subcutaneous diseases was in South Asia. Globally, most new cases were in the 0–4-year age group, with skin and subcutaneous disease incidence slightly higher in men than in women.
Fungal infections are major contributors to skin and subcutaneous diseases worldwide. Low–middle SDI states had the highest burden of skin and subcutaneous diseases, and this burden has increased globally. Targeted and effective management strategies based on the distribution characteristics of each country are, thus, required to reduce the burden of skin and subcutaneous diseases.
1. Introduction
Skin and subcutaneous diseases, such as acne, alopecia, bacterial skin infections, decubitus ulcers, fungal skin diseases, pruritus, psoriasis, scabies, urticaria, viral skin diseases, and other skin and subcutaneous diseases, are common health problems worldwide and are the leading causes of the global disease burden ( 1 ). Skin and subcutaneous diseases can lead to profound long-term alterations even after the disease has resolved, affecting not only the physical health but also the mental health and quality of life of the patient, placing a high burden on patients' families and national healthcare systems globally ( 2 – 7 ).
Knowledge of skin disease epidemiology is essential for policy development, resource allocation, and disease prevention. However, previous studies have mainly focused on specific skin and subcutaneous diseases or countries, which has led to a lack of geographically comparative information on all skin and subcutaneous diseases at different levels ( 1 , 8 – 14 ). Therefore, the latest spatial distribution and temporal trends in skin and subcutaneous diseases worldwide must be understood to develop policies to establish more reasonable and effective investigation, prevention, and treatment programs to improve patients' quality of life and reduce avoidable medical expenses.
In the present study, to identify the epidemiological differences between each skin and subcutaneous disease at the national, regional, and global levels, we extracted and analyzed annual data on skin and subcutaneous disease incidence, disability-adjusted life years (DALYs), and deaths by location, sex, and age ( 15 ). Our results provide a basis for developing policies and optimizing strategies to manage skin and subcutaneous diseases.
2. Materials and methods
We collected the annual case data and age-standardized rates for skin and subcutaneous disease incidence, deaths, and DALYs from 1990 to 2019 from the Institute for Health Metrics and Evaluation using the Global Health Data Exchange online query tool ( http://ghdx.healthdata.org/gbd-results-tool ) ( 16 ). Data on deaths were, however, only available for certain skin and subcutaneous diseases (e.g., bacterial skin diseases and decubitus ulcers).
A detailed introduction of the original data and general analysis methods of the Global Burden of Disease (GBD) Study 2019 have been described previously ( 15 – 19 ). The GBD estimation process was based on identifying multiple relevant data sources for each skin disease and correcting for known biases. The processed data were then modeled using standardized tools to generate estimates for each item of interest based on age, sex, location, and year. The cause-of-death ensemble model was used to analyze the death data of the causes (skin and subcutaneous diseases, bacterial skin diseases, decubitus ulcers, and other skin and subcutaneous diseases), using standard parameters. The years of life lost (YLLs) for each skin disease were calculated by multiplying the number of estimated deaths by the standard life expectancy at the age of death. The years lived with disability (YLDs) for each skin disease were computed by the sequelae, as prevalence was multiplied by disability weights for the health state associated with that sequela. The DALYs of each skin disease were calculated by adding the YLLs and YLDs. Meta-regression (with Bayesian priors, regularization, and trimming models) was used to evaluate the prevalence and incidence of all skin and subcutaneous diseases. Conceptually, the estimation effort of each skin disease was divided into eight major components: (1) compiling data sources through data identification and extraction, (2) data adjustment, (3) estimation of prevalence and incidence by cause and sequelae using meta-regression with Bayesian priors, regularization, and trimming or alternative modeling strategies for selected cause groups, (4) estimation by impairment, (5) severity distributions, (6) incorporation of disability weights, (7) comorbidity adjustment, and (8) estimation of YLDs by sequelae and causes. Age-standardized rates were calculated by adjusting for population size (per 100,000 individuals) and age distribution.
The 95% uncertainty intervals (UIs) for every metric in the GBD Study 2019 were calculated to reflect the certainty of the estimates, which were determined by the 25th and 975th values of the 1,000 values after ordering them from smallest to largest ( 15 , 16 , 20 ). Data extracted from the GBD Study 2019 were collected from 204 countries and territories (data sources including censuses, household surveys, civil registration and vital statistics, disease registries, health service use, air pollution monitors, satellite imaging, disease notifications, and other sources), and were divided into five regions, according to their sociodemographic index (SDI) developed by GBD researchers. This index is a composite indicator constructed from measures of per capita income, average years of education, and total fertility rate. Briefly, the SDI is the geometric mean of the 0 to 1 index of the total fertility rate for those younger than 25 years old, mean education for those 15 years old and older, and lag-distributed income per capita. After calculating the SDI for the GBD Study 2019, the values were multiplied by 100 on a scale of 0–100. Geographically, the 204 countries and territories are classified into 21 regions based on their locations. The annual age-standardized incidence rate of change was used to estimate disease trends.
To explore the potential impact factors of changing trends, we also calculated the association between universal health coverage (UHC) and gross domestic product (GDP) and the annual age-standardized incidence rate of changes in skin and subcutaneous diseases. The UHC was estimated using the UHC effective coverage index, which provides an understanding of whether health services are aligned with the countries' health profiles and are of adequate quality to produce health gains for populations ( 24 ). We then consider the policy implications of the analyzed data.
2.1. Data analysis
The correlations of the annual age-standardized incidence rate of change with UHC in 2019, GDP in 2019, and SDI value, and the correlations between skin diseases were determined using Pearson correlation analysis ( 21 ). All analyses were performed using R software, version 4.2.1, with two-sided p < 0.05 indicating a statistically significant difference.
3.1. General status of global skin and subcutaneous diseases burden
Globally, 4,859,267,654 new skin and subcutaneous disease cases (95% UI 4,680,693,440–5,060,498,767 cases) were identified in 2019, most of which were fungal and bacterial skin diseases. These accounted for 34% and 23%, respectively [fungal cases: 1,646,596,956 (95% UI 1,493,240,311–1,811,901,501); bacterial: 1,134,138,000 (95% UI 1,105,834,933–1,169,419,691) cases] ( Figure 1A and Supplementary Table S1 ). In total, 98.522 (95% UI, 75.116.11–123.948.56) people died from skin and subcutaneous diseases globally in 2019. This was twice the number in 1990, and most died due to bacterial skin diseases (72%) ( Figure 1C and Supplementary Table S2 ). The highest number of new cases and deaths due to skin and subcutaneous diseases were recorded in South Asia (new cases, 25%; deaths, 25%), and, especially in India (new cases,19%; deaths,25%) ( Figures 1A, C and Supplementary Tables S1 , S2 ). The burden of skin and subcutaneous diseases measured in DALYs in 2019 was 42,883,695.48 (95%UI, 28626,691.71–63,438,210.22), of which 5.26% and 94.74% could be attributed to YLLs and YLDs, respectively. In 2019, most DALYs were caused by bacterial skin diseases (23%) and were concentrated in South Asia (21%), particularly China (19%) ( Figure 1E and Supplementary Table S3 ). From 1990 to 2019, the new and deaths of all types of skin and subcutaneous diseases and their burden measured in DALYs increased substantially. The new cases and DALYs of decubitus ulcers and the number of deaths due to bacterial skin diseases increased from 1990 to 2019 by 106%, 80%, and 104% ( Figures 1A , ,C, C , ,E E ).

General status of global skin and subcutaneous diseases burden. (A) New cases of each skin and subcutaneous disease globally from 1990 to 2019. (B) ASIR of each skin and subcutaneous disease globally from 1990 to 2019. (C) Deaths from each skin and subcutaneous disease globally from 1990 to 2019. (D) ASDR from each skin and subcutaneous disease globally from 1990 to 2019. (E) DALYs from each skin and subcutaneous disease globally from 1990 to 2019. (F) ASDAR from each skin and subcutaneous disease globally from 1990 to 2019. ASIR, age-standardized incidence rate; DALYs, disability-adjusted life years; ASDAR, age-standardized DALYs rate; ASDR, age-standardized death rate.
For the age-standardized rates, the skin and subcutaneous diseases associated with the highest recorded age-standardized incidence rate (ASIR), age-standardized DALYs' rate (ASDAR), and age-standardized death rate (ASDR) in 2019 were fungal skin diseases (21,276.58 [95% UI, 19,297.52–23,398.73]), dermatitis (131.67 [95% UI, 77.59–206.87]), and bacterial skin diseases (0.92 [95% UI, 0.67–1.14]) ( Figures 1C , ,D, D , ,F). F ). Overall, the ASDAR and ASDR tended to decrease with slight fluctuations, whereas the ASIR continued to increase from 1990 to 2019 ( Figures 1C , ,D, D , ,F). F ). However, from the perspective of different skin and subcutaneous diseases, the ASIR of scabies, alopecia areata, psoriasis, decubitus ulcers, and viral skin diseases decreased, and the ASDAR of acne vulgaris, fungal skin diseases, pruritus, urticaria, other skin, and subcutaneous diseases, and the ASDR of bacterial skin diseases increased. The skin and subcutaneous diseases that showed a significant increase in the ASIR, ASDAR, and ASDR were acne vulgaris (18%), acne vulgaris (16%), and bacterial diseases (6%).
3.2. Skin and subcutaneous diseases burden among the different sexes and ages
Globally, in 2019, the new cases of skin and subcutaneous diseases in men were slightly higher than in women (men: 2,446,443,162.05 [95%UI, 2,349,576,424.39–2,548,351,122.13]; women: 2,412,824,491.40 [95%UI, 2,323,159,191.20–2,509,074,646.14]) ( Figure 2A ). Fungal skin diseases were the most common skin diseases in both sexes but tended to occur more frequently in men; their ASIR was the highest for both sexes, especially in men (22,075.82, 95%UI [19,998.15–24,357.26]) ( Figure 2B ). Based on 2019 data, the disease with the greatest difference in incidence between men and women is alopecia areata, which is more common in women. Dermatitis was also more common in women, and their ASIR was higher ( Figures 2A , ,B). B ). The skin and subcutaneous diseases that were more common in men were bacterial skin and subcutaneous diseases and scabies, whereas, in women, scabies, acne vulgaris, urticaria, alopecia areata, pruritus, and decubitus ulcers were more common. Scabies was notably prevalent in both sexes ( Figure 2A ). Viral skin diseases and psoriasis were almost evenly distributed among men and women ( Figure 2A ). The DALYs among women were slightly higher than those among men, and the ASDAR among women was also slightly higher than that among men in 2019 ( Supplementary Figures S1A , B ). Dermatitis contributed to the highest DALYs among the skin and subcutaneous diseases in both sexes and was associated with the highest recorded ASDAR in women (151.79 [95%UI, 89.09–239.06]) ( Supplementary Figures S1A , B ). The number of deaths from skin and subcutaneous diseases was higher in women than in men in 2019, whereas the ASDR was higher in men ( Supplementary Figures S1C , D ). Bacterial skin diseases accounted for the highest number of deaths among skin and subcutaneous diseases in both sexes, particularly in women ( Supplementary Figures S1C , D ).

Skin and subcutaneous diseases burden among different sexes and ages. (A) Each skin and subcutaneous disease's new cases distributions by sex in 2019. (B) Each skin and subcutaneous disease ASIR distribution by sex in 2019. (C) Global changing trend in each skin and subcutaneous disease ASIR by sex from 1990 to 2019. (D) Distribution of all skin and subcutaneous diseases new cases globally and SDI levels among different age categories in 2019. (E) Distribution of each skin and subcutaneous disease new cases among different age categories in 2019. (F) Distribution of each skin and subcutaneous disease incidence rate among different age categories in 2019. ASIR, age-standardized incidence rate; SDI, sociodemographic index.
From 1990 to 2019, the proportion of men and women with each type of skin and subcutaneous disease did not change significantly ( Supplementary Figure S2A ). During this period, the number of new cases, DALYs, and deaths increased substantially each year in both sexes ( Supplementary Figures S2B , C , E ). Compared to 1990, the types of skin and subcutaneous diseases that increased the most in new cases (108%), deaths (96%), and DALYs (80%) in men were decubitus ulcers, while decubitus ulcers also increased the most in women with increased new cases (103%) and DALYs (79%) ( Supplementary Table S4 ). However, the types of skin and subcutaneous diseases that increased the most concerning deaths in women were bacterial skin diseases, which increased by 119% ( Supplementary Table S4 ). With age-standardized rates, the ASDAR and ASDR tended to decrease with slight fluctuations, whereas the ASIR increased in both sexes ( Figure 2C and Supplementary Figures S2D , F ). However, the ASIR and ASDAR increased or decreased for different skin and subcutaneous diseases. In both sexes, the diseases that increased or decreased in the ASIR or ASDAR were the same as those at the global level as described previously. For ASDR, only the ASDR of bacterial skin diseases increased in women, whereas the other diseases decreased in both sexes ( Supplementary Table S4 ). From the perspective of sex, the skin and subcutaneous diseases that showed the greatest increases in ASIR, ASDAR, and ASDR were acne vulgaris (men: 21%; women: 21%), acne vulgaris (men: 15%; women: 13%), and bacterial skin diseases (women: 13%), respectively ( Supplementary Table S4 ).
Skin and subcutaneous diseases were more common in young patients, and most new cases were in the 0–4-year age group in 2019 ( Figure 2D ). DALYs were also high in the younger population, and the highest DALYs were observed in the 15–19-year age group; however, deaths were concentrated in those aged 80+ years for both sexes ( Supplementary Figures S3B , D ). ASIR, ASDAR, and ASDR were all higher in older individuals in 2019 ( Supplementary Figures S3A , C , E ). In addition, scabies, acne vulgaris, urticaria, and viral skin diseases were more common in the younger generation and contributed to the highest DALYs, while decubitus ulcers were more common in older individuals, contributing to the highest DALYs and deaths ( Figure 2E and Supplementary Figures S4A , B ). Fungal skin diseases, pruritus, and decubitus ulcers demonstrated significantly higher ASIR and ASDAR in older patients, whereas scabies, urticaria, and viral skin diseases are associated with high ASIR and ASDAR values in young individuals. In addition, bacterial skin diseases were associated with significantly higher ASDAR and ASDR values in older individuals ( Figure 2F and Supplementary Figures S4C , D ). From 1990 to 2019, there was little change in the age distribution of all the skin and subcutaneous diseases ( Supplementary Figure S3F ).
3.3. Skin and subcutaneous diseases burden among different social-economic status
From the perspective of the different SDI levels, countries with middle SDI levels had the highest number of new cases of skin and subcutaneous diseases (1,456,208,410 [95%UI, 1,399,357,076–1,514,043,198]), accounting for 30% of the global new cases in 2019, and had the highest number of new cases from 1990 to 2019 ( Figure 3A ). The number of new cases increased for all the SDI levels from 1990 to 2019; it significantly increased for the low SDI level (in 2019: 766,636,889.27 [95%UI, 721,990,381.15–814,942,421.22]), and the increase was 113% ( Figure 3A ). The highest ASIR was recorded for the low SDI level in 2019 (71,593.23, 95%UI [68,370.55–75,077.99]), but the ASIR increased for all SDI levels except the high SDI level from 1990 to 2019; the highest increase for those years was found at the middle SDI level (61,038.54 [95%UI, 58,612.56–63,536.31]), an increase of 3% ( Figure 3B ). Skin and subcutaneous diseases were more common in the young age groups for the lower SDI levels. However, they were more common in older patients at higher SDI levels, and the ASIR was higher in older individuals at all SDI levels in 2019 ( Figure 2D and Supplementary Figure S3A ). The number of new cases was higher in women than in men with higher SDI levels, whereas it was higher in men with lower SDI levels in 2019 ( Figure 3C ). Except for the high SDI level, the ASIR was higher in men at all SDI levels in 2019 ( Figure 3D ).

Skin and subcutaneous diseases burden among different social–economic states. (A) Changing trend in each skin and subcutaneous disease new cases by global and SDI levels from 1990 to 2019. (B) Changing trend in each skin and subcutaneous disease ASIR by global and SDI levels from 1990 to 2019. (C) Skin and subcutaneous diseases new cases distribution by sex at different SDI levels in 2019. (D) Skin and subcutaneous diseases ASIR distribution by sex at different SDI levels in 2019. (E) The proportion of new cases of each skin and subcutaneous disease at different SDI levels from 1990 to 2019. (F) Type of skin and subcutaneous diseases that contribute the highest new cases, DALYs, and deaths at different SDI levels from 1990 to 2019. (G) SDI levels recorded the highest new cases, DALYs, and deaths of each skin and subcutaneous disease from 1990 to 2019. ASIR, age-standardized incidence rate; DALYs, disability-adjusted life years; SDI, sociodemographic index.
In 2019, the highest DALYs were recorded for the middle SDI level [13,062,128.19 (95%UI, 8,598,805.41–19,549,276.27]). The DALYs increased for all SDI levels from 1990 to 2019, and the middle SDI level had the highest DALYs compared to the other SDI levels in this same period, whereas the DALYs significantly increased (by 110%) at the low SDI level (In 2019: 5,956,125.74 [95%UI, 3,925,718.84–8,999,847.35]) ( Supplementary Figure S5A ). From 1990 to 2019, the highest ASDAR was recorded at the high SDI level, and it significantly decreased at all SDI levels, except for the high-middle SDI level ( Supplementary Figure S5B ). The DALYs were also high in the younger population, whereas the ASDAR was higher in the older population at all SDI levels ( Supplementary Figures S3B , C ). Concerning the sex ratios of the DALYs and ASDARs among SDI levels, these were higher in women at all SDI levels in 2019 ( Supplementary Figures S5C , D ).
In 2019, the highest number of deaths was recorded at the middle SDI level (25,814.65, 95%UI [21,781.51–30,732.72]). Deaths increased at all SDI levels from 1990 to 2019, and the middle SDI level had the highest number of deaths at the same time, whereas the number of deaths significantly increased at the high-middle SDI level (In 2019: 20,014.62 [95%UI, 13,431.51–26,288.53]), with an increase of 138% ( Supplementary Figure S5E ). From 1990 to 2019, the highest ASDR was recorded at the low–middle SDI level. The ASDR decreased at lower SDI levels and increased at higher SDI levels ( Supplementary Figure S5F ). The deaths were concentrated in those aged 80+ years, and the ASDR was also recorded to be higher in the older population at all SDI levels ( Supplementary Figures S3D , F ). In terms of sex, the number of women who died was higher than men at all SDI levels in 2019. However, at higher SDI levels, the ASDR values were higher in men than in women in 2019 ( Supplementary Figures S5G , H ).
At all SDI levels, the skin and subcutaneous diseases that contributed to the highest number of new cases, DALYs, and deaths were fungal skin diseases, dermatitis, and bacterial skin diseases, respectively ( Figures 3E , ,F F and Supplementary Figures S6A , B ). Except for bacterial skin diseases and psoriasis, and the new cases of the decubitus ulcer, the SDI level associated with the highest number of new cases, DALYs, and deaths of different skin and subcutaneous diseases were all at the middle SDI level ( Figure 3G ). At all SDI levels, the skin and subcutaneous diseases that contributed the highest ASIR, ASDAR, and ASDR were fungal skin diseases, dermatitis, and bacterial skin diseases, respectively ( Supplementary Figures S6C – F ). The SDI values associated with the highest recorded ASIR, ASDAR, and ASDR values for each skin and subcutaneous disease are shown in Supplementary Figure S6G .
3.4. Skin and subcutaneous diseases burden in different regions
The absolute number of new cases, DALYs, and deaths due to skin and subcutaneous diseases increased in almost all GBD regions. South Asia had the highest number of new cases, DALYs, and deaths in 2019 (incidence: 1,208,952,415.44 [95% UI 1,162,257,056.17–1,259,536,849.02]; DALYs: 8,965,385.45 [95%UI, 5,949,896.33–13,202,307.74]; deaths: 8,965,385.45 [95%UI, 5,949,896.33–13,202,307.74]), which increased by 55%, 45%, and 71%, respectively, from 1990 and accounted for 21%, 20%, and 25% of the total global new cases, DALYs, and deaths, respectively. However, Oceania had the fewest new cases of DALYs in 2019 (incidence: 7,826,121.01 [95% UI, 7,387,971.02–8,259,634.86]; DALYs: 88,973.81 [95%UI, 58,072.08–129,933.28]) and the lowest number of deaths recorded in Central Asia (166.76 [95% UI, 114.60–249.40]). From 1990 to 2019, Western Sub-Saharan Africa exhibited the highest percentage increase in new cases and DALYs, increasing by 137% and 140%, respectively (in 2019, incidence: 336,459,662.65 [95% UI, 313,416,523.10–361,249,639.71]; DALYs: 2,329,711.49 [95% UI, 1,543,480.32–3,570,126.42]). The most significant increase in deaths detected in Tropical Latin America was 363% (1,639.44 [95% UI, 1,134.41–2,772.35] to 7,592.79 [95% UI, 3,452.25–9,329.05]).
From 1990 to 2019, the skin and subcutaneous diseases that contributed the most to the incidence in each region were mostly fungal skin diseases, followed by bacterial skin diseases, in which pyoderma was the most prevalent ( Figure 4 ). During the same period, the greatest contributors to DALYs and deaths in each region were dermatitis and bacterial skin diseases, respectively ( Supplementary Figures S7A , B , S8A ). The highest number of acne vulgaris, alopecia areata, dermatitis pruritus, scabies, viral skin diseases, and other skin and subcutaneous diseases was recorded in East Asia; the highest number of bacterial, fungal skin diseases, and urticaria was recorded in South Asia; the highest number of decubitus ulcers was recorded in high-income North America; and the highest number of decubitus ulcers was recorded in high-income North America. Except for acne vulgaris, decubitus ulcer, and viral skin diseases, the regions associated with the highest recorded DALYs and deaths associated with each skin and subcutaneous disease were the same as the regions that recorded the highest number of new cases; the regions associated with the highest recorded DALYs of acne vulgaris, decubitus ulcer, and viral skin diseases were East Asia, Southeast Asia, and East Asia, while the highest number of decubitus ulcers was found in Southeast Asia ( Supplementary Figure S8B ). The most geographically differentiated skin and subcutaneous disease is scabies, which is mainly distributed in East, South, and Southeast Asia and in Andean, Central, and Latin America, and is also the most common type of skin and subcutaneous disease in Oceania ( Supplementary Figure S9 ).

Skin and subcutaneous diseases burden in different regions. The proportion of new cases of each skin and subcutaneous disease at different GBD regions (21) in 1990, 2000, 2010, and 2019. GBD, Global Burden of Disease.
From 1990 to 2019, the ASIR, ASDAR, and ASDR increased in most regions. Southern Sub-Saharan Africa had the highest ASIR in 2019 (80,648.03 [95% UI, 77,453.40–84,092.88]), whereas high-income North America had the lowest ASIR (37,643.70 [95% UI, 36,577.88–38,763.59]). The greatest percentage reduction detected was in Central Europe (from 55,172.28 [95% UI, 52,989.80–57,557.31] to 54,784.19 [95% UI, 52,593.09–57,195.97]), whereas the most significant increase was detected in Central Latin America (from 63,994.58 [95% UI, 66,214.65–61,814.18] to 65,539.00 [95% UI, 63,387.70–67,847.44]). The highest ASDAR was observed in 2019 in Western Europe (739.59 [95%UI, 505.92–1,047.66]) and the lowest was in North Africa and the Middle East (419.87 [95%UI, 282.04–618.05). The most significant decrease in ASDAR was detected in high-income North America (from 681.57 [95% UI, 472.83–952.48] to 655.73 [95% UI, 455.25–918.96]), whereas the most significant increase was detected in Tropical Latin America (from 623.14 [95% UI, 420.53–918.43] to 650.68 [95% UI, 443.80–939.85]). Southern Latin America had the highest ASDR in 2019 and the most significantly increased ASDR among the 21 GBD regions (from 1.86 [95% UI, 1.27–4.19] to 3.61 [95% UI, 2.14 −5.31]). Central Asia had the lowest ASDR in 2019 (0.25 [95% UI, 0.17–0.36]) and the most significant decrease in ASDR was detected in East Asia (In 2019: 0.46 [95% UI, 0.39–0.57]). In most GBD regions, fungal and bacterial skin diseases had the highest ASIR, dermatitis had the highest ASDAR, and bacterial skin and subcutaneous diseases had the highest ASDR ( Supplementary Figures S10A–C ). Detailed information about the contribution of each disease concerning age-standardized rates in every region and the highest age-standardized rates for each disease was recorded and the information as to which region can be seen in Supplementary Figures S8C , D .
The new cases and the DALYs' distribution of skin and subcutaneous diseases by sex and age in the GBD regions are generally consistent with the global situation ( Supplementary Figures S11A , B ). However, in Central and Eastern Europe, high-income North America, and Southern Latin America, the proportion of female patients increased significantly, whereas the proportion of male patients increased significantly in North Africa, the Middle East, Oceania, and South Asia ( Supplementary Figures S11A , B ). The skin and subcutaneous diseases with large regional differences regarding the new cases in men and women were acne vulgaris and decubitus ulcers ( Supplementary Figures S11A , B ). Compared with the global age distribution of skin and subcutaneous diseases, patients with all skin and subcutaneous diseases tend to be younger in Central, Eastern, and Western Sub–Saharan Africa, whereas patients tend to be older in Australasia, Central Europe, High–income Asia Pacific, High–income North America, Tropical Latin America, and Western Europe ( Supplementary Figures S11C , D ). The diseases for which the age distribution varied greatly among the regions were bacterial skin diseases, fungal skin diseases, scabies, and urticaria ( Supplementary Figure S11C ). Concerning the distribution of skin and subcutaneous diseases by sex and age in the GBD regions of Asia, Central, Eastern, and Southern Sub-Saharan Africa, Southern Latin America, and Western Europe, the proportion of female patients increased significantly, while the proportion of male patients increased significantly in Oceania ( Supplementary Figure S11E ). Patients with all skin and subcutaneous diseases in Central, Eastern, and Western Sub-Saharan Africa tended to be younger ( Supplementary Figure S11F ). A detailed description of the diseases from the perspective of countries and territories can be found in the Supplementary material , which enable policymakers to address the particular skin and subcutaneous diseases affecting their populations.
3.5. Trend of skin and subcutaneous diseases and their relevance with social-economic states
We analyzed the temporal trends of different skin and subcutaneous diseases at the regional, SDI, and global levels using the annual age-standardized rate of change in incidence. The results showed that skin and subcutaneous diseases are anticipated to increase globally, especially in low–middle SDI states ( Figure 5A ). Most skin and subcutaneous diseases exhibited different trends at different levels, whereas acne vulgaris and psoriasis tended to increase and decrease, respectively, at all levels ( Figure 5A ). Temporal trends of different skin and subcutaneous diseases at the national level are shown in the Supplementary Figure S12 .

The trend of skin and subcutaneous diseases and the relevance with social-economic states. (A) Trends of each skin and subcutaneous disease at global, different SDI levels, and different GBD regions (21). (B) Correlation analysis of each skin and subcutaneous disease annual age-standardized incidence rate of changes with SDI, UHC, and GDP among 204 countries and territories in 2019. GBD, Global Burden of Disease; SDI, sociodemographic index; UHC, universal health coverage; GDP, gross domestic product.
The socioeconomic status of each nation can be evaluated by the SDI and/or GDP, and UHC is one of the indices used to estimate countries' healthcare system performance ( 22 , 23 ). To better understand the distribution of skin and subcutaneous diseases based on the SDI, GDP, and healthcare system performance of countries, we performed a correlation analysis between the SDI, GDP, and UHC values of 204 countries/territories in 2019 and the annual age-standardized rate of change in incidence. The SDI, GDP, and UHC values were negatively correlated with most skin and subcutaneous diseases' annual age-standardized rate of change in incidence, indicating that the incidence of most diseases declined with increasing SDI, GDP, and UHC values, suggesting that the SDI, GDP, and UHC indices have a vital impact on the temporal trends of skin and subcutaneous diseases ( Figure 5B ). However, the annual age-standardized rate of change in the incidence of some skin and subcutaneous diseases, such as fungal skin diseases, psoriasis, pruritus, and scabies, was positively correlated with the SDI, GDP, and UHC. This indicates that some skin and subcutaneous diseases will become increasingly prevalent in high socioeconomic states. Furthermore, we analyzed the relationship between skin and subcutaneous diseases and skin cancer, using the annual age-standardized rate of change in incidence. The results showed that psoriasis negatively correlated with acne vulgaris (r=-0.77), urticaria positively correlated with alopecia areata (r= 0.62), and viral skin diseases positively correlated with seborrheic dermatitis (r= 0.55) ( Supplementary Figure S13 ).
4. Discussion
In this study, we systematically assessed the burden of each skin and subcutaneous disease in terms of national, regional, global, sex, age, and socioeconomic status, including dermatitis (atopic, contact, and seborrheic), bacterial skin diseases (cellulitis and pyoderma), scabies, fungal skin diseases, viral skin diseases, acne vulgaris, alopecia areata, pruritus, urticaria, decubitus ulcers, and other skin and subcutaneous diseases. This study revealed the disparity in skin and subcutaneous disease burdens among different regions and countries.
The nations that recorded the highest number of skin and subcutaneous diseases were mostly India and China, mainly because of their large populations. The skin and subcutaneous diseases contributing the highest burden in most regions are fungal skin diseases, followed by bacterial skin diseases. Fungal skin diseases (such as dermatophytosis, subcutaneous opportunistic mycoses, talaromycosis, emergomycosis, and cutaneous phaeohyphomycosis), can occur not only as primary diseases but also because of the dissemination of systematic infections, and present with a diverse spectrum of infection extent, severity, and features ( 24 ). They have an ever-growing incidence in or outside their endemic areas, because of the increasing number of immunocompromised patients and worldwide traveling ( 25 , 26 ). Bacterial skin diseases, such as cellulitis and pyoderma, have also been increasing ( 27 ). Moreover, fungal infections are a risk factor for cellulitis ( 28 ). Bacterial skin diseases were the main contributors to deaths, indicating that fungal infections can increase the number of deaths. We also found that the ASIR of fungal skin diseases were higher in older individuals, which may be due to age-related anatomical, physiological, and environmental factors that increase their susceptibility to skin infections ( 29 ). Therefore, we need to pay more attention should be paid to older adults with fungal or bacterial infections.
Alopecia areata is a common inflammatory disease; however, its cause remains incompletely understood ( 30 , 31 ). Although previous studies have reported that alopecia areata affects both sexes equally or men more commonly, we found it to be more common in women ( 32 , 33 ). This may be because an unidentified factor makes women susceptible to alopecia areata, or the prevalence of the subtype of alopecia areata, such as acute diffuse and total alopecia Marie-Antoinette syndrome, mostly in women's scalps ( 32 , 34 ), is increasing. Unfortunately, the GBD Study 2019 did not provide data for each subtype of alopecia areata. As previously reported, scabies, acne vulgaris, and urticaria are more common in the young ( 35 – 37 ). Although we found that the combined incidence of viral skin diseases was higher in the young, several subtypes of viral skin diseases were observed mostly in older adults ( 38 , 39 ). Decubitus ulcers are more common in older individuals and contribute to the highest number of deaths, possibly because they are primarily associated with chronic health conditions, and their global prevalence is increasing globally ( 40 , 41 ).
The number of each type of skin and subcutaneous disease Increased from 1990 to 2019 worldwide; however, the ASIR of different types of skin and subcutaneous diseases exhibited different trends. The ASIR of decubitus ulcers, alopecia areata, psoriasis, viral skin diseases, and scabies decreased, while those of others increased globally, which highlights the remarkable achievements in preventing these diseases. Skin and subcutaneous diseases are common in patients with lower SDI scores, and most of their incidences are negatively correlated with SDI, GDP, and UHC. However, psoriasis, pruritus, and scabies are becoming increasingly prominent in high socioeconomic states. This may be due to the interplay between many factors, such as increased risk factors, differences in lifestyle and cultural perceptions, and overcrowding ( 1 , 42 , 43 ). The annual age-standardized incidence rate of change of most skin and subcutaneous diseases is negatively correlated with UHC, indicating that UHC has a vital impact on disease prevalence.
Moreover, public health policy is an important determining factor for access to healthcare, as it can influence aspects of health service delivery such as the availability of resources, organization, and financing ( 44 ). Healthcare policies for skin diseases vary by country and depend on factors such as the prevalence, severity, cost, and availability of treatments for different skin conditions. For example, in India, most dermatological or skin problems are covered by health insurance plans that can help patients afford treatment. However, some skin conditions, such as vitiligo, may not be covered by insurance owing to social stigma or a lack of awareness ( 11 ). In South Korea, the National Health Insurance Service covers most dermatological services and drugs; however, some cosmetic procedures or treatments that are not considered medically necessary may require out-of-pocket payments. In the United Kingdom, the National Health Service provides free dermatology services and prescriptions for skin diseases to all residents; however, there may be long waiting times or limited access to specialized treatments or referrals. In Brazil, the Unified Health System offers universal and free access to dermatology services and medications for skin diseases; however, there are challenges such as insufficient funding, human resources, and infrastructure. Generally speaking, these healthcare policies have benefited numerous people, for example, in Sub-Saharan Africa, where HIV-related skin problems are prevalent, a series of disease prevention policies and, in particular, in many countries the availability of free anti-retroviral drugs have significantly reduced the number of deaths and improved the quality of life ( 45 ).
However, skin diseases do not receive due importance in national health planning and policies in several countries, and there may be gaps or challenges in accessing quality and affordable care for skin conditions ( 46 ). No single best policy suits all countries because each country has its own needs, resources, and priorities. However, according to the World Health Organization (WHO), some common elements that may improve healthcare policies for skin diseases include increasing awareness and education about skin diseases among the public and healthcare providers and integrating approaches for the prevention, diagnosis, treatment, and management of skin diseases across different levels of the healthcare system. The WHO suggests that countries strengthen surveillance and data collection concerning the burden and impact of skin diseases; promote research and innovation for new diagnostics, therapies, and interventions for skin diseases; and enhance collaboration and coordination among stakeholders such as governments, health organizations, civil society groups, and patients. Furthermore, the integration of management strategies has the potential to significantly and cost-effectively bolster health systems in resource-poor areas, leading to improvements in the skin and overall health of many of the world's most disadvantaged individuals ( 47 ). Skin diseases affect people's quality of life, self-esteem, social relationships, and mental health. Providing support groups or online forums for people with skin diseases can help reduce feelings of isolation, stigma, or shame associated, for example, with acne vulgaris by sharing experiences, challenges, and coping strategies with others who understand what they are experiencing ( 48 ). Additionally, public health policies should timeously adjust to changes in disease risk factors and patterns. For example, with population growth and aging, the number of chronic skin wounds is increasing significantly, and China is accelerating the development of wound repair departments and relevant policies for managing chronic skin wounds ( 49 ). With the increase in global communication, it is necessary to develop a global control program for skin diseases that will effectively help measure and reduce the burden of skin diseases worldwide ( 50 – 52 ). Other actions such as increasing awareness and education about skin diseases and their prevention among the public and healthcare workers are suitable for countries with limited funding, especially low- and middle-income countries ( 14 , 53 ). Local governments struggle to provide adequate medicines and laboratory facilities to improve the diagnosis and treatment of skin diseases. They can set up publicly available webpages to provide information on common skin diseases, raise awareness, and encourage the early detection of skin diseases through media campaigns and community events. Our study indicates that the prevalence of different skin diseases varies from country to country. Strengthening surveillance and data collection on skin disease prevalence on a global scale will help establish more reasonable and effective investigation, prevention, and treatment programs. Thus, it is necessary to establish standardized disease surveillance and data collection procedures and protocols. Additionally, it is necessary to develop a unified system for disease diagnosis and registration. In addition, research resources for different skin diseases should be directed more toward countries where the different types of skin diseases are prevalent, which will enable the effective use of resources and can help obtain more convincing research results in a shorter time because of the large number of patients. Our study also highlights the importance of an integrated public health policy in different countries to promote public health more effectively. Globally, guidance for skin disease control is indispensable.
From our correlation analysis, psoriasis was negatively correlated with acne vulgaris, and the ASIR decreased with increasing acne ASIR. Psoriasis and acne vulgaris are both immune-mediated inflammatory diseases; however, psoriasis is mainly mediated by IL-17 and IL-23, and acne vulgaris by IL-1 ( 36 , 54 ), which may result in unique inflammatory circuits that promote different pathological processes. In addition, urticaria is positively correlated with alopecia areata, and viral skin diseases are positively correlated with seborrheic dermatitis, all of which are inflammatory diseases. Although the pathogenic relevance of different skin diseases may be determined by further in-depth genetic analyses and studies, the results provide new insights into skin disease investigation and emphasize the importance of integrative investigation, prevention, and treatment of different skin diseases.
4.1. Limitations
This study has limitations that are generally associated with GBD studies. First, the accuracy of GBD estimation depends largely on the quality and quantity of data used, as it is associated with underreporting and underdiagnosis during skin and subcutaneous disease registration. Second, the data could not be explored further to extract information related to the subtype of each skin and subcutaneous disease, severity, and treatment because such information was not provided by the Global Health Data Exchange. Therefore, we could not obtain a detailed etiological understanding of the global changes in the skin and subcutaneous disease patterns. Finally, owing to the observational nature of this analysis, some unmeasured confounding factors may not have been discussed, and causal statements could not be made about the trends observed.
4.2. Conclusion
Fungal skin diseases are the major contributors to skin and subcutaneous diseases worldwide. The low–middle SDI states had the highest burden of skin and subcutaneous diseases, especially India ( Figure 6 ). A significant negative correlation was observed between psoriasis and acne vulgaris. The burden of skin and subcutaneous diseases is increasing globally. Thus, targeted and effective management strategies are required globally based on the latest distribution characteristics of each country or territory and focused efforts to reduce the burden of skin and subcutaneous diseases are required.

Skin and subcutaneous disease burden in 2019. Distribution of new cases of each skin and subcutaneous disease at the global level, showing different SDI levels, different GBD regions (21), and 204 countries/territories in 2019. GBD, Global Burden of Disease; SDI, sociodemographic index.
Data availability statement
Ethics statement.
Requirement for ethical approval was waived by the Ethics Committee of Ruijin Hospital, Shanghai JiaoTong University School of Medicine because the results of GBD 2019 study are available in a publicly available database, and all data were anonymous. All methods were performed according to the relevant guidelines and regulations.
Author contributions
AY and RA analyzed and visualized the data. AY, RA, JD, and SL were the major contributors to writing the manuscript. All the authors have read and approved the final version of the manuscript.
Acknowledgments
The authors would like to thank the individuals who contributed to the GBD database.
Funding Statement
This study was supported by the National Natural Science Foundation of China, Grant/Award Numbers: 81671916.
Conflict of interest
The authors declare that the research was conducted in the absence of any commercial or financial relationships that could be construed as a potential conflict of interest.
Publisher's note
All claims expressed in this article are solely those of the authors and do not necessarily represent those of their affiliated organizations, or those of the publisher, the editors and the reviewers. Any product that may be evaluated in this article, or claim that may be made by its manufacturer, is not guaranteed or endorsed by the publisher.
Supplementary material
The Supplementary Material for this article can be found online at: https://www.frontiersin.org/articles/10.3389/fpubh.2023.1145513/full#supplementary-material
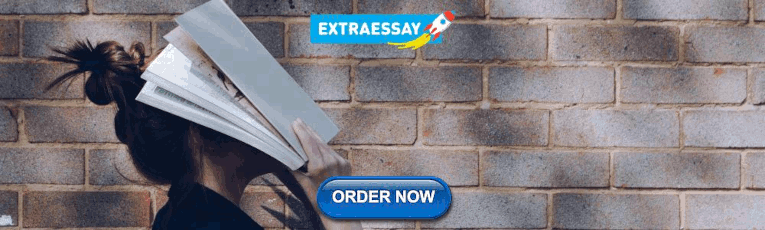
IMAGES
VIDEO
COMMENTS
Acne is projected to affect 9.4 % of the global population, ranking it eighth among skin diseases. Acne affects more than 85 % of teenagers, ... It is noteworthy that the number of research papers and scientific findings relevant to acne treatment regimens continues to increase, given the enormous interest in various research breakthroughs in ...
Chemical peels target acne and offer additional benefits such as refining skin texture and tone, reducing fine lines and wrinkles, and enhancing overall skin health. The selection of peel depth-whether superficial, medium, or deep-depends on factors including skin type, acne history, and desired outcomes . Chemical peels are a comprehensive ...
Recent advances in acne pathogenesis. Atrophy of the sebaceous gland is induced by signal transduction due to various factors. In the hair cycle, anagen in normal skin changes to telogen or catagen in the "comedo switch". Keratin (K) 75 and K79, which are present in the companion layer of normal hair follicles, are reduced in comedo.
The Global Burden of Disease Study 2010 found that acne vulgaris (henceforth acne) is the eight most common skin disease, with an estimated global prevalence (for all ages) of 9.38% 1.In different ...
Acne vulgaris is a common skin condition affecting 9.4% of the global population in 2010, representing the eighth most prevalent disease globally. 9 Acne affects approximately 85% of teenagers but can occur in most age groups and can persist into adulthood. 10 The burden of acne, as measured by disability-adjusted life years, ranked second ...
Acne is a common, complex, multifactorial inflammatory skin disease associated with epidermal barrier dysfunction. ... but further research is required. In acne-affected ... Selected articles included guidelines, consensus papers, reviews describing current best practices in acne treatment using acne products and skincare, and clinical research ...
Acne vulgaris was the eighth most prevalent disease globally in 2010, whereas fungal skin diseases was fourth in global prevalence, and other skin and subcutaneous diseases was in fifth place 2 ...
Importance: Acne vulgaris is an inflammatory disease of the pilosebaceous unit of the skin that primarily involves the face and trunk and affects approximately 9% of the population worldwide (approximately 85% of individuals aged 12-24 years, and approximately 50% of patients aged 20-29 years). Acne vulgaris can cause permanent physical scarring, negatively affect quality of life and self ...
Acne is a chronic inflammatory skin disease that primarily affects adolescents and is attributed to various factors, including hormonal changes, genetic predisposition, and environmental influences. It typically manifests in areas rich in sebaceous glands such as the face, chest, and back. Symptoms of acne can range from mild to severe and may ...
The Global Burden of Disease Project estimates the prevalence of acne at 9·4%, ranking it as the eighth most prevalent disease worldwide. 1, 2 This initiative established prevalence and disability data from primary research manuscripts and databases, and conducted subsequent data analysis with a Bayesian meta-regression methodology, providing ...
Acne vulgaris is a polymorphic skin disease with various clinical phenotypes (e.g., papules, pustules, nodules, cysts, scarring, and psychological sequelae)1. Adolescence, the period when acne first occurs, is a period of socialization, developmental issues with body image, and sexual maturation, which can affect emotional
Anti-Bacterial Agents. Acne is a common, chronic inflammatory condition affecting millions of people worldwide, with significant negative impact on quality of life and mental health. Acne is characterized by comedones, inflammatory papules, pustules, and nodulocystic lesions, with long-lasting sequelae including scarring ….
Acne vulgaris is a common skin disease 1 that affects almost all teenagers and many adults to a degree. It is estimated as the eighth most prevalent global disease, with 650 million people ...
Abstract. This review focuses on the treatment options for adult female patients with acne. Acne in adult female patients may start during adolescence and persist or have an onset in adulthood. Acne has various psychosocial effects that impact patients' quality of life. Treatment of acne in adult women specifically has its challenges due to ...
Discover the world's research. 25+ million members; ... Disease study. Acne vulgaris was the eighth most prevalent disease globally in 2010, whereas fungal skin diseases was fourth in global ...
Methods Papers were identified through Medline, EMBASE, PubMed, PsychINFO and CINAHL on 05 November 2019, forward and backward citation searching, Google Scholar and contacting authors. Inclusion criteria were studies reporting qualitative data and analysis, studies carried out among people with acne, their carers or HCPs and studies comprising different skin conditions, including acne.
Acne vulgaris or simply known as acne is a. human skin disease characterized by skin with. scaly red skin (seborrhea), blackheads and. whiteheads (comedones), pinheads (papules), large papules ...
The relationship between diet and acne has been a hot topic in the research of acne epidemiology. ... the skin barrier is damaged, the skin's defense system against external stimuli is weakened, which can further lead to skin diseases, especially acne ... The core merit of our paper lies in a thorough review of the relevant socio-economic ...
Acne-prone skin is a common condition in adult women, and skin imperfections could affect quality of life and self-esteem. This study aimed to investigate the efficacy of a cosmetic combination regimen for face care (a cleanser gel and a serum containing niacinamide, retinol, and alpha hydroxy acids). A total of 20 women with acne-prone mixed or oily skin were enrolled in a prospective 42-day ...
This review concentrates on the skin and gut microbes in acne, the role that the gut-brain-skin axis plays in the immunobiology of acne, and newly emerging microbiome-based therapies that can be applied to treat acne. Keywords: acne, microbiota, microbiome, skin, gut, brain, therapeutic implications. Go to: 1. Introduction.
Acne is a chronic skin disease whose characteristics and mechanisms are very complex. ... the skin detection method in this paper based on skin-and-non-skin binary-classifier is more effective and ...
Even though many research papers are done on skin disease detection and classification, there is still a gap to be filled. ... (KNN) classification method to classify skin diseases (Acne, Papillomas, Psoriasis, Melanoma, Mycosis, Vitiligo, Warts) that achieved 91.80% accuracy for HSV Color Phase Model and 81.60% accuracy for LAB Color Phase ...
Skin conditions rank as the fourth most prevalent category of human illnesses, impacting nearly a third of the global population Flohr and Hay [].In recent years, there has been a growing interest in leveraging advanced technologies for the analysis of skin images to address the challenges associated with traditional diagnostic methods Haenssle et al. [39, 40] Maron et al. [].
Acne is a chronic, inflammatory disease of the pilosebaceous unit estimated to affect 9.4% of ... inflammatory lesions (papules and pustules), and seborrhea (oily skin). Typically, acne starts in early puberty and is a chronic condition that ... Much of the previous research into the impact of acne on HRQL has used validated instruments such as ...
People with darker skin types are more likely than are people with lighter skin to experience these acne complications: Scars. Pitted skin (acne scars) and thick scars (keloids) can remain long-term after acne has healed. Skin changes. After acne has cleared, the affected skin may be darker (hyperpigmented) or lighter (hypopigmented) than ...
Methods. Data on the skin and subcutaneous diseases were obtained from the Global Burden of Disease Study 2019. The incidence, disability-adjusted life years (DALYs), and deaths due to skin and subcutaneous diseases in 204 countries and regions from 1990 to 2019 were analyzed and stratified by sex, age, geographical location, and sociodemographic index (SDI).